YOLOv5模块改进,适用于 YOLOv7、YOLOv5、YOLOv4、Scaled_YOLOv4、YOLOv3、YOLOR一系列YOLO算法的模块改进
一系列YOLO算法改进Trick组合!
很多Trick排列组合
助力论文
数据集涨点
创新点改进
博客
以下CBAM注意力机制 的改进
使用YOLOv5算法作为演示,模块可以无缝插入到YOLOv7、YOLOv5、YOLOv4、Scaled_YOLOv4、YOLOv3、YOLOR等一系列YOLO算法中
增加以下xx.yaml文件
# parameters
nc: 10 # number of classes
depth_multiple: 0.33 # model depth multiple
width_multiple: 0.50 # layer channel multiple
# anchors
anchors:
#- [5,6, 7,9, 12,10] # P2/4
- [10,13, 16,30, 33,23] # P3/8
- [30,61, 62,45, 59,119] # P4/16
- [116,90, 156,198, 373,326] # P5/32
# YOLOv5 backbone
backbone:
# [from, number, module, args] # [c=channels,module,kernlsize,strides]
[[-1, 1, Conv, [64, 6, 2, 2]], # 0-P1/2 [c=3,64*0.5=32,3]
[-1, 1, Conv, [128, 3, 2]], # 1-P2/4
[-1, 3, C3, [128]],
[-1, 1, Conv, [256, 3, 2]], # 3-P3/8
[-1, 6, C3, [256]],
[-1, 1, Conv, [512, 3, 2]], # 5-P4/16
[-1, 9, C3, [512]],
[-1, 1, Conv, [1024, 3, 2]], # 7-P5/32
[-1, 3, C3, [1024]],
[-1, 1, CBAM, [1024]], #9
[-1, 1, SPPF, [1024,5]], #10
]
# YOLOv5 head
head:
[[-1, 1, Conv, [512, 1, 1]],
[-1, 1, nn.Upsample, [None, 2, 'nearest']],
[[-1, 6], 1, Concat, [1]], # cat backbone P4
[-1, 3, C3, [512, False]], # 14
[-1, 1, Conv, [256, 1, 1]],
[-1, 1, nn.Upsample, [None, 2, 'nearest']],
[[-1, 4], 1, Concat, [1]], # cat backbone P3
[-1, 3, C3, [256, False]], # 18 (P3/8-small)
[-1, 1, CBAM, [256]], #19
[-1, 1, Conv, [256, 3, 2]],
[[-1, 15], 1, Concat, [1]], # cat head P4
[-1, 3, C3, [512, False]], # 22 (P4/16-medium) [256, 256, 1, False]
[-1, 1, CBAM, [512]],
[-1, 1, Conv, [512, 3, 2]], #[256, 256, 3, 2]
[[-1, 11], 1, Concat, [1]], # cat head P5
[-1, 3, C3, [1024, False]], # 25 (P5/32-large) [512, 512, 1, False]
[-1, 1, CBAM, [1024]],
[[19, 23, 27], 1, Detect, [nc, anchors]], # Detect(P3, P4, P5)
]
./models/common.py文件增加以下模块
class ChannelAttentionModule(nn.Module):
def __init__(self, c1, reduction=16):
super(ChannelAttentionModule, self).__init__()
mid_channel = c1 // reduction
self.avg_pool = nn.AdaptiveAvgPool2d(1)
self.max_pool = nn.AdaptiveMaxPool2d(1)
self.shared_MLP = nn.Sequential(
nn.Linear(in_features=c1, out_features=mid_channel),
nn.LeakyReLU(0.1, inplace=True),
nn.Linear(in_features=mid_channel, out_features=c1)
)
self.act = nn.Sigmoid()
# self.act=nn.SiLU()
def forward(self, x):
avgout = self.shared_MLP(self.avg_pool(x).view(x.size(0), -1)).unsqueeze(2).unsqueeze(3)
maxout = self.shared_MLP(self.max_pool(x).view(x.size(0), -1)).unsqueeze(2).unsqueeze(3)
return self.act(avgout + maxout)
class SpatialAttentionModule(nn.Module):
def __init__(self):
super(SpatialAttentionModule, self).__init__()
self.conv2d = nn.Conv2d(in_channels=2, out_channels=1, kernel_size=7, stride=1, padding=3)
self.act = nn.Sigmoid()
def forward(self, x):
avgout = torch.mean(x, dim=1, keepdim=True)
maxout, _ = torch.max(x, dim=1, keepdim=True)
out = torch.cat([avgout, maxout], dim=1)
out = self.act(self.conv2d(out))
return out
class CBAM(nn.Module):
def __init__(self, c1, c2):
super(CBAM, self).__init__()
self.channel_attention = ChannelAttentionModule(c1)
self.spatial_attention = SpatialAttentionModule()
def forward(self, x):
out = self.channel_attention(x) * x
out = self.spatial_attention(out) * out
return out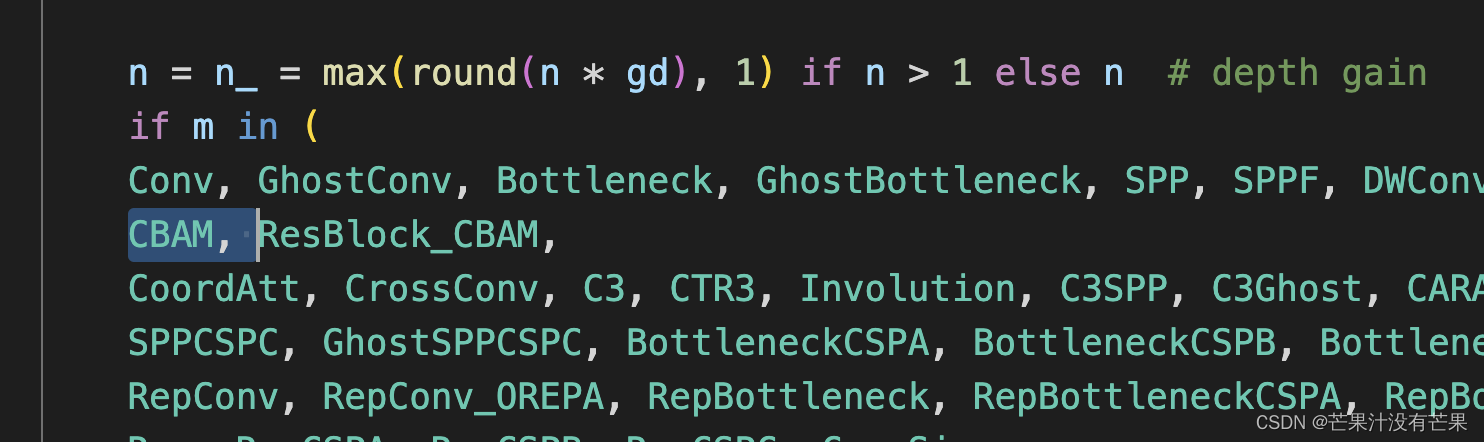
按以下图片展示添加CBAM
CBAM模块
python train.py --cfg xx.yaml
``