目录
- 1. Domain Adaptation for Image Dehazing (Yuanjie Shao, 2020)
- 2. Cycle-Dehaze: Enhanced CycleGAN for Single Image Dehazing (Deniz Engin, 2018)
- 3. Closed-loop Matters: Dual Regression Networks for Single Image Super-Resolution (Yong Guo, 2020)
- 4. PairedCycleGAN: Asymmetric Style Transfer for Applying and Removing Makeup (Huiwen Chang, 2018)
- 5. 小结
记第一次组会相关论文4篇,主要比较方法上的创新和差异,一些还没弄懂的技术细节先占个坑。
1. Domain Adaptation for Image Dehazing (Yuanjie Shao, 2020)
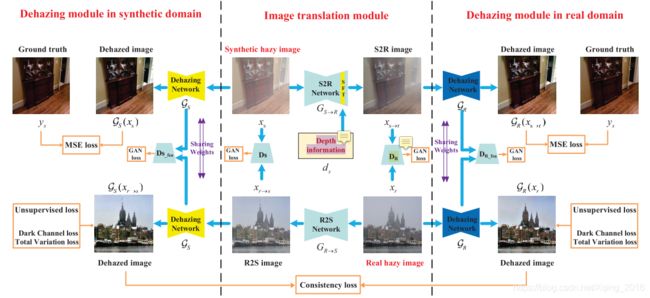
contributions:
- We propose an end-to-end domain adaptation framework for image dehazing, which effectively bridges the gap between the synthetic and real-world hazy images.
- We show that incorporating real hazy images into the training process can improve the dehazing performance.
首先在真实图像和合成雾图之间做了 domain adaptation,以解决 domain shift 的问题。然后在 synthetic domain 和 real domain 中分别训练去雾模型,输入是原始 domain 的图加上 image translation 模块生成的图。
Training Losses 包括 Image translation Losses 和 Image dehazing Losses。
Image translation Losses:image-level 和 feature-level 的常规 adversarial loss,cycle-consistency loss 以及 identity mapping loss(to encourage the generators to preserve content information between the input and output)。
Image dehazing Losses:包括有监督方面的 mean squared loss 和 无监督方面的 total variation and dark channel losses,以及两个域之间的 consistency loss:

2. Cycle-Dehaze: Enhanced CycleGAN for Single Image Dehazing (Deniz Engin, 2018)
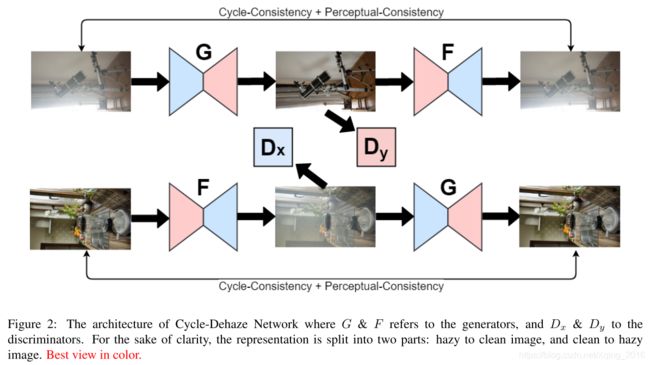
contributions:
- We enhance CycleGAN architecture for single image dehazing via adding cyclic perceptual-consistency loss besides cycle-consistency loss.
- Our method requires neither paired samples of hazy and ground truth images nor any parameters of atmospheric scattering model during the training and testing phases.
- We present a simple and efficient technique to upscale dehazed images by benefiting from Laplacian pyramid.
文中的网络和原始的 Cycle GAN 架构类似,只是加了 cyclic perceptual-consistency loss,以及 Laplacian pyramid(后处理)。提出的 cyclic perceptual-consistency loss 相当于在 high and low-level features 层面上添加约束。Cycle-Dehaze 架构的输入输出都是 256 x 256,利用 Laplacian pyramid 对输出图像进行上采样得到高分辨率的去雾图像。
3. Closed-loop Matters: Dual Regression Networks for Single Image Super-Resolution (Yong Guo, 2020)
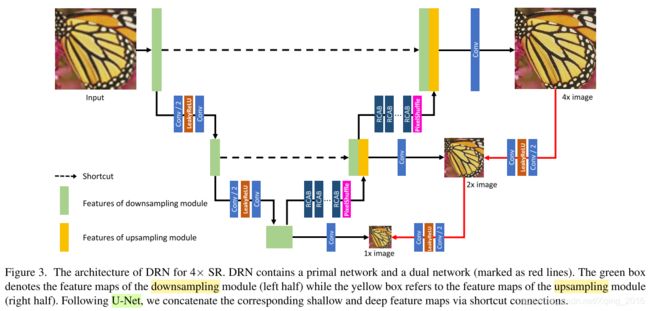
contributions:
- We develop a dual regression scheme by introducing an additional constraint such that the mappings can form a closed-loop and LR images can be reconstructed to enhance the performance of SR models.
- We study a more general super-resolution case where there is no corresponding HR data w.r.t. the real-world LR data.
文章通过添加额外的约束,即将生成的 SR 图像映射回对应的 LR 图像,以减少可能的函数空间。Loss 方面,对于 paired synthetic data,可以计算 primal regression loss 和 dual regression loss;对于 unpaired data,则只计算 dual regression loss。
DRN 与 Cycle GAN based SR methods 的差别:(1) 减少搜索空间;(2) simultaneously exploits both paired synthetic data and real-world unpaired data to enhance the training。
4. PairedCycleGAN: Asymmetric Style Transfer for Applying and Removing Makeup (Huiwen Chang, 2018)
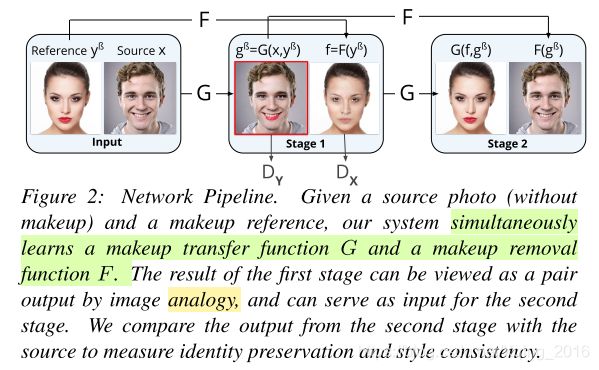
contributions:
- A feed-forward makeup transformation network that can quickly transfer the style from an arbitrary reference makeup photo to an arbitrary source photo.
- An asymmetric makeup transfer framework wherein we train a makeup removal network jointly with the transfer network to preserve the identity, augmented by a style discriminator.
Loss 方面:添加了 G 和 F 的 adversarial loss,输入 x 的 identity loss,以及 style loss:

公式(5)中 W ( x , y β ) W(x, y^{β}) W(x,yβ) 是 synthetic ground-truth。

具体实施的时候,是分别对 eyes, lip 和 skin 这些 facial components 训练 generators 和 discriminators。
5. 小结
第一第二篇都是去雾的工作,第三篇是 SR,第四篇是 Makeup(化妆风格转变)。这几篇的共同点都是使用了类似于 Cycle GAN 的架构,虽然在名字上有所不同,如 closed-loop 和 asymmetric style transfer,但大体上还是 consistency loss 的思路,也就是生成的图像要能够还原回去。另外,这里考虑的大都是 unpaired data 的问题,不过像第一和第三篇也借助了 synthetic paired data 做有监督训练。
从图像生成的角度,如果说第二篇仅仅是在 features 层面上多添加了一致性约束,那么第一篇走得更远,还添加了其他很多约束如 the total variation and dark channel losses(但个人感觉约束项太多,计算消耗是否太大?)
从 domain adaptation 的角度,第一篇和第三篇都没有解决第二篇作者提出的 domain shift 问题,第三篇仅仅是简单地把 synthetic data 和 real-world data 一起用作训练(无相互作用)。
第四篇主要是风格迁移的工作,有点像提了两个 Cycle GAN,如其名 PairedCycleGAN。
最后,第二篇的 Laplacian pyramid 可否用来做 SR 呢?