- 深度学习与机器学习的关系
数字化信息化智能化解决方案
深度学习机器学习人工智能
深度学习和机器学习的关系深度学习是机器学习的一个子领域,专注于使用神经网络,特别是深度神经网络(DNN)来解决各种问题。可以说,深度学习是机器学习的一种方法或技术。两者都致力于通过从数据中提取有用的信息或模式来自动改进算法的性能。机器学习涵盖了更广泛的算法和技术,包括决策树、支持向量机、随机森林、聚类算法等,而深度学习则专注于神经网络和相关的优化技术。优缺点比较机器学习:优点:通用性:机器学习算法
- 深度学习与机器学习的关系:比较、展望与交叉点
小厂程序猿
人工智能
深度学习和机器学习,作为人工智能领域的两个重要分支,常常被人们相提并论。虽然它们在很多方面都有共同之处,但也有各自的特点和优势。深入理解两者的关系,有助于我们更好地应用这两种技术,并预测其未来的发展趋势。首先,让我们理解一下什么是深度学习和机器学习。机器学习是一种通过经验自动改进算法的技术,它利用已知数据集来训练模型,使其能够自动提取特征、识别模式并进行分类和预测。而深度学习则是机器学习的一个子集
- 深度学习疆界:探索基本原理与算法,揭秘应用力量,展望未来发展与智能交互的新纪元
Srlua
人工智能深度学习人工智能
目录什么是深度学习深度学习的基本原理和算法深度学习的应用实例深度学习的挑战和未来发展方向挑战未来发展方向深度学习与机器学习的关系深度学习与人类的智能交互什么是深度学习深度学习是一种基于神经网络的机器学习方法,旨在模仿人类大脑分析和学习数据的方式。深度学习的核心在于能够自动学习和提取数据中的复杂特征,它通过构建深层的神经网络结构来实现对数据的高层次抽象和理解。这种方法在图像识别、语音识别、自然语言处
- 【日常聊聊】深度学习进度
还在路上的秃头
日常聊聊深度学习人工智能笔记面试
个人博客:个人主页个人专栏:日常聊聊⛳️功不唐捐,玉汝于成目录前言正文方向一:深度学习的基本原理和算法方向二:深度学习的应用实例方向三:深度学习的挑战和未来发展方向方向四:深度学习与机器学习的关系方向五:深度学习与人类的智能交互结语我的其他博客前言深度学习作为人工智能领域的重要分支,通过模拟人类大脑神经网络的学习过程,实现了在多个领域的突破性进展。其基本原理和算法以及在自然语言处理、计算机视觉、语
- 深度学习与机器学习研究综述
第欧根尼的酒桶
深度学习机器学习人工智能
一、引言你对科幻小说中描绘的人工智能的想法非常着迷,以至于你经常沉浸在幻想中,想象着拥有一台能够理解并执行你所有命令的智能电脑。这种对未来技术的憧憬成为了你踏入编程世界的初衷,并促使你在大学里选择了计算机科学作为你的专业。但随着学习的深入,你逐渐意识到,传统的人工智能并不像科幻小说中那样神奇,它更多的是在不同的应用场景中应用各种搜索算法。然而,在这个过程中,你意外地发现了一个更加吸引人的领域——机
- 浅谈人工智能之深度学习~
秋风起,再归来~
杂谈人工智能深度学习
目录前言:深度学习的进展一:深度学习的基本原理和算法二:深度学习的应用实例三:深度学习的挑战和未来发展方向四:深度学习与机器学习的关系五:深度学习与人类的智能交互悟已往之不谏,知来者犹可追创作不易,宝子们!如果这篇文章对你们有帮助的话,别忘了给个免费的赞哟~前言:深度学习的进展深度学习是人工智能领域的一个重要分支,它利用神经网络模拟人类大脑的学习过程,通过大量数据训练模型,使其能够自动提取特征、识
- 谈一谈深度学习与机器学习
流浪字节π
人工智能深度学习机器学习人工智能
深度学习是机器学习的一个子领域,其核心是使用多层神经网络来学习数据的复杂表示。机器学习是一种更广泛的概念,涵盖了许多不同的方法和技术,包括深度学习在内。关系:深度学习是机器学习的一种方法:深度学习利用多层神经网络进行特征学习和模式识别,是机器学习中的一种技术手段。机器学习包括多种方法:除了深度学习,机器学习还包括传统的监督学习、无监督学习、强化学习等方法,这些方法可以使用各种不同的算法和技术。优缺
- 也谈人工智能——AI科普入门
星猿杂谈
AI人工智能人工智能
文章目录1.科普入门人工智能的定义人工智能的类型-弱AI与强AI人工智能、深度学习与机器学习人工智能的应用和使用场景语音识别计算机视觉客户服务建议引擎数据分析网络安全行业应用人工智能发展史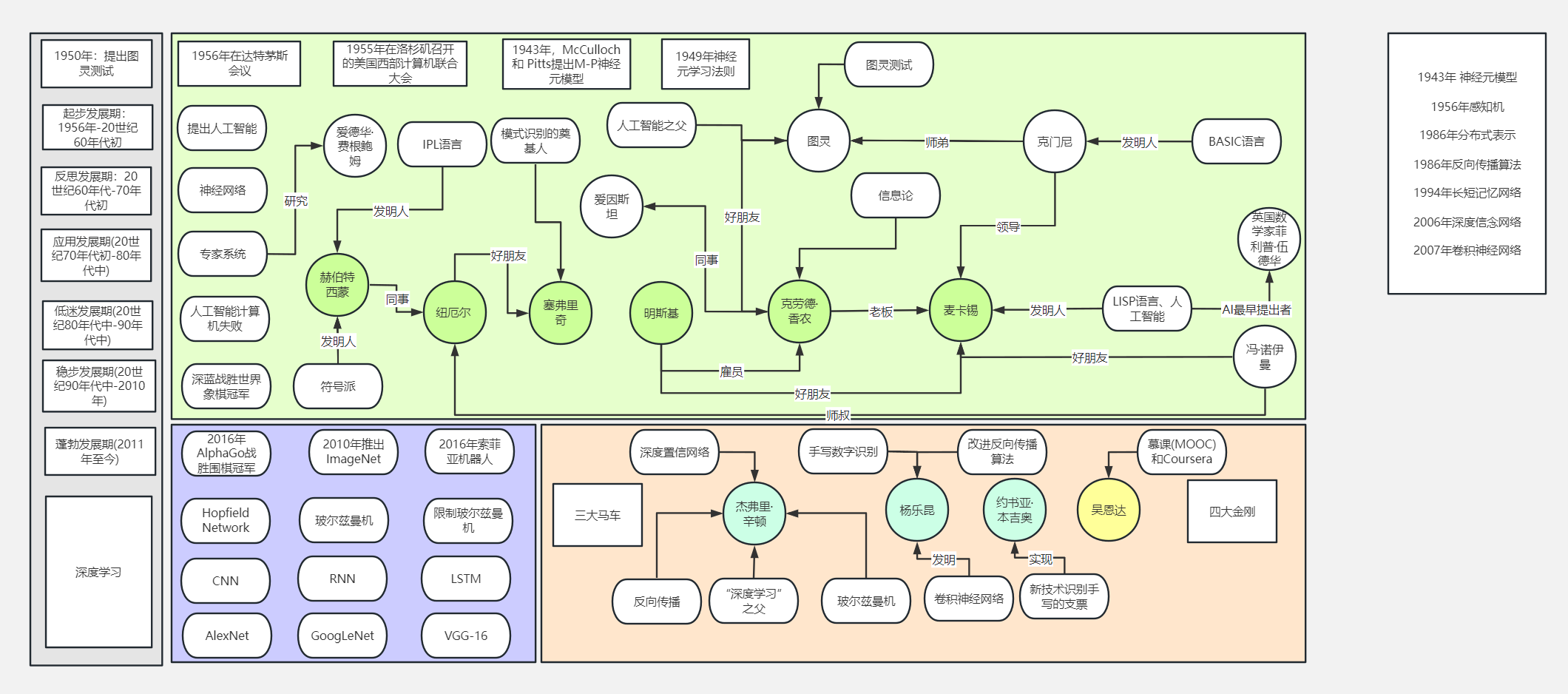产业结构层级系统架构**人工智能标准体系**
- 深度学习入门
AI-智能
深度学习人工智能机器学习
概述此学习路径专为有兴趣熟悉和探索深度学习主题的任何人而设计。目前,该学习路径涵盖了深度学习的基础知识,但将来将得到增强,以涵盖有监督和无监督的深度学习概念。深度学习基础知识了解深度学习与机器学习的关系,探索其基础知识,并了解在某些应用中使用深度学习算法的优势。技能水平初学者估计完成时间约2小时。学习目标通过此学习路径,你将获得:对深度学习概念的理解对深度学习架构的理解深度学习框架的比较如何在Te
- 人工智能,深度学习与机器学习
陶大明
从去年研究生入学开始,总是听到周围的人在说人工智能,机器学习,深度学习,,,,然而自己并不能完全明白这些到底是怎么回事,以及他们之间有着什么样的关系,近来也不知道都搞了些什么鬼,大概好像是知道了点什么。首先包含关系:人工智能包含机器学习,机器学习包含深度学习再来阐述一下机器学习的含义:机器学习:如果一个程序可以在任务T上,随着经验E的增加,效果P也随之增加,则称这个程序可以从经验中学习。(这个是某
- 深度学习速成版01---神经网络
__Miracle__
深度学习AI神经网络深度学习人工智能
深度学习与机器学习的区别机器学习的特征工程步骤是要靠手动完成的,而且需要大量领域专业知识深度学习通常由多个层组成,它们通常将更简单的模型组合在一起,通过将数据从一层传递到另一层来构建更复杂的模型。通过大量数据的训练自动得到模型,不需要人工设计特征提取环节。深度学习算法试图从数据中学习高级功能,这是深度学习的一个非常独特的部分。因此,减少了为每个问题开发新特征提取器的任务。适合用在难提取特征的图像、
- 【机器学习】学习笔记01-概论
NRbene
机器学习机器学习学习数据挖掘
机器学习简介文章目录机器学习简介机器学习辨析深度学习与机器学习机器学习与数据挖掘机器学习与统计学习机器学习与传统编程机器学习概念适用条件挑战模型的稳定性模型的可解释性历史符号主义贝叶斯学派连接主义其他概念基本概念三要素模型策略算法归纳偏好证明机器学习的目标欠拟合和过拟合泛化误差(重点)缓解过拟合深入理解泛化误差基本概念方法总结机器学习一般流程机器学习分类按有无标签分类按输出空间分类按模型分类按算法
- TensorFlow(1):深度学习的介绍
不死鸟.亚历山大.狼崽子
TensorFlow深度学习tensorflow人工智能
1深度学习与机器学习的区别学习目标:知道深度学习与机器学习的区别区别:深度学习没有特征提取1.1特征提取方面机器学习的特征工程步骤是要靠手动完成的,而且需要大量领域专业知识深度学习通常由多个层组成,它们通常将更简单的模型组合在一起,将数据从一层传递到另一层来构建更复杂的模型。通过训练大量数据自动得到模型,不需要人工特征提取环节深度学习算法试图从数据中学习高级功能,这是深度学习的一个非常独特的部分。
- 深度学习与机器学习啃书(10.20)
阿杰小子
深度学习机器学习python
深度学习神经网络的数据类型1神经网络的基本架构2神经网络入门1神经网络的基本架构1)神经网络的核心组件是层,即依靠对输入数据的“筛选”进行模型的训练。同时,要想训练网络,我们还需要选择编译步骤的三个参数:损失函数(lossfunction):用于衡量训练数据上的性能优化器:(optimizer):基于训练数据和损失函数来更新网络的机制监控指标:即训练还需关注的部分,如精度等2)在神经网络当中是如何
- 【深度学习】吴恩达课程笔记(一)——深度学习概论、神经网络基础
今天有没有吃饱饱
深度学习神经网络人工智能深度学习python1024程序员节
笔记为自我总结整理的学习笔记,若有错误欢迎指出哟~吴恩达课程笔记——深度学习概论、神经网络基础一、概念区别1.深度学习与机器学习2.深度学习与神经网络二、什么是神经网络1.分类2.特点3.工作原理4.神经网络示意图5.神经网络进行监督学习6.深度学习的发展三、神经网络基础1.二分分类(BinaryClassification)2.logistic回归变量定义损失函数(lossfunction)成本
- 机器学习——数据处理
北边一颗小星星
机器学习
机器学习简介机器学习是人工智能的一个实现途径深度学习是机器学习的一个方法发展而来机器学习:从数据中自动分析获得模型,并利用模型对未知数据进行预测。数据集的格式:特征值+目标值比如上图中房子的各种属性是特征值,然后房屋价格是目标值。注:对于每一行数据我们可以称之为样本有些数据集可以没有目标值——聚类深度学习与机器学习的关系:深度学习是使用深度神经网络的机器学习。——机器学习里面有种结构叫神经网络,神
- Python神经网络1之TensorFlow
VillanelleS
神经网络python
Python神经网络1之TensorFlow深度学习介绍深度学习与机器学习的区别特征提取方面数据量和计算性能方面要求算法代表深度学习框架介绍TensorFlow的安装TensorFlow框架介绍TensorFlow结构分析案例加法展示图与TensorBoard图相关操作默认图创建图TensorBoard:可视化学习OP深度学习介绍深度学习与机器学习的区别特征提取方面机器学习的特征工程步骤是要靠手动
- 基于深度学习的中学化学教学设计
千面英雄侠
基于深度学习的教学,有利于促进理解学习,发展高级思维,发展学生学科核心素养。依据学科核心素养的培养要求,分析教学核心内容,了解学生的学习基础和认知水平;确定切合于学生的学习要求;创设引导学生深入思考核心内容的问题情境,是做好基于深度学习教学设计的三个重要环节。教育领域所讨论的深度学习与机器学习研究的深度学习含义不同。它是对学习的一种理解模式,以及依据这种理解模式所确定的一种学习策略。深度学习的概念
- 神经网络学习(六)----深度学习与机器学习的关系理解
红枣燕麦
学习总结神经网络深度学习机器学习
1.复杂场景与多场景复杂场景是指某一种场景(场所)的环境复杂程度。复杂程度包括目标的复杂性和背景环境的复杂性。目标的复杂性通常是指目标姿态、尺度、速度的变化和目标遮挡、消失等问题,背景环境的复杂性是指动态背景、光照变化、摄像机抖动等问题,目标和背景环境的复杂性严重影响了现有目标检测算法的准确性和应用范围。多场景是指多种类场景(场所),例如交通十字路口、海岸边、花园里、室内和室外等。2.人工智能、机
- 【节选】吴恩达给出的AI职业生涯规划
hongxu000
机器学习的一些想法和笔记编辑器前端
吴恩达给出的AI职业生涯规划基础的机器学习技能深度学习与机器学习相关的数学软件开发如何获得这些技能?看到AI大神吴恩达给大家的技术学习以及职业规划的意见,受益匪浅。节选收藏,与君共勉~核心便是保持终身学习,每周学一点,快乐多一点~我认为对于机器学习的技术职业来说,最重要的课题包括:基础的机器学习技能例如,了解线性回归、逻辑回归、神经网络、决策树、聚类和异常检测等模型很重要。除了具体的模型,更重要的
- tensorflow人工智能(1)
刘竣熙
python人工智能tensorflow深度学习
1.1深度学习与机器学习的区别1.1.1.1特征提取方面机器学习:要靠手动完成的,而且需要大量领域专业知识深度学习:不需要人工设计特征提取环节数据量第一、它们需要大量的训练数据集第二、是训练深度神经网络需要大量的算力1.1.2算法代表机器学习朴素贝叶斯、决策树等深度学习神经网络图像识别自然语言处理技术1.2深度学习框架介绍1.2.2TensorFlow的特点1.2.3TensorFlow的安装ub
- 人脸识别美颜算法实战-深度学习基础知识
南妮儿
深度学习算法人工智能
深度学习与机器学习的区别:机器学习:人类定义输入数据的特征深度学习:机器自动找到输入数据的特征在深度学习中,采用多层的神经网络架构来提取图像信息,越靠近底层的神经网络提取出来的都是点、线等低维度特征,而高维度的神经网络层则会更多地保留比如耳朵、眼睛等高维度特征深度学习通过低维度特征到高维度特征一层层地构建,找到最终能够构成分类器的最佳组合。深度学习入门概念1.神经网络在深度学习中,神经网络由很多“
- 一文读懂深度学习与机器学习
互联互通社区
算法神经网络大数据编程语言python
如果你经常想让自己弄清楚机器学习和深度学习的区别,阅读该文章,我将用通俗易懂的语言为你介绍他们之间的差别。机器学习和深度学习变得越来越火。突然之间,不管是了解的还是不了解的,所有人都在谈论机器学习和深度学习。无论你是否主动关注过数据科学,你应该已经听说过这两个名词了。为了展示他们的火热程度,我在Googletrend上搜索了这些关键字:如果你想让自己弄清楚机器学习和深度学习的区别,请阅读本篇文章,
- 2021-08-21
z7mysun
自学深度学习
李宏毅机器学习P13深度学习简介DL的发展DL的步骤1.构建model2.设置lossfunction3.调整参数,使得totalloss最小(这样就获得最好的function)深度学习与机器学习反向传播机制深度学习简介DL的发展DL的步骤1.构建modeldefineafunctionset(neuralnetwork结构,没有参数)deep=manyhiddenlayersAlexnet(20
- 深度学习阅读笔记
mossfan
机器学习神经网络学习深度学习nlp
深度学习(DeepLearning):通过组合低层特征形成更加抽象的高层特征(或属性类别),像人脑一样深层次地思考。深度学习与机器学习有什么区别?数据依赖性:二者之间最主要的区别与数据的规模有关。当数据很小时,深度学习表现一般,而传统的机器学习表现较好;随着数据量的增加,深度学习的性能则远远高于机器学习。可解释性:假设使用深度学习给散文自动评分,并接近人类的表现,但没有揭示为什么它给出了这个分数。
- 深度学习期中复习
二战国科大
深度学习python
第一章深度学习和机器学习差异机器学习基本问题:分类,回归,聚类,降维算法:KNN,贝叶斯,决策树,SVM,逻辑回归,随机森林,集成学习改变结构,实现不同的玩法,适应不同场合深度学习主要是解决分类,回归,模拟感知机,多层神经网络,卷积,递归,GAN统一的计算单元,通过变换参数和连接实现不同应用场合1.深度学习与机器学习的主要区别是在于性能。当数据量很少的时候,深度学习的性能并不好,因为深度学习算法需
- [2022]李宏毅深度学习与机器学习第十三讲(必修)-神经网络压缩
走走走,快去看看世界
李宏毅深度学习笔记深度学习深度学习神经网络
[2022]李宏毅深度学习与机器学习第十三讲(必修)-Networkcompression做笔记的目的OutlineNetworkcanbeprunedWhyPruningKnowledgeDistillationEnsembleTemperatureforsoftmaxParameterQuantizationArchitectureDesign-DepthwiseSeparableConvol
- [2022]李宏毅深度学习与机器学习课程内容总结
走走走,快去看看世界
深度学习李宏毅深度学习笔记深度学习人工智能
[2022]李宏毅深度学习与机器学习课程内容总结课程感受第一讲必修ML的三个步骤第一讲选修深度学习发展趋势反向传播从线性模型到神经网络为什么要用正则化技术为什么分类是用交叉熵损失函数而不是SquareErrorDiscriminativeVsGenerative第二讲必修机器学习的任务攻略在训练集上损失较大时应该过拟合的方法N-foldCrossValidationlocalminima和sadd
- [2022]李宏毅深度学习与机器学习第十五讲(必修)-Meta Learning
走走走,快去看看世界
李宏毅深度学习笔记深度学习深度学习人工智能
[2022]李宏毅深度学习与机器学习第十五讲(必修)-MetaLearning做笔记的目的MetaLearningMLvsmetalearningWhatislearnableinlearningalgorithm?做笔记的目的1、监督自己把50多个小时的视频看下去,所以每看一部分内容做一下笔记,我认为这是比较有意义的一件事情。2、路漫漫其修远兮,学习是不断重复和积累的过程。怕自己看完视频不及时做
- [人工智能-深度学习-8]:神经网络基础 - 机器学习、深度学习模型、模型训练
文火冰糖的硅基工坊
人工智能-深度学习神经网络人工智能深度学习
作者主页(文火冰糖的硅基工坊):文火冰糖(王文兵)的博客_文火冰糖的硅基工坊_CSDN博客本文网址:https://blog.csdn.net/HiWangWenBing/article/details/120462734目录第1章白话机器学习第2章机器学习的模型与步骤2.1深度学习与机器学习2.2机器学习的模型第3章建模步骤1-1:研究问题领域的业务需求步骤1-2:用于业务领域的数据进行测试步骤
- jdk tomcat 环境变量配置
Array_06
javajdktomcat
Win7 下如何配置java环境变量
1。准备jdk包,win7系统,tomcat安装包(均上网下载即可)
2。进行对jdk的安装,尽量为默认路径(但要记住啊!!以防以后配置用。。。)
3。分别配置高级环境变量。
电脑-->右击属性-->高级环境变量-->环境变量。
分别配置 :
path
&nbs
- Spring调SDK包报java.lang.NoSuchFieldError错误
bijian1013
javaspring
在工作中调另一个系统的SDK包,出现如下java.lang.NoSuchFieldError错误。
org.springframework.web.util.NestedServletException: Handler processing failed; nested exception is java.l
- LeetCode[位运算] - #136 数组中的单一数
Cwind
java题解位运算LeetCodeAlgorithm
原题链接:#136 Single Number
要求:
给定一个整型数组,其中除了一个元素之外,每个元素都出现两次。找出这个元素
注意:算法的时间复杂度应为O(n),最好不使用额外的内存空间
难度:中等
分析:
题目限定了线性的时间复杂度,同时不使用额外的空间,即要求只遍历数组一遍得出结果。由于异或运算 n XOR n = 0, n XOR 0 = n,故将数组中的每个元素进
- qq登陆界面开发
15700786134
qq
今天我们来开发一个qq登陆界面,首先写一个界面程序,一个界面首先是一个Frame对象,即是一个窗体。然后在这个窗体上放置其他组件。代码如下:
public class First { public void initul(){ jf=ne
- Linux的程序包管理器RPM
被触发
linux
在早期我们使用源代码的方式来安装软件时,都需要先把源程序代码编译成可执行的二进制安装程序,然后进行安装。这就意味着每次安装软件都需要经过预处理-->编译-->汇编-->链接-->生成安装文件--> 安装,这个复杂而艰辛的过程。为简化安装步骤,便于广大用户的安装部署程序,程序提供商就在特定的系统上面编译好相关程序的安装文件并进行打包,提供给大家下载,我们只需要根据自己的
- socket通信遇到EOFException
肆无忌惮_
EOFException
java.io.EOFException
at java.io.ObjectInputStream$PeekInputStream.readFully(ObjectInputStream.java:2281)
at java.io.ObjectInputStream$BlockDataInputStream.readShort(ObjectInputStream.java:
- 基于spring的web项目定时操作
知了ing
javaWeb
废话不多说,直接上代码,很简单 配置一下项目启动就行
1,web.xml
<?xml version="1.0" encoding="UTF-8"?>
<web-app xmlns:xsi="http://www.w3.org/2001/XMLSchema-instance"
xmlns="h
- 树形结构的数据库表Schema设计
矮蛋蛋
schema
原文地址:
http://blog.csdn.net/MONKEY_D_MENG/article/details/6647488
程序设计过程中,我们常常用树形结构来表征某些数据的关联关系,如企业上下级部门、栏目结构、商品分类等等,通常而言,这些树状结构需要借助于数据库完成持久化。然而目前的各种基于关系的数据库,都是以二维表的形式记录存储数据信息,
- maven将jar包和源码一起打包到本地仓库
alleni123
maven
http://stackoverflow.com/questions/4031987/how-to-upload-sources-to-local-maven-repository
<project>
...
<build>
<plugins>
<plugin>
<groupI
- java IO操作 与 File 获取文件或文件夹的大小,可读,等属性!!!
百合不是茶
类 File
File是指文件和目录路径名的抽象表示形式。
1,何为文件:
标准文件(txt doc mp3...)
目录文件(文件夹)
虚拟内存文件
2,File类中有可以创建文件的 createNewFile()方法,在创建新文件的时候需要try{} catch(){}因为可能会抛出异常;也有可以判断文件是否是一个标准文件的方法isFile();这些防抖都
- Spring注入有继承关系的类(2)
bijian1013
javaspring
被注入类的父类有相应的属性,Spring可以直接注入相应的属性,如下所例:1.AClass类
package com.bijian.spring.test4;
public class AClass {
private String a;
private String b;
public String getA() {
retu
- 30岁转型期你能否成为成功人士
bijian1013
成长励志
很多人由于年轻时走了弯路,到了30岁一事无成,这样的例子大有人在。但同样也有一些人,整个职业生涯都发展得很优秀,到了30岁已经成为职场的精英阶层。由于做猎头的原因,我们接触很多30岁左右的经理人,发现他们在职业发展道路上往往有很多致命的问题。在30岁之前,他们的职业生涯表现很优秀,但从30岁到40岁这一段,很多人
- 【Velocity四】Velocity与Java互操作
bit1129
velocity
Velocity出现的目的用于简化基于MVC的web应用开发,用于替代JSP标签技术,那么Velocity如何访问Java代码.本篇继续以Velocity三http://bit1129.iteye.com/blog/2106142中的例子为基础,
POJO
package com.tom.servlets;
public
- 【Hive十一】Hive数据倾斜优化
bit1129
hive
什么是Hive数据倾斜问题
操作:join,group by,count distinct
现象:任务进度长时间维持在99%(或100%),查看任务监控页面,发现只有少量(1个或几个)reduce子任务未完成;查看未完成的子任务,可以看到本地读写数据量积累非常大,通常超过10GB可以认定为发生数据倾斜。
原因:key分布不均匀
倾斜度衡量:平均记录数超过50w且
- 在nginx中集成lua脚本:添加自定义Http头,封IP等
ronin47
nginx lua csrf
Lua是一个可以嵌入到Nginx配置文件中的动态脚本语言,从而可以在Nginx请求处理的任何阶段执行各种Lua代码。刚开始我们只是用Lua 把请求路由到后端服务器,但是它对我们架构的作用超出了我们的预期。下面就讲讲我们所做的工作。 强制搜索引擎只索引mixlr.com
Google把子域名当作完全独立的网站,我们不希望爬虫抓取子域名的页面,降低我们的Page rank。
location /{
- java-3.求子数组的最大和
bylijinnan
java
package beautyOfCoding;
public class MaxSubArraySum {
/**
* 3.求子数组的最大和
题目描述:
输入一个整形数组,数组里有正数也有负数。
数组中连续的一个或多个整数组成一个子数组,每个子数组都有一个和。
求所有子数组的和的最大值。要求时间复杂度为O(n)。
例如输入的数组为1, -2, 3, 10, -4,
- Netty源码学习-FileRegion
bylijinnan
javanetty
今天看org.jboss.netty.example.http.file.HttpStaticFileServerHandler.java
可以直接往channel里面写入一个FileRegion对象,而不需要相应的encoder:
//pipeline(没有诸如“FileRegionEncoder”的handler):
public ChannelPipeline ge
- 使用ZeroClipboard解决跨浏览器复制到剪贴板的问题
cngolon
跨浏览器复制到粘贴板Zero Clipboard
Zero Clipboard的实现原理
Zero Clipboard 利用透明的Flash让其漂浮在复制按钮之上,这样其实点击的不是按钮而是 Flash ,这样将需要的内容传入Flash,再通过Flash的复制功能把传入的内容复制到剪贴板。
Zero Clipboard的安装方法
首先需要下载 Zero Clipboard的压缩包,解压后把文件夹中两个文件:ZeroClipboard.js
- 单例模式
cuishikuan
单例模式
第一种(懒汉,线程不安全):
public class Singleton { 2 private static Singleton instance; 3 pri
- spring+websocket的使用
dalan_123
一、spring配置文件
<?xml version="1.0" encoding="UTF-8"?><beans xmlns="http://www.springframework.org/schema/beans" xmlns:xsi="http://www.w3.or
- 细节问题:ZEROFILL的用法范围。
dcj3sjt126com
mysql
1、zerofill把月份中的一位数字比如1,2,3等加前导0
mysql> CREATE TABLE t1 (year YEAR(4), month INT(2) UNSIGNED ZEROFILL, -> day
- Android开发10——Activity的跳转与传值
dcj3sjt126com
Android开发
Activity跳转与传值,主要是通过Intent类,Intent的作用是激活组件和附带数据。
一、Activity跳转
方法一Intent intent = new Intent(A.this, B.class); startActivity(intent)
方法二Intent intent = new Intent();intent.setCla
- jdbc 得到表结构、主键
eksliang
jdbc 得到表结构、主键
转自博客:http://blog.csdn.net/ocean1010/article/details/7266042
假设有个con DatabaseMetaData dbmd = con.getMetaData(); rs = dbmd.getColumns(con.getCatalog(), schema, tableName, null); rs.getSt
- Android 应用程序开关GPS
gqdy365
android
要在应用程序中操作GPS开关需要权限:
<uses-permission android:name="android.permission.WRITE_SECURE_SETTINGS" />
但在配置文件中添加此权限之后会报错,无法再eclipse里面正常编译,怎么办?
1、方法一:将项目放到Android源码中编译;
2、方法二:网上有人说cl
- Windows上调试MapReduce
zhiquanliu
mapreduce
1.下载hadoop2x-eclipse-plugin https://github.com/winghc/hadoop2x-eclipse-plugin.git 把 hadoop2.6.0-eclipse-plugin.jar 放到eclipse plugin 目录中。 2.下载 hadoop2.6_x64_.zip http://dl.iteye.com/topics/download/d2b
- 如何看待一些知名博客推广软文的行为?
justjavac
博客
本文来自我在知乎上的一个回答:http://www.zhihu.com/question/23431810/answer/24588621
互联网上的两种典型心态:
当初求种像条狗,如今撸完嫌人丑
当初搜贴像条犬,如今读完嫌人软
你为啥感觉不舒服呢?
难道非得要作者把自己的劳动成果免费给你用,你才舒服?
就如同 Google 关闭了 Gooled Reader,那是
- sql优化总结
macroli
sql
为了是自己对sql优化有更好的原则性,在这里做一下总结,个人原则如有不对请多多指教。谢谢!
要知道一个简单的sql语句执行效率,就要有查看方式,一遍更好的进行优化。
一、简单的统计语句执行时间
declare @d datetime ---定义一个datetime的变量set @d=getdate() ---获取查询语句开始前的时间select user_id
- Linux Oracle中常遇到的一些问题及命令总结
超声波
oraclelinux
1.linux更改主机名
(1)#hostname oracledb 临时修改主机名
(2) vi /etc/sysconfig/network 修改hostname
(3) vi /etc/hosts 修改IP对应的主机名
2.linux重启oracle实例及监听的各种方法
(注意操作的顺序应该是先监听,后数据库实例)
&nbs
- hive函数大全及使用示例
superlxw1234
hadoophive函数
具体说明及示例参 见附件文档。
文档目录:
目录
一、关系运算: 4
1. 等值比较: = 4
2. 不等值比较: <> 4
3. 小于比较: < 4
4. 小于等于比较: <= 4
5. 大于比较: > 5
6. 大于等于比较: >= 5
7. 空值判断: IS NULL 5
- Spring 4.2新特性-使用@Order调整配置类加载顺序
wiselyman
spring 4
4.1 @Order
Spring 4.2 利用@Order控制配置类的加载顺序
4.2 演示
两个演示bean
package com.wisely.spring4_2.order;
public class Demo1Service {
}
package com.wisely.spring4_2.order;
public class