综述:婴儿哭泣自动分析与分类分析
文章目录
- 综述:婴儿哭泣自动分析与分类分析
-
- 摘要
- 1.介绍
-
- 1.1方法
- 1.2婴儿哭声自动分类
- 1.3 婴儿哭声数据库
- 1.4信号处理
- 1.5 预处理
- 1.6特征提取
- 1.7提取的特征的后处理
- 1.8分类法
- 2结论
- 参考文献
摘要
自动婴儿哭声分类在生物工程里是一个至关重要的研究领域,它采用医学和工程技术分析婴儿哭声的信号来区分真实状态。这里有大量的研究在这个领域,扩展了婴儿哭声分析的潜在应用。但是目前来看,还没有一个很好的文章来总结现在该领域的研究状况以及强调婴儿哭声分类的研究趋势。这篇综述使用“婴儿哭声”和“自动分类”来搜索目前在线的资源,并为了做出来一个综合性的综述而忽略出版的时间。总体来看,我们搜集到了300篇论文,在经过一些整理去除之后101篇论文被选中。这篇论文尽力去阐述了婴儿哭声分析与分类的近期发展和优势,尤其集中在婴儿哭声数据集、信号处理和识别。最后,这篇论文还完成了一些可能的应用,这些应用可能会领导自动哭声分类的实际应用发展。
关键字: 婴儿哭声,自动分类,信号处理,分类模型
1.介绍
人类的健康和生活质量随着应用在逃避疾病攻击、减少疾病发生和限制影响上的医药科学和健康及科技提高而被满足。然而婴儿的死亡率和发病率仍然保持较高并且下降缓慢。根据数据统计,每年290万婴儿死亡,但是第一个月死亡的人数占前五年死亡的人数的44%。图1阐述了造成年龄在五岁以下婴儿死亡的原因百分比。新生婴儿(小于28天)死亡人数占五岁以下婴儿死亡人数的43%并且75%死亡是发生在第一周,25-45%发生在24小时内。从图1可以看出来多数婴儿正在遭受不同病理的折磨。根据世界健康组织预测如果在第一周及时进行治疗和诊断的话,三分之二的新生婴儿死亡可以被阻止。[1,2]
因此, 为了降低死亡率和发病率,通过自动的工具研究婴儿的哭声具有显著的影响,这种工具可以极大的帮助人们在早期婴儿状态分析上的研究。自动婴儿分类是一个巨大的研究领域,并且他可以根据之前的相关工作分为三个大子类:(1)识别新生儿某些病理状态的影响,即听力[3-15]残疾婴儿,婴儿猝死综合症[16],腭裂[17],脑损伤[19],窒息[11-15,
20- 29],高胆红素血症[30,31],孤独症[32,33],下丘脑-类固醇[34- 39],cri du chat[40],呼吸窘迫综合征(RSD)[18],会厌偏离的舌系带喉(ADEL) [41],染色体异常,内部-子宫发育迟缓,脑膜炎,舌系带,腹膜-tis,牛蛋白过敏,心脏缺陷[42- 45]和神经病学紊乱[46],(2)对婴儿身份的承认[47,48]和(3)新手身体情绪的分类,即生气,恐惧,不适,疼痛和饥饿[11-13,49-56]。
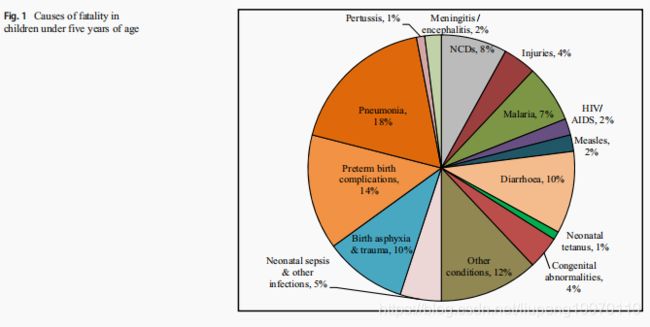
图1 五岁以下婴儿死亡原因
在自动化婴儿啼哭分类的发展中系统,重大的研究工作使用各种婴儿啼哭信号实施各种重要的信号处理方法和模式识别模型,有希望诊断结果。此外,重要的审议工作婴儿啼哭信号的声学特征还通过调查婴儿啼哭的质量和数量[31,56-65],到目前为止,还没有详细讨论婴儿啼哭数据库、信号处理和自动婴儿分类技术应用在哭泣识别文章。因此,目前的研究集中在前面提到的方面。
1.1方法
使用关键词进行文献综述“婴儿啼哭”和“自动分类”不同在线资源,即Springer、IEEE、Elsevier PubMed和ACM数字图书馆数据库。应用包含和排除标准如所列。
包含:
婴儿啼哭自动分类开始于20世纪60年代,论文限制发表年份。
研究论文包括各种婴儿哭声分类,例如痛苦状态察觉,现实心情分析和根据声音特征进行婴儿身份识别。
不包括:
综述性论文除外
一些包含小孩教育和发展、小孩照顾、婴儿哭声、父母监护的研究。
询的标准,我们搜集到了超过300份相关的资料。然后从我们发现其中大约200份论文是尤其关注婴儿哭声的自动分类。还有论文中有一些资料是重复的,因此我们从中再次挑选了104份作为研究的资料。最后这104篇论文中有3份是总数,所以只有101份论文被选中了。
图2 研究方法
1.2婴儿哭声自动分类
总的来说自动婴儿分类处理是一个模式识别的问题。它类似于声音识别处理,它包含信号处理和模式识别两个主要的阶段(图3)[4,5]。自动识别系统接受到原生的新生婴儿哭声输入信号,在信号处理阶段,原始的信号需要进行第一步预处理:正则化,清洗,过滤。经过这几个阶段之后光滑的信号可以用合适的特征提取技术进行分析,显著的特征向量也被分离出来。通过使用适当的特征选择或特征约简方法,可以将提取的高维特征缩减到较低维度的空间中。然后将减少的特征向量进行分类阶段,在此阶段将其与计算机必须产生结果的知识进行比较[3,10]。
1.3 婴儿哭声数据库
研究分析的结果在理论上取决于数据库的质量和一致性,并且数据库的设计对于术语识别任务也至关重要。因此,这是开发可靠的婴儿自动哭声识别器应考虑的基本方面之一。下一节简要概述了迄今为止在婴儿啼哭识别分析中已实施的数据库,并提出了提出的想法,以后可以给予更多的关注。
在婴儿哭声分类中,基于婴儿哭声的数据库并不多,并且大多数已开发的数据库都不可供公众使用。表1列出了在不同情况下开发的可用婴儿哭泣数据库的特征。在这些当中。该公司通常被称为“ Baby Chilanto”数据库[10],是各个研究社区中最常用的数据库,但是它也不是可自由评估以供公众使用的。从表1中可以看出,这些数据库是经过设计的,具有不同的特征,如受试者的身材,哭泣类型,哭泣的性质,哭泣信号的样本,记录的信号的长度,受累婴儿的年龄,记录的距离等,以提出统一的数据收集协议,应当研究和优化依赖的特征以开发数据集的基准化标准。
此外,还观察到激发和自发的哭声信号都是研究人员的选择,但是,在开发高度可靠的婴儿哭声数据库时,通常会鼓励由于某些自然动机而产生的自发性哭声,而不是仅在一次录音中记录的刺激哭声语境。在这种情况下,重要的是要宣布饥饿感是最大的自然哭泣含量,它是由饥饿引起的,可以通过进食合理地确保[60]。同样,婴儿的年龄是另一个决定因素,应该在绩效数据库的开发中加以考虑。有人断言,由于在整个生命过程中哭泣产生结构的生理和解剖结构发生了积极变化,因此1-2岁左右的新生婴儿的声音信号更适合早期诊断各种新生婴儿病理。
另外,关注这个可以解决该领域缺少统一的权威研究数据问题,发展可靠的婴儿哭声分类系统。我们需要合作建立一个真实的婴儿哭声系统给医院使用。
1.4信号处理
信号处理是自动婴儿啼哭识别中必不可少的部分。如上图(图2)所示,在模式分类之前,将通过三个主要步骤处理哭声信号,以生成干净,增强,突出和缩小的特征向量:预处理,特征提取和后处理[67, 68,80]。以下几节简要强调了婴儿哭声分类领域中使用的信号处理技术。
1.5 预处理
在提取特征之前,对记录的粗略哭声信号的时间样本执行的所有操作都被定义为预处理方法。通过消除或分割无声区间来进行哭声单元检测是婴儿哭声分类分析中众所周知的预处理技术之一,因为无声区间通常携带的重要信息较少,反而导致了计算成本。哭声单位被定义为仅在到期时发声的持续时间[76]。婴儿啼哭分析中使用的一些啼哭单元检测技术包括:信噪比测量[9,81,82],平均过零率(ZCR),平均过零率与短时能量的结合[34],能量阈值[18、65]和自相关函数[74]。
此外,通常为了消除不希望的和无关的信息,即背景噪声,回声,信道失真和其他特定信号的特性,通常使用截止频率为250 Hz的高通滤波器,因为在频域[9]中婴儿的哭声不小于250–300 Hz。总体而言,经过仔细检查后,所使用的预处理技术会根据录制环境中使用的哭声信号采集技术和仪器而有所不同。因此没有关于不同的预处理方法如何相互评分的明确标准或有效性度量。
1.6特征提取
对预处理的哭声信号执行特征提取,以提取显著特征以表征哭声的不同模式。在特征提取中,用于特征提取的分析区域是考虑的关键主题之一。根据分析区域的概念提取的特征可以分为两种:从哭声信号的短帧间隔中提取的局部特征和从整个哭声中提取的全局特征。由于哭声信号的高度非平稳性,据报道,与整体特征相比,局部特征在婴儿哭声分类系统的设计中起着重要作用[18]。婴儿哭泣分类分析中提取和展示的特征分为三个引物组,如时域,频域和时频域。
时域特征由于其在低噪声环境中的良好分类性能以及与分别在频域和时频域中的其他特征相比较低的计算复杂性而已广泛用于新生儿哭泣分类中。线性预测系数(LPC)和梅尔频率倒谱系数(MFCC)是常见的且众所周知的声学特征,研究人员在婴儿啼哭分类分析中一直在寻求这些特征,以获取多信息新生儿啼哭信号的性质。 [3-6、10-12、20-24]。还可以观察到,由于MFCC和LPC属于局部特征类别,涉及从不同短帧中提取窗口信号进行提取,因此提取的特征数量相对较高。由于计算量大,在婴儿哭声分析中采用了不同的特征减少和选择技术,以减少多余的哭声特征,尤其是主成分分析(PCA)。关于PCA以及其他降维方法的详细信息可以在以下部分中进行回顾。除上述属性外,还提出了其他时域功能来区分文献中的不同语言。大多数研究已经对婴儿哭泣的持续时间进行了调查和研究,以进行基于时域的分析[16,66,83]。但是,据报道,在大多数情况下,为达到良好的区分目的,在不同的婴儿哭声信号的持续时间之间未观察到显着差异[83]。
尽管基于时域的特征在婴儿啼哭分析中发挥了重要作用,但是基于时域的分析的主要缺点尤其是频域信息的丢失是无法避免的。除了时域特征外,还已知频率或频谱域特征可用于表示不同的婴儿哭声。
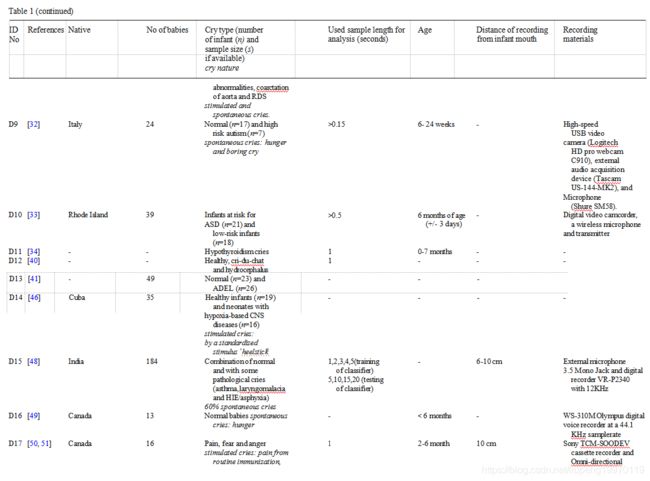
表1 :婴儿哭声数据库
1.7提取的特征的后处理
后处理方法通常被应用对所提取的特征哭声进行处理,然后在分类阶段中使用,以减少分类器的存储和计算要求。特征选择和特征降维的两种方法。
特征减少是变换的数据集到一个较小的,更紧凑的功能集的原始特征,同时保留尽可能多的信息可能的技术。所述PCA特征提取方法已在婴儿哭声识别的上下文中广泛使用[4-7,11-14]。据查明,PCA提供分类效果在整个功能集的改善。但是,占该PCA需要更多的处理时间和计算资源,以确定必须保持其更大的分类准确度贡献的相关信息元件的数量。因此,在[85],通统计操作快速和简单的还原数据即最小值,最大值,平均值,标准偏差和方差介绍 - duced和所提出的技术的有效性是通过应用对所提取的特征向量证明。在另一项研究中,为了减少计算能力,则个MFCC的算术平均值沿着一声信号的原始MFCC矩阵的时间轴计算[49]。
特征选择是最常用的降维技术之一,它与查找原始特征的最小子集有关,该子集可优化一个或多个标准并在类之间实现最佳分类。在婴儿哭泣分类分析中,特征子集的分类能力通常由易于计算的功能或算法(称为特征选择标准)来表征。
费舍尔比率通过方差分析实现,以选择哭声数据[21、34、35]。婴儿的重要哭声特征已使用名为二进制粒子群优化(BPSO)的算法进行了选择,该算法是原始PSO算法的改进形式[24,38]。如[21,22]中所述,一种名为正交最小二乘(OLS)的方法通过估计每个滞后特征对减少误差(ERR)的贡献而起作用,这在婴儿哭泣特征选择中得到了证明。
特征之间的信息增益和相关值用于在二元情况下选择最佳子集特征[12]。在[40]中,提出了一种受交叉验证启发的增强型前向特征方法(FSM)。
总体而言,观察到在降维任务中PCA方法的应用广泛。典型的特征选择方法的用法在此分析领域中是自定义的。
探索其他不同的高级特征选择方法可以增强对哭声信号的统计数据处理。
1.8分类法
不能否认智能识别器在自动婴儿啼哭识别分析中的作用。各种类型的分类器已用于婴儿哭泣识别的任务,例如人工神经网络(ANN),混合网络,统计分类器和其他具有各自预测结果的模型[87-100]。
实际上,关于哪种分类器最适合婴儿哭泣分类还没有达成共识。似乎每个分类器都有其自身的优点和局限性。总体而言,不同的分类模型旨在分类正常和疾病发展的哭声信号。然而,隐含地观察到,先驱者主要集中于将使用包含哭声特征的训练数据进行训练的监督学习算法模型,对于该哭泣特征,分类结果是已知的,随后使用结果未知的数据进行测试。使用这种模态的分类结果通常是有希望的,但是由于研究人员根据模态适用性对训练数据集的使用不同,因此尚无共识。无监督模态的应用可以导致进行系统的研究分析,从而可以比较和确定最佳分类模型。
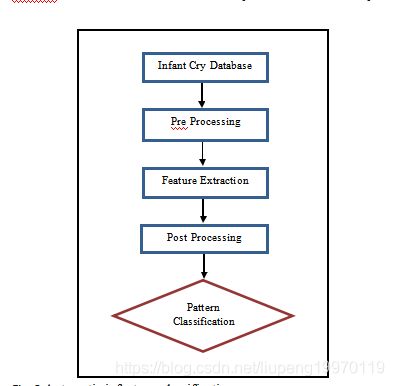
图三:婴儿哭声分类方法
2结论
迄今为止,以斯堪的纳维亚的经验为起点,婴儿哭声分类分析仍在迅速兴起,目的是为多学科应用开发基于健壮的哭声智能系统。本文提供了婴儿哭声分类分析的详细综述,该分析结合了哭声数据库,用于表示哭声信号不同性质的信号处理方法,模式分类技术以及哭声信号的重要应用。在开发可靠的自动分类系统时,可以考虑几种可能的建议。
广义上讲,对于自动分类系统的发展,人们对婴儿的身体特征没有更好的认识。在自动婴儿哭声分类中,应强调婴儿的许多身体特征,即性别,年龄,大小和原住民或血统,并将其纳入分析。
这是因为这些特征可能掩盖了哭声的独创性。根据医学理论,在音调,旋律形状和基本频率方面,男女婴儿哭声的声音含量存在显着差异[62]。在婴儿啼哭分类分析中,年龄差异也被报告为影响因素之一,因为在整个早期的几个月中,啼哭产生结构的生理和解剖结构的改变可能导致啼哭信号的分化[17、18、58、61]。应考虑婴儿的大小或体重指数(包括体重和身高),因为哭声与婴儿的大小显着相关[64]。此外,在基于哭声的分类系统的开发中应注意婴儿的本性或独创性,因为不同的方言可能会影响哭声信号的独创性[69]。
另外,已经使用了诸如婴儿面部表情之类的非听觉情感暗示,并且在区分不同的期望模式方面足智多谋。因此,必须明确指出,在增强识别系统的开发中,结合声学关联对其他信息源的处理是非常重要的。多模态系统的存在可能为更精确和改进的声明做出重要贡献。
研究界主要参与二进制方式分类。迄今为止,在婴儿啼哭分类分析中,N向分类和对无序啼哭信号分析的严重性级别(轻度,中度和重度)的筛选仍然是一项艰巨的任务。通常,轻度失调的哭泣所携带的内容或信息几乎与正常的哭泣信号相似,这通常会使医务人员更难区分哭声。因此,应尝试将严重性类型与正常的哭声进行分类,以调查正常和某些严重程度不同的无序哭泣信号之间的关系和相关性。此外,应该更多地对多类问题进行分类,以研究不同类型的哭声之间的关联和联系。我们相信,在上述建议中将进一步探索研究方向,以在婴儿啼哭识别领域中引起显着影响。
参考文献
- UNICEF, WHO. The World Bank, United Nations. Levels and trends in child mortality: Report 2013. New York: UNICEF; 2013. www.childinfo.org/files/Child_Mortality_Report_2013.pdf Accessed 9 June 2012.
- WHO. Every newborn: An action plan to end preventable deaths. www.who.int/pmnch/media/events/2013/paper_item_7.pdf Accessed 9 June 2012.
- Orozco J, Garcia C. Detecting pathologies from infant cry apply- ing scaled conjugate gradient neural networks. In: Proceedings of the European Symposium on Artificial Neural Networks, Bruges (Belgium), 2003: 349–354.
- Garcia JO, Reyes García CA. A study on the recognition of pat- terns of infant cry for the identification of deafness in just born babies with neural networks. Lect Notes Comput Sci. 2003;2905: 342–9.
- Garcia JO, Reyes García CA. Acoustic features analysis for rec- ognition of normal and hypoacoustic infant cry based on neural networks. Artificial Neural Nets Problem Solving Methods. 2003;2687:615–22.
- Garcia JO, Reyes Garcia CA. Mel frequency cepstrum coefficients extraction from infant cry for classification of normal and patho- logical cry with feed-forward neural networks. In: Proceedings of the International Joint Conference on Neural Networks, 2003: 3140–3145.
- Varallyay Jr G, Benyo Z, Illenyi A, Farkas Z, Kovacs L. Acoustic analysis of the infant cry: classical and new methods. In: Proceedings of the 26th Annual International Conference of the IEEE EMBS, San Francisco, CA, USA, 2004: 313–316.
- Barajas SE, Reyes CA. Your fuzzy relational neural network pa- rameters optimization with a genetic algorithm. In: Proceedings of the 2005 IEEE International Conference on Fuzzy Systems, 2005: 684–689.
- Jam M, Sadjedi H. Identification of hearing disorder by multi- band entropy cepstrum extraction from infant’s cry. In: Proceedings of the 2009 IEEE International Conference on Biomedical and Pharmaceutical Engineering, 2009: pp 1–5.
- Reyes-Galaviz OF, Cano-Ortiz S, Reyes-Garcia C. Evolutionary- neural system to classify infant cry units for pathologies identifi- cation in recently born babies. In: Proceedings of the 8th Mexican International Conference on Artificial Intelligence (MICAI-2009), Guanajuato, Mexico, 2009: 330–335.
- Rosales-Perez A, Reyes-Garcia CA, Gomez-Gil P. Genetic fuzzy relational neural network for infant cry classification. Lect Notes Comput Sci. 2011;6718:288–96.
- Rosales-Perez A, Reyes-Garcia CA, Gonzalez JA, Arch-Tirado E. Infant cry classification using genetic selection of a fuzzy model. Lect Notes Comput Sci. 2012;7441:212–9.
- Hariharan M, Sindhu R, Yaacob S. Normal and hypoacoustic in- fant cry signal classification using time-frequency analysis and general regression neural network. Comput Methods Prog Biomed. 2012;108:559–69.
- Saraswathy J, Hariharan M, Vijean V, Yaacob S, Khairunizam W. Performance comparison of daubechies wavelet family in infant cry classification. In: Proceedings of the 2012 IEEE 8th International Colloquium on Signal Processing and its Applications, 2012: 451–455.
- Saraswathy J, Hariharan M, Nadarajaw T, Khairunizam W, Yaacob S. Optimal selection of mother wavelet for accurate infant cry classification. Australas Phys Eng Sci Med. 2014;37:439–56.
- Robb MP, Crowell DH, Dunn-Rankin P. Sudden infant death syn- drome: cry characteristics. Int J Pediatr Otorhinolaryngol. 2013;77:1263–7.
- Lederman D, Cohen A, Zmora E, Wermke K, Hauschildt S, Stellzig-Eisenhauer A. On the use of hidden markov models in infants’ cry classification. In: Proceedings of the 22nd Convention in Electrical and Electronic Engineers in Israel, 2002: 350–352.
- Lederman D, Cohen A, Zmora E, Wermke K, Hauschildt S, Stellzig-Eisenhauer A. Classification of cries of infants with cleft-palate using parallel hidden markov models. Med Biol Eng Comput. 2008;46:965–75.
- Boukydis CFZ, Lester BM. Infant crying. Plenum press, 1995.
- Zabidi A, Mansor W, Khuan LY, Yassin IM, Sahak R. The effect of F-ratio in the classification of asphyxiated infant cries using multilayer perceptron neural network. In: Proceedings of the IEEE EMBS conference on biomedical engineering & sciences, Kuala Lumpur, Malaysia, 30th Nov-2nd Dec 2010, 2010: 126– 129.
- Zabidi, Mansor W, Khuan LY, Yassin IM, Sahak R. Classification of infant cries with asphyxia using multilayer perceptron neural network. In: Proceedings of the 2nd International Conference on Computer Engineering and Applications, IEEE, 2010: 204–208.
- Sahak R, Mansor W, Lee YK, Yassin IM, Zabidi A. Orthogonal least square based support vector machine for the classification of infant cry with asphyxia. In: Proceedings of the 3rd international conference on biomedical engineering and informatics, IEEE, 2010: 986–990.
- Sahak R, Mansor W, Lee YK, Yassin IM, Zabidi A. Performance of Combined Support Vector Machine and Principal Component Analysis in Recognizing Infant Cry with Asphyxia. In: Proceedings of the 32nd Annual International Conference of the IEEE EMBS Buenos Aires, 2010: 6292–6295.
- Zabidi A, Mansor W, Khuan LY, Yassin IM, Sahak R. Binary particle swarm optimization for selection of features in the recog- nition of infants cries with asphyxia. In: Proceedings of the 7th International Colloquium on signal processing & its applications, 2011: 272–276.
- Hariharan M, Yaacob S, Awang SA. Pathological infant cry anal- ysis using wavelet packet transform and probabilistic neural net- work. Exp Syst Appl. 2011;12:15377–82.
- Sahak R, Lee YK, Mansor W, Yassin IM, Zabidi A. Optimal features for classifying asphyxiated infant cry using support vector machine with RBF kernel. In: Proceedings of the 5th European IFMBE Conference, 2011: 98–101.
- Mohd Ali MZ, Mansor W, Khuan LY, Zabidi A. Simulink model of Mel frequency cepstral coefficient analysis for extracting as- phyxiated infant cry features. In: Proceedings of the 2012 International Conference on Biomedical Engineering (ICoBE), 2012: 475–477.
- Mohd Ali MZ, Mansor W, Khuan LY, Zabidi A. Asphyxiated infant cry classification using simulink model. In: Proceedings of the 2012 IEEE 8th International Colloquium on Signal Processing and its Applications, 2012; 491–494.
- Hariharan M, Saraswathy J, Sindhu R, Khairunizam W, Yaacob S. Infant cry classification to identify asphyxia using time-frequency analysis and radial basis neural networks. Exp Syst Appl. 2012;39: 9515–23.
- Santiago-Sanchez K, Reyes-Garcia CA, Gomez-Gil P. Type-2 fuzzy sets applied to pattern matching for the classification of cries of infants under neurological risk. Lect Notes Comput Sci. 2009;5754:201–10.
- Kheddache Y, Tadj C. Acoustic measures of the cry characteristics of healthy newborns and newborns with pathologies. J Biomed Sci Eng. 2013;6:796–804.
- Orlandi S, Manfredi C, Bocchi1 L, Scattoni ML. Automatic new- born cry analysis: a non-invasive tool to help autism early diag- nosis. In: Proceedings of the 34th Annual International Conference of the IEEE EMBS San Diego, California USA, 2012: 2953–2956.
- Sheinkopf SJ, Iverson JM, Rinaldi ML, Lester BM. A typical cry acoustics in 6-month-old infants at risk for autism spectrum disor- der. INSAR Research Article, Autism Research, 2012:1–9.
- Zabidi A, Mansor W, Khuan LY, Sahak R, Rahman YA. Mel- frequency cepstrum coefficient analysis of infant cry with hypo- thyroidism. In: Proceedings of the5th International Colloquium on signal processing & its applications, 2009: 204–208.
- Zabidi A, Mansor W, Khuan LY, Yassin IM, Sahak R. Classification of infant cries with hypothyroidism using multilay- er perceptron neural network. In: Proceedings of the 2009 IEEE International Conference on Signal and Image Processing Applications, 2009; 246–251.
- Zabidi A, Khuan LY, Mansor W, Yassin IM, Sahak R. Detection of infant hypothyroidism with mel frequency cepstrum analysis and multi-layerperceptron classification. In: Proceedings of the 6th International Colloquium on Signal Processing & Its Applications, 2010; 140–144.
- Zabidi A, Mansor W, Lee Yoot Khuan, Yassin IM, Sahak R. Investigation of mel frequency cepstrum coefficients parameters for classification of infant cries with hypothyroidism using MLP classifier. In: Proceedings of the 2010 International Joint Conference on Neural Networks, 2010: 1–4.
- Zabidi A, Mansor W, Khuan LY, Yassin IM, Sahak R. Optimization of MFCC parameters using particle swarm optimi- zation for diagnosis of infant hypothyroidism using multi-layer perceptron. In: Proceedings of the 32nd Annual International Conference of the IEEE EMBS Buenos Aires, 2010: 1417–1420.
- Zabidi A, Mansor W, Khuan LY, Yassin IM, Sahak R. Discrete mutative particle swarm optimisation of mfcc computation for classifying hypothyroidal infant cry. In: Proceedings of the 2010 International Conference on Computer Applications and Industrial Electronics, 2010: 588–592.
- Lederman D. Estimation of infant’s cry fundamental frequency using a modified SIFT algorithm. arXiv: 1009.2796v1 [cs. SD], 2010: 1–5.
- Okada Y, Fukuta K, Nagashima T. Iterative forward on cross- validation approach and its application to infant cry classification. In: Proceedings of the International Multi Conference of Engineers and Computer Scientists, 2011.
- Alaie TC. Cry-based classification of healthy and sick infants using adapted boosting mixture learning method for gaussian mix- ture models. Modeling and Simulation in Engineering. 2012;5
- Alaie TC. Spitting of Gaussian models via adapted BML method pertaining to cry-based diagnostic system. Engineering. 2013;5: 277–83.
- Kheddache Y, Tadj C. Newborn’s pathological identification sys- tem. In: Proceedings of the 11th International Conference on Information Science, Signal Processing and their Applications, 2012: 1024–1029.
- Kheddache T. Characterization of pathologic cries of newborns based on fundamental frequency estimation. Engineering. 2013;5:272–6.
- Kheddache T. Resonance frequencies behavior in pathologic cries of newborns. J Voice. 2014;29:1–12.
- Cano S, Suaste I, Escobedo D, Reyes-Garcia CA, Ekkel T. A radial basis function network oriented for infant cry classification. In Progress in Pattern Recognition, Image Analysis and Applications, 2004: 374–380.
- Patil HA. Infant identification from their cry. In: Proceedings of the 7th International Conference on Advances in Pattern Recognition; 2009. p. 107–10.
- Messaound A, Tadj C. A cry-based babies identification system. Lect Notes Comput Sci. 2010;6134:192–9.
- Petroni M, Malowany AS, Johnston CC, Stevens BJ. Classification of infant cry vocalization using artificial neural net- works (ANNs). In: Proceedings of the International Conference on Acoustics, Speech and Signal Processing, 1995: 3475–3478.
- Petroni M, Malowany AS, Johnston CC, Stevens BJ. Identification of pain from infant cry vocalizations using artificial neural networks (ANNs). In: Proceedings of the SPIE’s Symposium on OE/Aerospace Sensing and Dual Use Photonics, 1995: 729–738.
- Barajas-Montiel SE, Reyes-Garcia CA. Identifying pain and hun- ger in infant cry with classifiers ensembles. In: Proceedings of the International Conference on Computational Intelligence for Modeling, Control and Automation, and International Conference on Intelligent Agents, Web Technologies and Internet Commerce, 2005: 770–775.
- Barajas-Montiel SE, Reyes-Garcia CA, Arch-Tirado E, Mandujano M. Improving baby caring with automatic infant cry recognition. Lect Notes Comput Sci. 2006;4061:691–8.
- Abdulaziz Y, Ahmad SM. An accurate infant cry classification system based on continuos hidden markov model. In: Proceedings of the 2010 International Symposium in Information Technology, 2010: 1648–1652.
- Abdulaziz Y, Ahmad SM. Infant cry recognition system: A com- parison of system performance based on Mel frequency and linear prediction cepstral coefficients. In: Proceedings of the International Conference on Information Retrieval & Knowledge Management, 2010: 260–263.
- Vempada RR, Kumar B, Rao KS. Characterization of infant cries using spectral and prosodic features. In: Proceedings of the National Conference in Communications, 2012: 1–5.
- Fort A, Ismaelli A, Manfredi C, Bruscaglioni P. Parametric and non-parametric estimation of speech formants: application to in- fant cry. Med Eng Phys. 1996;18:677–91.
- Lind K, Wermke K. Development of the vocal fundamental fre- quency of spontaneous cries during the first 3 months. Int J Pediatr Otorhinolaryngol. 2002;64:97–104.
- Benyo Z, Farkas Z, Illenyi A, Katona G, Varallyay Jr G. In for- mation transfer of sound signals. A case study: The infant cry. Is it a noise or an information? In: Proceedings of the 22rd International Congress and exposition on noise Control Engineering, 2004: 1–8.
- Manickam K, Li H. Complexity analysis of normal and deaf infant cry acoustic waves. In: Proceedings of the 4th International Workshop. Models and Analysis of Vocal Emissions for Biomedical Applications, 2005: 105–108.
- Baeck HE, de Souza MN. Longitudinal study of the fundamental frequency of hunger cries along the first 6 months of healthy babies. J Voice. 2007;21:551–9.
- Rautava L, Lempinen A, Ojala S, Parkkola R, Rikalainen H, Lapinleimu H, et al. Acoustic quality of cry in very-low-birth- weight infants at the age of 1 1/2 years. Early Hum Dev. 2007;83:5–12.
- Verduzco-Mendoza A, Arch-Tirado E, Reyes-Garcia CA, Leybon-Ibarra J, Licona-Bonilla J. Qualitative and quantitative crying analysis of new born babies delivered under high risk ges- tation. Multimodal Signals: Cognitive and Algorithmic Issues, 2009: 320–327.
- Wermke K, Robb MP. Fundamental frequency of neonatal crying: does body size matter? J Voice. 2010;24:388–94.
- Verduzco-Mendoza A, Arch-Tirado E, Reyes-Garcia CA, Leybon-Ibarra J, Licona-Bonilla J. Spectrographic infant cry anal- ysis in newborns with profound hearing loss and perinatal high- risk newborns. Cir Cir. 2012;80:3–10.
- Varallyay G. Future prospects of the infant cry in the medicine. Periodica Polytechnica SerElEng. 2006;50:47–62.
- Reyes Galaviz OF, Reyes Garcia CA. Infant cry classification to identify hypo acoustics and asphyxia comparing an evolutionary- neural system with a neural network system. In: Proceedings of the MICAI: Advances in Artificial Intelligence, 2005: 949–958.
- Reyes-Galaviz OF, Cano-Ortiz SD, Reyes-Garcia CA. Validation of the cry unit as primary element for cry analysis using an evolutionary-neural approach. In: Proceedings of the Mexican International Conference on Computer Science, 2008: 261–267.
- Mampe B, Friederici AD, Christophe A, Wermke K. Newborns’ cry melody is shaped by their native language. Curr Biol. 2009;19: 1994–7.
- Daga RP, Panditrao AM. Acoustical analysis of pain cries’ in neonates: fundamental frequency. Special Issue of International Journal of Computer Applications on Electronics, Information and Communication Engineering. 2011;3:18–21.
- Baeck HE, Souza MN. Study of acoustic features of newborn cries that correlate with the context. In: Proceedings of the IEEE 23rd Annual Conference in Engineering in Medicine and Biology Society, 2001: 2174–2177.
- Varallyay Jr G, Benyo Z, Illenyi A. The development of the mel- ody of the infant cry to detect disorders during infancy. In: Proceedings of the IASTED International Conference on Biomedical Engineering, 2007; 14–16.
- Chittora A, Patil H. Data collection and corpus design for analysis of normal and pathological infant cry. In: Proceedings of the 2013 International Conference in Oriental COCOSDA held jointly with 2013 Conference on Asian Spoken Language Research and Evaluation, 2013: 1–6.
- Manfredi C, Bocchi L, Orlandi S, Spaccaterra L, Donzelli GP. High-resolution cry analysis in preterm newborn infants. Med Eng Phys. 2009;31:528–32.
- Sahak R, Mansor W, Khuan LY, Zabidi A, Yasmin F. An investi- gation into infant cry and apgar score using principle component analysis. In: Proceedings of the 2009 5th International Colloquium on Signal Processing & Its Applications, 2009: 209–214.
- Wermke K, Mende W, Manfredi C, Bruscaglioni P. Developmental aspects of infant’s cry melody and formants. Med Eng Phys. 2002;24:501–14.
- Mijovic B, Silva M, Van den BRH B, Allegaert K, Aerts JM, Berckmans D, et al. Assessment of pain expression in infant cry signals using empirical mode decomposition. Methods Inf Med. 2010;49:448–52.
- Abou-Abbas L, Montazeri L, Gargour C, Tadj C. On the use of EMD for automatic newborn cry segmentation. International Conference on Advances in Biomedical Engineering (ICABME), 2015, pp. 262–265.
- Chang C-Y, Li J-J. Application of Deep Learning for Recognizing Infant Cries. International Conference on Consumer Electronics- Taiwan. 2016.
- Ruiz Diaz MA, Reyes Garcia CA, Altamirano Robles LC, Xaltena Altamirano JE. Automatic infant cry analysis for the identification of qualitative features to help opportune diagnosis. Biomedical Signal Processing and Control. 2012;7:43–9.
- Michelsson K, Eklund K, Leepanen P, Lyytinen H. Cry character- istics of 172 healthy 1 to 7 day old infants. International Journal of Phoniatrics Speech Therapy and Communication Pathology. 2002;54:190–200.
- Ruiz MA, Reyes CA, Altamirano LC. On the implementation of a method for automatic detection of infant cry units. Procedia Engineering. 2012;35:217–22.
- Varallyay G. Infant cry analyzer system for hearing disorder de- tection. Periodica Politechnica, Transaction on Automatic Control and Computer Science. 2004;49:1–4.
- Fort A, Manfredi C. Acoustic analysis of newborn infant cry sig- nals. Med Eng Phys. 1998;20:432–42.
- Amaro-Camargo E, Reyes Garcia CA. Applying statistical vectors of acoustic characteristics for the automatic classification of infant cry. In Advanced Intelligent Computing Theories and
Applications, With Aspects of Theoretical and Methodological Issues, 2007: 1078–1085.
- Reyes-Galaviz OF, Reyes-Garcia CA. Fuzzy relational compres- sion applied on feature vectors for infant cry recognition. In MICAI 2009: Advances in Artificial Intelligence. 2009;5845: 420–31.
- Jam MM, Sadjedi H. A system for detecting of infants with pain from normal infants based on multi band spectral entropy by in- fant’s cry analysis. In: Proceedings of the Second International Conference on Computer and Electrical Engineering, 2009: 72– 76.
- Hariharan M, Chee LS, Yaacob S. Analysis of infant cry through weighted linear prediction cepstral coefficients and probabilistic neural network. J Med Syst. 2012;36:1309–15.
- Reyes-Galaviz OF, Reyes-Garcia CA. Infant cry classification to identify hypoacoustics and asphyxia with neural networks. In: Proceedings of 2004 MICAI: Advances in Artificial Intelligence, 2004: 69–78.
- Reyes-Galaviz OF, Verduzco A, Arch-Tirado E, Reyes-Garcia CA. Analysis of an infant cry recognizer for the early identifica- tion of pathologies. Nonlinear Speech Modeling and Applications. 2005;3445:404–9.
- Suaste-Rivas I, Reyes-Galviz OF, Diaz-Mendez A, Reyes Garcia CA. A fuzzy relational neural network for pattern classification. In CIARP. 2004;3287:358–65.
- Suaste-Rivas I, Reyes-Galviz OF, Diaz-Mendez A, Reyes Garcia CA. Implementation of a linguistic fuzzy relational neural network for detecting pathologies by infant cry recognition. In IBERAMIA. 2004;3315:953–62.
- Reyes-Galaviz OF, Arch Tirado E, Reyes Garcia CA. Classification of infant crying to identify pathologies in recently born babies with ANFIS. ICCHP Transaction, Lecture Notes in Artificial Intelligence. 2004;3118:408–15.
- Barajas-Montiel SE, Reyes-Garcia CA. Fuzzy support vector ma- chines for automatic infant cry recognition. In: Proceedings of the Intelligent Computing in Signal Processing and Pattern Recognition, 2006: 876–881.
- Suaste-Rivas I, Diaz-Mendez A, Reyes-Garcia C, Reyes-Galaviz
O. hybrid neural network design and implementation on FPGA for infant cry recognition. In Text, Speech and Dialogue, 2006: 703– 709.
- Messaoud A, Tadj C. Analysis of acoustic features of infant cry for classification purposes. In: Proceedings of the 24th Canadian Conference on Electrical and Computer Engineering, 2011: 89– 92.
- Singh AK, Mukhopadhyay J, Rao SK. Classification of infant cries using source, system and supra-segmental feature. In: Proceedings of the 2013 Indian Conference on Medical Informatics and Telemedicine (ICMIT), 2013: pp 58–63.
- Cohen R, Lavner Y. Infant cry analysis and detection. In: Proceedings of the 27th Convention of Electrical and Electronic Engineers, 2012: 1–5.
- Saraswathy J, Hariharan M, Khairunizam W, Yaacob S, Thiyagar
N. Infant Cry Classification: Time Frequency Analysis. In: Proceedings of the 2013 IEEE International Conference on Control System, Computing and Engineering, 2013: 499–504.
- Orlandi S, Reyes Garcia CA, Bandini A, Donzelli G, Manfredi C. Application of pattern recognition techniques to the classification of full-term and preterm infant cry. J Voice. 2015; https://doi.org/ 10.1016/j.jvoice.2015.08.007.