文章目录
-
-
- 1.数据准备
- 2.数据采样
- 3.建模与调参
- 最终代码
1.数据准备
import pandas as pd
import numpy as np
import matplotlib.pyplot as plt
data = pd.read_csv('data/creditcard.csv',delimiter=',')
print(data.shape)
print(data.head(5))
print(data['Class'].value_counts())
plt.subplots(1,1,figsize = (7,5))
count_classes = pd.value_counts(data['Class'],sort=True).sort_index()
count_classes.plot(kind='bar')
plt.title('Fraud class histogram',fontsize=13)
plt.xlabel('Class',fontsize=13)
plt.ylabel('Frequency',fontsize=15)
plt.xticks(rotation=0)
plt.show()
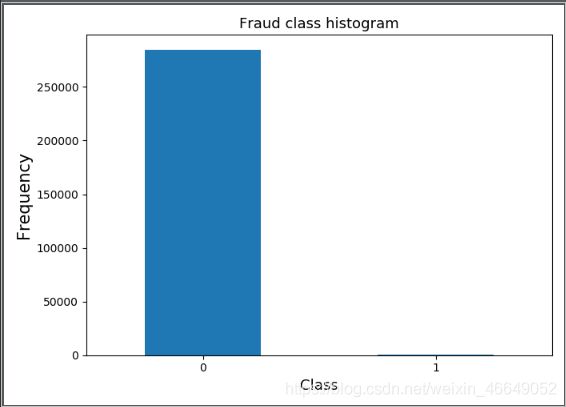
2.数据采样
data = data.drop(['Time'], axis=1)
X = np.array(data.loc[:, :'V28'])
y = np.array(data['Class'])
sess = StratifiedShuffleSplit(n_splits=1, test_size=0.4, random_state=0)
for train_index, test_index in sess.split(X, y):
print(len(train_index))
X_train, X_test = X[train_index], X[test_index]
y_train, y_test = y[train_index], y[test_index]
print('train_size:%s' % len(y_train),
'test_size:%s' % len(y_test))
plt.figure(figsize=(7, 5))
count_classes = pd.value_counts(y_train, sort=True)
count_classes.plot(kind='bar')
plt.title("The histogram of fraud class in trainingdata ", fontsize=13)
plt.xlabel("Class", fontsize=13)
plt.ylabel("Frequency", fontsize=15)
plt.xticks(rotation=0)
plt.show()
ros = RandomOverSampler(random_state=0)
sos = SMOTE(random_state=0)
kos = SMOTETomek(random_state=0)
X_ros, y_ros = ros.fit_sample(X_train, y_train)
X_sos, y_sos = sos.fit_sample(X_train, y_train)
X_kos, y_kos = kos.fit_sample(X_train, y_train)
print('ros:%s,sos:%s,kos:%s' % (len(y_ros), len(y_sos), len(y_kos)))
a = pd.DataFrame(y_ros)
print(a[0].value_counts())
284807, 31)
Time V1 V2 V3 ... V27 V28 Amount Class
0 0.0 -1.359807 -0.072781 2.536347 ... 0.133558 -0.021053 149.62 0
1 0.0 1.191857 0.266151 0.166480 ... -0.008983 0.014724 2.69 0
2 1.0 -1.358354 -1.340163 1.773209 ... -0.055353 -0.059752 378.66 0
3 1.0 -0.966272 -0.185226 1.792993 ... 0.062723 0.061458 123.50 0
4 2.0 -1.158233 0.877737 1.548718 ... 0.219422 0.215153 69.99 0
[5 rows x 31 columns]
0 284315
1 492
Name: Class, dtype: int64
170884
train_size:170884 test_size:113923
ros:341178,sos:341178,kos:341178
1 170589
0 170589
Name: 0, dtype: int64
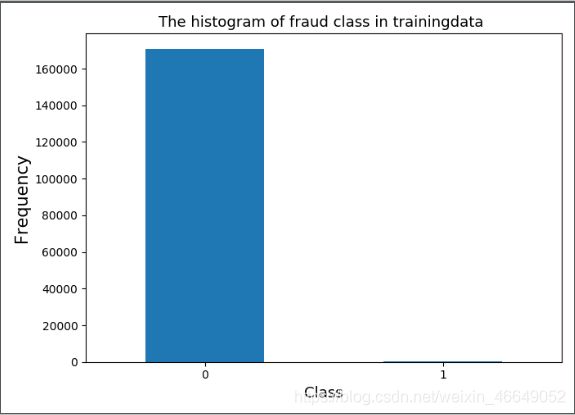
3.建模与调参
clf = DecisionTreeClassifier(criterion='gini',random_state=1234)
param_grid = {'max_depth':[3,4,5,6],',max_lesf_nodes':[4,6,8,10,12]}
cv = GridSearchCV(clf,param_grid = param_grid,scoring='f1')
data = [[X_train,y_train],
[X_ros,y_ros],
[X_sos,y_sos],
[X_kos,y_kos]]
for features,labels in data:
cv.fit(features,labels)
pred_test = cv.predict(X_test)
print('auc:%.3f' % roc_auc_score(y_test, pred_test),
'recall:%.3f' % recall_score(y_test, pred_test),
'precision:%.3f' % precision_score(y_test, pred_test))
train_data = X_ros
train_target = y_ros
test_target = y_test
test_data = X_test
lr = LogisticRegression(C = 1, penalty = 'l1')
lr.fit(train_data,train_target)
test_est = lr.predict(test_data)
print("Logistic Regression accuracy:")
print(classification_report(test_target,test_est))
fpr_test, tpr_test, th_test = roc_curve(test_target, test_est)
print('Logistic Regression AUC: %.4f' %auc(fpr_test, tpr_test))
rf = RandomForestClassifier(criterion = 'entropy',max_depth = 10,n_estimators = 15,
max_features = 0.6,min_samples_split = 50)
rf.fit(train_data, train_target)
test_est = rf.predict(test_data)
print("Random Forest accuracy:")
print(classification_report(test_target,test_est))
fpr_test, tpr_test, th_test = roc_curve(test_target, test_est)
print('Random Forest AUC: %.4f' %auc(fpr_test, tpr_test))
gb = GradientBoostingClassifier(loss = 'exponential',learning_rate = 0.2,n_estimators = 40,
max_depth = 3,min_samples_split = 30)
gb.fit(train_data, train_target)
test_est = gb.predict(test_data)
print("GradientBoosting accuracy:")
print(classification_report(test_target,test_est))
fpr_test, tpr_test, th_test = roc_curve(test_target, test_est)
print('GradientBoosting AUC : %.4f' %auc(fpr_test, tpr_test))
param_grid = {
'criterion':['entropy','gini'],
'max_depth':[8,10,12],
'n_estimators':[11,13,15],
'max_features':[0.3,0.4,0.5],
'min_samples_split':[4,8,12]
}
rfc = RandomForestClassifier()
rfccv = GridSearchCV(estimator = rfc, param_grid = param_grid, scoring = 'roc_auc', cv = 4)
rfccv.fit(train_data, train_target)
test_est = rfccv.predict(test_data)
print("Random Forest accuracy:")
print(classification_report(test_target,test_est))
fpr_test, tpr_test, th_test = roc_curve(test_target, test_est)
print('Random Forest AUC: %.4f' %auc(fpr_test, tpr_test))
print('最优参数模型为:\n',rfccv.best_params_)
param_grid = {
'learning_rate':[0.1,0.3,0.5],
'n_estimators':[15,20,30],
'max_depth':[1,2,3],
'min_samples_split':[12,16,20]
}
gbc = GradientBoostingClassifier()
gbccv = GridSearchCV(estimator = gbc, param_grid = param_grid, scoring = 'roc_auc', cv = 4)
gbccv.fit(train_data, train_target)
test_est = gbccv.predict(test_data)
print("Gradient Boosting accuracy:")
print(classification_report(test_target,test_est))
fpr_test, tpr_test, th_test = roc_curve(test_target, test_est)
print('Gradient Boosting AUC : %.4f' %auc(fpr_test, tpr_test))
print('最优参数模型:\n',gbccv.best_params_)
最终代码
import matplotlib.pyplot as plt
import numpy as np
import pandas as pd
from imblearn.combine import SMOTETomek
from imblearn.over_sampling import RandomOverSampler, SMOTE
from sklearn.ensemble import RandomForestClassifier, GradientBoostingClassifier
from sklearn.linear_model import LogisticRegression
from sklearn.metrics import (auc, roc_auc_score, precision_score, roc_curve, recall_score, classification_report)
from sklearn.model_selection import GridSearchCV
from sklearn.model_selection import StratifiedShuffleSplit
from sklearn.tree import DecisionTreeClassifier
data = pd.read_csv('data/creditcard.csv', delimiter=',')
print(data.shape)
print(data.head(5))
print(data['Class'].value_counts())
plt.subplots(1, 1, figsize=(7, 5))
count_classes = pd.value_counts(data['Class'], sort=True).sort_index()
count_classes.plot(kind='bar')
plt.title('Fraud class histogram', fontsize=13)
plt.xlabel('Class', fontsize=13)
plt.ylabel('Frequency', fontsize=15)
plt.xticks(rotation=0)
plt.show()
data = data.drop(['Time'], axis=1)
X = np.array(data.loc[:, :'V28'])
y = np.array(data['Class'])
sess = StratifiedShuffleSplit(n_splits=1, test_size=0.4, random_state=0)
for train_index, test_index in sess.split(X, y):
print(len(train_index))
X_train, X_test = X[train_index], X[test_index]
y_train, y_test = y[train_index], y[test_index]
print('train_size:%s' % len(y_train),
'test_size:%s' % len(y_test))
plt.figure(figsize=(7, 5))
count_classes = pd.value_counts(y_train, sort=True)
count_classes.plot(kind='bar')
plt.title("The histogram of fraud class in trainingdata ", fontsize=13)
plt.xlabel("Class", fontsize=13)
plt.ylabel("Frequency", fontsize=15)
plt.xticks(rotation=0)
plt.show()
ros = RandomOverSampler(random_state=0)
sos = SMOTE(random_state=0)
kos = SMOTETomek(random_state=0)
X_ros, y_ros = ros.fit_sample(X_train, y_train)
X_sos, y_sos = sos.fit_sample(X_train, y_train)
X_kos, y_kos = kos.fit_sample(X_train, y_train)
print('ros:%s,sos:%s,kos:%s' % (len(y_ros), len(y_sos), len(y_kos)))
a = pd.DataFrame(y_ros)
print(a[0].value_counts())
clf = DecisionTreeClassifier(criterion='gini', random_state=1234)
param_grid = {'max_depth': [3, 4, 5, 6], ',max_lesf_nodes': [4, 6, 8, 10, 12]}
cv = GridSearchCV(clf, param_grid=param_grid, scoring='f1')
data = [[X_train, y_train],
[X_ros, y_ros],
[X_sos, y_sos],
[X_kos, y_kos]]
for features, labels in data:
cv.fit(features, labels)
pred_test = cv.predict(X_test)
print('auc:%.3f' % roc_auc_score(y_test, pred_test),
'recall:%.3f' % recall_score(y_test, pred_test),
'precision:%.3f' % precision_score(y_test, pred_test))
train_data = X_ros
train_target = y_ros
test_target = y_test
test_data = X_test
lr = LogisticRegression(C=1, penalty='l1')
lr.fit(train_data, train_target)
test_est = lr.predict(test_data)
print("Logistic Regression accuracy:")
print(classification_report(test_target, test_est))
fpr_test, tpr_test, th_test = roc_curve(test_target, test_est)
print('Logistic Regression AUC: %.4f' % auc(fpr_test, tpr_test))
rf = RandomForestClassifier(criterion='entropy', max_depth=10, n_estimators=15,
max_features=0.6, min_samples_split=50)
rf.fit(train_data, train_target)
test_est = rf.predict(test_data)
print("Random Forest accuracy:")
print(classification_report(test_target, test_est))
fpr_test, tpr_test, th_test = roc_curve(test_target, test_est)
print('Random Forest AUC: %.4f' % auc(fpr_test, tpr_test))
gb = GradientBoostingClassifier(loss='exponential', learning_rate=0.2, n_estimators=40,
max_depth=3, min_samples_split=30)
gb.fit(train_data, train_target)
test_est = gb.predict(test_data)
print("GradientBoosting accuracy:")
print(classification_report(test_target, test_est))
fpr_test, tpr_test, th_test = roc_curve(test_target, test_est)
print('GradientBoosting AUC : %.4f' % auc(fpr_test, tpr_test))
param_grid = {
'criterion': ['entropy', 'gini'],
'max_depth': [8, 10, 12],
'n_estimators': [11, 13, 15],
'max_features': [0.3, 0.4, 0.5],
'min_samples_split': [4, 8, 12]
}
rfc = RandomForestClassifier()
rfccv = GridSearchCV(estimator=rfc, param_grid=param_grid, scoring='roc_auc', cv=4)
rfccv.fit(train_data, train_target)
test_est = rfccv.predict(test_data)
print("Random Forest accuracy:")
print(classification_report(test_target, test_est))
fpr_test, tpr_test, th_test = roc_curve(test_target, test_est)
print('Random Forest AUC: %.4f' % auc(fpr_test, tpr_test))
print('最优参数模型为:\n', rfccv.best_params_)
param_grid = {
'learning_rate': [0.1, 0.3, 0.5],
'n_estimators': [15, 20, 30],
'max_depth': [1, 2, 3],
'min_samples_split': [12, 16, 20]
}
gbc = GradientBoostingClassifier()
gbccv = GridSearchCV(estimator=gbc, param_grid=param_grid, scoring='roc_auc', cv=4)
gbccv.fit(train_data, train_target)
test_est = gbccv.predict(test_data)
print("Gradient Boosting accuracy:")
print(classification_report(test_target, test_est))
fpr_test, tpr_test, th_test = roc_curve(test_target, test_est)
print('Gradient Boosting AUC : %.4f' % auc(fpr_test, tpr_test))
print('最优参数模型:\n', gbccv.best_params_)