期刊名词 | 分区 | ||
---|---|---|---|
Multimedia Tools and Applications | 4 | 2.33 | |
Neural computing and application | 2 | 5.6 | |
Computer methods in applied mechanics and engineering | 2 | 5.763 | |
SIAM journal on scientific computing | 2 | 1.97 | |
Communication in Computational Physics | 3 | 2.6 | |
PLoS One(公共科学图书馆) | 3 | ||
Frontiers in Physics | 3 | 2.638 | |
Frontiers of Information Technology & Electronic Engineering | 3 | 2.161 | |
Nonlinear Dynamics | 1 | 5.022 | |
Applied Thermal Engineering | 2 | 5.295 |
特点
收录文章:
[1] A. R. Brink, D. A. Najera-Flores, and C. Martinez, “The neural network collocation method for solving partial differential equations,” Neural Comput. Appl., vol. 5, 2020, doi: 10.1007/s00521-020-05340-5.
[2] V. I. Avrutskiy, “Neural networks catching up with finite differences in solving partial differential equations in higher dimensions,” Neural Comput. Appl., vol. 32, no. 17, pp. 13425–13440, 2020, doi: 10.1007/s00521-020-04743-8.
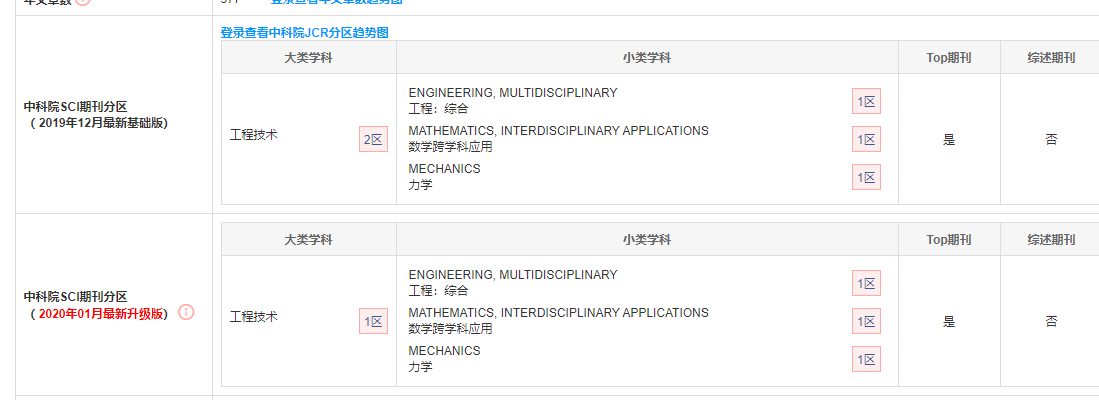
[1] H. Wessels, C. Weißenfels, and P. Wriggers, “The neural particle method – An updated Lagrangian physics informed neural network for computational fluid dynamics,” Comput. Methods Appl. Mech. Eng., vol. 368, p. 113127, 2020, doi: 10.1016/j.cma.2020.113127.
[2] M. Liu, L. Liang, and W. Sun, “A generic physics-informed neural network-based constitutive model for soft biological tissues,” Comput. Methods Appl. Mech. Eng., vol. 372, no. September, p. 113402, 2020, doi: 10.1016/j.cma.2020.113402.
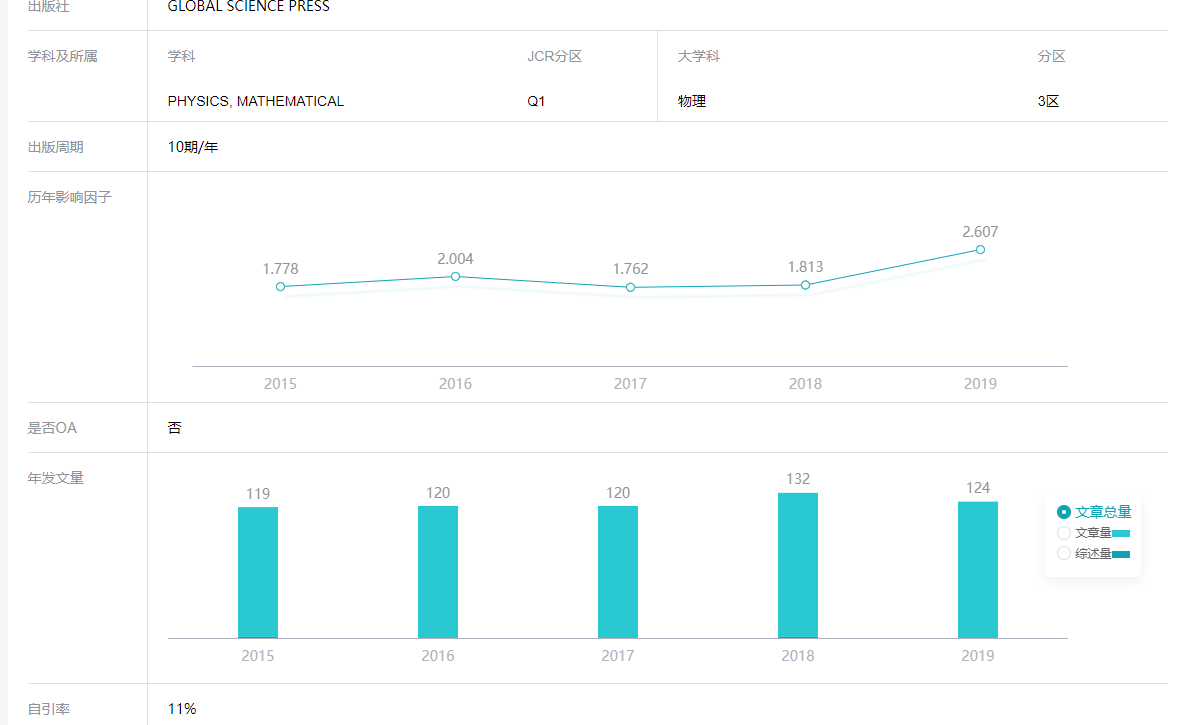
收录文章
[1] A. D. J. & G. E. Karniadakis, “Extended Physics-Informed Neural Networks (XPINNs): A Generalized Space-Time Domain Decomposition Based Deep Learning Framework for Nonlinear Partial Differential Equations,” Commun. Comput. Phys., vol. 28, no. 5, pp. 2002–2041, 2020, doi: 10.4208/cicp.oa-2020-0164.
[2] E. Weinan and B. Yu, “The Deep Ritz Method: A Deep Learning-Based Numerical Algorithm for Solving Variational Problems,” Commun. Math. Stat., vol. 6, no. 1, pp. 1–14, 2018, doi: 10.1007/s40304-018-0127-z.
收录文章
[1] F. Sahli Costabal, Y. Yang, P. Perdikaris, D. E. Hurtado, and E. Kuhl, “Physics-Informed Neural Networks for Cardiac Activation Mapping,” Front. Phys., vol. 8, no. February, pp. 1–12, 2020, doi: 10.3389/fphy.2020.00042.
收录论文
[1] FlowDNN: a physics-informed deep neural network for fast and accurate flow prediction
Solving Huxley equation using an improved PINN method