"""
Copyright 2022 The TBAALi Authors. All rights reserved.
Licensed under the Apache License, Version 2.0 (the "License");
you may not use this file except in compliance with the License.
You may obtain a copy of the License at
http://www.apache.org/licenses/LICENSE-2.0
Unless required by applicable law or agreed to in writing, software
distributed under the License is distributed on an "AS IS" BASIS,
WITHOUT WARRANTIES OR CONDITIONS OF ANY KIND, either express or implied.
See the License for the specific language governing permissions and
limitations under the License.
"""
"""
@Author: TBAALi
@Email: [email protected]
@FileName: ReRecognizeHandwrittenDigits.py
@DateTime: 5/16/22 8:48 PM
@SoftWare: PyCharm
@Copyright: Copyright (C) 2022 TBAALi
"""
import numpy as np
import torch
from torchvision.datasets import mnist
import torchvision.transforms as transforms
from torch.utils.data import DataLoader
import torch.nn.functional as F
from torch import nn, optim
import matplotlib.pyplot as plt
import pylab
train_batch_size = 64
test_batch_size = 128
learning_rate = 0.01
num_epoches = 20
lr = 0.01
momentum = 0.5
transform = transforms.Compose([transforms.ToTensor(), transforms.Normalize([0.5], [0.5])])
train_dataset = mnist.MNIST('./data', train = True, transform = transform, download = True)
test_dataset = mnist.MNIST('./data', train = False, transform = transform)
train_loader = DataLoader(train_dataset, batch_size = train_batch_size, shuffle = True)
test_loader = DataLoader(test_dataset, batch_size = test_batch_size, shuffle = False)
examples = enumerate(test_loader)
batch_idx, (example_data, example_targets) = next(examples)
fig = plt.figure()
for i in range(6) :
plt.subplot(2, 3, i + 1)
plt.tight_layout()
plt.imshow(example_data[i][0], cmap = 'gray', interpolation = 'none')
plt.title('Group Truth : {}'.format(example_targets[i]))
plt.xticks([])
plt.yticks([])
pylab.show()
class Net(nn.Module) :
"""
使用 sequential 构建网络, Sequential() 函数的作用是讲网络的层组合到一起
"""
def __init__(self, in_dim, n_hidden_1, n_hidden_2, out_dim):
super(Net, self).__init__()
self.layer1 = nn.Sequential(
nn.Linear(in_dim, n_hidden_1),
nn.BatchNorm1d(n_hidden_1)
)
self.layer2 = nn.Sequential(
nn.Linear(n_hidden_1, n_hidden_2),
nn.BatchNorm1d(n_hidden_2)
)
self.layer3 = nn.Sequential(
nn.Linear(n_hidden_2, out_dim)
)
def forward(self, x):
x = F.relu(self.layer1(x))
x = F.relu(self.layer2(x))
x = self.layer3(x)
return x
device = torch.device("cuda:0" if torch.cuda.is_available() else "cpu")
model = Net(28 * 28, 300, 100, 10)
model.to(device)
criterion = nn.CrossEntropyLoss()
optimizer = optim.SGD(model.parameters(), lr = lr, momentum = momentum)
losses = []
acces = []
eval_losses = []
eval_access = []
for epoch in range(num_epoches):
train_loss = 0
train_acc = 0
model.train()
if epoch % 5 == 0:
optimizer.param_groups[0]['lr'] *= 0.1
for img, label in train_loader:
img = img.to(device)
label = label.to(device)
img = img.view(img.size(0), -1)
out = model(img)
loss = criterion(out, label)
optimizer.zero_grad()
loss.backward()
optimizer.step()
train_loss += loss.item()
_, pred = out.max(1)
num_correct = (pred == label).sum().item()
acc = num_correct / img.shape[0]
train_acc += acc
losses.append(train_loss / len(train_loader))
acces.append(train_acc / len(train_loader))
eval_loss = 0
eval_acc = 0
model.eval()
for img, label in test_loader:
img = img.to(device)
label = label.to(device)
img = img.view(img.size(0), -1)
out = model(img)
loss = criterion(out, label)
eval_loss += loss.item()
_, pred = out.max(1)
num_correct = (pred == label).sum().item()
acc = num_correct / img.shape[0]
eval_acc += acc
eval_losses.append(eval_loss / len(test_loader))
eval_access.append(eval_acc / len(test_loader))
print('epoch: {}, Train Loss: {:.4f}, Train ACC: {:.4f}, Test Loss: {:.4f}, Test Acc: {:.4f}'
.format(epoch, train_loss / len(train_loader),
train_acc / len(train_loader),
eval_loss / len(test_loader),
eval_acc / len(test_loader)))
plt.title('Train Loss')
plt.plot(np.arange(len(losses)), losses, color = 'orange', linewidth = 1.0, linestyle = '--')
plt.plot(np.arange(len(acces)), acces, color = 'green', linewidth = 1.0, linestyle = '-')
plt.legend(['Train Loss', 'Train Acc'], loc = 'upper right')
plt.show()
def print_hi(name):
print(f'Hi, {name}')
if __name__ == '__main__':
print_hi('Python')
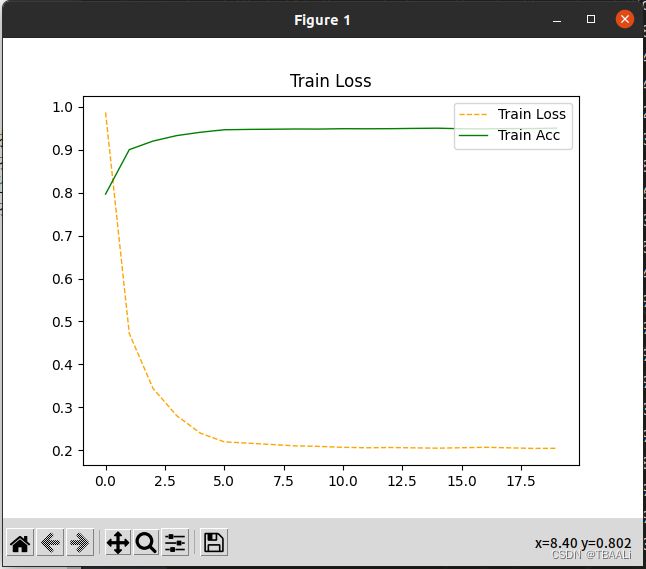