算法处理流程:
1. 读图像,依次读取多张图像
2. 应用光度立体得到反照率图像和表面梯度图像
3. 使用之前得到的表面梯度,计算表面的高斯曲率,得到高斯曲率图像
4. 对高斯曲率图像进行预处理和Blob分析,从而得到缺陷区域
* 该示例通过使用光度立体技术检测药片包装背面的缺陷
* 输入是4张不同的药片包装背面图片,光从不同的角度照射
* Initialization
dev_close_window ()
dev_update_off ()
dev_open_window (0, 0, 512, 512, 'black', WindowHandle)
set_display_font (WindowHandle, 14, 'mono', 'true', 'false')
Message := 'Inspect the backside of a blister'
Message[1] := 'using photometric stereo. In this case four'
Message[2] := 'different light orientations were used.'
disp_message (WindowHandle, Message, 'window', 12, 12, 'black', 'true')
disp_continue_message (WindowHandle, 'black', 'true')
stop ()
*
* Show input images with different illumination
* 1 读图像,依次读取多张图像
* 下面的for循环,依次显示4张从不同角度拍摄的药片包装的背面图像
read_image (Images, 'photometric_stereo/blister_back_0' + [1:4])
for I := 1 to 4 by 1
Message := 'Acquire image ' + I + ' of 4'
select_obj (Images, ObjectSelected, I)
dev_display (ObjectSelected)
disp_message (WindowHandle, Message, 'window', 12, 12, 'black', 'true')
wait_seconds (0.5)
endfor
*
* Apply photometric stereo to determine the albedo
* and the surface gradient.
* 2. 应用光度立体得到反照率图像和表面梯度图像
* 描述了从图像中心指向右侧的方向与投射到平面中的光的方向之间的角度。
* 也就是说,当观察图像(或相应的场景)时,倾斜角度为0表示光线来自右侧,
* 倾斜角度为90表示光线来自顶部,倾斜角度为180表示 光是从左边来的
Tilts := [6.1,95.0,-176.1,-86.8]
* 物平面与照明方向之间的角度
Slants := [41.4,42.6,41.7,40.9]
ResultType := ['gradient','albedo']
* 该算子得到反照率图像和表面梯度图像
photometric_stereo (Images, HeightField, Gradient, Albedo, Slants, Tilts, ResultType, 'poisson', [], [])
*
* Display the albedo image
* 显示反照率图像
dev_display (Albedo)
disp_message (WindowHandle, 'Albedo image', 'window', 12, 12, 'black', 'true')
disp_continue_message (WindowHandle, 'black', 'true')
stop ()
*
* Calculate the gaussian curvature of the surface
* using the gradient field as input for the operator
* 3. 使用之前得到的表面梯度,计算表面的高斯曲率,得到高斯曲率图像
* derivate_vector_field.
* Defects are usually easy to detect in the curvature image.
* 在曲率图像上能更容易的进行检测
* 该算子通过之前得到的表面梯度得到高斯曲率图像
derivate_vector_field (Gradient, GaussCurvature, 1, 'gauss_curvature')
*
* Detect defects
* 检测缺陷
*
* Segment the tablet areas in the curvature image
* 4. 对高斯曲率图像进行预处理和Blob分析,从而得到缺陷区域
* 在曲率图像中,我们先分开各个药片区域
regiongrowing (GaussCurvature, Regions, 1, 1, 0.001, 250)
* 通过宽和高的特征,进行特征选择
select_shape (Regions, TabletRegions, ['width','height'], 'and', [150,150], [200,200])
* 凸性形状转换,针对区域
shape_trans (TabletRegions, TabletRegions, 'convex')
* 区域联合
union1 (TabletRegions, TabletRegions)
* 腐蚀
erosion_circle (TabletRegions, TabletRegions, 3.5)
* Search for defects inside the tablet areas
* 在药片区域搜寻缺陷
* 抠图
reduce_domain (GaussCurvature, TabletRegions, ImageReduced)
* 计算图像各个像素的绝对值,存在此次处理的原因是:高斯曲率图像存在负值
* 缺陷处,高斯曲率会比较大
abs_image (ImageReduced, ImageAbs)
* 二值化 ,灰度直方图
threshold (ImageAbs, Region, 0.03, 255)
* 闭运算
closing_circle (Region, RegionClosing, 10.5)
* 断开得到连通域
connection (RegionClosing, ConnectedRegions)
* 通过面积特征,进行特征选择
select_shape (ConnectedRegions, Defects, 'area', 'and', 10, 99999)
* 获得缺陷区域的中心点行列坐标
area_center (Defects, Area, Row, Column)
* 生成圆形区域
gen_circle (Circle, Row, Column, gen_tuple_const(|Row|,20.5))
* Display the defects in curvature image
* 5. 接下来在高斯曲率图像中标记缺陷区域
dev_set_draw ('margin')
dev_set_color ('red')
dev_set_line_width (2)
dev_display (GaussCurvature)
dev_display (Circle)
Message := 'The defect can easily be detected'
Message[1] := 'in the surface curvature image'
disp_message (WindowHandle, Message, 'window', 12, 12, 'black', 'true')
stop ()
* Display the defects in the albedo image
* 6. 在反照率图像中标记出缺陷
dev_set_draw ('margin')
dev_set_color ('red')
dev_display (Albedo)
dev_display (Circle)
disp_message (WindowHandle, 'Defect in albedo image', 'window', 12, 12, 'black', 'true')

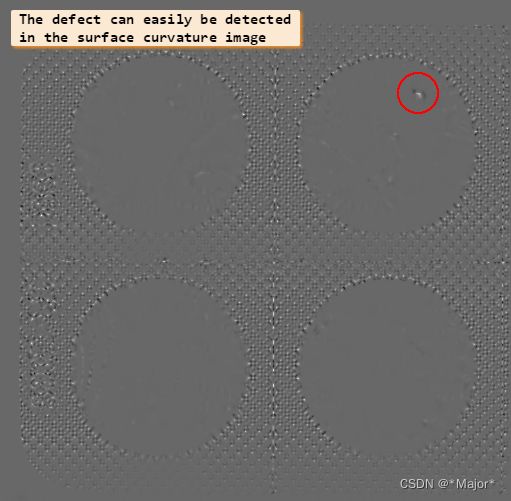