http://www.cybaea.net/Journal/2010/11/16/Feature-selection-Using-the-caret-package/
http://topepo.github.io/caret/recursive-feature-elimination.html
Recursive Feature Elimination
Backwards Selection
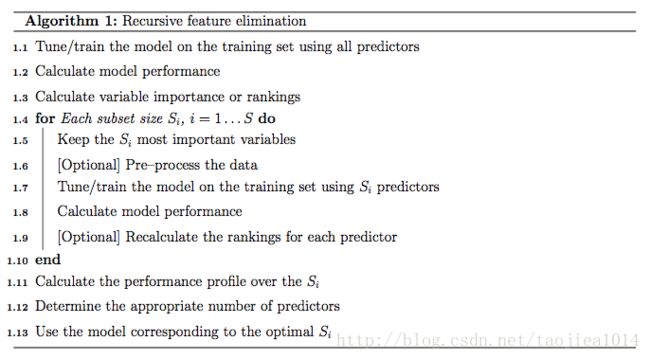
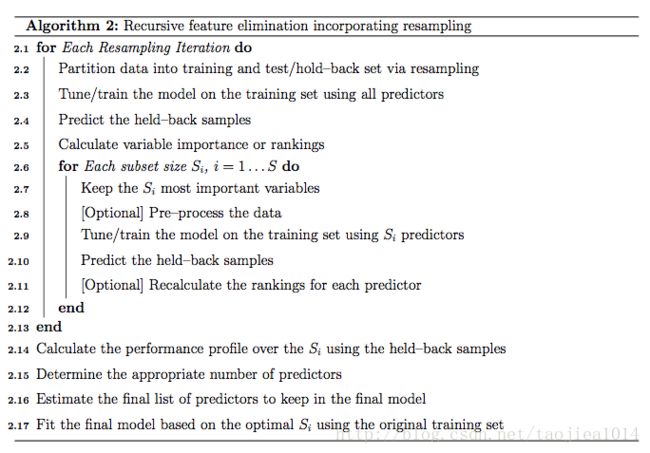
In caret
, Algorithm 1 is implemented by the function rfeIter
. The resampling-based Algorithm 2 is in the rfe
function. Given the potential selection bias issues, this document focuses on rfe
. There are several arguments:
For a specific model, a set of functions must be specified in rfeControl$functions
. Sections below has descriptions of these sub-functions. There are a number of pre-defined sets of functions for several models, including: linear regression (in the object lmFuncs
), random forests (rfFuncs
), naive Bayes (nbFuncs
), bagged trees (treebagFuncs
) and functions that can be used with caret
’s train
function (caretFuncs
). The latter is useful if the model has tuning parameters that must be determined at each iteration.
library("caret")
library("randomForest")
library("ipred")
library("gbm")
install.packages("multicore")
set.seed(1)
n.var <- 20
n.obs <- 200
x <- data.frame(V = matrix(rnorm(n.var*n.obs), n.obs, n.var))
n.dep <- floor(n.var/5)
cat( "Number of dependent variables is", n.dep, "\n")
m <- diag(n.dep:1)
y.1 <- factor( ifelse( x$V.1 >= 0, 'A', 'B' ) )
y.2 <- ifelse( rowSums(as.matrix(x[, 1:n.dep]) %*% m) >= 0, "A", "B" )
y.2 <- factor(y.2)
y.3 <- factor(rowSums(x[, 1:n.dep] >= 0))
y.4 <- factor(rowSums(x[, 1:n.dep] >= 0) %% 2)
control <- rfeControl(functions = rfFuncs, method = "boot", verbose = FALSE,
returnResamp = "final", number = 50)
if ( require("multicore", quietly = TRUE, warn.conflicts = FALSE) ) {
control$workers <- multicore:::detectCores()
control$computeFunction <- mclapply
control$computeArgs <- list(mc.preschedule = FALSE, mc.set.seed = FALSE)
}
sizes <- 1:6
## Use randomForest for prediction
profile.1 <- rfe(x, y.1, sizes = sizes, rfeControl = control)
cat( "rf : Profile 1 predictors:", predictors(profile.1), fill = TRUE )
profile.2 <- rfe(x, y.2, sizes = sizes, rfeControl = control)
cat( "rf : Profile 2 predictors:", predictors(profile.2), fill = TRUE )
profile.3 <- rfe(x, y.3, sizes = sizes, rfeControl = control)
cat( "rf : Profile 3 predictors:", predictors(profile.3), fill = TRUE )
profile.4 <- rfe(x, y.4, sizes = sizes, rfeControl = control)
cat( "rf : Profile 4 predictors:", predictors(profile.4), fill = TRUE )