2.3 处理缺失数据
与本节相关的视频课程:处理缺失数据
检查缺失数据
基础知识
def foo(): pass
f = foo()
print(f)
None
type(f)
NoneType
None + 2
TypeError: unsupported operand type(s) for +: 'NoneType' and 'int'
import numpy as np
np.nan + 2
nan
type(np.nan)
float
import pandas as pd
s = pd.Series([1, 2, None, np.nan])
s
0 1.0
1 2.0
2 NaN
3 NaN
dtype: float64
s.sum()
3.0
s.isna()
0 False
1 False
2 True
3 True
dtype: bool
df = pd.DataFrame({"one":[1, 2, np.nan], "two":[np.nan, 3, 4]})
df.isna()
|
one |
two |
0 |
False |
True |
1 |
False |
False |
2 |
True |
False |
项目案例
hitters = pd.read_csv("/home/aistudio/data/data20507/Hitters.csv")
hitters.isna().any()
AtBat False
Hits False
HmRun False
Runs False
RBI False
Walks False
Years False
CAtBat False
CHits False
CHmRun False
CRuns False
CRBI False
CWalks False
League False
Division False
PutOuts False
Assists False
Errors False
Salary True
NewLeague False
dtype: bool
(hitters.shape[0] - hitters.count()) / hitters.shape[0]
AtBat 0.00000
Hits 0.00000
HmRun 0.00000
Runs 0.00000
RBI 0.00000
Walks 0.00000
Years 0.00000
CAtBat 0.00000
CHits 0.00000
CHmRun 0.00000
CRuns 0.00000
CRBI 0.00000
CWalks 0.00000
League 0.00000
Division 0.00000
PutOuts 0.00000
Assists 0.00000
Errors 0.00000
Salary 0.18323
NewLeague 0.00000
dtype: float64
hitters.shape
(322, 20)
hitters.count()
AtBat 322
Hits 322
HmRun 322
Runs 322
RBI 322
Walks 322
Years 322
CAtBat 322
CHits 322
CHmRun 322
CRuns 322
CRBI 322
CWalks 322
League 322
Division 322
PutOuts 322
Assists 322
Errors 322
Salary 263
NewLeague 322
dtype: int64
(df.shape[1] - df.T.count()) / df.shape[1]
0 0.5
1 0.0
2 0.5
dtype: float64
df.dropna()
df = pd.concat([df, pd.DataFrame({"one": [np.nan], "two": [np.nan], "three": [np.nan]})],
ignore_index=True, sort=False)
df
|
one |
two |
three |
0 |
1.0 |
NaN |
NaN |
1 |
2.0 |
3.0 |
NaN |
2 |
NaN |
4.0 |
NaN |
3 |
NaN |
NaN |
NaN |
df.dropna(axis=0, how='all')
|
one |
two |
three |
0 |
1.0 |
NaN |
NaN |
1 |
2.0 |
3.0 |
NaN |
2 |
NaN |
4.0 |
NaN |
df.dropna(thresh=2)
|
one |
two |
three |
1 |
2.0 |
3.0 |
NaN |
new_hitters = hitters.dropna()
new_hitters.isna().any()
AtBat False
Hits False
HmRun False
Runs False
RBI False
Walks False
Years False
CAtBat False
CHits False
CHmRun False
CRuns False
CRBI False
CWalks False
League False
Division False
PutOuts False
Assists False
Errors False
Salary False
NewLeague False
dtype: bool
动手练习
eles = pd.read_csv("/home/aistudio/data/data20507/elements.csv")
eles.isna().any()
atomic number False
symbol False
name False
atomic mass False
CPK False
electronic configuration False
electronegativity True
atomic radius True
ion radius True
van der Waals radius True
IE-1 True
EA True
standard state True
bonding type True
melting point True
boiling point True
density True
metal False
year discovered False
group False
period False
dtype: bool
(eles.shape[0] - eles.count()) / eles.shape[0]
atomic number 0.000000
symbol 0.000000
name 0.000000
atomic mass 0.000000
CPK 0.000000
electronic configuration 0.000000
electronegativity 0.177966
atomic radius 0.398305
ion radius 0.220339
van der Waals radius 0.677966
IE-1 0.135593
EA 0.279661
standard state 0.161017
bonding type 0.169492
melting point 0.144068
boiling point 0.203390
density 0.186441
metal 0.000000
year discovered 0.000000
group 0.000000
period 0.000000
dtype: float64
eles_nona = eles.dropna()
eles_nona.isna().any()
atomic number False
symbol False
name False
atomic mass False
CPK False
electronic configuration False
electronegativity False
atomic radius False
ion radius False
van der Waals radius False
IE-1 False
EA False
standard state False
bonding type False
melting point False
boiling point False
density False
metal False
year discovered False
group False
period False
dtype: bool
2.3.2 用指定值填补缺失数据
基础知识
df = pd.DataFrame({"one":[10, 11, 12], 'two':[np.nan, 21, 22], "three":[30, np.nan, 33]})
df
|
one |
two |
three |
0 |
10 |
NaN |
30.0 |
1 |
11 |
21.0 |
NaN |
2 |
12 |
22.0 |
33.0 |
df = pd.DataFrame({'ColA':[1, np.nan, np.nan, 4, 5, 6, 7], 'ColB':[1, 1, 1, 1, 2, 2, 2]})
df['ColA'].fillna(method='ffill')
0 1.0
1 1.0
2 1.0
3 4.0
4 5.0
5 6.0
6 7.0
Name: ColA, dtype: float64
df['ColA'].fillna(method='bfill')
0 1.0
1 4.0
2 4.0
3 4.0
4 5.0
5 6.0
6 7.0
Name: ColA, dtype: float64
项目案例
persons = pd.read_csv("/home/aistudio/data/data20507/Person.csv")
pdf = persons.sample(20)
pdf['Height-na'] = np.where(pdf['Height'] % 5 == 0, np.nan, pdf['Height'])
pdf
|
Gender |
Height |
Weight |
Index |
Height-na |
64 |
Male |
175 |
135 |
5 |
NaN |
225 |
Female |
155 |
144 |
5 |
NaN |
484 |
Female |
188 |
115 |
4 |
188.0 |
293 |
Female |
165 |
83 |
4 |
NaN |
102 |
Male |
161 |
155 |
5 |
161.0 |
282 |
Female |
147 |
94 |
5 |
147.0 |
139 |
Male |
159 |
124 |
5 |
159.0 |
66 |
Female |
172 |
96 |
4 |
172.0 |
365 |
Male |
141 |
80 |
5 |
141.0 |
397 |
Male |
169 |
136 |
5 |
169.0 |
18 |
Male |
144 |
145 |
5 |
144.0 |
172 |
Male |
167 |
151 |
5 |
167.0 |
443 |
Male |
152 |
146 |
5 |
152.0 |
358 |
Female |
180 |
58 |
1 |
NaN |
447 |
Female |
176 |
121 |
4 |
176.0 |
251 |
Male |
140 |
143 |
5 |
NaN |
360 |
Female |
193 |
61 |
1 |
193.0 |
346 |
Female |
191 |
68 |
2 |
191.0 |
5 |
Male |
189 |
104 |
3 |
189.0 |
294 |
Female |
168 |
143 |
5 |
168.0 |
pdf['Height-na'].fillna(pdf['Height-na'].mean(), inplace=True)
pdf
|
Gender |
Height |
Weight |
Index |
Height-na |
64 |
Male |
175 |
135 |
5 |
167.8 |
225 |
Female |
155 |
144 |
5 |
167.8 |
484 |
Female |
188 |
115 |
4 |
188.0 |
293 |
Female |
165 |
83 |
4 |
167.8 |
102 |
Male |
161 |
155 |
5 |
161.0 |
282 |
Female |
147 |
94 |
5 |
147.0 |
139 |
Male |
159 |
124 |
5 |
159.0 |
66 |
Female |
172 |
96 |
4 |
172.0 |
365 |
Male |
141 |
80 |
5 |
141.0 |
397 |
Male |
169 |
136 |
5 |
169.0 |
18 |
Male |
144 |
145 |
5 |
144.0 |
172 |
Male |
167 |
151 |
5 |
167.0 |
443 |
Male |
152 |
146 |
5 |
152.0 |
358 |
Female |
180 |
58 |
1 |
167.8 |
447 |
Female |
176 |
121 |
4 |
176.0 |
251 |
Male |
140 |
143 |
5 |
167.8 |
360 |
Female |
193 |
61 |
1 |
193.0 |
346 |
Female |
191 |
68 |
2 |
191.0 |
5 |
Male |
189 |
104 |
3 |
189.0 |
294 |
Female |
168 |
143 |
5 |
168.0 |
pdf['Height'].describe()
count 20.000000
mean 166.600000
std 16.740748
min 140.000000
25% 154.250000
50% 167.500000
75% 177.000000
max 193.000000
Name: Height, dtype: float64
pdf['Height-na'].describe()
count 20.000000
mean 167.800000
std 14.882699
min 141.000000
25% 160.500000
50% 167.800000
75% 173.000000
max 193.000000
Name: Height-na, dtype: float64
扩展研究
pdf2 = persons.sample(20)
pdf2['Height-na'] = np.where(pdf2['Height'] % 5 == 0, np.nan, pdf2['Height'])
from sklearn.impute import SimpleImputer
imp_mean = SimpleImputer(missing_values=np.nan, strategy='mean')
col_values = imp_mean.fit_transform(pdf2['Height-na'].values.reshape((-1, 1)))
col_values
array([[169. ],
[188. ],
[178.33333333],
[178.33333333],
[166. ],
[193. ],
[178.33333333],
[178. ],
[142. ],
[178.33333333],
[197. ],
[178.33333333],
[186. ],
[171. ],
[178. ],
[176. ],
[183. ],
[184. ],
[188. ],
[176. ]])
df = pd.DataFrame({"name": ["Google", "Huawei", "Facebook", "Alibaba"],
"price": [100, -1, -1, 90]
})
df
|
name |
price |
0 |
Google |
100 |
1 |
Huawei |
-1 |
2 |
Facebook |
-1 |
3 |
Alibaba |
90 |
imp = SimpleImputer(missing_values=-1, strategy='constant', fill_value=110)
imp.fit_transform(df['price'].values.reshape((-1, 1)))
array([[100],
[110],
[110],
[ 90]])
2.3.3 根据规律填补缺失值
df = pd.DataFrame({"one":np.random.randint(1, 100, 10),
"two": [2, 4, 6, 8, 10, 12, 14, 16, 18, 20],
"three":[5, 9, 13, np.nan, 21, np.nan, 29, 33, 37, 41]})
df
|
one |
two |
three |
0 |
38 |
2 |
5.0 |
1 |
69 |
4 |
9.0 |
2 |
86 |
6 |
13.0 |
3 |
79 |
8 |
NaN |
4 |
73 |
10 |
21.0 |
5 |
90 |
12 |
NaN |
6 |
77 |
14 |
29.0 |
7 |
31 |
16 |
33.0 |
8 |
66 |
18 |
37.0 |
9 |
22 |
20 |
41.0 |
from sklearn.linear_model import LinearRegression
df_train = df.dropna()
df_test = df[df['three'].isnull()]
regr = LinearRegression()
regr.fit(df_train['two'].values.reshape(-1, 1), df_train['three'].values.reshape(-1, 1))
df_three_pred = regr.predict(df_test['two'].values.reshape(-1, 1))
df.loc[(df.three.isnull()), 'three'] = df_three_pred
df
|
one |
two |
three |
0 |
38 |
2 |
5.0 |
1 |
69 |
4 |
9.0 |
2 |
86 |
6 |
13.0 |
3 |
79 |
8 |
17.0 |
4 |
73 |
10 |
21.0 |
5 |
90 |
12 |
25.0 |
6 |
77 |
14 |
29.0 |
7 |
31 |
16 |
33.0 |
8 |
66 |
18 |
37.0 |
9 |
22 |
20 |
41.0 |
项目案例
import pandas as pd
train_data = pd.read_csv("/home/aistudio/data/data20507/train.csv")
train_data.info()
RangeIndex: 891 entries, 0 to 890
Data columns (total 12 columns):
PassengerId 891 non-null int64
Survived 891 non-null int64
Pclass 891 non-null int64
Name 891 non-null object
Sex 891 non-null object
Age 714 non-null float64
SibSp 891 non-null int64
Parch 891 non-null int64
Ticket 891 non-null object
Fare 891 non-null float64
Cabin 204 non-null object
Embarked 889 non-null object
dtypes: float64(2), int64(5), object(5)
memory usage: 83.6+ KB
train_data.isna().any()
PassengerId False
Survived False
Pclass False
Name False
Sex False
Age True
SibSp False
Parch False
Ticket False
Fare False
Cabin True
Embarked True
dtype: bool
df = train_data[['Age','Fare', 'Parch', 'SibSp', 'Pclass']]
known_age = df[df['Age'].notnull()].values
unknown_age = df[df['Age'].isnull()].values
y = known_age[:, 0]
X = known_age[:, 1:]
from sklearn.ensemble import RandomForestRegressor
rfr = RandomForestRegressor(random_state=0, n_estimators=2000, n_jobs=-1)
rfr.fit(X, y)
pred_age = rfr.predict(unknown_age[:, 1:])
pred_age.mean()
29.438010170664793
train_data.loc[(train_data.Age.isnull()), 'Age'] = pred_age
train_data.isna().any()
PassengerId False
Survived False
Pclass False
Name False
Sex False
Age False
SibSp False
Parch False
Ticket False
Fare False
Cabin True
Embarked True
dtype: bool
!mkdir /home/aistudio/external-libraries
!pip install seaborn -t /home/aistudio/external-libraries
import sys
sys.path.append('/home/aistudio/external-libraries')
%matplotlib inline
import seaborn as sns
sns.distplot(y)
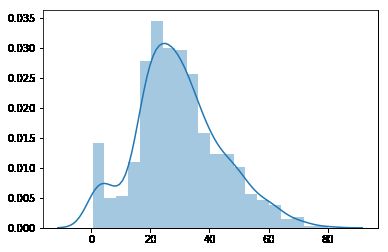
sns.distplot(train_data['Age'])
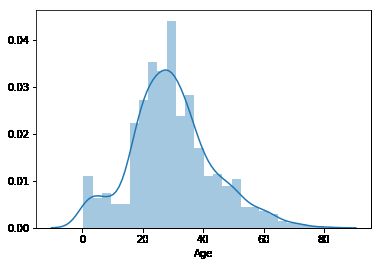
df_mean = df['Age'].fillna(df['Age'].mean())
sns.distplot(df_mean)
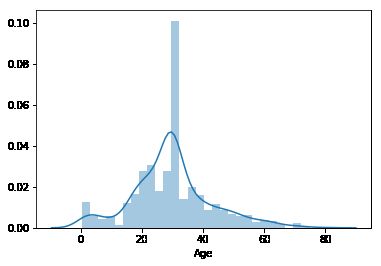
扩展研究
!pip install missingpy -t /home/aistudio/external-libraries
from sklearn.datasets import load_iris
import numpy as np
iris = load_iris()
X = iris.data
rng = np.random.RandomState(0)
X_missing = X.copy()
mask = np.abs(X[:, 2] - rng.normal(loc=5.5, scale=0.7, size=X.shape[0])) < 0.6
X_missing[mask, 3] = np.nan
from missingpy import KNNImputer
imputer = KNNImputer(n_neighbors=3, weights="uniform")
X_imputed = imputer.fit_transform(X_missing)
/home/aistudio/external-libraries/missingpy/utils.py:124: RuntimeWarning: invalid value encountered in sqrt
return distances if squared else np.sqrt(distances, out=distances)
sns.distplot(X.reshape((-1, 1)))
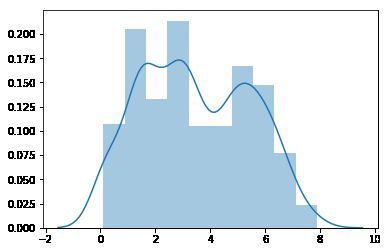
sns.distplot(X_imputed.reshape((-1, 1)))
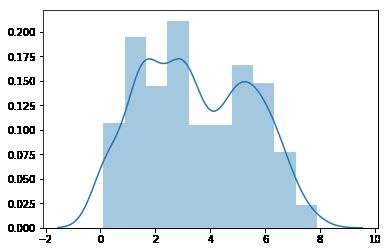