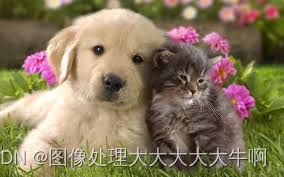
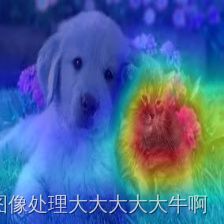
安装pytorch_grad_cam
pip install grad-cam
自动化生成不同层的bash脚本
for i in $(seq 0 13)
do
echo "Running iteration $i";
python yolov8_heatmap.py $i;
done
热力图生成python代码
import warnings
warnings.filterwarnings('ignore')
warnings.simplefilter('ignore')
import torch, yaml, cv2, os, shutil
import numpy as np
np.random.seed(0)
import sys
import matplotlib.pyplot as plt
from tqdm import trange
from PIL import Image
from ultralytics.nn.tasks import DetectionModel as Model
from ultralytics.yolo.utils.torch_utils import intersect_dicts
from ultralytics.yolo.utils.ops import xywh2xyxy
from pytorch_grad_cam import GradCAMPlusPlus, GradCAM, XGradCAM
from pytorch_grad_cam.utils.image import show_cam_on_image
from pytorch_grad_cam.activations_and_gradients import ActivationsAndGradients
def letterbox(im, new_shape=(640, 640), color=(114, 114, 114), auto=True, scaleFill=False, scaleup=True, stride=32):
shape = im.shape[:2]
if isinstance(new_shape, int):
new_shape = (new_shape, new_shape)
r = min(new_shape[0] / shape[0], new_shape[1] / shape[1])
if not scaleup:
r = min(r, 1.0)
ratio = r, r
new_unpad = int(round(shape[1] * r)), int(round(shape[0] * r))
dw, dh = new_shape[1] - new_unpad[0], new_shape[0] - new_unpad[1]
if auto:
dw, dh = np.mod(dw, stride), np.mod(dh, stride)
elif scaleFill:
dw, dh = 0.0, 0.0
new_unpad = (new_shape[1], new_shape[0])
ratio = new_shape[1] / shape[1], new_shape[0] / shape[0]
dw /= 2
dh /= 2
if shape[::-1] != new_unpad:
im = cv2.resize(im, new_unpad, interpolation=cv2.INTER_LINEAR)
top, bottom = int(round(dh - 0.1)), int(round(dh + 0.1))
left, right = int(round(dw - 0.1)), int(round(dw + 0.1))
im = cv2.copyMakeBorder(im, top, bottom, left, right, cv2.BORDER_CONSTANT, value=color)
return im, ratio, (dw, dh)
class yolov8_heatmap:
def __init__(self, weight, cfg, device, method, layer, backward_type, conf_threshold, ratio):
device = torch.device(device)
ckpt = torch.load(weight)
model_names = ckpt['model'].names
csd = ckpt['model'].float().state_dict()
model = Model(cfg, ch=3, nc=len(model_names)).to(device)
csd = intersect_dicts(csd, model.state_dict(), exclude=['anchor'])
model.load_state_dict(csd, strict=False)
model.eval()
print(f'Transferred {len(csd)}/{len(model.state_dict())} items')
target_layers = [eval(layer)]
method = eval(method)
colors = np.random.uniform(0, 255, size=(len(model_names), 3)).astype(np.int64)
self.__dict__.update(locals())
def post_process(self, result):
logits_ = result[:, 4:]
boxes_ = result[:, :4]
sorted, indices = torch.sort(logits_.max(1)[0], descending=True)
return torch.transpose(logits_[0], dim0=0, dim1=1)[indices[0]], torch.transpose(boxes_[0], dim0=0, dim1=1)[indices[0]], xywh2xyxy(torch.transpose(boxes_[0], dim0=0, dim1=1)[indices[0]]).cpu().detach().numpy()
def draw_detections(self, box, color, name, img):
xmin, ymin, xmax, ymax = list(map(int, list(box)))
cv2.rectangle(img, (xmin, ymin), (xmax, ymax), tuple(int(x) for x in color), 2)
cv2.putText(img, str(name), (xmin, ymin - 5), cv2.FONT_HERSHEY_SIMPLEX, 0.8, tuple(int(x) for x in color), 2, lineType=cv2.LINE_AA)
return img
def crop(self,box,img):
xmin, ymin, xmax, ymax = list(map(int, list(box)))
return img[ymin:ymax,xmin:xmax].copy()
def __call__(self, img_path, save_path):
if os.path.exists(save_path):
shutil.rmtree(save_path)
os.makedirs(save_path, exist_ok=True)
image = cv2.imread(img_path)
img,(wratio,hratio), (dw, dh) = letterbox(image)
img = cv2.cvtColor(img, cv2.COLOR_BGR2RGB)
img = np.float32(img) / 255.0
image = cv2.cvtColor(image, cv2.COLOR_BGR2RGB)
image = np.float32(image) / 255.0
tensor = torch.from_numpy(np.transpose(img, axes=[2, 0, 1])).unsqueeze(0).to(self.device)
grads = ActivationsAndGradients(self.model, self.target_layers, reshape_transform=None)
result = grads(tensor)
activations = grads.activations[0].cpu().detach().numpy()
post_result, pre_post_boxes, post_boxes = self.post_process(result[0])
for i in trange(int(post_result.size(0) * self.ratio)):
if float(post_result[i].max()) < self.conf_threshold:
break
self.model.zero_grad()
if self.backward_type == 'class' or self.backward_type == 'all':
score = post_result[i].max()
score.backward(retain_graph=True)
if self.backward_type == 'box' or self.backward_type == 'all':
for j in range(4):
score = pre_post_boxes[i, j]
score.backward(retain_graph=True)
if self.backward_type == 'class':
gradients = grads.gradients[0]
elif self.backward_type == 'box':
gradients = grads.gradients[0] + grads.gradients[1] + grads.gradients[2] + grads.gradients[3]
else:
gradients = grads.gradients[0] + grads.gradients[1] + grads.gradients[2] + grads.gradients[3] + grads.gradients[4]
b, k, u, v = gradients.size()
weights = self.method.get_cam_weights(self.method, None, None, None, activations, gradients.detach().numpy())
weights = weights.reshape((b, k, 1, 1))
saliency_map = np.sum(weights * activations, axis=1)
saliency_map = np.squeeze(np.maximum(saliency_map, 0))
saliency_map = cv2.resize(saliency_map, (tensor.size(3), tensor.size(2)))
saliency_map_min, saliency_map_max = saliency_map.min(), saliency_map.max()
if (saliency_map_max - saliency_map_min) == 0:
continue
saliency_map = (saliency_map - saliency_map_min) / (saliency_map_max - saliency_map_min)
saliency_map = cv2.resize(saliency_map[int(dh):-int(dh),:], (image.shape[1],image.shape[0]))
winv_ratio = 1.0 / wratio
hinv_ratio = 1.0 / hratio
det_box_restored = [
int((post_boxes[i][0] - (dw+0.1)) * winv_ratio),
int((post_boxes[i][1] - (dh+0.1)) * hinv_ratio),
int((post_boxes[i][2] - (dw-0.1)) * winv_ratio),
int((post_boxes[i][3] - (dh-0.1)) * hinv_ratio)
]
det_box_restored = [int(coord) for coord in det_box_restored]
cam_image = show_cam_on_image(image.copy(), saliency_map, use_rgb=True)
crop_cam_image = self.crop(det_box_restored,cam_image)
crop_cam_image = Image.fromarray(crop_cam_image)
crop_cam_image.save(f'{save_path}/{i}_crop.png')
cam_image = self.draw_detections(det_box_restored, self.colors[int(post_result[i, :].argmax())], f'{self.model_names[int(post_result[i, :].argmax())]} {float(post_result[i].max()):.2f}', cam_image)
cam_image = Image.fromarray(cam_image)
cam_image.save(f'{save_path}/{i}.png')
def get_params():
params = {
'weight': '../runs/detect/my-person73-small/weights/best.pt',
'cfg': 'models/small-yolov8.yaml',
'device': 'cuda:0',
'method': 'GradCAM',
'layer': f'model.model[{sys.argv[1]}]',
'backward_type': 'all',
'conf_threshold': 0.6,
'ratio': 0.02
}
return params
if __name__ == '__main__':
model = yolov8_heatmap(**get_params())
model(r'1.jpg', f'result/{sys.argv[1]}')