鲍鱼数据集案例实战)
- 数据集探索性分析
- 鲍鱼数据预处理
- 对sex特征进行OneHot编码,便于后续模型纳入哑变量
- 筛选特征
- 将鲍鱼数据集划分为训练集和测试集
- 实现线性回归和岭回归
- 使用numpy实现线性回归
- 使用sklearn实现线性回归
- 使用Numpy实现岭回归
- 利用sklearn实现岭回归
- 岭迹分析
- 使用LASSO构建鲍鱼年龄预测模型
- LASSO的正则化路径
- 残差图
数据集探索性分析
import pandas as pd
import warnings
warnings.filterwarnings('ignore')
data=pd.read_csv(r"E:\大二下\机器学习实践\abalone_dataset.csv")
data.head()
|
sex |
length |
diameter |
height |
whole weight |
shucked weight |
viscera weight |
shell weight |
rings |
0 |
M |
0.455 |
0.365 |
0.095 |
0.5140 |
0.2245 |
0.1010 |
0.150 |
15 |
1 |
M |
0.350 |
0.265 |
0.090 |
0.2255 |
0.0995 |
0.0485 |
0.070 |
7 |
2 |
F |
0.530 |
0.420 |
0.135 |
0.6770 |
0.2565 |
0.1415 |
0.210 |
9 |
3 |
M |
0.440 |
0.365 |
0.125 |
0.5160 |
0.2155 |
0.1140 |
0.155 |
10 |
4 |
I |
0.330 |
0.255 |
0.080 |
0.2050 |
0.0895 |
0.0395 |
0.055 |
7 |
data.shape
(4177, 9)
data.info()
RangeIndex: 4177 entries, 0 to 4176
Data columns (total 9 columns):
sex 4177 non-null object
length 4177 non-null float64
diameter 4177 non-null float64
height 4177 non-null float64
whole weight 4177 non-null float64
shucked weight 4177 non-null float64
viscera weight 4177 non-null float64
shell weight 4177 non-null float64
rings 4177 non-null int64
dtypes: float64(7), int64(1), object(1)
memory usage: 293.8+ KB
data.describe()
|
length |
diameter |
height |
whole weight |
shucked weight |
viscera weight |
shell weight |
rings |
count |
4177.000000 |
4177.000000 |
4177.000000 |
4177.000000 |
4177.000000 |
4177.000000 |
4177.000000 |
4177.000000 |
mean |
0.523992 |
0.407881 |
0.139516 |
0.828742 |
0.359367 |
0.180594 |
0.238831 |
9.933684 |
std |
0.120093 |
0.099240 |
0.041827 |
0.490389 |
0.221963 |
0.109614 |
0.139203 |
3.224169 |
min |
0.075000 |
0.055000 |
0.000000 |
0.002000 |
0.001000 |
0.000500 |
0.001500 |
1.000000 |
25% |
0.450000 |
0.350000 |
0.115000 |
0.441500 |
0.186000 |
0.093500 |
0.130000 |
8.000000 |
50% |
0.545000 |
0.425000 |
0.140000 |
0.799500 |
0.336000 |
0.171000 |
0.234000 |
9.000000 |
75% |
0.615000 |
0.480000 |
0.165000 |
1.153000 |
0.502000 |
0.253000 |
0.329000 |
11.000000 |
max |
0.815000 |
0.650000 |
1.130000 |
2.825500 |
1.488000 |
0.760000 |
1.005000 |
29.000000 |

import seaborn as sns
import matplotlib.pyplot as plt
%matplotlib inline
sns.countplot(x = "sex",data=data)
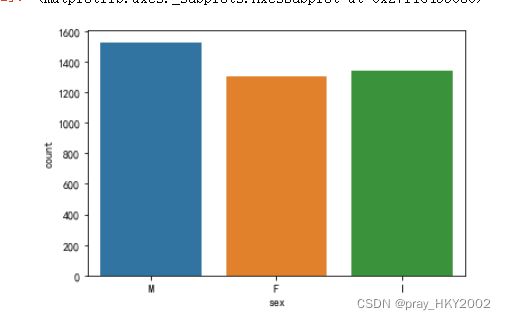
data['sex'].value_counts()
M 1528
I 1342
F 1307
Name: sex, dtype: int64
i=1
plt.figure(figsize=(16,8))
for col in data.columns[1:]:
plt.subplot(4,2,i)
i = i + 1
sns.distplot(data[col])
plt.tight_layout()
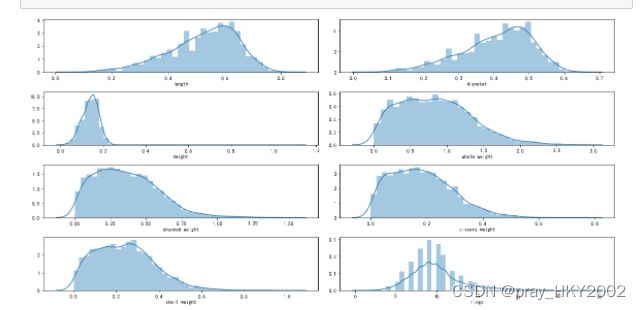
sns.pairplot(data,hue="sex")
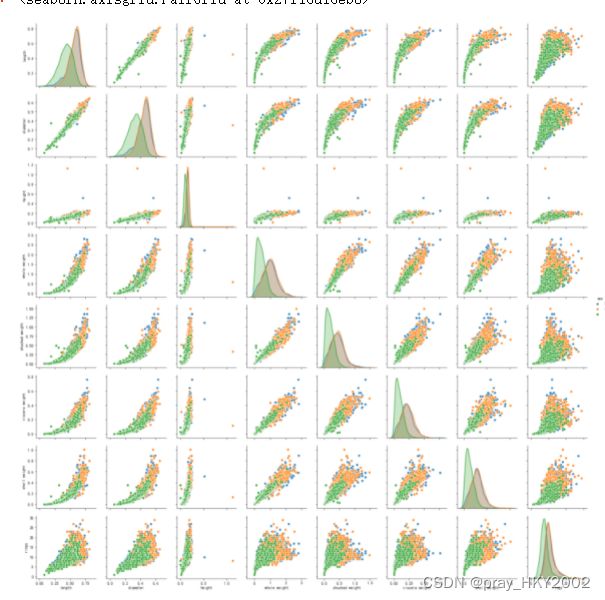
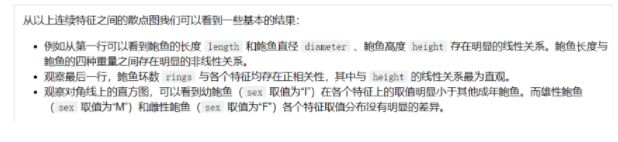
corr_df = data.corr()
corr_df
|
length |
diameter |
height |
whole weight |
shucked weight |
viscera weight |
shell weight |
rings |
length |
1.000000 |
0.986812 |
0.827554 |
0.925261 |
0.897914 |
0.903018 |
0.897706 |
0.556720 |
diameter |
0.986812 |
1.000000 |
0.833684 |
0.925452 |
0.893162 |
0.899724 |
0.905330 |
0.574660 |
height |
0.827554 |
0.833684 |
1.000000 |
0.819221 |
0.774972 |
0.798319 |
0.817338 |
0.557467 |
whole weight |
0.925261 |
0.925452 |
0.819221 |
1.000000 |
0.969405 |
0.966375 |
0.955355 |
0.540390 |
shucked weight |
0.897914 |
0.893162 |
0.774972 |
0.969405 |
1.000000 |
0.931961 |
0.882617 |
0.420884 |
viscera weight |
0.903018 |
0.899724 |
0.798319 |
0.966375 |
0.931961 |
1.000000 |
0.907656 |
0.503819 |
shell weight |
0.897706 |
0.905330 |
0.817338 |
0.955355 |
0.882617 |
0.907656 |
1.000000 |
0.627574 |
rings |
0.556720 |
0.574660 |
0.557467 |
0.540390 |
0.420884 |
0.503819 |
0.627574 |
1.000000 |
fig ,ax =plt.subplots(figsize=(12,12))
ax = sns.heatmap(corr_df,linewidths=.5,
cmap="Greens",
annot=True,
xticklabels=corr_df.columns,
yticklabels=corr_df.index)
ax.xaxis.set_label_position('top')
ax.xaxis.tick_top()
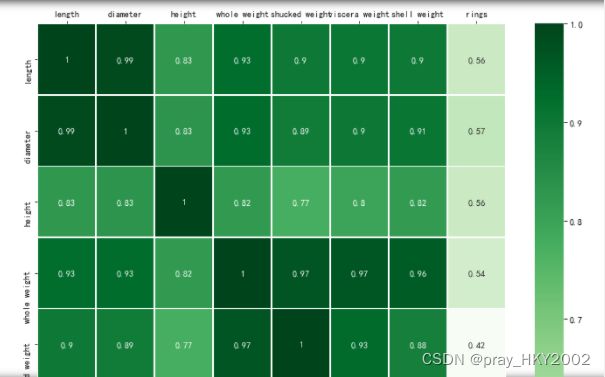
鲍鱼数据预处理
对sex特征进行OneHot编码,便于后续模型纳入哑变量
sex_onehot = pd.get_dummies(data["sex"],prefix="sex")
data[sex_onehot.columns] = sex_onehot
data.head()
|
sex |
length |
diameter |
height |
whole weight |
shucked weight |
viscera weight |
shell weight |
rings |
sex_F |
sex_I |
sex_M |
0 |
M |
0.455 |
0.365 |
0.095 |
0.5140 |
0.2245 |
0.1010 |
0.150 |
15 |
0 |
0 |
1 |
1 |
M |
0.350 |
0.265 |
0.090 |
0.2255 |
0.0995 |
0.0485 |
0.070 |
7 |
0 |
0 |
1 |
2 |
F |
0.530 |
0.420 |
0.135 |
0.6770 |
0.2565 |
0.1415 |
0.210 |
9 |
1 |
0 |
0 |
3 |
M |
0.440 |
0.365 |
0.125 |
0.5160 |
0.2155 |
0.1140 |
0.155 |
10 |
0 |
0 |
1 |
4 |
I |
0.330 |
0.255 |
0.080 |
0.2050 |
0.0895 |
0.0395 |
0.055 |
7 |
0 |
1 |
0 |
data["ones"]=1
data.head()
|
sex |
length |
diameter |
height |
whole weight |
shucked weight |
viscera weight |
shell weight |
rings |
sex_F |
sex_I |
sex_M |
ones |
0 |
M |
0.455 |
0.365 |
0.095 |
0.5140 |
0.2245 |
0.1010 |
0.150 |
15 |
0 |
0 |
1 |
1 |
1 |
M |
0.350 |
0.265 |
0.090 |
0.2255 |
0.0995 |
0.0485 |
0.070 |
7 |
0 |
0 |
1 |
1 |
2 |
F |
0.530 |
0.420 |
0.135 |
0.6770 |
0.2565 |
0.1415 |
0.210 |
9 |
1 |
0 |
0 |
1 |
3 |
M |
0.440 |
0.365 |
0.125 |
0.5160 |
0.2155 |
0.1140 |
0.155 |
10 |
0 |
0 |
1 |
1 |
4 |
I |
0.330 |
0.255 |
0.080 |
0.2050 |
0.0895 |
0.0395 |
0.055 |
7 |
0 |
1 |
0 |
1 |
data["age"]=data["rings"] + 1.5
data.head()
|
sex |
length |
diameter |
height |
whole weight |
shucked weight |
viscera weight |
shell weight |
rings |
sex_F |
sex_I |
sex_M |
ones |
age |
0 |
M |
0.455 |
0.365 |
0.095 |
0.5140 |
0.2245 |
0.1010 |
0.150 |
15 |
0 |
0 |
1 |
1 |
16.5 |
1 |
M |
0.350 |
0.265 |
0.090 |
0.2255 |
0.0995 |
0.0485 |
0.070 |
7 |
0 |
0 |
1 |
1 |
8.5 |
2 |
F |
0.530 |
0.420 |
0.135 |
0.6770 |
0.2565 |
0.1415 |
0.210 |
9 |
1 |
0 |
0 |
1 |
10.5 |
3 |
M |
0.440 |
0.365 |
0.125 |
0.5160 |
0.2155 |
0.1140 |
0.155 |
10 |
0 |
0 |
1 |
1 |
11.5 |
4 |
I |
0.330 |
0.255 |
0.080 |
0.2050 |
0.0895 |
0.0395 |
0.055 |
7 |
0 |
1 |
0 |
1 |
8.5 |
筛选特征
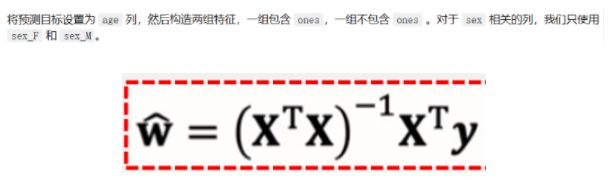
data.columns
Index(['sex', 'length', 'diameter', 'height', 'whole weight', 'shucked weight',
'viscera weight', 'shell weight', 'rings', 'sex_F', 'sex_I', 'sex_M',
'ones', 'age'],
dtype='object')
y = data["age"]
features_with_ones = ["length", "diameter", "height", "whole weight", "shucked weight",
"viscera weight", "shell weight", "sex_F", "sex_M","ones"]
features_without_ones = ["length", "diameter", "height", "whole weight", "shucked weight",
"viscera weight", "shell weight", "sex_F", "sex_M"]
X=data[features_with_ones]
将鲍鱼数据集划分为训练集和测试集
![[外链图片转存失败,源站可能有防盗链机制,建议将图片保存下来直接上传(img-9FJF6qY1-1648017224763)(attachment:image.png)]](http://img.e-com-net.com/image/info8/b04edbe48f5a4f9cb4310b8cee0aa74e.jpg)
from sklearn.model_selection import train_test_split
X_train,X_test,y_train,y_test = train_test_split(X,y,test_size=0.2,random_state=111)
X
|
length |
diameter |
height |
whole weight |
shucked weight |
viscera weight |
shell weight |
sex_F |
sex_M |
ones |
0 |
0.455 |
0.365 |
0.095 |
0.5140 |
0.2245 |
0.1010 |
0.1500 |
0 |
1 |
1 |
1 |
0.350 |
0.265 |
0.090 |
0.2255 |
0.0995 |
0.0485 |
0.0700 |
0 |
1 |
1 |
2 |
0.530 |
0.420 |
0.135 |
0.6770 |
0.2565 |
0.1415 |
0.2100 |
1 |
0 |
1 |
3 |
0.440 |
0.365 |
0.125 |
0.5160 |
0.2155 |
0.1140 |
0.1550 |
0 |
1 |
1 |
4 |
0.330 |
0.255 |
0.080 |
0.2050 |
0.0895 |
0.0395 |
0.0550 |
0 |
0 |
1 |
5 |
0.425 |
0.300 |
0.095 |
0.3515 |
0.1410 |
0.0775 |
0.1200 |
0 |
0 |
1 |
6 |
0.530 |
0.415 |
0.150 |
0.7775 |
0.2370 |
0.1415 |
0.3300 |
1 |
0 |
1 |
7 |
0.545 |
0.425 |
0.125 |
0.7680 |
0.2940 |
0.1495 |
0.2600 |
1 |
0 |
1 |
8 |
0.475 |
0.370 |
0.125 |
0.5095 |
0.2165 |
0.1125 |
0.1650 |
0 |
1 |
1 |
9 |
0.550 |
0.440 |
0.150 |
0.8945 |
0.3145 |
0.1510 |
0.3200 |
1 |
0 |
1 |
10 |
0.525 |
0.380 |
0.140 |
0.6065 |
0.1940 |
0.1475 |
0.2100 |
1 |
0 |
1 |
11 |
0.430 |
0.350 |
0.110 |
0.4060 |
0.1675 |
0.0810 |
0.1350 |
0 |
1 |
1 |
12 |
0.490 |
0.380 |
0.135 |
0.5415 |
0.2175 |
0.0950 |
0.1900 |
0 |
1 |
1 |
13 |
0.535 |
0.405 |
0.145 |
0.6845 |
0.2725 |
0.1710 |
0.2050 |
1 |
0 |
1 |
14 |
0.470 |
0.355 |
0.100 |
0.4755 |
0.1675 |
0.0805 |
0.1850 |
1 |
0 |
1 |
15 |
0.500 |
0.400 |
0.130 |
0.6645 |
0.2580 |
0.1330 |
0.2400 |
0 |
1 |
1 |
16 |
0.355 |
0.280 |
0.085 |
0.2905 |
0.0950 |
0.0395 |
0.1150 |
0 |
0 |
1 |
17 |
0.440 |
0.340 |
0.100 |
0.4510 |
0.1880 |
0.0870 |
0.1300 |
1 |
0 |
1 |
18 |
0.365 |
0.295 |
0.080 |
0.2555 |
0.0970 |
0.0430 |
0.1000 |
0 |
1 |
1 |
19 |
0.450 |
0.320 |
0.100 |
0.3810 |
0.1705 |
0.0750 |
0.1150 |
0 |
1 |
1 |
20 |
0.355 |
0.280 |
0.095 |
0.2455 |
0.0955 |
0.0620 |
0.0750 |
0 |
1 |
1 |
21 |
0.380 |
0.275 |
0.100 |
0.2255 |
0.0800 |
0.0490 |
0.0850 |
0 |
0 |
1 |
22 |
0.565 |
0.440 |
0.155 |
0.9395 |
0.4275 |
0.2140 |
0.2700 |
1 |
0 |
1 |
23 |
0.550 |
0.415 |
0.135 |
0.7635 |
0.3180 |
0.2100 |
0.2000 |
1 |
0 |
1 |
24 |
0.615 |
0.480 |
0.165 |
1.1615 |
0.5130 |
0.3010 |
0.3050 |
1 |
0 |
1 |
25 |
0.560 |
0.440 |
0.140 |
0.9285 |
0.3825 |
0.1880 |
0.3000 |
1 |
0 |
1 |
26 |
0.580 |
0.450 |
0.185 |
0.9955 |
0.3945 |
0.2720 |
0.2850 |
1 |
0 |
1 |
27 |
0.590 |
0.445 |
0.140 |
0.9310 |
0.3560 |
0.2340 |
0.2800 |
0 |
1 |
1 |
28 |
0.605 |
0.475 |
0.180 |
0.9365 |
0.3940 |
0.2190 |
0.2950 |
0 |
1 |
1 |
29 |
0.575 |
0.425 |
0.140 |
0.8635 |
0.3930 |
0.2270 |
0.2000 |
0 |
1 |
1 |
... |
... |
... |
... |
... |
... |
... |
... |
... |
... |
... |
4147 |
0.695 |
0.550 |
0.195 |
1.6645 |
0.7270 |
0.3600 |
0.4450 |
0 |
1 |
1 |
4148 |
0.770 |
0.605 |
0.175 |
2.0505 |
0.8005 |
0.5260 |
0.3550 |
0 |
1 |
1 |
4149 |
0.280 |
0.215 |
0.070 |
0.1240 |
0.0630 |
0.0215 |
0.0300 |
0 |
0 |
1 |
4150 |
0.330 |
0.230 |
0.080 |
0.1400 |
0.0565 |
0.0365 |
0.0460 |
0 |
0 |
1 |
4151 |
0.350 |
0.250 |
0.075 |
0.1695 |
0.0835 |
0.0355 |
0.0410 |
0 |
0 |
1 |
4152 |
0.370 |
0.280 |
0.090 |
0.2180 |
0.0995 |
0.0545 |
0.0615 |
0 |
0 |
1 |
4153 |
0.430 |
0.315 |
0.115 |
0.3840 |
0.1885 |
0.0715 |
0.1100 |
0 |
0 |
1 |
4154 |
0.435 |
0.330 |
0.095 |
0.3930 |
0.2190 |
0.0750 |
0.0885 |
0 |
0 |
1 |
4155 |
0.440 |
0.350 |
0.110 |
0.3805 |
0.1575 |
0.0895 |
0.1150 |
0 |
0 |
1 |
4156 |
0.475 |
0.370 |
0.110 |
0.4895 |
0.2185 |
0.1070 |
0.1460 |
0 |
1 |
1 |
4157 |
0.475 |
0.360 |
0.140 |
0.5135 |
0.2410 |
0.1045 |
0.1550 |
0 |
1 |
1 |
4158 |
0.480 |
0.355 |
0.110 |
0.4495 |
0.2010 |
0.0890 |
0.1400 |
0 |
0 |
1 |
4159 |
0.560 |
0.440 |
0.135 |
0.8025 |
0.3500 |
0.1615 |
0.2590 |
1 |
0 |
1 |
4160 |
0.585 |
0.475 |
0.165 |
1.0530 |
0.4580 |
0.2170 |
0.3000 |
1 |
0 |
1 |
4161 |
0.585 |
0.455 |
0.170 |
0.9945 |
0.4255 |
0.2630 |
0.2845 |
1 |
0 |
1 |
4162 |
0.385 |
0.255 |
0.100 |
0.3175 |
0.1370 |
0.0680 |
0.0920 |
0 |
1 |
1 |
4163 |
0.390 |
0.310 |
0.085 |
0.3440 |
0.1810 |
0.0695 |
0.0790 |
0 |
0 |
1 |
4164 |
0.390 |
0.290 |
0.100 |
0.2845 |
0.1255 |
0.0635 |
0.0810 |
0 |
0 |
1 |
4165 |
0.405 |
0.300 |
0.085 |
0.3035 |
0.1500 |
0.0505 |
0.0880 |
0 |
0 |
1 |
4166 |
0.475 |
0.365 |
0.115 |
0.4990 |
0.2320 |
0.0885 |
0.1560 |
0 |
0 |
1 |
4167 |
0.500 |
0.380 |
0.125 |
0.5770 |
0.2690 |
0.1265 |
0.1535 |
0 |
1 |
1 |
4168 |
0.515 |
0.400 |
0.125 |
0.6150 |
0.2865 |
0.1230 |
0.1765 |
1 |
0 |
1 |
4169 |
0.520 |
0.385 |
0.165 |
0.7910 |
0.3750 |
0.1800 |
0.1815 |
0 |
1 |
1 |
4170 |
0.550 |
0.430 |
0.130 |
0.8395 |
0.3155 |
0.1955 |
0.2405 |
0 |
1 |
1 |
4171 |
0.560 |
0.430 |
0.155 |
0.8675 |
0.4000 |
0.1720 |
0.2290 |
0 |
1 |
1 |
4172 |
0.565 |
0.450 |
0.165 |
0.8870 |
0.3700 |
0.2390 |
0.2490 |
1 |
0 |
1 |
4173 |
0.590 |
0.440 |
0.135 |
0.9660 |
0.4390 |
0.2145 |
0.2605 |
0 |
1 |
1 |
4174 |
0.600 |
0.475 |
0.205 |
1.1760 |
0.5255 |
0.2875 |
0.3080 |
0 |
1 |
1 |
4175 |
0.625 |
0.485 |
0.150 |
1.0945 |
0.5310 |
0.2610 |
0.2960 |
1 |
0 |
1 |
4176 |
0.710 |
0.555 |
0.195 |
1.9485 |
0.9455 |
0.3765 |
0.4950 |
0 |
1 |
1 |
4177 rows × 10 columns
实现线性回归和岭回归
使用numpy实现线性回归
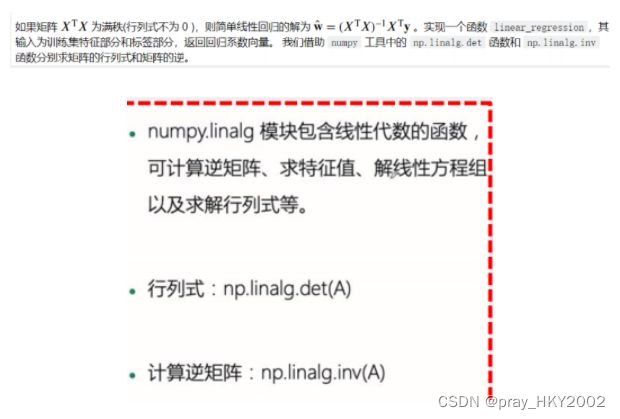
import numpy as np
def linear_regression(X,y):
w = np.zeros_like(X.shape[1])
if np.linalg.det(X.T.dot(X)) != 0:
w = np.linalg.inv(X.T.dot(X)).dot(X.T).dot(y)
return w
w1 = linear_regression(X_train,y_train)
w1 = pd.DataFrame(data = w1,index=X.columns,columns =["numpy_w"])
w1.round(decimals=2)
|
numpy_w |
length |
-1.12 |
diameter |
10.00 |
height |
20.74 |
whole weight |
9.61 |
shucked weight |
-20.05 |
viscera weight |
-12.07 |
shell weight |
6.55 |
sex_F |
0.88 |
sex_M |
0.87 |
ones |
4.32 |
![[外链图片转存失败,源站可能有防盗链机制,建议将图片保存下来直接上传(img-ev9UGYGo-1648017224765)(attachment:image.png)]](http://img.e-com-net.com/image/info8/08331409f5c842e5983a23720b4da7c9.jpg)
使用sklearn实现线性回归
from sklearn.linear_model import LinearRegression
lr = LinearRegression()
lr.fit(X_train[features_without_ones],y_train)
print(lr.coef_)
[ -1.118146 10.00094599 20.73712616 9.61484657 -20.05079291
-12.06849193 6.54529076 0.87855188 0.87283083]
w1
|
numpy_w |
length |
-1.118146 |
diameter |
10.000946 |
height |
20.737126 |
whole weight |
9.614847 |
shucked weight |
-20.050793 |
viscera weight |
-12.068492 |
shell weight |
6.545291 |
sex_F |
0.878552 |
sex_M |
0.872831 |
ones |
4.324477 |
w_lr=[]
w_lr.extend(lr.coef_)
w_lr.append(lr.intercept_)
w1["lr_sklearn_w"]=w_lr
w1.round(decimals=2)
|
numpy_w |
lr_sklearn_w |
length |
-1.12 |
-1.12 |
diameter |
10.00 |
10.00 |
height |
20.74 |
20.74 |
whole weight |
9.61 |
9.61 |
shucked weight |
-20.05 |
-20.05 |
viscera weight |
-12.07 |
-12.07 |
shell weight |
6.55 |
6.55 |
sex_F |
0.88 |
0.88 |
sex_M |
0.87 |
0.87 |
ones |
4.32 |
4.32 |
使用Numpy实现岭回归
def ridge_regression(X,y,ridge_lambda):
penalty_matrix = np.eye(X.shape[1])
penalty_matrix[X.shape[1]-1][X.shape[1]-1] = 0
w = np.linalg.inv(X.T.dot(X) + ridge_lambda * penalty_matrix).dot(X.T).dot(y)
return w
在鲍鱼训练集上使用ridge_regression函数训练岭回归模型,正则化系数设置为1
w2 = ridge_regression(X_train,y_train,1.0)
print(w2)
[ 2.30976528 6.72038628 10.23298909 7.05879189 -17.16249532
-7.2343118 9.3936994 0.96869974 0.9422174 4.80583032]
w1["numpy_ridge_w"] = w2
w1.round(decimals=2)
|
numpy_w |
lr_sklearn_w |
numpy_ridge_w |
length |
-1.12 |
-1.12 |
2.31 |
diameter |
10.00 |
10.00 |
6.72 |
height |
20.74 |
20.74 |
10.23 |
whole weight |
9.61 |
9.61 |
7.06 |
shucked weight |
-20.05 |
-20.05 |
-17.16 |
viscera weight |
-12.07 |
-12.07 |
-7.23 |
shell weight |
6.55 |
6.55 |
9.39 |
sex_F |
0.88 |
0.88 |
0.97 |
sex_M |
0.87 |
0.87 |
0.94 |
ones |
4.32 |
4.32 |
4.81 |
利用sklearn实现岭回归
与sklearn中岭回归对比,同样正则化叙述设置为1
from sklearn.linear_model import Ridge
ridge = Ridge(alpha=1.0)
ridge.fit(X_train[features_without_ones],y_train)
w_ridge = []
w_ridge.extend(ridge.coef_)
w_ridge.append(ridge.intercept_)
w1["ridge_sklearn_w"] = w_ridge
w1.round(decimals=2)
|
numpy_w |
lr_sklearn_w |
numpy_ridge_w |
ridge_sklearn_w |
length |
-1.12 |
-1.12 |
2.31 |
2.31 |
diameter |
10.00 |
10.00 |
6.72 |
6.72 |
height |
20.74 |
20.74 |
10.23 |
10.23 |
whole weight |
9.61 |
9.61 |
7.06 |
7.06 |
shucked weight |
-20.05 |
-20.05 |
-17.16 |
-17.16 |
viscera weight |
-12.07 |
-12.07 |
-7.23 |
-7.23 |
shell weight |
6.55 |
6.55 |
9.39 |
9.39 |
sex_F |
0.88 |
0.88 |
0.97 |
0.97 |
sex_M |
0.87 |
0.87 |
0.94 |
0.94 |
ones |
4.32 |
4.32 |
4.81 |
4.81 |
岭迹分析
alphas = np.logspace(-10,10,20)
coef = pd.DataFrame()
for alpha in alphas:
ridge_clf = Ridge(alpha=alpha)
ridge_clf.fit(X_train[features_without_ones],y_train)
df = pd.DataFrame([ridge_clf.coef_],columns=X_train[features_without_ones].columns)
df['alpha']=alpha
coef =coef.append(df,ignore_index=True)
coef.round(decimals=2)
|
length |
diameter |
height |
whole weight |
shucked weight |
viscera weight |
shell weight |
sex_F |
sex_M |
alpha |
0 |
-1.12 |
10.00 |
20.74 |
9.61 |
-20.05 |
-12.07 |
6.55 |
0.88 |
0.87 |
0.000000e+00 |
1 |
-1.12 |
10.00 |
20.74 |
9.61 |
-20.05 |
-12.07 |
6.55 |
0.88 |
0.87 |
0.000000e+00 |
2 |
-1.12 |
10.00 |
20.74 |
9.61 |
-20.05 |
-12.07 |
6.55 |
0.88 |
0.87 |
0.000000e+00 |
3 |
-1.12 |
10.00 |
20.74 |
9.61 |
-20.05 |
-12.07 |
6.55 |
0.88 |
0.87 |
0.000000e+00 |
4 |
-1.12 |
10.00 |
20.74 |
9.61 |
-20.05 |
-12.07 |
6.55 |
0.88 |
0.87 |
0.000000e+00 |
5 |
-1.12 |
10.00 |
20.74 |
9.61 |
-20.05 |
-12.07 |
6.55 |
0.88 |
0.87 |
0.000000e+00 |
6 |
-1.12 |
10.00 |
20.73 |
9.61 |
-20.05 |
-12.07 |
6.55 |
0.88 |
0.87 |
0.000000e+00 |
7 |
-1.10 |
9.98 |
20.68 |
9.60 |
-20.04 |
-12.05 |
6.56 |
0.88 |
0.87 |
0.000000e+00 |
8 |
-0.88 |
9.79 |
20.13 |
9.50 |
-19.94 |
-11.86 |
6.71 |
0.88 |
0.88 |
3.000000e-02 |
9 |
0.73 |
8.33 |
15.60 |
8.55 |
-18.97 |
-10.05 |
7.98 |
0.92 |
0.90 |
3.000000e-01 |
10 |
3.20 |
5.02 |
5.40 |
5.11 |
-13.71 |
-3.67 |
9.61 |
1.07 |
1.00 |
3.360000e+00 |
11 |
1.66 |
1.76 |
1.12 |
2.53 |
-3.54 |
-0.09 |
3.67 |
1.33 |
1.11 |
3.793000e+01 |
12 |
0.51 |
0.47 |
0.22 |
1.63 |
0.18 |
0.30 |
0.79 |
0.89 |
0.69 |
4.281300e+02 |
13 |
0.12 |
0.10 |
0.04 |
0.46 |
0.15 |
0.09 |
0.16 |
0.21 |
0.16 |
4.832930e+03 |
14 |
0.01 |
0.01 |
0.00 |
0.05 |
0.02 |
0.01 |
0.02 |
0.02 |
0.02 |
5.455595e+04 |
15 |
0.00 |
0.00 |
0.00 |
0.00 |
0.00 |
0.00 |
0.00 |
0.00 |
0.00 |
6.158482e+05 |
16 |
0.00 |
0.00 |
0.00 |
0.00 |
0.00 |
0.00 |
0.00 |
0.00 |
0.00 |
6.951928e+06 |
17 |
0.00 |
0.00 |
0.00 |
0.00 |
0.00 |
0.00 |
0.00 |
0.00 |
0.00 |
7.847600e+07 |
18 |
0.00 |
0.00 |
0.00 |
0.00 |
0.00 |
0.00 |
0.00 |
0.00 |
0.00 |
8.858668e+08 |
19 |
0.00 |
0.00 |
0.00 |
0.00 |
0.00 |
0.00 |
0.00 |
0.00 |
0.00 |
1.000000e+10 |
plt.rcParams['figure.dpi'] = 300
plt.figure(figsize=(9,6))
coef['alpha']=coef['alpha']
for feature in X_train.columns[:-1]:
plt.plot('alpha',feature,data=coef)
ax = plt.gca()
ax.set_xscale('log')
plt.legend(loc='upper right')
plt.xlabel(r'$\alpha$',fontsize=15)
plt.ylabel('系数',fontsize=15)
Text(0, 0.5, '系数')
Font 'default' does not have a glyph for '-' [U+2212], substituting with a dummy symbol.
Font 'default' does not have a glyph for '-' [U+2212], substituting with a dummy symbol.
Font 'default' does not have a glyph for '-' [U+2212], substituting with a dummy symbol.
Font 'default' does not have a glyph for '-' [U+2212], substituting with a dummy symbol.
Font 'default' does not have a glyph for '-' [U+2212], substituting with a dummy symbol.
Font 'default' does not have a glyph for '-' [U+2212], substituting with a dummy symbol.
Font 'default' does not have a glyph for '-' [U+2212], substituting with a dummy symbol.
Font 'default' does not have a glyph for '-' [U+2212], substituting with a dummy symbol.
Font 'default' does not have a glyph for '-' [U+2212], substituting with a dummy symbol.
Font 'default' does not have a glyph for '-' [U+2212], substituting with a dummy symbol.
Font 'default' does not have a glyph for '-' [U+2212], substituting with a dummy symbol.
Font 'default' does not have a glyph for '-' [U+2212], substituting with a dummy symbol.
Font 'default' does not have a glyph for '-' [U+2212], substituting with a dummy symbol.
Font 'default' does not have a glyph for '-' [U+2212], substituting with a dummy symbol.
Font 'default' does not have a glyph for '-' [U+2212], substituting with a dummy symbol.
Font 'default' does not have a glyph for '-' [U+2212], substituting with a dummy symbol.
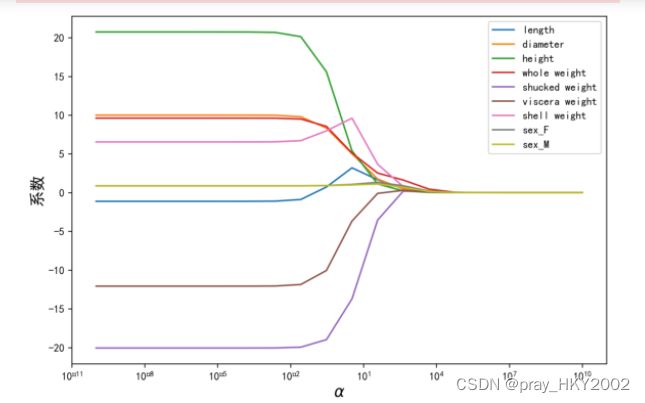
使用LASSO构建鲍鱼年龄预测模型
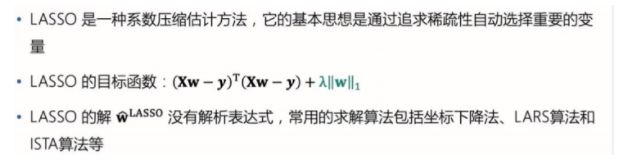
from sklearn.linear_model import Lasso
lasso = Lasso(alpha=0.01)
lasso.fit(X_train[features_without_ones],y_train)
print(lasso.coef_)
print(lasso.intercept_)
[ 0. 6.37435514 0. 4.46703234 -13.44947667
-0. 11.85934842 0.98908791 0.93313403]
6.500338023591298
LASSO的正则化路径
coef = pd.DataFrame()
for alpha in np.linspace(0.0001,0.2,20):
lasso_clf = Lasso(alpha=alpha)
lasso_clf.fit(X_train[features_without_ones],y_train)
df = pd.DataFrame([lasso_clf.coef_],columns=X_train[features_without_ones].columns)
df['alpha']=alpha
coef = coef.append(df,ignore_index=True)
coef.head()
plt.figure(figsize=(9,6),dpi=600)
for feature in X_train.columns[:-1]:
plt.plot('alpha',feature,data=coef)
plt.legend(loc='upper right')
plt.xlabel(r'$\alpha$',fontsize=15)
plt.ylabel('系数',fontsize=15)
plt.show()
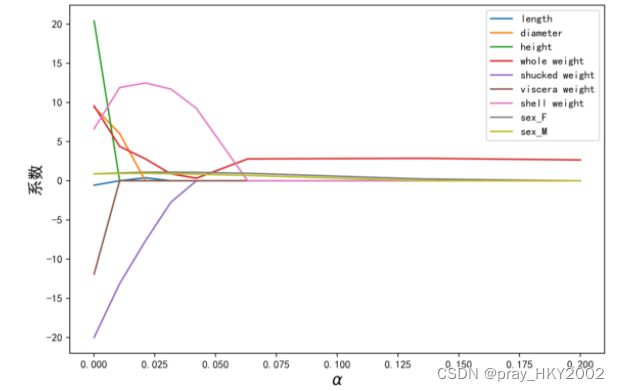
coef
|
length |
diameter |
height |
whole weight |
shucked weight |
viscera weight |
shell weight |
sex_F |
sex_M |
alpha |
0 |
-0.568043 |
9.39275 |
20.390041 |
9.542038 |
-19.995972 |
-11.900326 |
6.635352 |
0.881496 |
0.875132 |
0.000100 |
1 |
0.000000 |
6.02573 |
0.000000 |
4.375754 |
-13.127223 |
-0.000000 |
11.897189 |
0.995137 |
0.934129 |
0.010621 |
2 |
0.384927 |
0.00000 |
0.000000 |
2.797815 |
-7.702209 |
-0.000000 |
12.478541 |
1.093479 |
0.948281 |
0.021142 |
3 |
0.000000 |
0.00000 |
0.000000 |
0.884778 |
-2.749504 |
0.000000 |
11.705974 |
1.098990 |
0.897673 |
0.031663 |
4 |
0.000000 |
0.00000 |
0.000000 |
0.322742 |
-0.000000 |
0.000000 |
9.225919 |
1.072991 |
0.834021 |
0.042184 |
5 |
0.000000 |
0.00000 |
0.000000 |
1.555502 |
-0.000000 |
0.000000 |
4.610425 |
1.013824 |
0.757891 |
0.052705 |
6 |
0.000000 |
0.00000 |
0.000000 |
2.786784 |
-0.000000 |
0.000000 |
0.000000 |
0.954710 |
0.681821 |
0.063226 |
7 |
0.000000 |
0.00000 |
0.000000 |
2.797514 |
-0.000000 |
0.000000 |
0.000000 |
0.848412 |
0.581613 |
0.073747 |
8 |
0.000000 |
0.00000 |
0.000000 |
2.807843 |
-0.000000 |
0.000000 |
0.000000 |
0.742529 |
0.481711 |
0.084268 |
9 |
0.000000 |
0.00000 |
0.000000 |
2.818184 |
-0.000000 |
0.000000 |
0.000000 |
0.636632 |
0.381799 |
0.094789 |
10 |
0.000000 |
0.00000 |
0.000000 |
2.828630 |
-0.000000 |
0.000000 |
0.000000 |
0.530615 |
0.281801 |
0.105311 |
11 |
0.000000 |
0.00000 |
0.000000 |
2.838944 |
-0.000000 |
0.000000 |
0.000000 |
0.424750 |
0.181912 |
0.115832 |
12 |
0.000000 |
0.00000 |
0.000000 |
2.849325 |
-0.000000 |
0.000000 |
0.000000 |
0.318807 |
0.081967 |
0.126353 |
13 |
0.000000 |
0.00000 |
0.000000 |
2.851851 |
-0.000000 |
0.000000 |
0.000000 |
0.225024 |
0.000000 |
0.136874 |
14 |
0.000000 |
0.00000 |
0.000000 |
2.819079 |
-0.000000 |
0.000000 |
0.000000 |
0.186157 |
0.000000 |
0.147395 |
15 |
0.000000 |
0.00000 |
0.000000 |
2.786307 |
-0.000000 |
0.000000 |
0.000000 |
0.147290 |
0.000000 |
0.157916 |
16 |
0.000000 |
0.00000 |
0.000000 |
2.753535 |
0.000000 |
0.000000 |
0.000000 |
0.108422 |
0.000000 |
0.168437 |
17 |
0.000000 |
0.00000 |
0.000000 |
2.720762 |
0.000000 |
0.000000 |
0.000000 |
0.069555 |
0.000000 |
0.178958 |
18 |
0.000000 |
0.00000 |
0.000000 |
2.687990 |
0.000000 |
0.000000 |
0.000000 |
0.030688 |
0.000000 |
0.189479 |
19 |
0.000000 |
0.00000 |
0.000000 |
2.652940 |
0.000000 |
0.000000 |
0.000000 |
0.000000 |
0.000000 |
0.200000 |
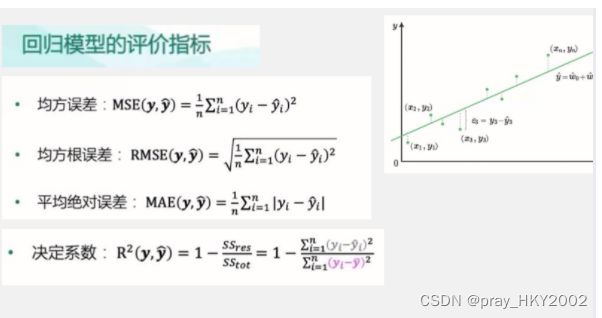
from sklearn.metrics import mean_squared_error
from sklearn.metrics import mean_absolute_error
from sklearn.metrics import r2_score
y_test_pred_lr = lr.predict(X_test.iloc[:,:-1])
print(round(mean_absolute_error(y_test,y_test_pred_lr),4))
1.6016
y_test_pred_ridge = ridge.predict(X_test[features_without_ones])
print(round(mean_absolute_error(y_test,y_test_pred_ridge),4))
1.5984
y_test_pred_lasso = lasso.predict(X_test[features_without_ones])
print(round(mean_absolute_error(y_test,y_test_pred_lasso),4))
1.6402
y_test_pred_lr = lr.predict(X_test.iloc[:,:-1])
print(round(mean_squared_error(y_test,y_test_pred_lr),4))
5.3009
y_test_pred_ridge = ridge.predict(X_test[features_without_ones])
print(round(mean_squared_error(y_test,y_test_pred_ridge),4))
4.959
y_test_pred_lasso = lasso.predict(X_test[features_without_ones])
print(round(mean_squared_error(y_test,y_test_pred_lasso),4))
5.1
print(round(r2_score(y_test,y_test_pred_lr),4))
print(round(r2_score(y_test,y_test_pred_ridge),4))
print(round(r2_score(y_test,y_test_pred_lasso),4))
0.5257
0.5563
0.5437
残差图
plt.figure(figsize=(9,6),dpi=600)
y_train_pred_ridge = ridge.predict(X_train[features_without_ones])
plt.scatter(y_train_pred_ridge,y_train_pred_ridge - y_train,c="g",alpha=0.6)
plt.scatter(y_test_pred_ridge,y_test_pred_ridge - y_test,c="r",alpha=0.6)
plt.hlines(y=0,xmin=0,xmax=30,color="b",alpha=0.6)
plt.ylabel("Residuals")
plt.xlabel("Predict")
Text(0.5, 0, 'Predict')
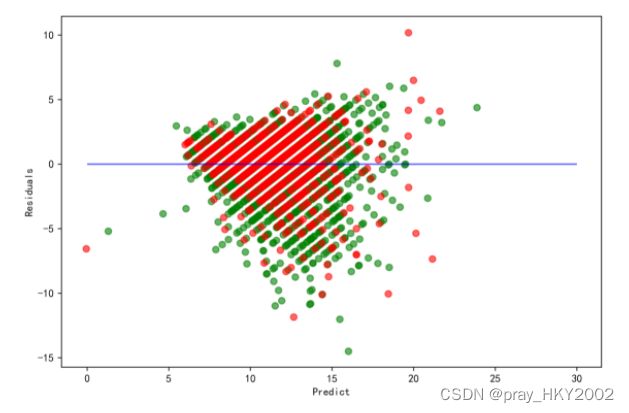