目录
1.什么是Markdown?
2.Markdown与HTML的简单对比
3.Markdown的基本语法
4.GitHub中Markdown的使用
4.1.GitHub上自定义的md文件格式与markdown pad(IDE)的区别,
4.2.GitHub上超链接的使用方法(遇到的错误及修改)
5.应用:论文项目中YOLOV5的md文档
参考文献
Markdown是一种轻量级的标记语言(lightweight markup language),并不是编程语言,它用于使用纯文本编辑器创建格式化文本。主要用于博客,即时消息,在线论坛,协作软件,文档页面和自述文件。Markdown 是一种最小的标记语言,可以使用普通的文本编辑器进行读取和编辑,但有专门设计的编辑器可以使用样式预览文件,这些样式适用于所有主要平台【1】。
Markdown 是一种用来书写网页内容的方法。它实际上在使用我们在日常生活中用来书写和发送信息的纯文本格式来书写。纯文本仅由常见的字母、文字和一些特殊符号来构成,例如星号(\*
)和反引号(`
)。
斜体:句子或单词前后加下划线 : _ 内容 _
实例1
加粗: 使用两个星号写到文本前后: **内容**
实例2
斜体和加粗混用实例3
实例4:加粗的同时设置为斜体,虽然不分顺序,但是第二个相对更容易区分。
这里有6种不同的标题,他们的大小递减
在Markdown里面添加标题,你要在文本的前面添加一个井号 (#
)。 你可以键入多个井号来生成你想要的几级标题。 例如,一级标题你需要添加一个井号 (# 一级标题
), 当你需要一个三级标题时你就要三个井号 (### 三级标题
).
实例5
- 不能将标题加粗,但是可以将其中的文字设置为斜体。
在 Markdown 中有两种不同的链接种类,分为内联链接和全局链接,但是它们的渲染方式完全相同。
内联链接:创建一个内联的链接, 你需要将被链接的文本用方括号 ( [ ]
)包起来, 然后将链接地址用圆括号 ( ( )
)起来。 *这些括号及以后出现的括号均为英文半角符号,译者注。 比方说,创建一个写着 "Visit Github!" 到 www.github.com 的超链接, 你应该使用Markdown这样书写: [Visit GitHub!](www.github.com)
.
实例6
实例7:加粗局部,整句话连接到新链接
实例8:标题设置为四号,然后将短语"the BBC" 链接到 www.bbc.com/news:
另外一种链接叫做 全局 链接,也就是这个链接链接到文档的另外一个位置。格式如下
[内容1][网址名称1]. [内容2][网址名称2]. [内容3][网址名称1]. #内容为链接的单词或句子,网址名称为定点名称,下面为定点名称指向的网址。 [网址名称1]: www.github.com [网址名称2]: www.google.com#优点:减少链接重复
上面的“全局”链接是第二组方括号: [another place]
和 [another-link]
。在Markdown文档的底部, 这些方括号被适当的定义为外部网页链接。使用全局链接的一个优点是如果文档中有多个指向统一网址的链接只需要书写或更新一次。 例如,我们觉得将所有的 [another place]
链接到其它的地方,我们只需要更改那一个全局链接即可。
全局链接本身不会被Markdown渲染出来,需要提供同名的用方括号包裹的标签来定义它们,然后是冒号,然后是链接。[内容]:链接
实例9:在底部的文本框,写一些全局链接。将第一个链接命名为 "a fun place", 然后将它链接到 www.zombo.com;将第二个链接到 www.stumbleupon.com。
添加图片也有两种方法,分为内联图片链接和。就像链接一样,并且渲染出来的结果是一样的。 添加链接和图片的区别仅仅在于前面的感叹号 ( !
)。
第一种图片的格式叫做 内联图片链接。创建一个内联的图片链接, 需要输入一个感叹号 ( !
)将方括号 ( [ ]
)中的描述性文本包起来, 然后使用圆括号 ( ( )
)包链接地址。 (描述性文本是为查看解释图片短语或者句子的语句。)
实例10:创建一个描述文本为"Benjamin Bannekat"的内联的链接地址为 https://octodex.github.com/images/bannekat.png 的图片, 应该这样写Markdown: 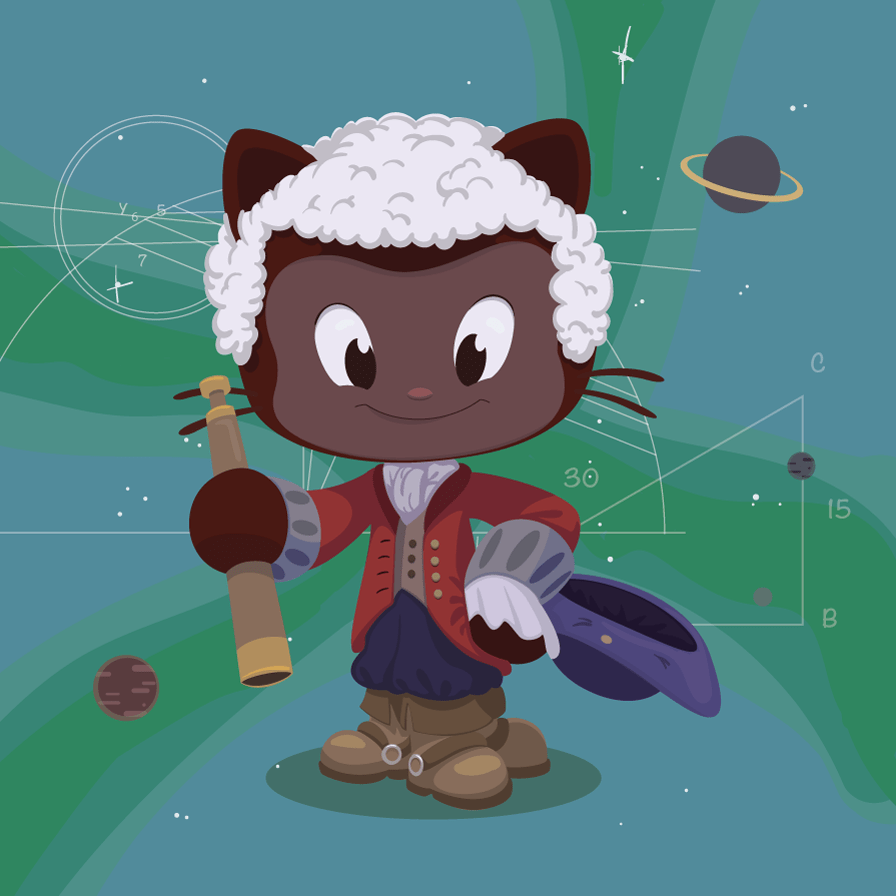
。
实例11:在下面的文本框里面,将链接转换为图片,并且在方括号内填写描述文本"A pretty tiger":
对于全局图片来说,使用同样的标签模式。在标记前添加一个感叹号然后跟两个方括号。 对于描述文本来说,应该添加两个或以上的图片标签,比如这样: ![The founding father][Father]
在Markdown页面的底部, 定义一个图片标签,就像这样: [Father]: http://octodex.github.com/images/founding-father.jpg
.
实例12:在文本框底部,放置了一些全局图像; 给第一个全局图片标签命名为 "Black", 然后把它链接到 https://upload.wikimedia.org/wikipedia/commons/a/a3/81_INF_DIV_SSI.jpg
; 给第二个图片链接到http://icons.iconarchive.com/icons/google/noto-emoji-animals-nature/256/22221-cat-icon.png
。
如果需要为其他来源的内容添加特殊的格式来引起读者的注意, 或为其他杂志的文章设计特殊的格式,那么 Markdown 的引用块语法将会非常有用。 引用块是一个拥有特殊格式的句子或段落,为了引起读者的注意。例如:
“无为而治的罪是所有七种罪中最致命的。有传言说,要使邪恶的人实现其目的,只需要好人无所事事。”
要创建一个引用块,只需要在行首添加大于号(>
)。>内容
实例13:
实例14: 可以在每一行引用前都添加一个大于号。 当有多个段落时,他就是一个整体的块。
实例15:以上内容的全部应用、斜体,加粗,图片,超链接
这个世界上有俩种不同的列表:无序列表和有序列表。 无序列表前是黑点,有序列表前是数字。
要创建一个无序列表,需要在每一个列表项前加一个星号( *
)。 每个列表要占一行。例如,一个杂货店商品的列表可以用 Markdown 写成这样* 内容:
* 牛奶 * 鸡蛋 * 三文鱼 * 黄油
这个 Markdown 列表将会显示成这样:
实例16
注意:中间一定要加空格
实例17:有序列表
注意:数字+点 后一定要加空格
实例18:无序列表和斜体,加粗,链接一起的实现
如果需要将一个列表变得更深,或者,将一个列表放置在另一个列表中。 不用担心,因为 Markdown 的语法完全相同。 只需要在每个星号前比上一个项目多缩进一个空格。
实例19:将人物的特征变成子列表。
分为段落换行和段落间换行。可以通过在每一行的结尾输入俩个空格来实现这一点。 空格是不可见的,输入的内容就像这样:
我有自相矛盾么?·· 很好,那我是在自相矛盾了,·· (我是庞大的,我承载着很多个自己。)
每个点( ·
)都代表一个空格:内容+空格。
实例20
- 注意:是空格,不是点
markdown pad(IDE)
* 代表列表
GitHub上自定义的md文件:
- 代表列表
- 超链接如果不生效的话,需要顶头
- GitHub仓库可直接引用作网页
错误1:markdown的超链接无法生效
解决办法:超链接如果不生效的话,需要顶格(如图)
显示为:
YOLOv5 is a family of object detection architectures and models pretrained on the COCO dataset, and represents Ultralytics
open-source research into future vision AI methods, incorporating lessons learned and best practices evolved over thousands of hours of research and development.
## Documentation
See the [YOLOv5 Docs](https://docs.ultralytics.com) for full documentation on training, testing and deployment.
## Quick Start Examples
Install
Clone repo and install [requirements.txt](https://github.com/ultralytics/yolov5/blob/master/requirements.txt) in a
[**Python>=3.6.0**](https://www.python.org/) environment, including
[**PyTorch>=1.7**](https://pytorch.org/get-started/locally/).
```bash
git clone https://github.com/ultralytics/yolov5 # clone
cd yolov5
pip install -r requirements.txt # install
```
Inference
Inference with YOLOv5 and [PyTorch Hub](https://github.com/ultralytics/yolov5/issues/36)
. [Models](https://github.com/ultralytics/yolov5/tree/master/models) download automatically from the latest
YOLOv5 [release](https://github.com/ultralytics/yolov5/releases).
```python
import torch
# Model
model = torch.hub.load('ultralytics/yolov5', 'yolov5s') # or yolov5m, yolov5l, yolov5x, custom
# Images
img = 'https://ultralytics.com/images/zidane.jpg' # or file, Path, PIL, OpenCV, numpy, list
# Inference
results = model(img)
# Results
results.print() # or .show(), .save(), .crop(), .pandas(), etc.
```
Inference with detect.py
`detect.py` runs inference on a variety of sources, downloading [models](https://github.com/ultralytics/yolov5/tree/master/models) automatically from
the latest YOLOv5 [release](https://github.com/ultralytics/yolov5/releases) and saving results to `runs/detect`.
```bash
python detect.py --source 0 # webcam
img.jpg # image
vid.mp4 # video
path/ # directory
path/*.jpg # glob
'https://youtu.be/Zgi9g1ksQHc' # YouTube
'rtsp://example.com/media.mp4' # RTSP, RTMP, HTTP stream
```
Training
The commands below reproduce YOLOv5 [COCO](https://github.com/ultralytics/yolov5/blob/master/data/scripts/get_coco.sh)
results. [Models](https://github.com/ultralytics/yolov5/tree/master/models)
and [datasets](https://github.com/ultralytics/yolov5/tree/master/data) download automatically from the latest
YOLOv5 [release](https://github.com/ultralytics/yolov5/releases). Training times for YOLOv5n/s/m/l/x are
1/2/4/6/8 days on a V100 GPU ([Multi-GPU](https://github.com/ultralytics/yolov5/issues/475) times faster). Use the
largest `--batch-size` possible, or pass `--batch-size -1` for
YOLOv5 [AutoBatch](https://github.com/ultralytics/yolov5/pull/5092). Batch sizes shown for V100-16GB.
```bash
python train.py --data coco.yaml --cfg yolov5n.yaml --weights '' --batch-size 128
yolov5s 64
yolov5m 40
yolov5l 24
yolov5x 16
```
Tutorials
* [Train Custom Data](https://github.com/ultralytics/yolov5/wiki/Train-Custom-Data) RECOMMENDED
* [Tips for Best Training Results](https://github.com/ultralytics/yolov5/wiki/Tips-for-Best-Training-Results) ☘️
RECOMMENDED
* [Weights & Biases Logging](https://github.com/ultralytics/yolov5/issues/1289) NEW
* [Roboflow for Datasets, Labeling, and Active Learning](https://github.com/ultralytics/yolov5/issues/4975) NEW
* [Multi-GPU Training](https://github.com/ultralytics/yolov5/issues/475)
* [PyTorch Hub](https://github.com/ultralytics/yolov5/issues/36) ⭐ NEW
* [TFLite, ONNX, CoreML, TensorRT Export](https://github.com/ultralytics/yolov5/issues/251)
* [Test-Time Augmentation (TTA)](https://github.com/ultralytics/yolov5/issues/303)
* [Model Ensembling](https://github.com/ultralytics/yolov5/issues/318)
* [Model Pruning/Sparsity](https://github.com/ultralytics/yolov5/issues/304)
* [Hyperparameter Evolution](https://github.com/ultralytics/yolov5/issues/607)
* [Transfer Learning with Frozen Layers](https://github.com/ultralytics/yolov5/issues/1314) ⭐ NEW
* [TensorRT Deployment](https://github.com/wang-xinyu/tensorrtx)
## Environments
Get started in seconds with our verified environments. Click each icon below for details.
## Integrations
|Weights and Biases|Roboflow ⭐ NEW|
|:-:|:-:|
|Automatically track and visualize all your YOLOv5 training runs in the cloud with [Weights & Biases](https://wandb.ai/site?utm_campaign=repo_yolo_readme)|Label and export your custom datasets directly to YOLOv5 for training with [Roboflow](https://roboflow.com/?ref=ultralytics) |
## Why YOLOv5
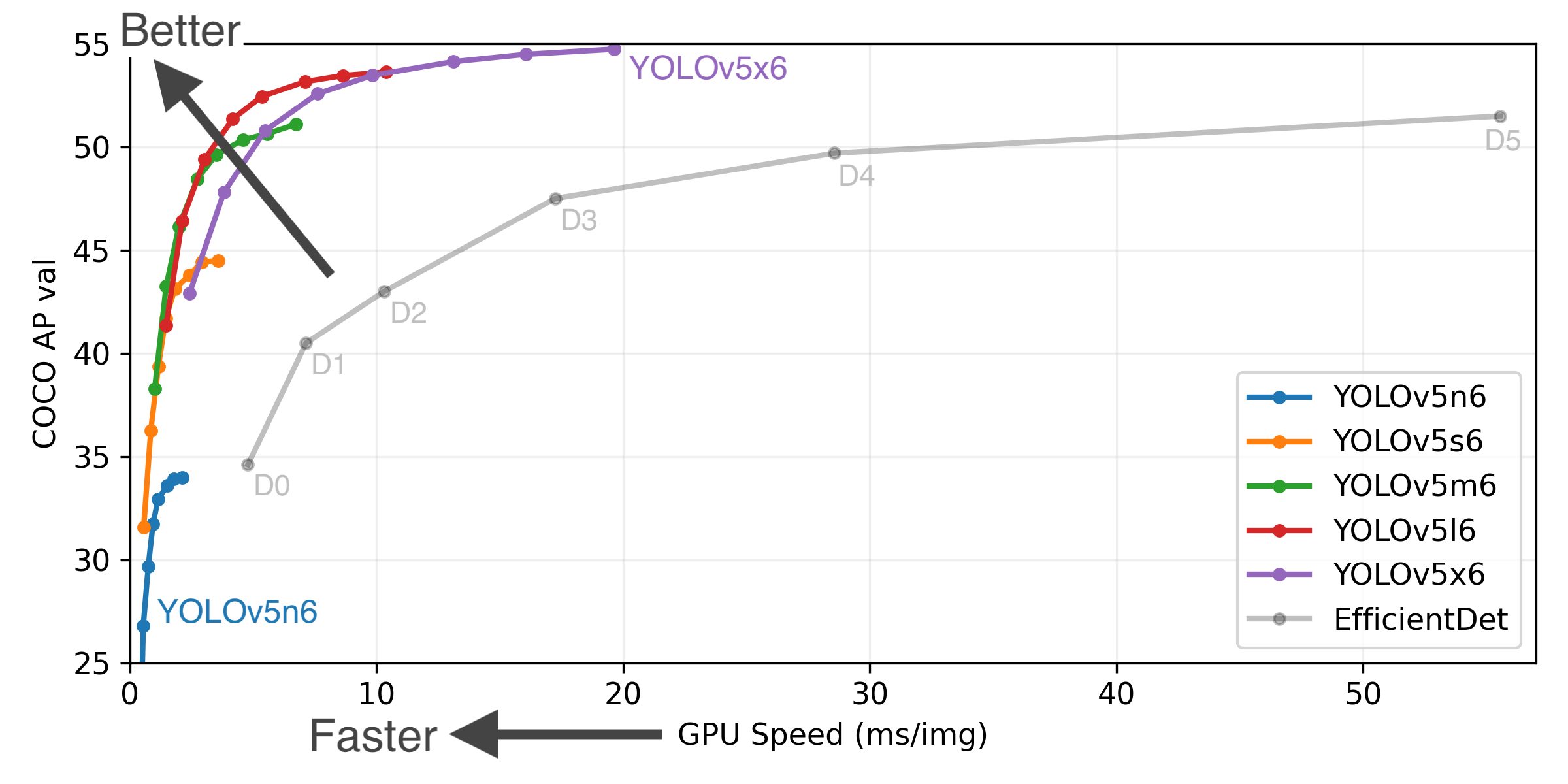
YOLOv5-P5 640 Figure (click to expand)
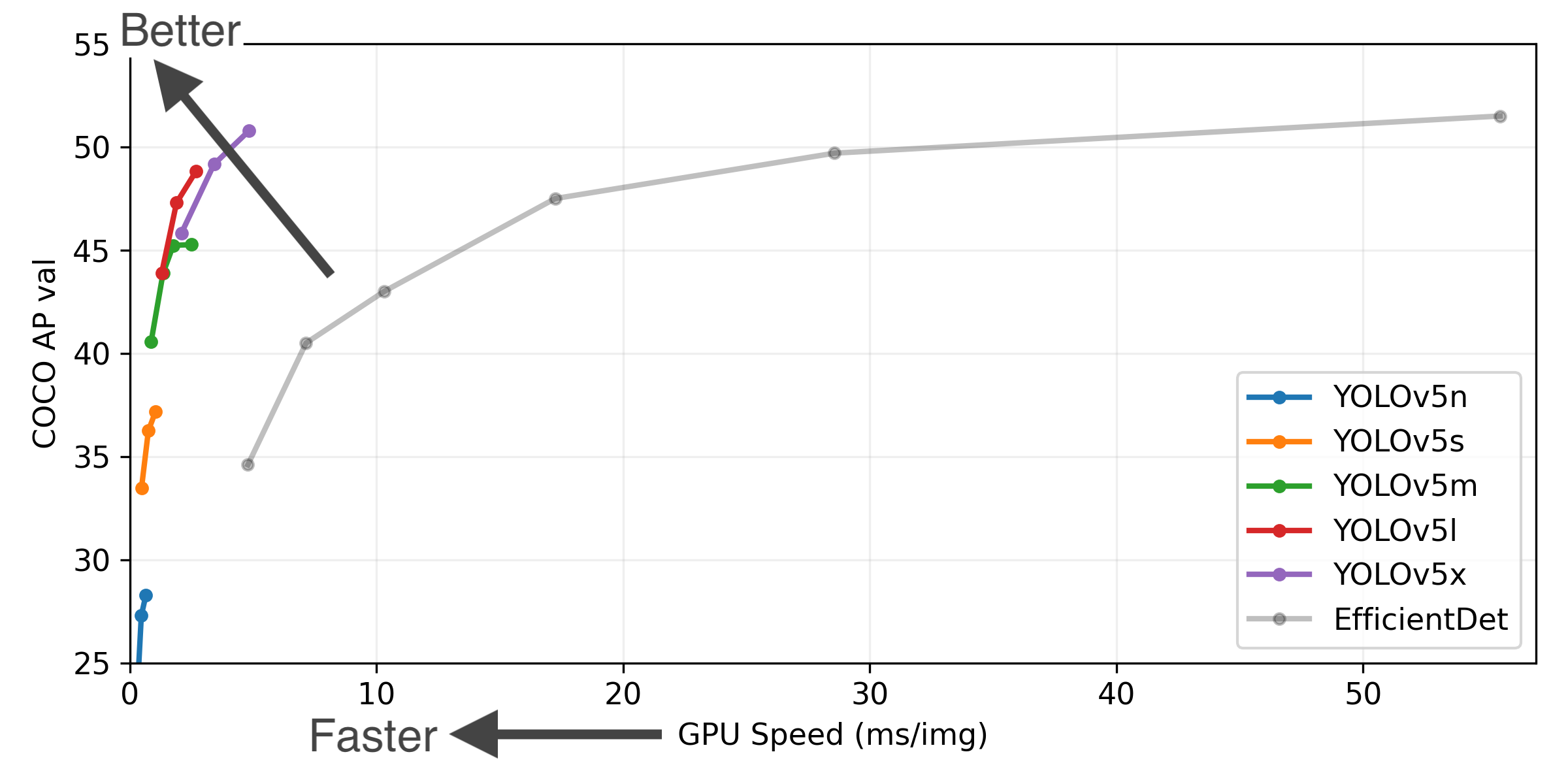
Figure Notes (click to expand)
* **COCO AP val** denotes [email protected]:0.95 metric measured on the 5000-image [COCO val2017](http://cocodataset.org) dataset over various inference sizes from 256 to 1536.
* **GPU Speed** measures average inference time per image on [COCO val2017](http://cocodataset.org) dataset using a [AWS p3.2xlarge](https://aws.amazon.com/ec2/instance-types/p3/) V100 instance at batch-size 32.
* **EfficientDet** data from [google/automl](https://github.com/google/automl) at batch size 8.
* **Reproduce** by `python val.py --task study --data coco.yaml --iou 0.7 --weights yolov5n6.pt yolov5s6.pt yolov5m6.pt yolov5l6.pt yolov5x6.pt`
### Pretrained Checkpoints
[assets]: https://github.com/ultralytics/yolov5/releases
[TTA]: https://github.com/ultralytics/yolov5/issues/303
|Model |size
(pixels) |mAPval
0.5:0.95 |mAPval
0.5 |Speed
CPU b1
(ms) |Speed
V100 b1
(ms) |Speed
V100 b32
(ms) |params
(M) |FLOPs
@640 (B)
|--- |--- |--- |--- |--- |--- |--- |--- |---
|[YOLOv5n][assets] |640 |28.4 |46.0 |**45** |**6.3**|**0.6**|**1.9**|**4.5**
|[YOLOv5s][assets] |640 |37.2 |56.0 |98 |6.4 |0.9 |7.2 |16.5
|[YOLOv5m][assets] |640 |45.2 |63.9 |224 |8.2 |1.7 |21.2 |49.0
|[YOLOv5l][assets] |640 |48.8 |67.2 |430 |10.1 |2.7 |46.5 |109.1
|[YOLOv5x][assets] |640 |50.7 |68.9 |766 |12.1 |4.8 |86.7 |205.7
| | | | | | | | |
|[YOLOv5n6][assets] |1280 |34.0 |50.7 |153 |8.1 |2.1 |3.2 |4.6
|[YOLOv5s6][assets] |1280 |44.5 |63.0 |385 |8.2 |3.6 |12.6 |16.8
|[YOLOv5m6][assets] |1280 |51.0 |69.0 |887 |11.1 |6.8 |35.7 |50.0
|[YOLOv5l6][assets] |1280 |53.6 |71.6 |1784 |15.8 |10.5 |76.7 |111.4
|[YOLOv5x6][assets]
+ [TTA][TTA]|1280
1536 |54.7
**55.4** |**72.4**
72.3 |3136
- |26.2
- |19.4
- |140.7
- |209.8
-
Table Notes (click to expand)
* All checkpoints are trained to 300 epochs with default settings and hyperparameters.
* **mAPval** values are for single-model single-scale on [COCO val2017](http://cocodataset.org) dataset.
Reproduce by `python val.py --data coco.yaml --img 640 --conf 0.001 --iou 0.65`
* **Speed** averaged over COCO val images using a [AWS p3.2xlarge](https://aws.amazon.com/ec2/instance-types/p3/) instance. NMS times (~1 ms/img) not included.
Reproduce by `python val.py --data coco.yaml --img 640 --task speed --batch 1`
* **TTA** [Test Time Augmentation](https://github.com/ultralytics/yolov5/issues/303) includes reflection and scale augmentations.
Reproduce by `python val.py --data coco.yaml --img 1536 --iou 0.7 --augment`
## Contribute
We love your input! We want to make contributing to YOLOv5 as easy and transparent as possible. Please see our [Contributing Guide](CONTRIBUTING.md) to get started, and fill out the [YOLOv5 Survey](https://ultralytics.com/survey?utm_source=github&utm_medium=social&utm_campaign=Survey) to send us feedback on your experiences. Thank you to all our contributors!
## Contact
For YOLOv5 bugs and feature requests please visit [GitHub Issues](https://github.com/ultralytics/yolov5/issues). For business inquiries or
professional support requests please visit [https://ultralytics.com/contact](https://ultralytics.com/contact).
效果
【1】Markdown - Wikipedia
【2】Markdown Tutorial