蓦然回首,那人却在灯火阑珊处。
此文从20220421开始着手,
至20220425为初稿,
从基础理论看起,
以官方文档为根本,
经过自己的一番摸索,
也倒是大致了解了整个过程,
最后却发现最有用的却是已经被官方总结好了
放在这里让后来人少走弯路。
虽然自己摸爬滚打许多天不如一篇官方文章,
但是,
真的没有收获吗?
我觉得未必。
编辑记录
————————————————
20220421 起稿
20220425 21:49 一稿发布
20220426 编辑
20220427 已成功能够开始训练,涉及到手写体数据库格式转换和配置过程后面补齐
20220428 摸索服务器训练
20220502 尝试使用epoch31的训练数据识别(epoch: [31/500], iter: 10105, lr: 0.000993, loss: 7.327022, acc: 0.558589,)
20220503 修补训练数据集的生成与配置使用;测试训练模型的识别能力
202205__ 有使用epoch117数据识别,应该为第10节的测试
202205__ 未完待续…
20220512 搞清train与test数据的相关性——不同作者书写,不相关
PaddleOCR/train_data/
(引用——by程序员阿德)
**什么是lmdb数据集?**
1.英文全名:Lightning Memory-Mapped Database (LMDB);
2.对应中文名:轻量级内存映射数据库。
3.因为最开始 Caffe 就是使用的这个数据库,所以网上的大多数关于 LMDB 的教程都通过 Caffe 实现的
4.LMDB属于key-value数据库,而不是关系型数据库( 比如 MySQL ),LMDB提供 key-value 存储,其中每个键值对都是我们数据集中的一个样本。
LMDB的主要作用是提供数据管理,可以将各种各样的原始数据转换为统一的key-value存储。
5.LMDB的文件结构很简单,一个文件夹,里面是一个数据文件和一个锁文件。
数据随意复制,随意传输。
它的访问简单,不需要单独的数据管理进程。
只要在访问代码里引用LMDB库,访问时给文件路径即可。
env = lmdb.open()
:创建 lmdb 环境txn = env.begin()
:建立事务txn.put(key, value)
:进行插入和修改txn.delete(key)
:进行删除txn.get(key)
:进行查询txn.cursor()
:进行遍历txn.commit()
:提交更改# 安装:pip install lmdb
import lmdb
env = lmdb.open(lmdb_path, map_size=1099511627776)
# lmdb_path:指定存放生成的lmdb数据库的文件夹路径,如果没有该文件夹则自动创建。
# map_size: 指定创建的新数据库所需磁盘空间的最小值,1099511627776B=1T。
# 会在指定路径下创建 data.mdb 和 lock.mdb 两个文件,一是个数据文件,一个是锁文件。
# 创建一个事务(transaction) 对象 txn,所有的操作都必须经过这个事务对象。
# 因为我们要对数据库进行写入操作,所以将 write 参数置为 True,默认其为 False。
txn = env.begin(write=True)
# 使用 .put(key, value) 对数据库进行插入和修改操作,传入的参数为键值对。
# 需要在键值字符串后加 .encode() 改变其编码格式,
# 将 str 转换为 bytes 格式,否则会报该错误:# TypeError: Won't implicitly convert Unicode to bytes; use .encode()。
# 在后面使用 .decode() 对其进行解码得到原数据。
# insert/modify
txn.put(str(1).encode(), "Alice".encode())
txn.put(str(2).encode(), "Bob".encode())
# 使用 .delete(key) 删除指定键值对。
# delete
txn.delete(str(1).encode())
# 对LMDB的读写操作在事务中执行,需要使用 commit 方法提交待处理的事务。
txn.commit()
# 每次 commit() 之后都要用 env.begin() 更新 txn(得到最新的lmdb数据库)。
txn = env.begin()
# 使用 .get(key) 查询数据库中的单条记录。
print(txn.get(str(2).encode()))
# 使用 .cursor() 遍历数据库中的所有记录,
# 其返回一个可迭代对象,相当于关系数据库中的游标,每读取一次,游标下移一位。
for key, value in txn.cursor():
print(key, value)
env.close()
import lmdb
import os, sys
def initialize():
env = lmdb.open("lmdb_dir")
return env
def insert(env, sid, name):
txn = env.begin(write=True)
txn.put(str(sid).encode(), name.encode())
txn.commit()
def delete(env, sid):
txn = env.begin(write=True)
txn.delete(str(sid).encode())
txn.commit()
def update(env, sid, name):
txn = env.begin(write=True)
txn.put(str(sid).encode(), name.encode())
txn.commit()
def search(env, sid):
txn = env.begin()
name = txn.get(str(sid).encode())
return name
def display(env):
txn = env.begin()
cur = txn.cursor()
for key, value in cur:
print(key, value)
env = initialize()
print("Insert 3 records.")
insert(env, 1, "Alice")
insert(env, 2, "Bob")
insert(env, 3, "Peter")
display(env)
print("Delete the record where sid = 1.")
delete(env, 1)
display(env)
print("Update the record where sid = 3.")
update(env, 3, "Mark")
display(env)
print("Get the name of student whose sid = 3.")
name = search(env, 3)
print(name)
# 最后需要关闭关闭lmdb数据库
env.close()
# 执行系统命令
os.system("rm -r lmdb_dir")
在这里插入代码片
在这里插入代码片
做OCR,在搜索中常常碰到一个优质博主:冠军的试炼(【OCR技术系列之八】端到端不定长文本识别CRNN代码实现)
这篇文章对理解CRNN训练的数据输入有帮助
在数据准备部分还有一个操作需要强调的,那就是文字标签数字化,即我们用数字来表示每一个文字(汉字,英文字母,标点符号)。
比如“我”字对应的id是1,
“l”对应的id是1000,
“?”对应的id是90,
如此类推,这种编解码工作使用字典数据结构存储即可,训练时先把标签编码(encode),预测时就将网络输出结果解码(decode)成文字输出。
参考代码:
# 定义str to label 类
class strLabelConverter(object):
"""Convert between str and label.
转换str和label
NOTE:
Insert `blank` to the alphabet for CTC.
Args:
alphabet (str): set of the possible characters.
ignore_case (bool, default=True): whether or not to ignore all of the case.
"""
def __init__(self, alphabet, ignore_case=False):
self._ignore_case = ignore_case
if self._ignore_case:
alphabet = alphabet.lower()
self.alphabet = alphabet + '-' # for `-1` index
self.dict = {}
for i, char in enumerate(alphabet):
# NOTE: 0 is reserved for 'blank' required by wrap_ctc
self.dict[char] = i + 1
def encode(self, text):
"""Support batch or single str.
Args:
text (str or list of str): texts to convert.
Returns:
torch.IntTensor [length_0 + length_1 + ... length_{n - 1}]: encoded texts.
torch.IntTensor [n]: length of each text.
"""
length = []
result = []
for item in text:
item = item.decode('utf-8', 'strict')
length.append(len(item))
for char in item:
index = self.dict[char]
result.append(index)
text = result
# print(text,length)
return (torch.IntTensor(text), torch.IntTensor(length))
def decode(self, t, length, raw=False):
"""Decode encoded texts back into strs.
Args:
torch.IntTensor [length_0 + length_1 + ... length_{n - 1}]: encoded texts.
torch.IntTensor [n]: length of each text.
Raises:
AssertionError: when the texts and its length does not match.
Returns:
text (str or list of str): texts to convert.
"""
if length.numel() == 1:
length = length[0]
assert t.numel() == length, "text with length: {} does not match declared length: {}".format(t.numel(),
length)
if raw:
return ''.join([self.alphabet[i - 1] for i in t])
else:
char_list = []
for i in range(length):
if t[i] != 0 and (not (i > 0 and t[i - 1] == t[i])):
char_list.append(self.alphabet[t[i] - 1])
return ''.join(char_list)
else:
# batch mode
assert t.numel() == length.sum(), "texts with length: {} does not match declared length: {}".format(
t.numel(), length.sum())
texts = []
index = 0
for i in range(length.numel()):
l = length[i]
texts.append(
self.decode(
t[index:index + l], torch.IntTensor([l]), raw=raw))
index += l
return texts
A:可以先试用预训练模型测试一下,例如DB+CRNN,判断下密集文字图片中是检测还是识别的问题,然后针对性的改善。还有一种是如果图象中密集文字较小,可以尝试增大图像分辨率,对图像进行一定范围内的拉伸,将文字稀疏化,提高识别效果。
A:使用基于分割的方法,如DB,检测密集文本行时,最好收集一批数据进行训练,并且在训练时,并将生成二值图像的shrink_ratio参数调小一些。
A:可以在预测时调小 det_db_box_thresh 阈值,默认为0.5, 可调小至0.3观察效果。
A: db后处理中计算文本框平均得分时,是求rectangle区域的平均分数,容易造成弯曲文本漏检,已新增求polygon区域的平均分数,会更准确,但速度有所降低,可按需选择,在相关pr中可查看可视化对比效果。该功能通过参数 det_db_score_mode进行选择,参数值可选[fast(默认)、slow],fast对应原始的rectangle方式,slow对应polygon方式。感谢用户buptlihang提pr帮助解决该问题。
A:在中文识别模型训练时,并不是采用直接将训练样本缩放到[3,32,320]进行训练,而是先等比例缩放图像,保证图像高度为32,宽度不足320的部分补0,宽高比大于10的样本直接丢弃。预测时,如果是单张图像预测,则按上述操作直接对图像缩放,不做宽度320的限制。如果是多张图预测,则采用batch方式预测,每个batch的宽度动态变换,采用这个batch中最长宽度。
A: 由于我们所提供的识别模型是基于通用大规模数据集进行训练的,部分字符可能在训练集中包含较少,因此您可以构建特定场景的数据集,基于我们提供的预训练模型进行微调。建议用于微调的数据集中,每个字符出现的样本数量不低于300,但同时需要注意不同字符的数量均衡。具体可以参考:微调。
A:可以看下训练的尺度和预测的尺度是否相同,如果训练的尺度为[3, 32, 320],预测的尺度为[3, 64, 640],则会有比较多的重复识别现象。
A:可以的。PaddleOCR的检测、识别、方向分类器三个模型是独立的,在实际使用中可以优化和替换其中任何一个模型。
A:如果希望识别中英文识别模型中不支持的字符,需要更新识别的字典,并完成微调过程。比如说如果希望模型能够进一步识别罗马数字,可以按照以下步骤完成模型微调过程。
A:首先请您确认要识别的特殊字符是否在字典中。 如果字符在已经字典中但效果依然不好,可能是由于识别数据较少导致的,您可以增加相应数据finetune模型。
A:单张图像中存在多种类型文本的情况很常见,典型的以学生的试卷为代表,一张图像同时存在手写体和印刷体两种文本,这类情况下,可以尝试”1个检测模型+1个N分类模型+N个识别模型”的解决方案。 其中不同类型文本共用同一个检测模型,N分类模型指额外训练一个分类器,将检测到的文本进行分类,如手写+印刷的情况就是二分类,N种语言就是N分类,在识别的部分,针对每个类型的文本单独训练一个识别模型,如手写+印刷的场景,就需要训练一个手写体识别模型,一个印刷体识别模型,如果一个文本框的分类结果是手写体,那么就传给手写体识别模型进行识别,其他情况同理。
A:无论是文字检测,还是文字识别,骨干网络的选择是预测效果和预测效率的权衡。一般,选择更大规模的骨干网络,例如ResNet101_vd,则检测或识别更准确,但预测耗时相应也会增加。而选择更小规模的骨干网络,例如MobileNetV3_small_x0_35,则预测更快,但检测或识别的准确率会大打折扣。幸运的是不同骨干网络的检测或识别效果与在ImageNet数据集图像1000分类任务效果正相关。飞桨图像分类套件PaddleClas汇总了ResNet_vd、Res2Net、HRNet、MobileNetV3、GhostNet等23种系列的分类网络结构,在上述图像分类任务的top1识别准确率,GPU(V100和T4)和CPU(骁龙855)的预测耗时以及相应的117个预训练模型下载地址。
(1)文字检测骨干网络的替换,主要是确定类似与ResNet的4个stages,以方便集成后续的类似FPN的检测头。此外,对于文字检测问题,使用ImageNet训练的分类预训练模型,可以加速收敛和效果提升。
(2)文字识别的骨干网络的替换,需要注意网络宽高stride的下降位置。由于文本识别一般宽高比例很大,因此高度下降频率少一些,宽度下降频率多一些。可以参考PaddleOCR中MobileNetV3骨干网络的改动。
A: 基于官方提供的模型,进行finetune的话,收敛会更快一些。 具体操作上,以识别模型训练为例:如果修改了字符文件,可以设置pretraind_model为官方提供的预训练模型
A: 这里有两个不同的概念:
pretrained_model:指预训练模型,是已经训练完成的模型。这时会load预训练模型的参数,但并不会load学习率、优化器以及训练状态等。
如果需要finetune,应该使用pretrained。
checkpoints:指之前训练的中间结果,例如前一次训练到了100个epoch,想接着训练。这时会load尝试所有信息,包括模型的参数,之前的状态等。
A:如果是冻结某些层,可以将变量的stop_gradient属性设置为True,这样计算这个变量之前的所有参数都不会更新了,参考:
如果对某些层使用更小的学习率学习,静态图里还不是很方便,一个方法是在参数初始化的时候,给权重的属性设置固定的学习率,参考:
实际上我们实验发现,直接加载模型去fine-tune,不设置某些层不同学习率,效果也都不错
A: stride为(2, 1),表示在图像y方向(高度方向)上stride为2,x方向(宽度方向)上为1。由于待识别的文本图像通常为长方形,这样只在高度方向做下采样,尽量保留宽度方向的序列信息,避免宽度方向下采样后丢失过多的文字信息。
A:一般高度采用32,最长宽度的选择,有两种方法:
(1)统计训练样本图像的宽高比分布。最大宽高比的选取考虑满足80%的训练样本。
(2)统计训练样本文字数目。最长字符数目的选取考虑满足80%的训练样本。然后中文字符长宽比近似认为是1,英文认为3:1,预估一个最长宽度。
A:可以在命令中加入 --det_db_unclip_ratio ,参数定义位置,这个参数是检测后处理时控制文本框大小的,默认1.6,可以尝试改成2.5或者更大,反之,如果觉得文本框不够紧凑,也可以把该参数调小。
A:Backbone的识别效果在CRNN模型上的效果,与Imagenet 1000 图像分类任务上识别效果和效率一致。在图像分类任务上ResnNet_vd(79%+)的识别精度明显优于DenseNet(77%+),此外对于GPU,Nvidia针对ResNet系列模型做了优化,预测效率更高,所以相对而言,resnet_vd是较好选择。如果是移动端,可以优先考虑MobileNetV3系列。
A:在不同的硬件上,不同的backbone的速度优势不同,可以根据不同平台的速度-精度图来确定backbone,这里可以参考PaddleClas模型速度-精度图。
A:实验发现,使用贪心的方法去做解码,识别精度影响不大,但是速度方面的优势比较明显,因此PaddleOCR中使用贪心算法去做识别的解码。
A: 具体问题具体分析: 如果在你的场景上检测效果不可用,首选是在你的数据上做finetune训练; 如果图像过大,文字过于密集,建议不要过度压缩图像,可以尝试修改检测预处理的resize逻辑,防止图像被过度压缩; 检测框大小过于紧贴文字或检测框过大,可以调整db_unclip_ratio这个参数,加大参数可以扩大检测框,减小参数可以减小检测框大小; 检测框存在很多漏检问题,可以减小DB检测后处理的阈值参数det_db_box_thresh,防止一些检测框被过滤掉,也可以尝试设置det_db_score_mode为’slow’; 其他方法可以选择use_dilation为True,对检测输出的feature map做膨胀处理,一般情况下,会有效果改善;
A:可以把后处理的参数unclip_ratio适当调大一点。
A:1. 检查两个模型使用的后处理参数是否是一样的,训练的后处理参数在配置文件中的PostProcess部分,测试模型的后处理参数在tools/infer/utility.py中,最新代码中两个后处理参数已保持一致。
A:tools/train.py中有一个test_reader()函数用于调试数据读取。
A:检测需要的数据相对较少,在PaddleOCR模型的基础上进行Fine-tune,一般需要500张可达到不错的效果。 识别分英文和中文,一般英文场景需要几十万数据可达到不错的效果,中文则需要几百万甚至更多。
A: 在动态图中,ratio_list在有多个数据源的情况下使用,ratio_list中的每个值是每个epoch从对应数据源采样数据的比例。如ratio_list=[0.3,0.2],label_file_list=[‘data1’,‘data2’],代表每个epoch的训练数据包含data1 30%的数据,和data2里 20%的数据,ratio_list中数值的和不需要等于1。ratio_list和label_file_list的长度必须一致。
静态图检测数据采样的逻辑与动态图不同,但基本不影响训练精度。
在静态图中,使用 检测 dataloader读取数据时,会先设置每个epoch的数据量,比如这里设置为1000,ratio_list中的值表示在1000中的占比,比如ratio_list是[0.3, 0.7],则表示使用两个数据源,每个epoch从第一个数据源采样1000*0.3=300张图,从第二个数据源采样700张图。ratio_list的值的和也不需要等于1。
A:OCR模型训练过程中一般包含大量的数据增广,这些数据增广是比较耗时的,因此可以离线生成大量增广后的图像,直接送入网络进行训练,机器资源充足的情况下,也可以使用分布式训练的方法,可以参考分布式训练教程文档。
A:您可以合成一些接近使用场景的数据用于训练。 我们计划推出基于特定场景的文本数据合成工具,请您持续关注PaddleOCR的近期更新。
A: 手写数据集可以通过手写单字数据集合成得到。随机选取一定数量的单字图片和对应的label,将图片高度resize为随机的统一高度后拼接在一起,即可得到合成数据集。对于需要添加文字背景的情况,建议使用阈值化将单字图片的白色背景处理为透明背景,再与真实背景图进行合成。具体可以参考文档手写数据集。
A:因为默认保存的起始点不是0,而是4000,将eval_batch_step [4000, 5000]改为[0, 2000] 就是从第0次迭代开始,每2000迭代保存一次模型
A:cosine_decay表示在训练的过程中,学习率按照cosine的变化趋势逐渐下降至0,在迭代轮数更长的情况下,比常量的学习率变化策略会有更好的收敛效果,因此在实际训练的时候,均采用了cosine_decay,来获得精度更高的模型。
A: Cosine学习率的说明可以参考这里
在PaddleOCR中,为了让学习率更加平缓,我们将其中的epoch调整成了iter。 学习率的更新会和总的iter数量有关。当iter比较大时,会经过较多iter才能看出学习率的值有变化。
A: 我们对代码结构进行了调整,目前的Cosine可以覆盖原有的CosineWarmup的功能,只需要在配置文件中增加相应配置即可。 例如下面的代码,可以设置warmup为2个epoch:
lr:
name: Cosine
learning_rate: 0.001
warmup_epoch: 2
A: 考虑内存,显存(使用GPU训练的话)是否不足,可在配置文件中,将训练和评估的batch size调小一些。需要注意,训练batch size调小时,学习率learning rate也要调小,一般可按等比例调整。
A: 可以从以下三方面考虑:
1. 检查训练进程是否正常退出、显存占用是否释放、是否有残留进程,如果确定是训练程序卡死,可以检查环境配置,遇到环境问题建议使用docker,可以参考说明文档[安装](https://github.com/PaddlePaddle/PaddleOCR/blob/release/2.1/doc/doc_ch/installation.md)。
2. 检查数据集的数据量是否太小,可调小batch size从而增加一个epoch中的训练step数量,或在训练config文件中,将参数print_batch_step改为1,即每一个step打印一次log信息。
3. 如果使用私有数据集训练,可先用PaddleOCR提供/推荐的数据集进行训练,排查私有数据集是否存在问题。
A: 训练数据的读取需要硬盘IO,而硬盘IO速度远小于GPU运算速度,为了避免数据读取成为训练速度瓶颈,可以使用多进程读取数据,
num workers表示数据读取的进程数量,
0表示不使用多进程读取。
在Linux系统下,多进程读取数据时,进程间通信需要基于共享内存,因此使用多进程读取数据时,建议设置共享内存不低于2GB,最好可以达到8GB,此时,num workers可以设置为CPU核心数。
如果机器硬件配置较低,或训练进程卡死、dataloader报错,可以将num workers设置为0,即不使用多进程读取数据。
Global:
use_gpu: True
epoch_num: 2
log_smooth_window: 20
# 每( 1000 )iter打印一次当前 iter: lr: loss: acc: norm_edit_dis: reader_cost: batch_cost: samples: ips:
print_batch_step: 1000
save_model_dir: ./output/rec_chinese_common_v2.0
# 每()epoc保存一次模型参数
save_epoch_step: 1
# evaluation is run every 5000 iterations after the 4000th iteration
# 每()iter保存一次模型参数
eval_batch_step: [0, 100]
cal_metric_during_train: True
pretrained_model: F:/OCR/PaddleOCR-release-2.4/inference/server model/pre-train model/rec/ch_ppocr_server_v2.0_rec_pre/ch_ppocr_server_v2.0_rec_pre/best_accuracy
checkpoints:
save_inference_dir: F:/OCR/PaddleOCR-release-2.4/inference/self_train
use_visualdl: True
infer_img: doc/imgs_words/hand_write/1241-P16_0.jpg
# for data or label process
character_dict_path: ppocr/utils/ppocr_keys_v1.txt
max_text_length: 35
infer_mode: False
use_space_char: True
save_res_path: ./output/rec/predicts_chinese_common_v2.0.txt
Optimizer:
name: Adam
beta1: 0.9
beta2: 0.999
lr:
name: Cosine
learning_rate: 0.001
warmup_epoch: 1
regularizer:
name: 'L2'
factor: 0.00004
Architecture:
model_type: rec
algorithm: CRNN
Transform:
Backbone:
name: ResNet
layers: 34
Neck:
name: SequenceEncoder
encoder_type: rnn
hidden_size: 256
Head:
name: CTCHead
fc_decay: 0.00004
Loss:
name: CTCLoss
PostProcess:
name: CTCLabelDecode
Metric:
name: RecMetric
main_indicator: acc
Train:
dataset:
name: SimpleDataSet
data_dir: ./train_data/
label_file_list: ["./train_data/rec_gt_train.txt"]
transforms:
- DecodeImage: # load image
img_mode: BGR
channel_first: False
- RecAug:
- CTCLabelEncode: # Class handling label
- RecResizeImg:
image_shape: [3, 32, 320]
- KeepKeys:
keep_keys: ['image', 'label', 'length'] # dataloader will return list in this order
loader:
shuffle: True
batch_size_per_card: 8
drop_last: True
num_workers: 4
Eval:
dataset:
name: SimpleDataSet
data_dir: ./train_data/
label_file_list: ["./train_data/rec_gt_test.txt"]
transforms:
- DecodeImage: # load image
img_mode: BGR
channel_first: False
- CTCLabelEncode: # Class handling label
- RecResizeImg:
image_shape: [3, 32, 320]
- KeepKeys:
keep_keys: ['image', 'label', 'length'] # dataloader will return list in this order
loader:
shuffle: False
drop_last: False
batch_size_per_card: 8
num_workers: 4
什么是CRNN?
CRNN是由CNN-RNN-CTC三大部分架构而成
分别对应 卷积层、循环层和转录层
(1)CNN提取图像卷积特征
(2)深层双向LSTM网络,在卷积特征的基础上继续提取文字序列特征,用于学习关联序列信息并预测标签分布
(3)CTC,解决训练时字符无法对齐的问题用于序列对齐,输出预测结果。
什么是CNN——卷积神经网络(by深度学习花书)?
什么是RNN?——循环神经网络
是一种用来处理序列数据的神经网络
循环网络可以扩展到更长的序列,大多数循环网络也能处理可变长度序列
虽然卷积操作允许网络跨时间共享参数,但只是浅层的
RNN以不同的形式共享参数:输出的每一项是前一项的函数
RNN的设计模式
什么是CTC(Connectionist temporal classification)?
参考网页:超详细讲解CTC理论和实战
文本行是如何被检测的?
只调用识别可以识别多行文本吗?
特征提取部分使用主流卷积结构,常用的有
因为文本识别任务的特殊性,数据中存在大量上下文信息,卷积神经网络很难挖掘到文本之间的上下文联系。为解决这一问题,CRNN引入双向LSTM来增强上下文建模
最终将输出的特征序列输入到CTC中,直接解码序列结果。
详解:
head:
数据送入网络前需要缩放到统一尺寸(3,32,320),并完成归一化处理。
import cv2
import math
import numpy as np
def resize_norm_img(img):
"""
数据缩放和归一化
:param img: 输入图片
"""
# 默认输入尺寸
imgC = 3
imgH = 32
imgW = 320
# 图片的真实高宽
h, w = img.shape[:2]
# 图片真实长宽比
ratio = w / float(h)
# 按比例缩放
# math.ceil( )函数————向上取整,四舍五入
# math.floor()函数————向下取整
if math.ceil(imgH * ratio) > imgW:
# 如大于默认宽度,则宽度为imgW
resized_w = imgW
else:
# 如小于默认宽度则以图片真实宽为准
resized_w = int(math.ceil(imgH * ratio))
# 缩放
resized_image = cv2.resize(img, (resized_w, imgH))
# astype() 对数据类型进行转换
resized_image = resized_image.astype('float32')
# 归一化
# 此处导致颜色变化是因为/255再减均值除方差
resized_image = resized_image.transpose((2, 0, 1)) / 255
resized_image -= 0.5
resized_image /= 0.5
# 对宽度不足的位置,补0
padding_im = np.zeros((imgC, imgH, imgW), dtype=np.float32)
padding_im[:, :, 0:resized_w] = resized_image
# 转置 padding 后的图片用于可视化
draw_img = padding_im.transpose((1,2,0))
return padding_im, draw_img
import matplotlib.pyplot as plt
# 读图
raw_img = cv2.imread("/home/aistudio/work/word_1.png")
plt.figure()
plt.subplot(2,1,1)
# 可视化原图
plt.imshow(raw_img)
# 缩放并归一化
padding_im, draw_img = resize_norm_img(raw_img)
plt.subplot(2,1,2)
# 可视化网络输入图
plt.imshow(draw_img)
plt.show()
import paddle
import paddle.nn as nn
import paddle.nn.functional as F
class ConvBNLayer(nn.Layer):
def __init__(self,
in_channels,
out_channels,
kernel_size,
stride,
padding,
groups=1,
if_act=True,
act=None):
"""
卷积BN层
:param in_channels: 输入通道数
:param out_channels: 输出通道数
:param kernel_size: 卷积核尺寸
:parma stride: 步长大小
:param padding: 填充大小
:param groups: 二维卷积层的组数
:param if_act: 是否添加激活函数
:param act: 激活函数
"""
super(ConvBNLayer, self).__init__()
self.if_act = if_act
self.act = act
# nn.Conv2d()详解 [网址](https://blog.csdn.net/qq_42079689/article/details/102642610)
#
self.conv = nn.Conv2D(
in_channels=in_channels,
out_channels=out_channels,
kernel_size=kernel_size,
stride=stride,
padding=padding,
groups=groups,
bias_attr=False)
# 批规范化,为了解决每一个batch输入的数据分布变化问题。
self.bn = nn.BatchNorm(num_channels=out_channels, act=None)
def forward(self, x):
# conv层
x = self.conv(x)
# batchnorm层
x = self.bn(x)
# 是否使用激活函数
if self.if_act:
if self.act == "relu":
x = F.relu(x)
elif self.act == "hardswish":
x = F.hardswish(x)
else:
print("The activation function({}) is selected incorrectly.".
format(self.act))
exit()
return x
# mobilenetv3中调用
class SEModule(nn.Layer):
def __init__(self, in_channels, reduction=4):
"""
SE模块
:param in_channels: 输入通道数
:param reduction: 通道缩放率
"""
super(SEModule, self).__init__()
self.avg_pool = nn.AdaptiveAvgPool2D(1)
self.conv1 = nn.Conv2D(
in_channels=in_channels,
out_channels=in_channels // reduction,
kernel_size=1,
stride=1,
padding=0)
self.conv2 = nn.Conv2D(
in_channels=in_channels // reduction,
out_channels=in_channels,
kernel_size=1,
stride=1,
padding=0)
def forward(self, inputs):
# 平均池化
outputs = self.avg_pool(inputs)
# 第一个卷积层
outputs = self.conv1(outputs)
# relu激活函数
outputs = F.relu(outputs)
# 第二个卷积层
outputs = self.conv2(outputs)
# hardsigmoid 激活函数
outputs = F.hardsigmoid(outputs, slope=0.2, offset=0.5)
return inputs * outputs
class ResidualUnit(nn.Layer):
def __init__(self,
in_channels,
mid_channels,
out_channels,
kernel_size,
stride,
use_se,
act=None):
"""
残差层
:param in_channels: 输入通道数
:param mid_channels: 中间通道数
:param out_channels: 输出通道数
:param kernel_size: 卷积核尺寸
:parma stride: 步长大小
:param use_se: 是否使用se模块
:param act: 激活函数
"""
super(ResidualUnit, self).__init__()
self.if_shortcut = stride == 1 and in_channels == out_channels
self.if_se = use_se
self.expand_conv = ConvBNLayer(
in_channels=in_channels,
out_channels=mid_channels,
kernel_size=1,
stride=1,
padding=0,
if_act=True,
act=act)
self.bottleneck_conv = ConvBNLayer(
in_channels=mid_channels,
out_channels=mid_channels,
kernel_size=kernel_size,
stride=stride,
padding=int((kernel_size - 1) // 2),
groups=mid_channels,
if_act=True,
act=act)
if self.if_se:
self.mid_se = SEModule(mid_channels)
self.linear_conv = ConvBNLayer(
in_channels=mid_channels,
out_channels=out_channels,
kernel_size=1,
stride=1,
padding=0,
if_act=False,
act=None)
def forward(self, inputs):
x = self.expand_conv(inputs)
x = self.bottleneck_conv(x)
if self.if_se:
x = self.mid_se(x)
x = self.linear_conv(x)
if self.if_shortcut:
x = paddle.add(inputs, x)
return x
def make_divisible(v, divisor=8, min_value=None):
"""
确保被8整除
"""
if min_value is None:
min_value = divisor
new_v = max(min_value, int(v + divisor / 2) // divisor * divisor)
if new_v < 0.9 * v:
new_v += divisor
return new_v
利用公共模块搭建骨干网络
class MobileNetV3(nn.Layer):
def __init__(self,
in_channels=3,
model_name='small',
# 代表网络的宽度,1是标准宽度,最大
scale=0.5,
small_stride=None,
disable_se=False,
**kwargs):
super(MobileNetV3, self).__init__()
self.disable_se = disable_se
small_stride = [1, 2, 2, 2]
if model_name == "small":
cfg = [
# k, exp, c, se, nl, s,
[3, 16, 16, True, 'relu', (small_stride[0], 1)],
[3, 72, 24, False, 'relu', (small_stride[1], 1)],
[3, 88, 24, False, 'relu', 1],
[5, 96, 40, True, 'hardswish', (small_stride[2], 1)],
[5, 240, 40, True, 'hardswish', 1],
[5, 240, 40, True, 'hardswish', 1],
[5, 120, 48, True, 'hardswish', 1],
[5, 144, 48, True, 'hardswish', 1],
[5, 288, 96, True, 'hardswish', (small_stride[3], 1)],
[5, 576, 96, True, 'hardswish', 1],
[5, 576, 96, True, 'hardswish', 1],
]
cls_ch_squeeze = 576
else:
raise NotImplementedError("mode[" + model_name +
"_model] is not implemented!")
supported_scale = [0.35, 0.5, 0.75, 1.0, 1.25]
assert scale in supported_scale, \
"supported scales are {} but input scale is {}".format(supported_scale, scale)
inplanes = 16
# conv1
self.conv1 = ConvBNLayer(
in_channels=in_channels,
out_channels=make_divisible(inplanes * scale),
kernel_size=3,
stride=2,
padding=1,
groups=1,
if_act=True,
act='hardswish')
i = 0
block_list = []
inplanes = make_divisible(inplanes * scale)
for (k, exp, c, se, nl, s) in cfg:
se = se and not self.disable_se
block_list.append(
ResidualUnit(
in_channels=inplanes,
mid_channels=make_divisible(scale * exp),
out_channels=make_divisible(scale * c),
kernel_size=k,
stride=s,
use_se=se,
act=nl))
inplanes = make_divisible(scale * c)
i += 1
self.blocks = nn.Sequential(*block_list)
self.conv2 = ConvBNLayer(
in_channels=inplanes,
out_channels=make_divisible(scale * cls_ch_squeeze),
kernel_size=1,
stride=1,
padding=0,
groups=1,
if_act=True,
act='hardswish')
self.pool = nn.MaxPool2D(kernel_size=2, stride=2, padding=0)
self.out_channels = make_divisible(scale * cls_ch_squeeze)
def forward(self, x):
x = self.conv1(x)
x = self.blocks(x)
x = self.conv2(x)
x = self.pool(x)
return x
# 图片输入骨干网络
backbone = MobileNetV3()
# 将numpy数据转换为Tensor
input_data = paddle.to_tensor([padding_im])
# 骨干网络输出
feature = backbone(input_data)
# 查看feature map的纬度
print("backbone output:", feature.shape)
- neck
neck 部分将backbone输出的视觉特征图转换为1维向量输入送到 LSTM 网络中,输出序列特征( 源码位置 )
class Im2Seq(nn.Layer):
def __init__(self, in_channels, **kwargs):
"""
图像特征转换为序列特征
:param in_channels: 输入通道数
"""
super().__init__()
self.out_channels = in_channels
def forward(self, x):
# 4维压缩到3维
B, C, H, W = x.shape
assert H == 1
x = x.squeeze(axis=2)
x = x.transpose([0, 2, 1]) # (NWC)(batch, width, channels)
return x
class EncoderWithRNN(nn.Layer):
def __init__(self, in_channels, hidden_size):
super(EncoderWithRNN, self).__init__()
self.out_channels = hidden_size * 2
self.lstm = nn.LSTM(
in_channels, hidden_size, direction='bidirectional', num_layers=2)
def forward(self, x):
x, _ = self.lstm(x)
return x
class SequenceEncoder(nn.Layer):
def __init__(self, in_channels, hidden_size=48, **kwargs):
"""
序列编码
:param in_channels: 输入通道数
:param hidden_size: 隐藏层size
"""
super(SequenceEncoder, self).__init__()
self.encoder_reshape = Im2Seq(in_channels)
self.encoder = EncoderWithRNN(self.encoder_reshape.out_channels, hidden_size)
self.out_channels = self.encoder.out_channels
def forward(self, x):
x = self.encoder_reshape(x)
x = self.encoder(x)
return x
neck = SequenceEncoder(in_channels=288)
sequence = neck(feature)
print("sequence shape:", sequence.shape)
- head
预测头部分由全连接层和softmax组成,用于计算序列特征时间步上的标签概率分布,本示例仅支持模型识别小写英文字母和数字(26+10)36个类别(源码位置)
class CTCHead(nn.Layer):
def __init__(self,
in_channels,
out_channels,
**kwargs):
"""
CTC 预测层
:param in_channels: 输入通道数
:param out_channels: 输出通道数
"""
super(CTCHead, self).__init__()
self.fc = nn.Linear(
in_channels,
out_channels)
# 思考:out_channels 应该等于多少?
self.out_channels = out_channels
def forward(self, x):
#
predicts = self.fc(x)
result = predicts
if not self.training:
predicts = F.softmax(predicts, axis=2)
result = predicts
return result
在网络随机初始化的情况下,输出结果是无序的,
经过SoftMax之后,可以得到各时间步上的概率最大的预测结果,
其中:
pred_id 代表预测的标签ID,
pre_scores 代表预测结果的置信度
ctc_head = CTCHead(in_channels=96, out_channels=37)
predict = ctc_head(sequence)
print("predict shape:", predict.shape)
result = F.softmax(predict, axis=2)
pred_id = paddle.argmax(result, axis=2)
pred_socres = paddle.max(result, axis=2)
print("pred_id:", pred_id)
print("pred_scores:", pred_socres)
- 后处理-postprocess
识别网络最终返回的结果是各个时间步上的最大索引值,最终期望的输出是对应的文字结果,因此CRNN的后处理是一个解码过程,主要逻辑如下:
def decode(text_index, text_prob=None, is_remove_duplicate=False):
""" convert text-index into text-label. """
character = "-0123456789abcdefghijklmnopqrstuvwxyz"
result_list = []
# 忽略tokens [0] 代表ctc中的blank位
ignored_tokens = [0]
batch_size = len(text_index)
for batch_idx in range(batch_size):
char_list = []
conf_list = []
for idx in range(len(text_index[batch_idx])):
if text_index[batch_idx][idx] in ignored_tokens:
continue
# 合并blank之间相同的字符
if is_remove_duplicate:
# only for predict
if idx > 0 and text_index[batch_idx][idx - 1] == text_index[
batch_idx][idx]:
continue
# 将解码结果存在char_list内
char_list.append(character[int(text_index[batch_idx][
idx])])
# 记录置信度
if text_prob is not None:
conf_list.append(text_prob[batch_idx][idx])
else:
conf_list.append(1)
text = ''.join(char_list)
# 输出结果
result_list.append((text, np.mean(conf_list)))
return result_list
以 head 部分随机初始化预测出的结果为例,进行解码得到:
pred_id = paddle.argmax(result, axis=2)
pred_socres = paddle.max(result, axis=2)
print(pred_id)
decode_out = decode(pred_id, pred_socres)
print("decode out:", decode_out)
# codecs用法
codecs.open(filepath,method,encoding)
filepath--文件路径
method--打开方式,r为读,w为写,rw为读写
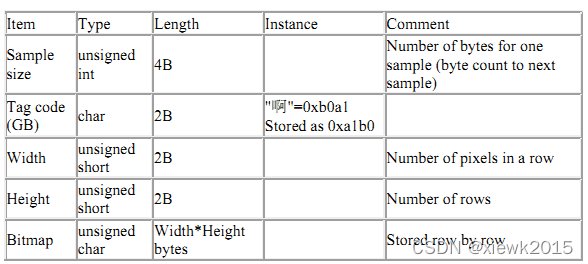
encoding--文件的编码,中文文件使用utf-8
# Python使用struct处理二进制(pack和unpack用法)
# 按照给定的格式(fmt),把数据封装成字符串(实际上是类似于c结构体的字节流)
struct.pack(fmt, v1, v2, ...)
# 按照给定的格式(fmt)解析字节流string,返回解析出来的tuple
struct.unpack(fmt, string)
# 计算给定的格式(fmt)占用多少字节的内存
calcsize(fmt)
# .read用法
# read() 函数:逐个字节或者字符读取文件中的内容
# readline() 函数:逐行读取文件中的内容;
# readlines() 函数:一次性读取文件中多行内容。
# 如果文件是以文本模式(非二进制模式)打开的,
# 则 read() 函数会逐个字符进行读取;
# 如果文件以二进制模式打开,
# 则 read() 函数会逐个字节进行读取。
file.read([size])
# file 表示已打开的文件对象;
# size 作为一个可选参数,用于指定一次最多可读取的字符(字节)个数,如果省略,则默认一次性读取所有内容。
# 注意,当操作文件结束后,必须调用 close() 函数手动将打开的文件进行关闭,这样可以避免程序发生不必要的错误。
def decode_GNT_to_imgs(gnt):
'''
:param gnt: a writer's enco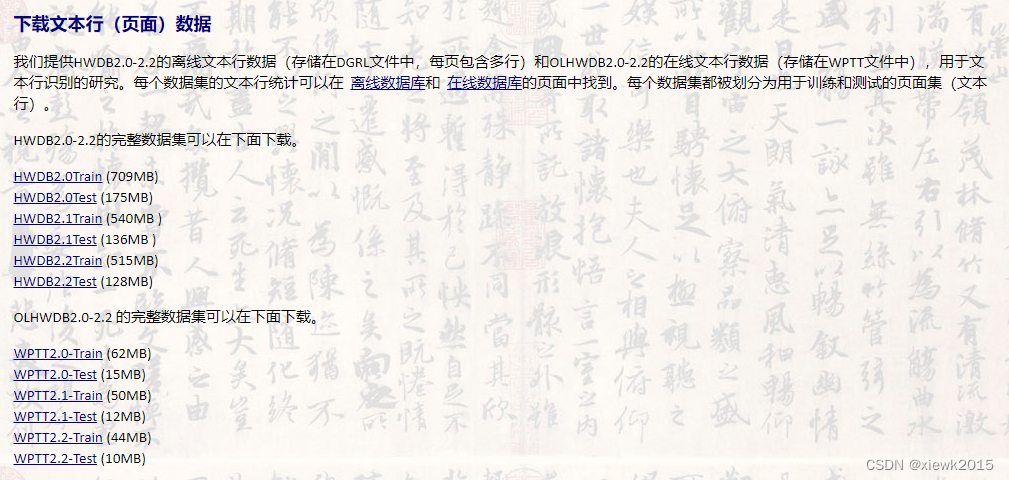
ded ground truth file.(gnt文件路径)
:return: samples list, each sample with format (charname, img)
'''
samples = []
with codecs.open(gnt, mode='rb') as fin:
while (True):
# 读取4B的长度到缓存中,4B是从文件格式中得到的
left_cache = fin.read(4)
# 如果读到的<4B说明读取错误,跳出
if len(left_cache) < 4:
break
# left_cache:
# Sample size:4B unsigned int;
# Tag code :2B char;
# Width :2B unsigned short;
# Hight :2B unsigned short;
# Bitmap :矩阵 前两个相乘的长度bytes unsigned char
sample_size = struct.unpack("I", left_cache)[0]# I: unsigned int struct.unpack返回的是tuple,那么[0]则是指tuple里的第一个元素
print(sample_size)
tag_code = str(fin.read(2), 'gbk')
print(tag_code[0])
width = struct.unpack("H", fin.read(2))[0] # H:unsigned short
print(width)
height = struct.unpack("H", fin.read(2))[0] # H:unsigned short
print(height)
# 创建img数组,将数据写入
img = np.zeros(shape=[height, width], dtype=np.uint8)
for r in range(height):
for c in range(width):
img[r, c] = struct.unpack("B", fin.read(1))[0]
# 校验,10是前面字节的总长度,sample_size为存储的一个字的所有总长度
if width * height + 10 != sample_size:
break
samples.append((tag_code[0], img))
# print(samples)
return samples
VOC标签格式说明:链接
此处我们不使用这种格式,下一节会对其进行转换
def decode_DGR_to_imgs_and_vocxml(dgr):
'''
:param dgr: a writer's encoded ground truth file.
:return: samples list, each sample with format (charname, img)
'''
doc_img, voc_xml = None, None
with codecs.open(dgr, mode='rb') as fin:
while(True):
left_cache = fin.read(4)
if len(left_cache) < 4:
break
#FILE HEAFER
size_of_header = struct.unpack("I", left_cache)[0]
format_code = fin.read(8)
illus_len = size_of_header - 36
illus = fin.read(illus_len)
if sys.version_info < (3, 0, 0):
code_type = fin.read(20).decode('ASCII')
else:
code_type = str(fin.read(20), 'ASCII')
code_len = struct.unpack("h", fin.read(2))[0]
bits_per_pix = struct.unpack("h", fin.read(2))[0]
if bits_per_pix == 1:
break
#Image Records (concatenated)
height = struct.unpack("I", fin.read(4))[0]
width = struct.unpack("I", fin.read(4))[0]
doc_img = np.zeros(shape=[height, width], dtype=np.uint8) + 255
voc_xml = PascalVocWriter(os.path.dirname(dgr), os.path.split(dgr)[-1][:-4] + '.jpg', doc_img.shape)
# Line Records (concatenated)
line_num = struct.unpack("I", fin.read(4))[0]
for i in range(line_num):
# Character Records (concatenated)
word_num = struct.unpack("I", fin.read(4))[0]
for j in range(word_num):
tmp_code = fin.read(code_len)
try:
if sys.version_info < (3, 0, 0):
label = tmp_code.decode('gbk')[0]
else:
label = str(tmp_code, ('gbk'))[0]
except:
label = u'Unknown'
top = struct.unpack("H", fin.read(2))[0]
left = struct.unpack("H", fin.read(2))[0]
char_height = struct.unpack("H", fin.read(2))[0]
char_width = struct.unpack("H", fin.read(2))[0]
tmp_img = np.zeros(shape=[char_height, char_width], dtype=np.uint8)
#Image data
for r in range(char_height):
for c in range(char_width):
tmp_img[r, c] = struct.unpack("B", fin.read(1))[0]
# 务必注意此处缩进,怎样实现单行图片对应单行文字输出有待编写
doc_img[top:top+char_height, left:left+char_width] = tmp_img
voc_xml.addBndBox(left, top, left + char_width, top+char_height, label)
return doc_img, voc_xml
测试数据共10449行
共 7356+1859 = 9215 类。即中文+符号+部分英文等,共9215种不同的字符
按照文本行生成数据集怎样切分标注与编号???
paddlepaddle下载地址
def decode_DGR_to_imgs_and_vocxml(dgr):
'''
:param dgr: a writer's encoded ground truth file.
:return: samples list, each sample with format (charname, img)
'''
doc_img, voc_xml = None, None
label_all = ''
lable_every_line = {}
doc_img_every_line = {}
with codecs.open(dgr, mode='rb') as fin:
while(True):
# Size of Header int 4B
left_cache = fin.read(4)
if len(left_cache) < 4:
break
# FILE HEAFER
size_of_header = struct.unpack("I", left_cache)[0]
format_code = fin.read(8)
# 36 = 4+8+20+2+2
# Illustration 类型:Text 长度:Arbitrary(任意长度)
illus_len = size_of_header - 36
# 此处读入数据
illus = fin.read(illus_len)
# 用于返回你使用的python版本号
# 此处应该是版本不同对字节的处理方式不同
# Code_type 类型:ASCII (char*) 长度:20B 举例:"ASCII", "GB", etc.
# by xie
if sys.version_info < (3, 0, 0):
code_type = fin.read(20).decode('ASCII')
else:
code_type = str(fin.read(20), 'ASCII')
# Code length 类型:Short 长度:2B 举例: 1, 2, 4, etc.
code_len = struct.unpack("h", fin.read(2))[0]
# print(code_len)
# Bits per pixel 类型:Short 长度:2B 举例:Typically1(B / Wimage), 8(Grayimage)
bits_per_pix = struct.unpack("h", fin.read(2))[0]
#
if bits_per_pix == 1:
break
# Image Records (concatenated)
height = struct.unpack("I", fin.read(4))[0]
# print(height)
width = struct.unpack("I", fin.read(4))[0]
# print(width)
doc_img = np.zeros(shape=[height, width], dtype=np.uint8) + 255
# print(doc_img)
voc_xml = PascalVocWriter(os.path.dirname(dgr), os.path.split(dgr)[-1][:-4] + '.jpg', doc_img.shape)
# Line Records (concatenated连接)
# 行数
line_num = struct.unpack("I", fin.read(4))[0]
# print(line_num)
# 循环遍历所有行
for i in range(line_num):
# Character Records (concatenated)每行字符个数
word_num = struct.unpack("I", fin.read(4))[0]
# print(word_num)
for j in range(word_num):
tmp_code = fin.read(code_len)
try:
if sys.version_info < (3, 0, 0):
label = tmp_code.decode('gbk')[0]
else:
label = str(tmp_code, ('gbk'))[0]
# print(label)
label_all = label_all + label
# print(label_all)
except:
label = u'Unknown'
lable_every_line[int(f'{i}')] = label_all
# if i+1 == line_num:
# print(lable_every_line)
label_all = ''
top = struct.unpack("I", fin.read(4))[0]
# print(top)
left = struct.unpack("I", fin.read(4))[0]
# print(left)
# 一行字符的高度
char_height = struct.unpack("I", fin.read(4))[0]
# print(char_height)
# 一行字符的宽度
char_width = struct.unpack("I", fin.read(4))[0]
# print(char_width)
tmp_img = np.zeros(shape=[char_height, char_width], dtype=np.uint8)
# print(tmp_img)
# Image data
for r in range(char_height):
for c in range(char_width):
tmp_img[r, c] = struct.unpack("B", fin.read(1))[0]
doc_img[top:top + char_height, left:left + char_width] = tmp_img
voc_xml.addBndBox(left, top, left + char_width, top+char_height, label)
doc_img_every_line[int(f'{i}')] = tmp_img
# print(doc_img_every_line)
# cv2.imwrite(os.path.join('F:/OCR/PaddleOCR-release-2.4/train_data/img', str(i) + '.jpg'), doc_img_every_line[int(f'{i}')])
return doc_img, voc_xml, line_num, doc_img_every_line, lable_every_line
def decode_HWDB_subset_v2(dgr_data_dir, img_save_dir, xml_writing_dir):
'''
解析并保存HWDB-v2的dgr格式数据到图像和相应的VOC格式的xml文件
:param dgr_data_dir:
:param img_save_dir:
:param xml_writing_dir:
:return:
'''
dgrs = os.listdir(dgr_data_dir)
for dgr in dgrs:
doc_img, voc_xml, line_num, doc_img_every_line, lable_every_line = decode_DGR_to_imgs_and_vocxml(os.path.join(dgr_data_dir, dgr))
for i in range(line_num):
cv2.imwrite(os.path.join('F:/OCR/PaddleOCR-release-2.4/train_data/img', str(i) + '.jpg'), doc_img_every_line[i])
print(lable_every_line[i])
# cv2.imwrite(os.path.join(img_save_dir, dgr[:-4] + '.jpg'), doc_img)
# voc_xml.save(xml_writing_dir + "/" + dgr[:-4] + XML_EXT)
print('Processed file %s.' % dgr)
if __name__ == '__main__':
# transform_labelmap_to_utf8('F:/OCR/PaddleOCR-release-2.4/train_data/labelmap/2.txt',
# 'utf-8',
# 'F:/OCR/PaddleOCR-release-2.4/train_data/labelmap/2.txt')
# decode_HWDB_subset_v1('F:/OCR/PaddleOCR-release-2.4/train_data/HWDB1.0-1.2/Gnt1.0Test/',
# 'F:/OCR/PaddleOCR-release-2.4/train_data/img',
# 'F:/OCR/PaddleOCR-release-2.4/train_data/labelmap/2.txt')
decode_HWDB_subset_v2('F:/OCR/PaddleOCR-release-2.4/train_data/HWDB2.0Test',
'F:/OCR/PaddleOCR-release-2.4/train_data/img',
'F:/OCR/PaddleOCR-release-2.4/train_data/img')
# 创建txt并写入
转换了一个训练集 HWDB2.0Train
转换一个测试集 HWDB2.0Test
行最大字符长度设置,现在看到的最长在46左右
KeyError: ‘LinearWarmup_LR’
见 第四节
cuda :10.2
conda install cudatoolkit=10.2
cudnn : 适配cuda10.2的 cudnn7.6.5
conda install cudnn=7.6.5
参考:https://zhuanlan.zhihu.com/p/367740437
nohup python tools/train.py -c ./configs/rec/ch_ppocr_v2.0/rec_chinese_common_train_v2.0.yml &
python tools/export_model.py -c configs/rec/ch_ppocr_v2.0/rec_chinese_common_train_v2.0.yml -o Global.pretrained_model=./inference/self_train/epoch31/best_accuracy Global.save_inference_dir=./inference/self_train/epoch31/
基本不可用
————原因在于新的训练集中并无大量的英文
- 训练后
- 分割结果
使用 det_db_score_mode=‘slow’ 后对文本行弯曲有缓解
主要在于DB分割,若分割无问题就转换为单行文本识别的问题
.
- 训练前
#### 10.7.6.2
## 10.8 compare_results.py的使用 ——功能理解错误
python test_tipc/compare_results.py --gt_file=./test_tipc/configs/ch_ppocr_server_v2.0_rec/model_linux_gpu_normal_normal_serving_python_linux_gpu_cpu.txt --log_file=./output/compare_results/train.log
- 训练前
- 训练后
- 百度手写识别API
- 自己的识别有弯曲文本的处理问题,只在图片类中弯曲文本被检测到的能力较强
第一次测试时很多是无法识别的,修改了检测边框的裁切ratio后可识别了
现阶段不具备可使用的能力
原因:
【Paddle打比赛】基于PaddleOCR的AIWIN手写体识别:关于测试评估方面
PaddleOCR 使用以及用自己的数据训练
PaddleOCR
├── configs ├── 配置文件,可通过 yml 文件选择模型结构并修改超参
│ ├── cls │ ├── 方向分类器相关配置文件
│ │ ├── cls_mv3.yml │ │ ├── 训练配置相关,包括骨干网络、head、loss、优化器和数据
│ ├── det │ ├── 检测相关配置文件
│ │ ├── det_mv3_db.yml │ │ ├── 训练配置
│ │ ...
│ └── rec │ └── 识别相关配置文件
│ ├── rec_mv3_none_bilstm_ctc.yml │ ├── crnn 训练配置
│ ...
├── deploy ├── 部署相关
│ ├── android_demo │ ├── android_demo
│ │ ...
│ ├── cpp_infer │ ├── C++ infer
│ │ ├── CMakeLists.txt │ │ ├── Cmake 文件
│ │ ├── docs │ │ ├── 说明文档
│ │ │ └── windows_vs2019_build.md
│ │ ├── include │ │ ├── 头文件
│ │ │ ├── clipper.h │ │ │ ├── clipper 库
│ │ │ ├── config.h │ │ │ ├── 预测配置
│ │ │ ├── ocr_cls.h │ │ │ ├── 方向分类器
│ │ │ ├── ocr_det.h │ │ │ ├── 文字检测
│ │ │ ├── ocr_rec.h │ │ │ ├── 文字识别
│ │ │ ├── postprocess_op.h │ │ │ ├── 检测后处理
│ │ │ ├── preprocess_op.h │ │ │ ├── 检测预处理
│ │ │ └── utility.h │ │ │ └── 工具
│ │ ├── readme.md │ │ ├── 说明文档
│ │ ├── ...
│ │ ├── src │ │ ├── 源文件
│ │ │ ├── clipper.cpp
│ │ │ ├── config.cpp
│ │ │ ├── main.cpp
│ │ │ ├── ocr_cls.cpp
│ │ │ ├── ocr_det.cpp
│ │ │ ├── ocr_rec.cpp
│ │ │ ├── postprocess_op.cpp
│ │ │ ├── preprocess_op.cpp
│ │ │ └── utility.cpp
│ │ └── tools │ │ └── 编译、执行脚本
│ │ ├── build.sh │ │ ├── 编译脚本
│ │ ├── config.txt │ │ ├── 配置文件
│ │ └── run.sh │ │ └── 测试启动脚本
│ ├── docker
│ │ └── hubserving
│ │ ├── cpu
│ │ │ └── Dockerfile
│ │ ├── gpu
│ │ │ └── Dockerfile
│ │ ├── README_cn.md
│ │ ├── README.md
│ │ └── sample_request.txt
│ ├── hubserving │ ├── hubserving
│ │ ├── ocr_cls │ │ ├── 方向分类器
│ │ │ ├── config.json │ │ │ ├── serving 配置
│ │ │ ├── __init__.py
│ │ │ ├── module.py │ │ │ ├── 预测模型
│ │ │ └── params.py │ │ │ └── 预测参数
│ │ ├── ocr_det │ │ ├── 文字检测
│ │ │ ├── config.json │ │ │ ├── serving 配置
│ │ │ ├── __init__.py
│ │ │ ├── module.py │ │ │ ├── 预测模型
│ │ │ └── params.py │ │ │ └── 预测参数
│ │ ├── ocr_rec │ │ ├── 文字识别
│ │ │ ├── config.json
│ │ │ ├── __init__.py
│ │ │ ├── module.py
│ │ │ └── params.py
│ │ └── ocr_system │ │ └── 系统预测
│ │ ├── config.json
│ │ ├── __init__.py
│ │ ├── module.py
│ │ └── params.py
│ ├── imgs │ ├── 预测图片
│ │ ├── cpp_infer_pred_12.png
│ │ └── demo.png
│ ├── ios_demo │ ├── ios demo
│ │ ...
│ ├── lite │ ├── lite 部署
│ │ ├── cls_process.cc │ │ ├── 方向分类器数据处理
│ │ ├── cls_process.h
│ │ ├── config.txt │ │ ├── 检测配置参数
│ │ ├── crnn_process.cc │ │ ├── crnn 数据处理
│ │ ├── crnn_process.h
│ │ ├── db_post_process.cc │ │ ├── db 数据处理
│ │ ├── db_post_process.h
│ │ ├── Makefile │ │ ├── 编译文件
│ │ ├── ocr_db_crnn.cc │ │ ├── 串联预测
│ │ ├── prepare.sh │ │ ├── 数据准备
│ │ ├── readme.md │ │ ├── 说明文档
│ │ ...
│ ├── pdserving │ ├── pdserving 部署
│ │ ├── det_local_server.py │ │ ├── 检测 快速版,部署方便预测速度快
│ │ ├── det_web_server.py │ │ ├── 检测 完整版,稳定性高分布式部署
│ │ ├── ocr_local_server.py │ │ ├── 检测+识别 快速版
│ │ ├── ocr_web_client.py │ │ ├── 客户端
│ │ ├── ocr_web_server.py │ │ ├── 检测+识别 完整版
│ │ ├── readme.md │ │ ├── 说明文档
│ │ ├── rec_local_server.py │ │ ├── 识别 快速版
│ │ └── rec_web_server.py │ │ └── 识别 完整版
│ └── slim
│ └── quantization │ └── 量化相关
│ ├── export_model.py │ ├── 导出模型
│ ├── quant.py │ ├── 量化
│ └── README.md │ └── 说明文档
├── doc ├── 文档教程
│ ...
├── ppocr ├── 网络核心代码
│ ├── data │ ├── 数据处理
│ │ ├── imaug │ │ ├── 图片和 label 处理代码
│ │ │ ├── text_image_aug │ │ │ ├── 文本识别的 tia 数据扩充
│ │ │ │ ├── __init__.py
│ │ │ │ ├── augment.py │ │ │ │ ├── tia_distort,tia_stretch 和 tia_perspective 的代码
│ │ │ │ ├── warp_mls.py
│ │ │ ├── __init__.py
│ │ │ ├── east_process.py │ │ │ ├── EAST 算法的数据处理步骤
│ │ │ ├── make_border_map.py │ │ │ ├── 生成边界图
│ │ │ ├── make_shrink_map.py │ │ │ ├── 生成收缩图
│ │ │ ├── operators.py │ │ │ ├── 图像基本操作,如读取和归一化
│ │ │ ├── randaugment.py │ │ │ ├── 随机数据增广操作
│ │ │ ├── random_crop_data.py │ │ │ ├── 随机裁剪
│ │ │ ├── rec_img_aug.py │ │ │ ├── 文本识别的数据扩充
│ │ │ └── sast_process.py │ │ │ └── SAST 算法的数据处理步骤
│ │ ├── __init__.py │ │ ├── 构造 dataloader 相关代码
│ │ ├── lmdb_dataset.py │ │ ├── 读取lmdb数据集的 dataset
│ │ ├── simple_dataset.py │ │ ├── 读取文本格式存储数据集的 dataset
│ ├── losses │ ├── 损失函数
│ │ ├── __init__.py │ │ ├── 构造 loss 相关代码
│ │ ├── cls_loss.py │ │ ├── 方向分类器 loss
│ │ ├── det_basic_loss.py │ │ ├── 检测基础 loss
│ │ ├── det_db_loss.py │ │ ├── DB loss
│ │ ├── det_east_loss.py │ │ ├── EAST loss
│ │ ├── det_sast_loss.py │ │ ├── SAST loss
│ │ ├── rec_ctc_loss.py │ │ ├── CTC loss
│ │ ├── rec_att_loss.py │ │ ├── Attention loss
│ ├── metrics │ ├── 评估指标
│ │ ├── __init__.py │ │ ├── 构造 metric 相关代码
│ │ ├── cls_metric.py │ │ ├── 方向分类器 metric
│ │ ├── det_metric.py │ │ ├── 检测 metric
│ │ ├── eval_det_iou.py │ │ ├── 检测 iou 相关
│ │ ├── rec_metric.py │ │ ├── 识别 metric
│ ├── modeling │ ├── 组网相关
│ │ ├── architectures │ │ ├── 网络
│ │ │ ├── __init__.py │ │ │ ├── 构造 model 相关代码
│ │ │ ├── base_model.py │ │ │ ├──/ 组网代码
│ │ ├── backbones │ │ ├── 骨干网络
│ │ │ ├── __init__.py │ │ │ ├── 构造 backbone 相关代码
│ │ │ ├── det_mobilenet_v3.py │ │ │ ├── 检测 mobilenet_v3
│ │ │ ├── det_resnet_vd.py │ │ │ ├── 检测 resnet
│ │ │ ├── det_resnet_vd_sast.py │ │ │ ├── 检测 SAST算法的resnet backbone
│ │ │ ├── rec_mobilenet_v3.py │ │ │ ├── 识别 mobilenet_v3
│ │ │ └── rec_resnet_vd.py │ │ │ ├── 识别 resnet
│ │ ├── necks │ │ ├── 颈函数
│ │ │ ├── __init__.py │ │ │ ├── 构造 neck 相关代码
│ │ │ ├── db_fpn.py │ │ │ ├── 标准 fpn 网络
│ │ │ ├── east_fpn.py │ │ │ ├── EAST 算法的 fpn 网络
│ │ │ ├── sast_fpn.py │ │ │ ├── SAST 算法的 fpn 网络
│ │ │ ├── rnn.py │ │ │ ├── 识别 序列编码
│ │ ├── heads │ │ ├── 头函数
│ │ │ ├── __init__.py │ │ │ ├── 构造 head 相关代码
│ │ │ ├── cls_head.py │ │ │ ├── 方向分类器 分类头
│ │ │ ├── det_db_head.py │ │ │ ├── DB 检测头
│ │ │ ├── det_east_head.py │ │ │ ├── EAST 检测头
│ │ │ ├── det_sast_head.py │ │ │ ├── SAST 检测头
│ │ │ ├── rec_ctc_head.py │ │ │ ├── 识别 ctc
│ │ │ ├── rec_att_head.py │ │ │ ├── 识别 attention
│ │ ├── transforms │ │ ├── 图像变换
│ │ │ ├── __init__.py │ │ │ ├── 构造 transform 相关代码
│ │ │ └── tps.py │ │ │ └── TPS 变换
│ ├── optimizer │ ├── 优化器
│ │ ├── __init__.py │ │ ├── 构造 optimizer 相关代码
│ │ └── learning_rate.py │ │ └── 学习率衰减
│ │ └── optimizer.py │ │ └── 优化器
│ │ └── regularizer.py │ │ └── 网络正则化
│ ├── postprocess │ ├── 后处理
│ │ ├── cls_postprocess.py │ │ ├── 方向分类器 后处理
│ │ ├── db_postprocess.py │ │ ├── DB 后处理
│ │ ├── east_postprocess.py │ │ ├── EAST 后处理
│ │ ├── locality_aware_nms.py │ │ ├── NMS
│ │ ├── rec_postprocess.py │ │ ├── 识别网络 后处理
│ │ └── sast_postprocess.py │ │ └── SAST 后处理
│ └── utils │ └── 工具
│ ├── dict │ ├── 小语种字典
│ ....
│ ├── ic15_dict.txt │ ├── 英文数字字典,区分大小写
│ ├── ppocr_keys_v1.txt │ ├── 中文字典,用于训练中文模型
│ ├── logging.py │ ├── logger
│ ├── save_load.py │ ├── 模型保存和加载函数
│ ├── stats.py │ ├── 统计
│ └── utility.py │ └── 工具函数
├── tools
│ ├── eval.py │ ├── 评估函数
│ ├── export_model.py │ ├── 导出 inference 模型
│ ├── infer │ ├── 基于预测引擎预测
│ │ ├── predict_cls.py
│ │ ├── predict_det.py
│ │ ├── predict_rec.py
│ │ ├── predict_system.py
│ │ └── utility.py
│ ├── infer_cls.py │ ├── 基于训练引擎 预测分类
│ ├── infer_det.py │ ├── 基于训练引擎 预测检测
│ ├── infer_rec.py │ ├── 基于训练引擎 预测识别
│ ├── program.py │ ├── 整体流程
│ ├── test_hubserving.py
│ └── train.py │ └── 启动训练
├── paddleocr.py
├── README_ch.md ├── 中文说明文档
├── README_en.md ├── 英文说明文档
├── README.md ├── 主页说明文档
├── requirements.txt ├── 安装依赖
├── setup.py ├── whl包打包脚本
├── train.sh ├── 启动训练脚本