github
项目地址:https://github.com/liangzhicheng120/bayes
一、简介
项目使用
SpringBoot
做了一层web
封装项目使用的分词工具hanlp
项目使用
JDK8
贝叶斯法则
事件A
在事件B
(发生)的条件下的概率,与事件B
在事件A
的条件下的概率是不一样的;然而,这两者是有确定的关系,贝叶斯法则就是这种关系的陈述。贝叶斯术语
[图片上传失败...(image-d286a7-1547375244426)]
其中L(A|B)
是在B
发生的情况下A发生的可能性。
在贝叶斯法则中,每个名词都有约定俗成的名称:
Pr(A)
是A
的先验概率或边缘概率。之所以称为"先验"是因为它不考虑任何B方面的因素。
Pr(A|B)
是已知B
发生后A的条件概率,也由于得自B
的取值而被称作A的后验概率。
Pr(B|A)
是已知A
发生后B的条件概率,也由于得自A
的取值而被称作B的后验概率。
Pr(B)
是B
的先验概率或边缘概率,也作标准化常量(normalized constant
)。
后验概率 = (似然度 * 先验概率)/标准化常量 也就是说,后验概率与先验概率和似然度的乘积成正比。贝叶斯推断的含义
对条件概率公式进行变形,可以得到如下形式:
[图片上传失败...(image-3fbd35-1547375244427)]
我们把
P(A)
称为"先验概率"(Prior probability
),即在B
事件发生之前,我们对A
事件概率的一个判断。P(A|B)
称为"后验概率"(Posterior probability
),即在B
事件发生之后,我们对A
事件概率的重新评估。P(B|A)/P(B)
称为"可能性函数"(Likelyhood
),这是一个调整因子,使得预估概率更接近真实概率。
后验概率 = 先验概率 x 调整因子这就是贝叶斯推断的含义。我们先预估一个"先验概率",然后加入实验结果,看这个实验到底是增强还是削弱了"先验概率",由此得到更接近事实的"后验概率"。
在这里,如果"可能性函数"P(B|A)/P(B)>1
,意味着"先验概率"被增强,事件A的发生的可能性变大;如果"可能性函数"=1,意味着B
事件无助于判断事件A的可能性;如果"可能性函数"<1,意味着"先验概率"被削弱,事件A
的可能性变小。
二、例子
- 别墅和狗
一座别墅在过去的 20 年里一共发生过 2 次被盗,别墅的主人有一条狗,狗平均每周晚上叫 3 次,在盗贼入侵时狗叫的概率被估计为 0.9,问题是:在狗叫的时候发生入侵的概率是多少?
我们假设A
事件为狗在晚上叫,B
为盗贼入侵,则P(A) = 3 / 7,P(B)=2/(20·365)=2/7300,P(A | B) = 0.9
,按照公式很容易得出结果:P(B|A)=0.9*(2/7300)/(3/7)=0.00058
三、实战代码
- 模型文件(
classify.txt
)
火影忍者 火影
火影忍者 秘传
火影忍者 大蛇丸
火影忍者 剧场版
火影忍者 动作
火影忍者 激斗
火影忍者 战斗
火影忍者 转生
火影忍者 佐助
火影忍者 村子
火影忍者 第六代火影
火影忍者 克拉
火影忍者 卡卡
火影忍者 带土
火影忍者 疾风
火影忍者 自来
火影忍者 火影忍者
火影忍者 仙人
火影忍者 六道
火影忍者 大战
火影忍者 九尾
火影忍者 忍者
火影忍者 究极
火影忍者 纲手
火影忍者 鸣人
火影忍者 木叶
火影忍者 忍术
火影忍者 秽土
火影忍者 宇智波
火影忍者 九尾妖狐
火影忍者 阿飞
海贼王 正文
海贼王 尾田
海贼王 海贼王
海贼王 弗兰奇
海贼王 草帽
海贼王 海贼
海贼王 武海
海贼王 事件
海贼王 悬赏
海贼王 第话
海贼王 梦想
海贼王 血型
海贼王 王下
海贼王 航路
海贼王 历史
海贼王 德雷斯
海贼王 船长
海贼王 恶魔
海贼王 路飞
海贼王 漫画
海贼王 超新星
海贼王 罗萨篇
海贼王 世界
海贼王 果实
海贼王 冥王
海贼王 荣一郎
海贼王 海贼团
海贼王 司法
海贼王 超人
海贼王 成为
海贼王 寻找
海贼王 传说
海贼王 海贼王
海贼王 中海
海贼王 罗杰
海贼王 秘宝
海贼王 留下
海贼王 伙伴
海贼王 ONE
海贼王 PIECE
海贼王 海贼
海贼王 志同道合
海贼王 扬起
海贼王 实现
龙珠 复活
龙珠 仙人
龙珠 武道
龙珠 得到
龙珠 军团
龙珠 找寻
龙珠 魔王
龙珠 饺子
龙珠 特典
龙珠 打败
龙珠 花梨
龙珠 缎带
龙珠 发售日期
龙珠 龙珠
龙珠 天津
龙珠 七龙珠
龙珠 比克
龙珠 天神
龙珠 修练
龙珠 悟空
龙珠 封入
龙珠 次郎
龙珠 拉夫
龙珠 封印
龙珠 许愿
龙珠 兵卫
龙珠 一武道
龙珠 动画
TestBayes.java
package com.xinrui.util;
import java.io.BufferedReader;
import java.io.File;
import java.io.FileReader;
import java.util.ArrayList;
import java.util.HashMap;
import java.util.List;
import java.util.Map;
import org.apache.commons.io.Charsets;
import org.apache.commons.io.FileUtils;
import org.apache.commons.lang.StringUtils;
import org.apache.log4j.Logger;
import com.hankcs.hanlp.HanLP;
/**
* 贝叶斯计算器主体类
*/
public class Bayes {
private static Logger logger = Logger.getLogger(Bayes.class);
/**
* 将原训练元组按类别划分
*
* @param datas
* 训练元组
* @return Map<类别,属于该类别的训练元组>
*/
public static Map>> classifyByCategory(ArrayList> datas) {
if (datas == null) {
return null;
}
Map>> map = new HashMap>>();
ArrayList singleTrainning = null;
String classificaion = "";
for (int i = 0; i < datas.size(); i++) {
singleTrainning = datas.get(i);
classificaion = singleTrainning.get(0);
singleTrainning.remove(0);
if (map.containsKey(classificaion)) {
map.get(classificaion).add(singleTrainning);
} else {
ArrayList> list = new ArrayList>();
list.add(singleTrainning);
map.put(classificaion, list);
}
}
return map;
}
/**
* 在训练数据的基础上预测测试元组的类别
*
* @param datas
* 训练元组
* @param testData
* 测试元组
* @return 测试元组的类别
*/
public static String predictClassify(ArrayList> datas, ArrayList testData) {
if (datas == null || testData == null) {
return null;
}
int maxPIndex = -1;
Map>> map = classifyByCategory(datas);
Object[] classes = map.keySet().toArray();
double maxProbability = 0.0;
for (int i = 0; i < map.size(); i++) {
double p = 0.0;
for (int j = 0; j < testData.size(); j++) {
p += calProbabilityClassificationInKey(map, classes[i].toString(), testData.get(j));
}
if (p > maxProbability) {
maxProbability = p;
maxPIndex = i;
}
}
return maxPIndex == -1 ? "其他" : classes[maxPIndex].toString();
}
/**
* 在训练数据的基础上预测测试元组的类别
*
* @param testData
* 测试元组
* @return 测试元组的类别
* @throws Exception
*/
public String predictClassify(ArrayList testData, String mId) throws Exception {
return predictClassify(read(mId), testData);
}
/**
* 某一特征值在某一分类上的概率分布[ P(key|Classify) ]
*
* @param classify
* 某一分类特征向量集
* @param value
* 某一特征值
* @return 概率分布
*/
private static double calProbabilityKeyInClassification(ArrayList> classify, String value) {
if (classify == null || StringUtils.isEmpty(value)) {
return 0.0;
}
int totleKeyCount = 0;
int foundKeyCount = 0;
ArrayList featureVector = null; // 分类中的某一特征向量
for (int i = 0; i < classify.size(); i++) {
featureVector = classify.get(i);
for (int j = 0; j < featureVector.size(); j++) {
totleKeyCount++;
if (featureVector.get(j).equalsIgnoreCase(value)) {
foundKeyCount++;
}
}
}
return totleKeyCount == 0 ? 0.0 : 1.0 * foundKeyCount / totleKeyCount;
}
/**
* 获得某一分类的概率 [ P(Classify) ]
*
* @param classes
* 分类集合
* @param classify
* 某一特定分类
* @return 某一分类的概率
*/
private static double calProbabilityClassification(Map>> map, String classify) {
if (map == null | StringUtils.isEmpty(classify)) {
return 0;
}
Object[] classes = map.keySet().toArray();
int totleClassifyCount = 0;
for (int i = 0; i < classes.length; i++) {
totleClassifyCount += map.get(classes[i].toString()).size();
}
return 1.0 * map.get(classify).size() / totleClassifyCount;
}
/**
* 获得关键词的总概率
*
* @param map
* 所有分类的数据集
* @param key
* 某一特征值
* @return 某一特征值在所有分类数据集中的比率
*/
private static double calProbabilityKey(Map>> map, String key) {
if (map == null || StringUtils.isEmpty(key)) {
return 0;
}
int foundKeyCount = 0;
int totleKeyCount = 0;
Object[] classes = map.keySet().toArray();
for (int i = 0; i < map.size(); i++) {
ArrayList> classify = map.get(classes[i]);
ArrayList featureVector = null; // 分类中的某一特征向量
for (int j = 0; j < classify.size(); j++) {
featureVector = classify.get(j);
for (int k = 0; k < featureVector.size(); k++) {
totleKeyCount++;
if (featureVector.get(k).equalsIgnoreCase(key)) {
foundKeyCount++;
}
}
}
}
return totleKeyCount == 0 ? 0.0 : 1.0 * foundKeyCount / totleKeyCount;
}
/**
* 计算在出现key的情况下,是分类classify的概率 [ P(Classify | key) ]
*
* @param map
* 所有分类的数据集
* @param classify
* 某一特定分类
* @param key
* 某一特定特征
* @return P(Classify | key)
*/
private static double calProbabilityClassificationInKey(Map>> map, String classify, String key) {
ArrayList> classifyList = map.get(classify);
double pkc = calProbabilityKeyInClassification(classifyList, key); // p(key|classify)
double pc = calProbabilityClassification(map, classify); // p(classify)
double pk = calProbabilityKey(map, key); // p(key)
return pk == 0 ? 0 : pkc * pc / pk; // p(classify | key)
}
/**
* 读取训练文档中的训练数据 并进行封装
*
* @param filePath
* 训练文档的路径
* @return 训练数据集
* @throws Exception
*/
public static ArrayList> read(String clzss) throws Exception {
ArrayList singleTrainning = null;
ArrayList> trainningSet = new ArrayList>();
List datas = new ArrayList(FileUtils.readLines(new File(clzss), Charsets.UTF_8));
if (datas.size() == 0) {
logger.error("[" + "模型文件加载错误" + "]" + clzss);
throw new Exception("模型文件加载错误!");
}
for (int i = 0; i < datas.size(); i++) {
String[] characteristicValues = datas.get(i).split(" ");
singleTrainning = new ArrayList();
for (int j = 0; j < characteristicValues.length; j++) {
if (StringUtils.isNotEmpty(characteristicValues[j])) {
singleTrainning.add(characteristicValues[j]);
}
}
trainningSet.add(singleTrainning);
}
return trainningSet;
}
/**
*
* @param fileName
* 训练文件
* @param size
* 关键词个数
*/
public static void trainBayes(String fileName, String mId, int size) {
try {
Bayes bayes = new Bayes();
BufferedReader reader = new BufferedReader(new FileReader(fileName));
String line = null;
int total = 0;
int right = 0;
long start = System.currentTimeMillis();
while ((line = reader.readLine()) != null) {
ArrayList testData = (ArrayList) HanLP.extractKeyword(line, size);
String classification = bayes.predictClassify(testData, mId);
if (classification.equals(fileName.split("\\.")[0])) {
right += 1;
}
System.out.print("\n分类:" + classification);
total++;
}
reader.close();
long end = System.currentTimeMillis();
System.out.println("正确分类:" + right);
System.out.println("总行数:" + total);
System.out.println("正确率:" + MathUtil.div(right, total, 4) * 100 + "%");
System.out.println("程序运行时间: " + (end - start) / 1000 + "s");
} catch (Exception e) {
e.printStackTrace();
}
}
}
TestBayes.java
package com.xinrui.test;
import java.util.ArrayList;
import com.hankcs.hanlp.HanLP;
import com.xinrui.util.Bayes;
public class TestBayes {
public static void main(String[] args) throws Exception {
// 获取当前工程存放位置
String path = TestBayes.class.getResource("").getPath();
String classPath = path.substring(0, path.indexOf("/com/xinrui"));
// 模型文件存放位置
String modelName = classPath + "/model/classify_model.txt";
ArrayList> model = Bayes.read(modelName);
// 抽取10个关键词组成一个元祖
ArrayList testData = (ArrayList) HanLP
.extractKeyword(
"时值“大海贼时代”,为了寻找传说中海贼王罗杰所留下的大秘宝“ONE PIECE”,无数海贼扬起旗帜,互相争斗。有一个梦想成为海盗的少年叫路飞,他因误食“恶魔果实”而成为了橡皮人,在获得超人能力的同时付出了一辈子无法游泳的代价。十年后,路飞为实现与因救他而断臂的香克斯的约定而出海,他在旅途中不断寻找志同道合的伙伴,开始了以成为海贼王为目标的伟大的冒险旅程[9] ",
15);
// 输出预测结果
System.out.println(Bayes.predictClassify(model, testData));
}
}
-
结果
image
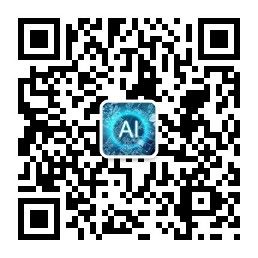