文章目录
- 摘要:
- 一、本文工作
- 二、相关工作
- (1)目标检测模型
- 1)backbone
- 2)neck
- 3)Head
- (2)稀疏预测(两阶段)
- (3)数据增强
- (4)IoU
- (5)扩大接受域的模块
- (6)注意力模块
- 1)channel-wise attention
- 2)point-wise attention
- (7)特征集成
- (8)激活函数
- (9)后处理
- 三、方法
- (1)架构选择
- (2)BoF和BoS的选择
- (3)其他的改进
- (4)YOLOv4
- 四、实验
- (1)实验装置
- (2)不同特征对分类器训练的影响
- (3)不同特征对探测器训练的影响
- (4)不同骨干和预训练权重对检测器训练的影响
- (5)不同的 mini-batch size 对检测器训练的影响
- 五、结果
- 六、结论
- 参考
- yolov4论文地址
- yolov4 GitHub链接
摘要:
有许多功能可以提高卷积神经网络(CNN)的准确性。需要在大型数据集上对这些特征的组合进行实际测试,并在理论上证明结果的正确性。某些功能仅在某些模型上运行,并且仅在某些问题上运行,或者仅在小型数据集上运行;而某些功能(例如批归一化和残差连接)适用于大多数模型,任务和数据集。我们假设此类通用功能包括加权残差连接(WRC),跨阶段部分连接(CSP),跨小批量标准化(CmBN),自对抗训练(SAT)和Mish激活。我们使用以下新功能:WRC,CSP,CmBN,SAT,Mish激活,马赛克数据增强,DropBlock正则化和CIoU loss,并结合使用其中的一些功能以实现最新的结果:43.5%的AP(65.7% AP50)。在Tesla V100上,MS COCO数据集的实时速度约为65 FPS。源代码位于https://github.com/AlexeyAB/darknet。
一、本文工作
-
开发了一种有效而强大的对象检测模型。它使每个人都可以使用1080 Ti或2080 Ti GPU训练超快速和准确的物体检测器。
-
验证了在探测器训练过程中最新的 Bag-of-Freebies 和 Bag-of-Specials 检测方法的影响。
-
修改了最先进的方法,使它们更有效,更适合单GPU训练,包括CBN [89],PAN [49],SAM [85]等。
CBN
- Zhuliang Yao, Yue Cao, Shuxin Zheng, Gao Huang, andStephen Lin. Cross-iteration batch normalization.arXivpreprint arXiv:2002.05712, 2020.
PAN
- Shu Liu, Lu Qi, Haifang Qin, Jianping Shi, and Jiaya Jia.Path aggregation network for instance segmentation. InProceedings of the IEEE Conference on Computer Visionand Pattern Recognition (CVPR), pages 8759–8768, 2018.
SAM
- Sanghyun Woo, Jongchan Park, Joon-Young Lee, and InSo Kweon. CBAM: Convolutional block attention module.InProceedings of the European Conference on ComputerVision (ECCV), pages 3–19, 2018.
(1)本文添加的功能
加权残差连接(WRC)
跨阶段部分连接(CSP)
跨小批量标准化(CmBN)
自对抗训练(SAT)
Mish激活
马赛克数据增强
DropBlock正则化
(2)本文对比方法
EfficientDet
- Mingxing Tan, Ruoming Pang, and Quoc V Le. Efficient-Det: Scalable and efficient object detection. InProceedingsof the IEEE Conference on Computer Vision and PatternRecognition (CVPR), 2020.
ASFF
- Songtao Liu, Di Huang, and Yunhong Wang. Learning spa-tial fusion for single-shot object detection.arXiv preprintarXiv:1911.09516, 2019.
YOLOv3
- Joseph Redmon and Ali Farhadi. YOLOv3: An incrementalimprovement.arXiv preprint arXiv:1804.02767, 2018.
ATSS
- Shifeng Zhang, Cheng Chi, Yongqiang Yao, Zhen Lei, andStan Z Li. Bridging the gap between anchor-based andanchor-free detection via adaptive training sample selec-tion. InProceedings of the IEEE Conference on ComputerVision and Pattern Recognition (CVPR), 2020.
CenterMask
- Youngwan Lee and Jongyoul Park. CenterMask: Real-timeanchor-free instance segmentation. InProceedings of theIEEE Conference on Computer Vision and Pattern Recog-nition (CVPR), 2020.
二、相关工作
- 现代检测器通常由两部分组成,一个是在ImageNet上经过预训练的骨架(backbone),另一个是用来预测物体的类别和边界框的头部(head)。对于在GPU平台上运行的那些检测器,其主干可能是VGG ,ResNet ,ResNeXt 或DenseNet 。对于在CPU平台上运行的那些检测器,其主干可以是SqueezeNet ,MobileNet 或ShuffleNet 。至于头部,通常分为两类,即一级目标检测器和二级目标检测器。最有代表性的两级对象检测器是R-CNN 系列,包括fast R-CNN ,faster R-CNN ,R-FCN 和Libra R-CNN 。使二级对象检测器成为无锚对象检测器也是可以的,例如RepPoints 。对于一级目标检测器,最具代表性的模型是YOLO ,SSD 和RetinaNet 。近年来,开发了无锚的一级物体检测器。这种检测器是CenterNet ,CornerNet ,FCOS 等。近年来开发的目标检测器通常在骨架和头部之间插入一些层,这些层通常用于收集不同阶段的特征图。我们可以称其为对象检测器的颈部。通常,颈部由几个自下而上的路径和几个自上而下的路径组成。配备此机制的网络包括功能金字塔网络(FPN),路径聚合网络(PAN),BiFPN 和NAS-FPN 。
(1)目标检测模型

1)backbone
VGG
- Karen Simonyan and Andrew Zisserman. Very deep convo-lutional networks for large-scale image recognition.arXivpreprint arXiv:1409.1556, 2014.
Resnet-50
- Kaiming He, Xiangyu Zhang, Shaoqing Ren, and Jian Sun.Deep residual learning for image recognition. In Proceedings of the IEEE Conference on Computer Vision and Pattern Recognition (CVPR), pages 770–778, 2016.
ResNetXt-101
- Saining Xie, Ross Girshick, Piotr Doll ́ar, Zhuowen Tu, andKaiming He. Aggregated residual transformations for deepneural networks. InProceedings of the IEEE Conference onComputer Vision and Pattern Recognition (CVPR), pages1492–1500, 2017.
Darknet53,YOLOv3
- Joseph Redmon and Ali Farhadi. YOLOv3: An incrementalimprovement.arXiv preprint arXiv:1804.02767, 2018.
SpineNet
- Xianzhi Du, Tsung-Yi Lin, Pengchong Jin, Golnaz Ghiasi,Mingxing Tan, Yin Cui, Quoc V Le, and Xiaodan Song.SpineNet: Learning scale-permuted backbone for recog-nition and localization.arXiv preprint arXiv:1912.05027,2019.
EfficientNet-B0 / B7
- Mingxing Tan and Quoc V Le. EfficientNet: Rethinkingmodel scaling for convolutional neural networks. InPro-ceedings of International Conference on Machine Learning(ICML), 2019.
CSPResNeXt50
- Chien-Yao Wang, Hong-Yuan Mark Liao, Yueh-Hua Wu,Ping-Yang Chen, Jun-Wei Hsieh, and I-Hau Yeh. CSPNet:A new backbone that can enhance learning capability ofcnn.Proceedings of the IEEE Conference on Computer Vi-sion and Pattern Recognition Workshop (CVPR Workshop),2020.
2)neck
1. 附加块
SPP
- Kaiming He, Xiangyu Zhang, Shaoqing Ren, and Jian Sun.Spatial pyramid pooling in deep convolutional networks forvisual recognition.IEEE Transactions on Pattern Analy-sis and Machine Intelligence (TPAMI), 37(9):1904–1916,2015.
ASPP
- Liang-Chieh Chen, George Papandreou, Iasonas Kokkinos,Kevin Murphy, and Alan L Yuille. DeepLab: Semantic im-age segmentation with deep convolutional nets, atrous con-volution, and fully connected CRFs.IEEE Transactionson Pattern Analysis and Machine Intelligence (TPAMI),40(4):834–848, 2017.
RFB
- Songtao Liu, Di Huang, et al. Receptive field block net foraccurate and fast object detection. InProceedings of theEuropean Conference on Computer Vision (ECCV), pages385–400, 2018.
SAM
- Sanghyun Woo, Jongchan Park, Joon-Young Lee, and InSo Kweon. CBAM: Convolutional block attention module.InProceedings of the European Conference on ComputerVision (ECCV), pages 3–19, 2018.
2. 路径聚合块
FPN
- Tsung-Yi Lin, Piotr Doll ́ar, Ross Girshick, Kaiming He,Bharath Hariharan, and Serge Belongie. Feature pyramidnetworks for object detection. InProceedings of the IEEEConference on Computer Vision and Pattern Recognition(CVPR), pages 2117–2125, 2017.
PANet
- Shu Liu, Lu Qi, Haifang Qin, Jianping Shi, and Jiaya Jia.Path aggregation network for instance segmentation. InProceedings of the IEEE Conference on Computer Visionand Pattern Recognition (CVPR), pages 8759–8768, 2018.
Bi-FPN
- Mingxing Tan, Ruoming Pang, and Quoc V Le. Efficient-Det: Scalable and efficient object detection. InProceedingsof the IEEE Conference on Computer Vision and PatternRecognition (CVPR), 2020.
NAS-FPN
- Golnaz Ghiasi, Tsung-Yi Lin, and Quoc V Le. NAS-FPN:Learning scalable feature pyramid architecture for objectdetection. InProceedings of the IEEE Conference on Com-puter Vision and Pattern Recognition (CVPR), pages 7036–7045, 2019.
全连接FPN,BiFPN
- Mingxing Tan, Ruoming Pang, and Quoc V Le. Efficient-Det: Scalable and efficient object detection. InProceedingsof the IEEE Conference on Computer Vision and PatternRecognition (CVPR), 2020.
ASFF
- Songtao Liu, Di Huang, and Yunhong Wang. Learning spa-tial fusion for single-shot object detection.arXiv preprintarXiv:1911.09516, 2019.
SFAM
- Qijie Zhao, Tao Sheng, Yongtao Wang, Zhi Tang, YingChen, Ling Cai, and Haibin Ling. M2det: A single-shotobject detector based on multi-level feature pyramid net-work. InProceedings of the AAAI Conference on ArtificialIntelligence (AAAI), volume 33, pages 9259–9266, 2019.
3)Head
1. 有锚
RPN
- Shaoqing Ren, Kaiming He, Ross Girshick, and Jian Sun.Faster R-CNN: Towards real-time object detection with re-gion proposal networks. InAdvances in Neural InformationProcessing Systems (NIPS), pages 91–99, 2015.
YOLO123
-
Joseph Redmon, Santosh Divvala, Ross Girshick, and AliFarhadi. You only look once: Unified, real-time object de-tection. InProceedings of the IEEE Conference on Com-puter Vision and Pattern Recognition (CVPR), pages 779–788, 2016.
-
Joseph Redmon and Ali Farhadi. YOLO9000: better, faster,stronger. InProceedings of the IEEE Conference on Com-puter Vision and Pattern Recognition (CVPR), pages 7263–7271, 2017.
-
Joseph Redmon and Ali Farhadi. YOLOv3: An incrementalimprovement.arXiv preprint arXiv:1804.02767, 2018.
SSD
- Wei Liu, Dragomir Anguelov, Dumitru Erhan, ChristianSzegedy, Scott Reed, Cheng-Yang Fu, and Alexander CBerg. SSD: Single shot multibox detector. InProceedingsof the European Conference on Computer Vision (ECCV),pages 21–37, 2016.
RetinaNet
- Tsung-Yi Lin, Priya Goyal, Ross Girshick, Kaiming He,and Piotr Doll ́ar. Focal loss for dense object detection. InProceedings of the IEEE International Conference on Com-puter Vision (ICCV), pages 2980–2988, 2017.
2. 无锚
CornerNet
- Hei Law and Jia Deng. CornerNet: Detecting objects aspaired keypoints. InProceedings of the European Confer-ence on Computer Vision (ECCV), pages 734–750, 2018
CenterNet
- Kaiwen Duan, Song Bai, Lingxi Xie, Honggang Qi, Qing-ming Huang, and Qi Tian. CenterNet: Keypoint triplets forobject detection. InProceedings of the IEEE InternationalConference on Computer Vision (ICCV), pages 6569–6578,2019.
MatrixNet
- Abdullah Rashwan, Agastya Kalra, and Pascal Poupart.Matrix Nets: A new deep architecture for object detection.InProceedings of the IEEE International Conference onComputer Vision Workshop (ICCV Workshop), pages 0–0,2019.
FCOS
- Zhi Tian, Chunhua Shen, Hao Chen, and Tong He. FCOS:Fully convolutional one-stage object detection. InProceed-ings of the IEEE International Conference on Computer Vi-sion (ICCV), pages 9627–9636, 2019.
(2)稀疏预测(两阶段)
Faster R-CNN
- Shaoqing Ren, Kaiming He, Ross Girshick, and Jian Sun.Faster R-CNN: Towards real-time object detection with re-gion proposal networks. InAdvances in Neural InformationProcessing Systems (NIPS), pages 91–99, 2015.
R-FCN
- Jifeng Dai, Yi Li, Kaiming He, and Jian Sun. R-FCN:Object detection via region-based fully convolutional net-works. InAdvances in Neural Information Processing Sys-tems (NIPS), pages 379–387, 2016.
Mask RCNN(基于锚)
- Kaiming He, Georgia Gkioxari, Piotr Doll ́ar, and Ross Gir-shick. Mask R-CNN. InProceedings of the IEEE In-ternational Conference on Computer Vision (ICCV), pages2961–2969, 2017.
RepPoints(无锚)
- Ze Yang, Shaohui Liu, Han Hu, Liwei Wang, and StephenLin. RepPoints: Point set representation for object detec-tion. InProceedings of the IEEE International Conferenceon Computer Vision (ICCV), pages 9657–9666, 2019.
Usually, a conventional object detector is trained offline. Therefore, researchers always like to take this advantage and develop better training methods which can make the object detector receive better accuracy without increasing the inference cost. We call these methods that only change the training strategy or only increase the training cost as “bag of freebies.”
(3)数据增强
- 目的是增加输入图像的可变性,从而使设计的物体检测模型对从不同环境获得的图像具有更高的鲁棒性。例如,光度畸变和几何畸变是两种常用的数据增强方法,它们无疑有益于物体检测任务。在处理光度失真时,我们会调整图像的亮度,对比度,色相,饱和度和噪点。对于几何变形,我们添加了随机缩放,裁剪,翻转和旋转。
(4)IoU
-
IoU loss
Jiahui Yu, Yuning Jiang, Zhangyang Wang, Zhimin Cao,and Thomas Huang. UnitBox: An advanced object detec-tion network. InProceedings of the 24th ACM internationalconference on Multimedia, pages 516–520, 2016
它考虑了 predicted BBox 区域和 ground truth BBox 区域的覆盖范围。 IoU损失计算过程将通过使用ground truth 执行 IoU,然后将生成的结果连接到整个代码中,从而触发BBox的四个坐标点的计算。它可以解决传统方法计算{x,y,w,h)的L1和L2损耗时,损耗会随比例增大的问题。
-
GIoU loss
Hamid Rezatofighi, Nathan Tsoi, JunYoung Gwak, AmirSadeghian, Ian Reid, and Silvio Savarese. Generalized in-tersection over union: A metric and a loss for boundingbox regression. InProceedings of the IEEE Conference onComputer Vision and Pattern Recognition (CVPR), pages658–666, 2019.
GioU损失除了覆盖区域外还包括对象的形状和方向。他们提出了寻找可以同时覆盖 predicted BBox 和 ground truth BBox 的最小面积的BBox,并使用此BBox作为分母来代替最初用于IoU损失的分母。
-
DIoU loss
Zhaohui Zheng, Ping Wang, Wei Liu, Jinze Li, RongguangYe, and Dongwei Ren. Distance-IoU Loss: Faster and bet-ter learning for bounding box regression. InProceedingsof the AAAI Conference on Artificial Intelligence (AAAI),2020.
它还考虑了对象中心的距离
-
CIoUloss
另一方面,它同时考虑了重叠区域,中心点之间的距离和纵横比。 在BBox回归问题上,CIoU可以实现更好的收敛速度和准确性。
For those plugin modules and post-processing methodsthat only increase the inference cost by a small amount but can significantly improve the accuracy of object detec-tion, we call them “bag of specials”.
(5)扩大接受域的模块
-
SPP
Kaiming He, Xiangyu Zhang, Shaoqing Ren, and Jian Sun.Spatial pyramid pooling in deep convolutional networks forvisual recognition.IEEE Transactions on Pattern Analy-sis and Machine Intelligence (TPAMI), 37(9):1904–1916,2015.
SPP将SPM集成到CNN中,并使用最大池化操作而不是bag-of-word操作。
SPP模块源于 Spatial Pyramid Matching:
Svetlana Lazebnik, Cordelia Schmid, and Jean Ponce. Be-yond bags of features: Spatial pyramid matching for recog-nizing natural scene categories. InProceedings of the IEEEConference on Computer Vision and Pattern Recognition(CVPR), volume 2, pages 2169–2178. IEEE, 2006.
SPM的原始方法是将特征图分割成几个dxd不等的块,其中 d 可以为{1,2,3,…},从而形成空间金字塔,然后提取词袋特征。
-
ASPP
Liang-Chieh Chen, George Papandreou, Iasonas Kokkinos,Kevin Murphy, and Alan L Yuille. DeepLab: Semantic im-age segmentation with deep convolutional nets, atrous con-volution, and fully connected CRFs.IEEE Transactionson Pattern Analysis and Machine Intelligence (TPAMI),40(4):834–848, 2017.
ASPP模块和改进的SPP模块之间的操作差异主要在于在膨胀卷积运算中原始k×k内核大小,步幅的最大池等于1到几个3×3内核大小,膨胀比等于k,步幅等于1 。
-
RFB
Songtao Liu, Di Huang, et al. Receptive field block net foraccurate and fast object detection. InProceedings of theEuropean Conference on Computer Vision (ECCV), pages385–400, 2018.
RFB仅需额外花费7%的推断时间即可将MS COCO上SSD的AP50提高5.7%
(6)注意力模块
1)channel-wise attention
- Squeeze-and-Excitation(SE)
Jie Hu, Li Shen, and Gang Sun. Squeeze-and-excitationnetworks. InProceedings of the IEEE Conference on Com-puter Vision and Pattern Recognition (CVPR), pages 7132–7141, 2018.
尽管SE模块可以以仅将计算工作量增加2%的代价将ImNet.NetNet图像分类任务中的ResNet50的功能提高1%的top-1准确性,但是在GPU上通常会增加大约10%的推理时间,因此更适合在移动设备中使用。
2)point-wise attention
- Spatial Attention Module(SAM)
Sanghyun Woo, Jongchan Park, Joon-Young Lee, and InSo Kweon. CBAM: Convolutional block attention module.InProceedings of the European Conference on ComputerVision (ECCV), pages 3–19, 2018.
对于SAM,它只需要支付0.1%的额外计算费用,就可以将ResNet50-SE在ImageNet图像分类任务上提高0.5%的top-1准确性。 最棒的是,它根本不影响GPU上的推理速度。
(7)特征集成
-
在特征集成方面,早期的实践是使用 跳过连接(skip connection)或超列(hyper-column)将低层物理特征集成到高层语义特征。
skip connection
Jonathan Long, Evan Shelhamer, and Trevor Darrell. Fullyconvolutional networks for semantic segmentation. InPro-ceedings of the IEEE Conference on Computer Vision andPattern Recognition (CVPR), pages 3431–3440, 2015. 4[52] Ilya Loshchilov and Frank Hutter.SGDR: Stochas-tic gradient descent with warm restarts.arXiv preprintarXiv:1608.03983, 2016.
hyper-column
Bharath Hariharan, Pablo Arbel ́aez, Ross Girshick, andJitendra Malik.Hypercolumns for object segmentationand fine-grained localization. InProceedings of the IEEEConference on Computer Vision and Pattern Recognition(CVPR), pages 447–456, 2015.
-
由于诸如FPN的多尺度预测方法已变得流行,因此提出了许多集成了不同特征金字塔的轻量级模块。 此类模块包括SFAM ,ASFF 和BiFPN。 SFAM的主要思想是使用SE模块对多尺度级联的特征图执行通道级的重新加权。 对于ASFF,它使用softmax作为逐点级别重新加权,然后添加不同比例的特征图。在BiFPN中,提出了多输入加权残差连接以执行按比例级别重新加权,然后添加 不同的规模。
SFAM
Qijie Zhao, Tao Sheng, Yongtao Wang, Zhi Tang, YingChen, Ling Cai, and Haibin Ling. M2det: A single-shotobject detector based on multi-level feature pyramid net-work. InProceedings of the AAAI Conference on ArtificialIntelligence (AAAI), volume 33, pages 9259–9266, 2019.
ASFF
Songtao Liu, Di Huang, and Yunhong Wang. Learning spa-tial fusion for single-shot object detection.arXiv preprintarXiv:1911.09516, 2019.
BiFPN
Mingxing Tan, Ruoming Pang, and Quoc V Le. Efficient-Det: Scalable and efficient object detection. InProceedingsof the IEEE Conference on Computer Vision and PatternRecognition (CVPR), 2020
(8)激活函数
- 在2010年,Nair和Hin-ton提出了ReLU,以基本上解决传统tanh和S形激活函数中经常遇到的梯度消失问题。
Vinod Nair and Geoffrey E Hinton. Rectified linear unitsimprove restricted boltzmann machines. InProceedingsof International Conference on Machine Learning (ICML),pages 807–814, 2010
- 其他激活函数
- LReLU和PReLU的主要目的是解决当输出小于零时ReLU的梯度为零的问题。 至于ReLU6和hard-Swish,它们是专门为量化网络设计的。 为了对神经网络进行自归一化,提出了SELU激活函数来满足这一目标。 要注意的一件事是,Swish和Mish都具有连续可区分的激活功能。
LReLU
Andrew L Maas, Awni Y Hannun, and Andrew Y Ng. Rec-tifier nonlinearities improve neural network acoustic mod-els. InProceedings of International Conference on Ma-chine Learning (ICML), volume 30, page 3, 2013
PReLU
Kaiming He, Xiangyu Zhang, Shaoqing Ren, and Jian Sun.Delving deep into rectifiers: Surpassing human-level per-formance on ImageNet classification. InProceedings ofthe IEEE International Conference on Computer Vision(ICCV), pages 1026–1034, 2015.
ReLU6
Andrew G Howard, Menglong Zhu, Bo Chen, DmitryKalenichenko, Weijun Wang, Tobias Weyand, Marco An-dreetto, and Hartwig Adam. MobileNets: Efficient con-volutional neural networks for mobile vision applications.arXiv preprint arXiv:1704.04861, 2017.
Scaled ExponentialLinear Unit (SELU)
G ̈unter Klambauer, Thomas Unterthiner, Andreas Mayr,and Sepp Hochreiter. Self-normalizing neural networks.InAdvances in Neural Information Processing Systems(NIPS), pages 971–980, 2017
Swish
Prajit Ramachandran, Barret Zoph, and Quoc V Le.Searching for activation functions.arXiv preprintarXiv:1710.05941, 2017.
hard-Swish
Andrew Howard, Mark Sandler, Grace Chu, Liang-ChiehChen, Bo Chen, Mingxing Tan, Weijun Wang, Yukun Zhu,Ruoming Pang, Vijay Vasudevan, et al. Searching for Mo-bileNetV3. InProceedings of the IEEE International Con-ference on Computer Vision (ICCV), 2019.
Mish
Diganta Misra.Mish:A self regularized non-monotonic neural activation function.arXiv preprintarXiv:1908.08681, 2019.
(9)后处理
-
NMS(非极大抑制)
在基于深度学习的对象检测中通常使用的后处理方法是NMS,它可以用于过滤那些无法预测相同对象的BBox,并仅保留响应速度更快的候选BBox。NMS尝试改进的方法与优化目标函数的方法一致。 NMS提出的原始方法没有考虑上下文信息。
-
在R-CNN中添加分类置信度作为参考,并根据置信度分数的顺序,按从高分到低分的顺序执行贪婪的NMS。
Ross Girshick, Jeff Donahue, Trevor Darrell, and JitendraMalik.Rich feature hierarchies for accurate object de-tection and semantic segmentation. InProceedings of theIEEE Conference on Computer Vision and Pattern Recog-nition (CVPR), pages 580–587, 2014.
-
soft NMS
Navaneeth Bodla, Bharat Singh, Rama Chellappa, andLarry S Davis. Soft-NMS–improving object detection withone line of code. InProceedings of the IEEE InternationalConference on Computer Vision (ICCV), pages 5561–5569,2017.
它考虑了一个问题,即物体的遮挡可能会导致带有IoU分数的贪婪NMS中置信度得分的下降。
-
DIoU NMS
Zhaohui Zheng, Ping Wang, Wei Liu, Jinze Li, RongguangYe, and Dongwei Ren. Distance-IoU Loss: Faster and bet-ter learning for bounding box regression. InProceedingsof the AAAI Conference on Artificial Intelligence (AAAI),2020.
DIoU NMS [99]开发人员的思维方式是在soft NMS的基础上将中心点距离的信息添加到BBox筛选过程中。
-
值得一提的是,由于上述后处理方法都没有直接涉及捕获的图像特征,因此在随后的无锚定方法开发中不再需要后处理。
三、方法
- 对于GPU,使用少量的(1-8)组卷积层:CSPResNeXt50 / CSPDarknet53
- 对于VPU ,使用分组卷积,但是不再使用 Squeeze-and-excitemen(SE)模块 - 它包括以下模型:EfficientNet-lite / MixNet / GhostNet / MobileNetV3
MixNet
Mingxing Tan and Quoc V Le. MixNet: Mixed depthwiseconvolutional kernels. InProceedings of the British Ma-chine Vision Conference (BMVC), 2019
GhostNet
Kai Han, Yunhe Wang, Qi Tian, Jianyuan Guo, ChunjingXu, and Chang Xu. GhostNet: More features from cheapoperations. InProceedings of the IEEE Conference onComputer Vision and Pattern Recognition (CVPR), 2020.
(1)架构选择
我们的目标是在输入网络分辨率,卷积层数,参数数( f i l t e r _ s i z e 2 ∗ 过 滤 器 ∗ 通 道 / 组 filter\_size^2 * 过滤器 * 通道 / 组 filter_size2∗过滤器∗通道/组)和层输出(过滤器)数目之间找到最佳平衡。 例如,大量研究表明,在ILSVRC2012(ImageNet)数据集上的对象分类方面,CSPResNext50比CSPDarknet53更好。 但是,相反,在检测MS COCO数据集上的对象方面,CSPDarknet53比CSPResNext50更好。
ILSVRC2012 (ImageNet) dataset
Jia Deng, Wei Dong, Richard Socher, Li-Jia Li, Kai Li,and Li Fei-Fei. ImageNet: A large-scale hierarchical im-age database. InProceedings of the IEEE Conference onComputer Vision and Pattern Recognition (CVPR), pages248–255, 2009.
MS COCO dataset
Tsung-Yi Lin, Michael Maire, Serge Belongie, JamesHays, Pietro Perona, Deva Ramanan, Piotr Doll ́ar, andC Lawrence Zitnick. Microsoft COCO: Common objectsin context. InProceedings of the European Conference onComputer Vision (ECCV), pages 740–755, 2014.
下一个目标是为不同的检测器级别从不同的主干级别中选择其他块以增加接收场和参数聚集的最佳方法。例如:FPN,PAN,ASFF,BiFPN。
对于分类而言最佳的参考模型对于检测器而言并不总是最佳的。 与分类器相比,检测器需要满足以下要求:
- 更高的输入网络大小(分辨率)–用于检测多个小型物体
- 层数更多 – 更高的接收域可以覆盖增加的输入网络规模
- 更多参数–具有更大的模型容量,可在单个图像中检测不同大小的多个对象
假设来说,我们可以假设应该选择一个具有更大的接收域大小(具有更多的卷积层3×3)和更多的参数的模型作为主干。 表1显示了CSPResNeXt50,CSPDarknet53和Effi-cientNet B3的信息。 CSPResNext50仅包含16个卷积层3×3,a425×425接收域和20.6M参数,而CSPDarknet53包含29个卷积层3×3,a725×725接收域和27.6M参数。 这种理论上的论证,再加上我们的大量实验,表明CSPDarknet53神经网络是两者作为检测器骨干的最佳模型。

不同大小的接收域的影响总结如下:
- 取决于物体尺寸 - 允许查看整个对象
- 取决于网络规模 - 允许查看对象周围的上下文
- 超出网络规模 - 增加图像点和最终激活之间的连接数
作者在CSPDarknet53上添加了SPP块,因为它显着增加了接收域,分离出了最重要的上下文特征,并且几乎没有降低网络运行速度。 作者使用PANet作为来自不同主干级别,针对不同检测器级别的参数聚合方法,而不是YOLOv3中使用的FPN。
作者选择CSPDarknet53骨干网,SPP附加模块,PANet路径聚合颈部和YOLOv3(基于锚)头作为YOLOv4的体系结构。 将来,作者计划大幅扩展探测器的Bag of Freebies(BoF)的内容,从理论上讲,它可以解决一些问题并提高探测器的准确性,并以实验方式依次检查每个功能的影响
(2)BoF和BoS的选择
(3)其他的改进
为了使设计的检测器更适合在单个GPU上进行训练,我们进行了以下附加设计和改进:
-
介绍了一种新的数据增强Mosaic和自我专业训练(SAT)方法。
-
在应用遗传算法时选择最佳超参数。
-
作者修改了一些现有方法以使我们的设计适合进行有效的训练和检测 - modified SAM,modified PAN和交叉小批量标准化(CmBN)。
-
Mosaic 代表了一种新的数据增强方法,该方法混合了4个训练图像。 因此,有4种不同的环境混合,而CutMix仅混合2个输入图像。 这允许检测对象超出其正常上下文。 此外,批量归一化还根据每层上的4张不同图像计算激活统计信息。这大大减少了对大的 mini-batch size 的需求。

-
自我专业训练(SAT) 也代表了一种新的数据增强技术,该技术可在2个前向和后向阶段中进行操作。 在第一阶段,神经网络会更改原始图像,而不是网络权重。 这样,神经网络会对其自身执行对抗性攻击,从而更改原始图像以产生一种欺骗,即图像上没有所需的对象。 在第二阶段,训练神经网络以正常方式检测该修改图像上的物体。

-
CmBN表示CBN修改版本,如图4所示,定义为 Cross mini-Batch Normalization(CmBN)。 这仅收集单个批次中的mini-batche之间的统计信息。
-
作者将SAM从spatial-wise attention 改为point-wise attention,并将PAN的快捷连接替换为串联,分别如图5和图6所示。

(4)YOLOv4
-
在本节中,我们将详细介绍YOLOv4.
- Backbone: CSPDarknet53 [81]
- Neck: SPP [25], PAN [49]
- Head: YOLOv3
-
YOLOv4使用:
- 支持主干的 Bag of Freebies(BoF):CutMix和Mosaic数据增强,DropBlock正则化,类标签平滑
- 专为骨干网设计的 Bag of Specials(BoS):Mish激活,跨阶段部分连接(CSP),多输入加权剩余连接(MiWRC)
- 用于检测器的 Bag of Freebies(BoF):CIoU损失,CmBN,DropBlock正则化,镶嵌数据增强,自我专家训练,消除网格敏感性,对单个地面使用多个锚,余弦退火调度器[52],最佳超参数,随机训练形状
- 用于检测器的 Bag of Specials(BoS):灭碟激活,SPP块,SAM块,PAN路径聚集块,DIoU-NMS
四、实验
我们测试了不同训练改进技术对ImageNet(ILSVRC 2012 val)数据集上分类器准确性的影响,然后对MS COCO(test-dev 2017)数据集上检测器的准确性进行了影响。
(1)实验装置
-
在ImageNet图像分类实验中,默认的超参数如下:训练步骤为8,000,000;batch size 和 mini-batch size 分别为128和32;采用多项式衰减学习速率调度策略,初始学习速率为0.1;预热步骤为1000; 动量和权重偏差分别设置为 0.9 和 0.005 。我们所有的BoS实验都使用与默认设置相同的超参数,并且在BoF实验中,我们添加了额外的50%训练步骤。在BoF实验中,我们验证了MixUp,CutMix,Mosaic,模糊数据增强和标签平滑正则化方法。 在BoS实验中,我们比较了LReLU,Swish和Mishactivation功能的效果。 所有实验均使用1080Ti或2080 Ti GPU进行培训。
-
在MS COCO对象检测实验中,默认的超参数如下:训练步骤为500,500; 采用步阶衰减学习率调度策略,初始学习率为0.01,分别在400,000步和450,000步处乘以0.1。动量和重量衰减分别设置为0.9和0.0005。 所有架构都使用单个GPU以64的批处理大小执行多尺度训练,而最小批处理大小为8或4,具体取决于架构和GPU内存限制。 动量和重量衰减分别设置为0.9和0.0005。 所有架构都使用single GPU以64的批处理大小执行多尺度训练,而 mini-batch size 为8或4则取决于架构和GPU内存限制。除了使用遗传算法进行超参数搜索实验外,所有其他实验均使用默认设置。 遗传算法使用YOLOv3-SPP训练GIoU损失,并搜索300个epochs的最小值5k集。对于遗传算法实验,我们采用搜索学习率0.00261,动量0.949,IoU阈值分配地面实况0.213,损失归一化器0.07。
我们已经验证了许多BoF,包括消除网格敏感性,mosaic数据增强,IoU阈值,遗传算法,类标签平滑,交叉小批量标准化,自对抗训练,余弦退火调度程序,动态小批量大小,DropBlock ,优化的锚点,不同类型的IoU损失。
We have verified a largenumber of BoF, including grid sensitivity elimination, mosaic data augmentation, IoU threshold, genetic algorithm,class label smoothing, cross mini-batch normalization, self-adversarial training, cosine annealing scheduler, dynamicmini-batch size, DropBlock, Optimized Anchors, differentkind of IoU losses.
我们还对各种BoS进行了实验,包括Mish,SPP,SAM,RFB,BiFPN和高斯YOLO [8]。 对于所有实验,我们仅使用一个GPU进行训练,因此未使用可优化多个GPU的诸如syncBN之类的技术。
(2)不同特征对分类器训练的影响
- 首先,我们研究不同特征对分类器训练的影响;具体来说,如图9所示,类标签平滑的影响,不同数据增强技术的影响,双边模糊,MixUp,CutMix和Mosaic的影响(如图7所示)以及诸如Leaky-ReLU(默认情况下)之类的不同激活的影响,Swish和Mish。
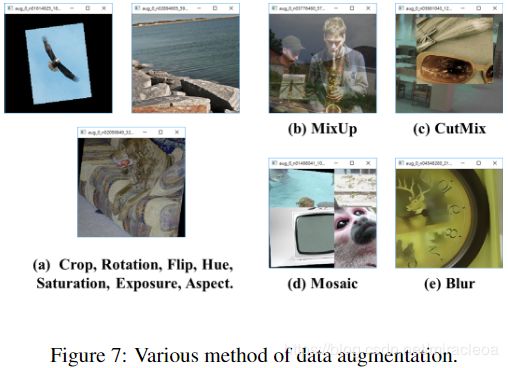
- 在我们的实验中,如表2所示,通过引入以下功能提高了分类器的准确性:CutMix和Mosaic数据增强,Class label平滑和Mish激活。 结果,我们用于分类器训练的BoF-backbone((Bag of Freebies)包括以下内容:CutMix 和 Mosaic 数据增强和类标签平滑。 此外,我们使用Mish激活作为补充选项,如表2和表3所示:
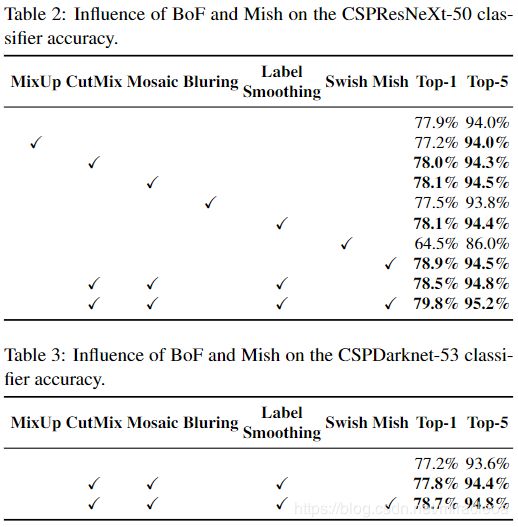
(3)不同特征对探测器训练的影响
- 进一步的研究涉及到不同的Bag-of-Freebies(BoF-detector)对探测器训练精度的影响,如表4所示。
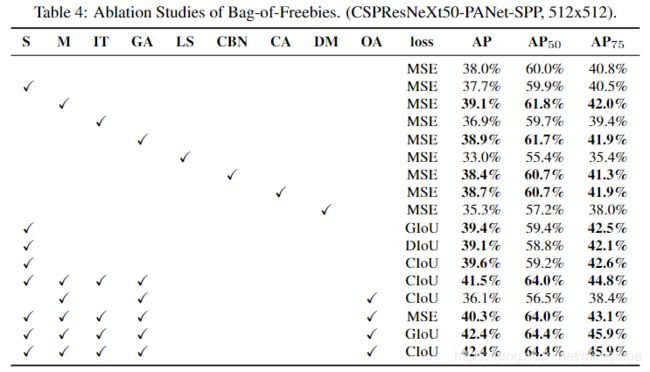
我们通过研究在不影响FPS的情况下提高探测器准确性的各种功能,大大扩展了BoF列表:
-
S: 消除网格敏感性,YOLOv3使用方程 b x = σ ( t x ) + c x , b y = σ ( t y ) + c y b_x = σ(t_x) + c_x,b_y = \sigma(t_y) + c_y bx=σ(tx)+cx,by=σ(ty)+cy,其中 c x c_x cx和 c y c_y cy始终是整数来评估对象坐标,因此,对于接近 c x 或 c x + 1 c_x 或 c_x + 1 cx或cx+1 值的 b x b_x bx值,需要非常高的 t x t_x tx绝对值。 我们通过将sigmoid乘以超过1.0的因子来解决此问题,从而消除了无法检测到物体的网格的影响。
-
M:Mosaic data augmentation 马赛克数据增强 - 在训练过程中使用4图像马赛克代替单个图像
-
IT:IoU threshold IoU阈值 - 将多个锚点用于ground truth IoU(truth,anchor)> IoU_threshold
-
GA:Genetic algorithms 遗传算法 - 使用遗传算法在前10%的时间段进行网络训练期间选择最佳超参数
-
LS:Class label smoothing 类标签平滑 - 使用类标签平滑进行sigmoid激活
-
CBN:CmBN - 使用交叉微型批处理规范化来收集整个批处理中的统计信息,而不是在单个微型批处理中收集统计信息
-
CA:Cosine annealing scheduler 余弦退火调度器 - 在正弦曲线训练中改变学习率
-
DM: Dynamic mini-batch size 动态mini-batch size - 通过使用随机训练形状在小分辨率训练期间自动增加 mini-batch 大小
-
OA: Optimized Anchors 优化的锚点 - 使用优化的锚点以 512x512 网络分辨率进行训练
-
GIoU, CIoU, DIoU, MSE - 使用不同的损失算法进行边界框回归
进一步的研究涉及不同的 Bag-of-Specials (BoS-detector)对探测器训练准确性的影响,包括PAN,RFB,SAM,高斯YOLO(G)和ASFF,如表5所示。在我们的实验中, 使用SPP,PAN和SAM时,检测器可获得最佳性能。
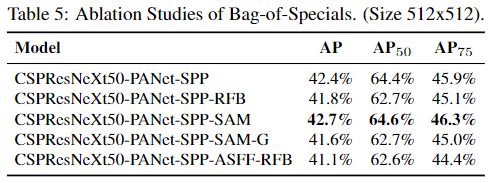
(4)不同骨干和预训练权重对检测器训练的影响
进一步,我们研究了不同骨干模型对检测器精度的影响,如表6所示。请注意,具有最佳分类精度的模型在检测器精度方面并不总是最佳的
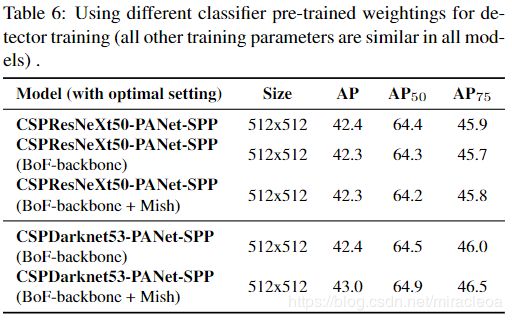
首先,尽管与CSPDarknet53模型相比,经过不同功能训练的CSPResNeXt-50模型的分类准确性更高,但CSPDarknet53模型在对象检测方面显示出更高的准确性。
其次,使用BoF和Mish进行CSPResNeXt50分类器训练会提高其分类准确性,但是将这些预先训练的权重进一步应用于检测器训练会降低检测器准确性。 然而,将BoF和Mish用于CSPDarknet53分类器训练可以提高分类器和使用该分类器预训练加权的检测器的准确性。 最终结果是,与CSPResNeXt50相比,主干CSPDarknet53更适合于检测器。
我们观察到,由于各种改进,CSPDarknet53模型具有更大的能力来提高检测器精度。
(5)不同的 mini-batch size 对检测器训练的影响
最后,我们分析了使用不同 mini-batch sizes 训练的模型获得的结果,结果显示在表7中。从表7中显示的结果中,我们发现在添加BoF和BoS训练策略之后,mini-batch size 几乎没有影响在检测器的性能上。 该结果表明,在引入BoF和BoS之后,不再需要使用昂贵的GPU进行训练。 换句话说,任何人都只能使用传统的GPU来训练出色的探测器。
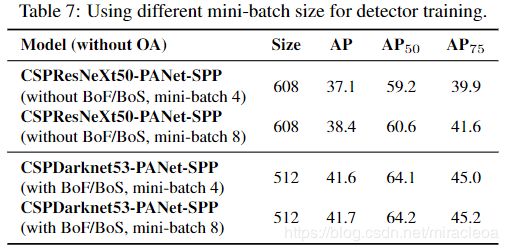
五、结果
- 图8显示了与其他最新对象探测器获得的结果的比较.我们的YOLOv4位于Pareto 最优曲线上,在速度和准确性方面均优于最快,最准确的探测器。
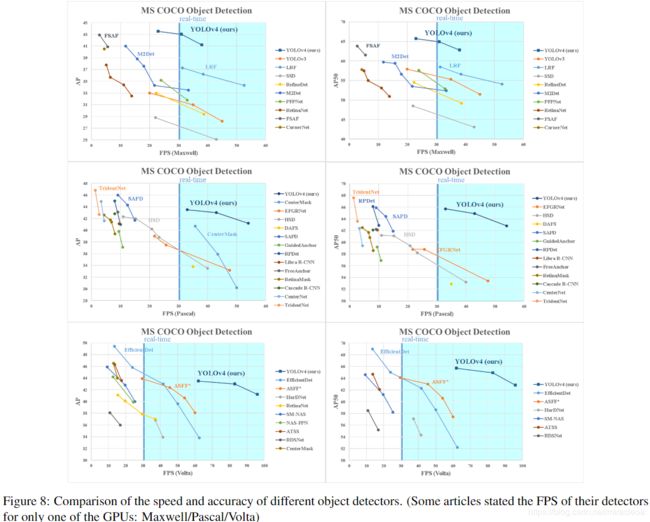
- 由于不同的方法使用不同架构的GPU进行推理时间验证,因此我们在Maxwell,Pascal和Volta architectures常用的GPU上运行YOLOv4,并将它们与其他最新方法进行比较。 表8列出了使用Maxwell GPU的帧速率比较结果,可以是GTX Titan X(Maxwell)或 Tesla M40 GPU。 表9列出了使用Pascal GPU的帧率比较结果,可以是Titan X(Pascal),Titan Xp,GTX 1080 Ti或Tesla P100 GPU。 至于表10,它列出了使用Volta GPU的帧率比较结果,可以是Titan Volta或Tesla V100 GPU。
六、结论
- 我们提供最先进的检测器,其速度(FPS)和准确度( M S C O C O A P 50...95 和 A P 50 MS COCO AP_{50 ... 95}和AP_{50} MSCOCOAP50...95和AP50)比所有可用的替代检测器都高。 所描述的检测器可以在具有8-16GB-VRAM的常规GPU上进行训练和使用,这使得它的广泛使用成为可能。一阶段基于锚的探测器的原始概念已证明其可行性。 我们已经验证了许多功能,并选择使用这些功能以提高分类器和检测器的准确性。 这些功能可以用作将来研究和开发的最佳实践。

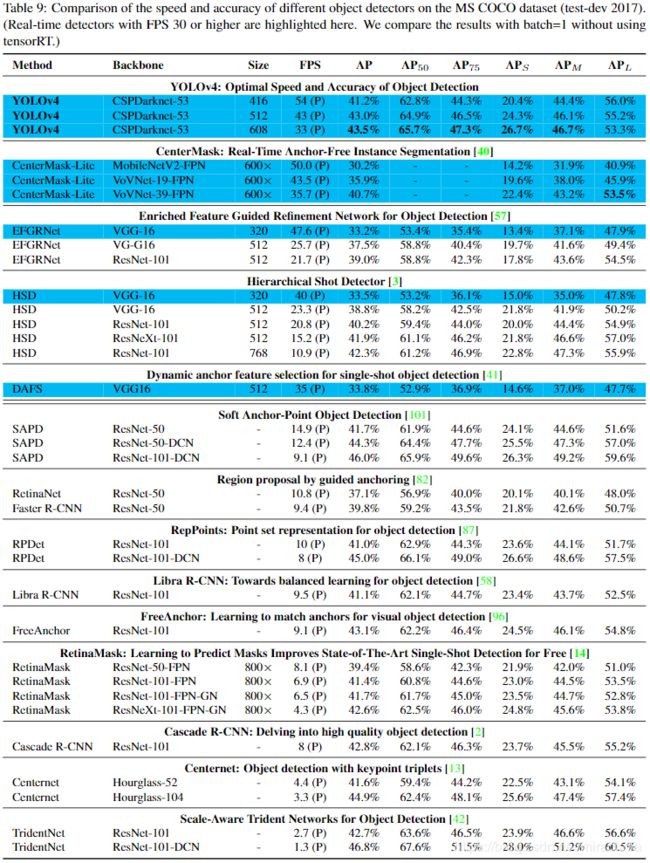
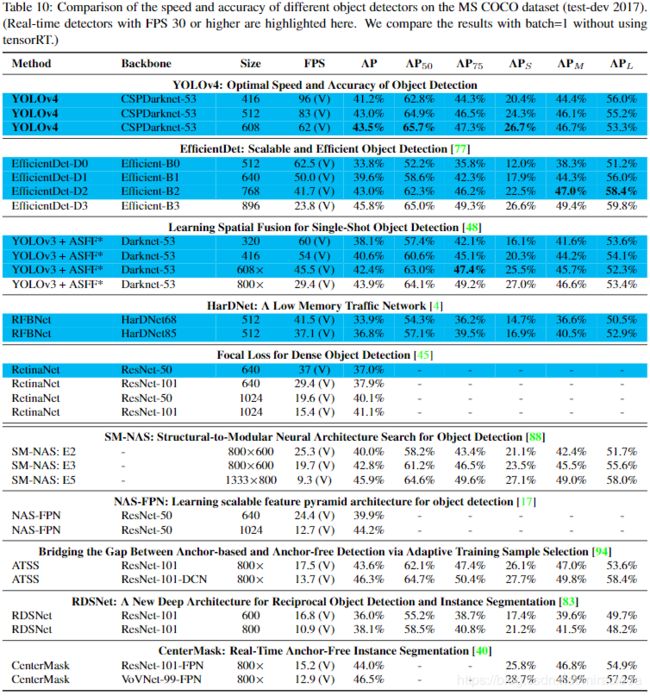
参考
深度学习-目标检测评估指标P-R曲线、AP、mAP