文章目录
- 1. 深度图像转点云
-
- 2. 点云转深度图
-
- 3. 无法打开输入文件“vtkViewsInfovisopencv_calib3d.lib”
1. 深度图像转点云
- 深度图转点云 并进行欧式聚类分割等处理
- 主要是将相机内参替换成自己的参数,图像路径改一改就行
#include
#include
#include
#include
#include
#include
#include
#include
#include
#include
#include
#include
#include
#include
#include
typedef pcl::PointXYZRGBA PointT;
typedef pcl::PointCloud<PointT> PointCloud;
pcl::search::KdTree<pcl::PointXYZRGBA>::Ptr tree(new pcl::search::KdTree<pcl::PointXYZRGBA>);
pcl::PointXYZRGBA p_min, p_max;
float colors[] = {
255, 0, 0,
0, 255, 0,
0, 0, 255,
255, 255, 0,
0, 255, 255,
255, 0, 255,
255, 255, 255,
255, 128, 0,
255, 153, 255,
51, 153, 255,
153, 102, 51,
128, 51, 153,
153, 153, 51,
163, 38, 51,
204, 153, 102,
204, 224, 255,
128, 179, 255,
206, 255, 0,
255, 204, 204,
204, 255, 153,
};
const double camera_factor = 1000;
const double camera_cx = 1595.4377;
const double camera_cy = 1001.2092;
const double camera_fx = 2407.4058;
const double camera_fy = 2409.0186;
void PassThrough(pcl::PointCloud<pcl::PointXYZRGBA>::Ptr incloud, pcl::PointCloud<pcl::PointXYZRGBA>::Ptr outcloud, float min, float max, int mode)
{
pcl::PassThrough<pcl::PointXYZRGBA> pass;
pass.setInputCloud(incloud);
switch (mode)
{
case 1:
pass.setFilterFieldName("x");
break;
case 2:
pass.setFilterFieldName("y");
break;
case 3:
pass.setFilterFieldName("z");
break;
}
pass.setFilterLimits(min, max);
pass.filter(*outcloud);
};
void StatisticalOutlierRemoval(pcl::PointCloud<pcl::PointXYZRGBA>::Ptr incloud, pcl::PointCloud<pcl::PointXYZRGBA>::Ptr outcloud, int k, double sigma)
{
pcl::StatisticalOutlierRemoval<pcl::PointXYZRGBA> sor;
sor.setInputCloud(incloud);
sor.setMeanK(k);
sor.setStddevMulThresh(sigma);
sor.filter(*outcloud);
}
void RadiusOutlierRemoval(pcl::PointCloud<pcl::PointXYZRGBA>::Ptr incloud, pcl::PointCloud<pcl::PointXYZRGBA>::Ptr outcloud, double r, int n)
{
pcl::RadiusOutlierRemoval<pcl::PointXYZRGBA> outrem;
outrem.setInputCloud(incloud);
outrem.setRadiusSearch(r);
outrem.setMinNeighborsInRadius(n);
outrem.filter(*outcloud);
}
void RANSAC(pcl::PointCloud<pcl::PointXYZRGBA>::Ptr incloud, pcl::PointCloud<pcl::PointXYZRGBA>::Ptr outcloud, pcl::PointIndices::Ptr inliers)
{
pcl::ModelCoefficients::Ptr coefficients(new pcl::ModelCoefficients);
pcl::SACSegmentation<pcl::PointXYZRGBA> seg;
seg.setOptimizeCoefficients(true);
seg.setModelType(pcl::SACMODEL_PLANE);
seg.setMethodType(pcl::SAC_RANSAC);
seg.setDistanceThreshold(0.1);
seg.setInputCloud(incloud);
seg.segment(*inliers, *coefficients);
pcl::copyPointCloud<pcl::PointXYZRGBA>(*incloud, *inliers, *outcloud);
}
void ExtractIndices(pcl::PointCloud<pcl::PointXYZRGBA>::Ptr incloud, pcl::PointCloud<pcl::PointXYZRGBA>::Ptr outcloud, pcl::PointIndices::Ptr index)
{
pcl::ExtractIndices<pcl::PointXYZRGBA> extract;
extract.setInputCloud(incloud);
extract.setIndices(index);
extract.setNegative(true);
extract.filter(*outcloud);
}
void EuclideanClusterExtraction(pcl::PointCloud<pcl::PointXYZRGBA>::Ptr incloud)
{
pcl::PCDWriter writer;
std::vector<pcl::PointIndices> cluster_indices;
pcl::EuclideanClusterExtraction<pcl::PointXYZRGBA> ec;
ec.setClusterTolerance(0.02);
ec.setMinClusterSize(99);
ec.setMaxClusterSize(999999);
ec.setSearchMethod(tree);
ec.setInputCloud(incloud);
ec.extract(cluster_indices);
pcl::visualization::PCLVisualizer::Ptr viewer(new pcl::visualization::PCLVisualizer("Point cloud processing"));
int v1(0);
viewer->createViewPort(0.0, 0.0, 0.5, 1.0, v1);
viewer->setBackgroundColor(0.1, 0.1, 0.1, v1);
viewer->addText("before", 10, 10, "v1 text", v1);
viewer->addPointCloud<pcl::PointXYZRGBA>(incloud, "cloud_in", v1);
viewer->setPointCloudRenderingProperties(pcl::visualization::PCL_VISUALIZER_POINT_SIZE, 2, "cloud_in", v1);
int v2(0);
viewer->createViewPort(0.5, 0.0, 1.0, 1.0, v2);
viewer->setBackgroundColor(0.3, 0.3, 0.3, v2);
viewer->addText("after", 10, 10, "v2 text", v2);
int j = 0;
for (std::vector<pcl::PointIndices>::const_iterator it = cluster_indices.begin(); it != cluster_indices.end(); ++it)
{
pcl::PointCloud<pcl::PointXYZRGBA>::Ptr cloud_cluster(new pcl::PointCloud<pcl::PointXYZRGBA>);
for (std::vector<int>::const_iterator pit = it->indices.begin(); pit != it->indices.end(); pit++)
{
cloud_cluster->points.push_back(incloud->points[*pit]);
}
cloud_cluster->width = cloud_cluster->points.size();
cloud_cluster->height = 1;
cloud_cluster->is_dense = true;
std::stringstream ss;
ss << "cloud_cluster_" << j << ".pcd";
writer.write<pcl::PointXYZRGBA>(ss.str(), *cloud_cluster, false);
cout << "-----" << ss.str() << "-----" << endl;
std::cout << "Segmentation" << cloud_cluster->size() << endl;
viewer->addPointCloud<pcl::PointXYZRGBA>(cloud_cluster, ss.str(), v2);
viewer->setPointCloudRenderingProperties(pcl::visualization::PCL_VISUALIZER_POINT_SIZE, 2, ss.str(), v2);
viewer->setPointCloudRenderingProperties(pcl::visualization::PCL_VISUALIZER_COLOR, colors[j * 3] / 255, colors[j * 3 + 1] / 255, colors[j * 3 + 2] / 255, ss.str(), v2);
j++;
}
viewer->setCameraPosition(-0.7, -0.4, -1, -0.7, -0.4, 1, 0, -1, 0);
while (!viewer->wasStopped())
{
viewer->spinOnce(100);
boost::this_thread::sleep(boost::posix_time::microseconds(100000));
}
}
void visualization(pcl::PointCloud<pcl::PointXYZRGBA>::Ptr cloud)
{
boost::shared_ptr<pcl::visualization::PCLVisualizer> viewer(new pcl::visualization::PCLVisualizer("cloud"));
viewer->addPointCloud<pcl::PointXYZRGBA>(cloud, "example");
viewer->setPointCloudRenderingProperties(pcl::visualization::PCL_VISUALIZER_POINT_SIZE, 2, "example");
viewer->resetCamera();
while (!viewer->wasStopped()) {
viewer->spinOnce(100);
boost::this_thread::sleep(boost::posix_time::microseconds(100000));
}
}
int main(int argc, char** argv)
{
cv::Mat rgb, depth;
rgb = cv::imread("E:/DataSet/lidar/translate/RGB.bmp");
depth = cv::imread("E:/DataSet/lidar/translate/D.tiff", -1);
PointCloud::Ptr cloud(new PointCloud);
PointCloud::Ptr cloud1(new PointCloud);
pcl::PointIndices::Ptr inliers(new pcl::PointIndices);
for (int m = 0; m < depth.rows; m++)
{
for (int n = 0; n < depth.cols; n++)
{
ushort d = depth.ptr<ushort>(m)[n];
if (d == 0)
continue;
PointT p;
p.z = double(d) / camera_factor;
p.x = (n - camera_cx) * p.z / camera_fx;
p.y = (m - camera_cy) * p.z / camera_fy;
p.b = rgb.ptr<uchar>(m)[n * 3];
p.g = rgb.ptr<uchar>(m)[n * 3 + 1];
p.r = rgb.ptr<uchar>(m)[n * 3 + 2];
cloud->points.push_back(p);
}
}
cloud->height = 1;
cloud->width = cloud->points.size();
pcl::getMinMax3D(*cloud, p_min, p_max);
std::cout << "points" << cloud->size() << endl;
std::cout << "minpoint" << p_min.x << "," << p_min.y << "," << p_min.z << endl;
std::cout << "maxpoint" << p_max.x << "," << p_max.y << "," << p_max.z << endl;
cloud->is_dense = false;
pcl::io::savePCDFile("pointcloud2.pcd", *cloud);
cloud->points.clear();
cout << "Point cloud saved." << endl;
pcl::io::loadPCDFile("pointcloud2.pcd", *cloud);
EuclideanClusterExtraction(cloud);
return 0;
}
1.1 效果图
2. 点云转深度图
#define _CRT_SECURE_NO_WARNINGS
#pragma warning(disable:4996)
#include
#include
#include
#include
#include
#include
#include
#include
#include
#include
#include
void
setViewerPose(pcl::visualization::PCLVisualizer& viewer, const Eigen::Affine3f& viewer_pose)
{
Eigen::Vector3f pos_vector = viewer_pose * Eigen::Vector3f(0, 0, 0);
Eigen::Vector3f look_at_vector = viewer_pose.rotation() * Eigen::Vector3f(0, 0, 1) + pos_vector;
Eigen::Vector3f up_vector = viewer_pose.rotation() * Eigen::Vector3f(0, -1, 0);
viewer.setCameraPosition(
pos_vector[0],
pos_vector[1],
pos_vector[2],
look_at_vector[0],
look_at_vector[1],
look_at_vector[2],
up_vector[0],
up_vector[1],
up_vector[2]);
}
#if 1
int
main(int argc, char** argv) {
pcl::PointCloud<pcl::PointXYZ>::Ptr cloud(new pcl::PointCloud<pcl::PointXYZ>);
pcl::io::loadPCDFile("pointcloud2.pcd", *cloud);
pcl::PointCloud<pcl::PointXYZ> pointCloud;
pointCloud = *cloud;
float angularResolution = (float)(1.0f * (M_PI / 180.0f));
float maxAngleWidth = (float)(360.0f * (M_PI / 180.0f));
float maxAngleHeight = (float)(180.0f * (M_PI / 180.0f));
Eigen::Affine3f sensorPose = (Eigen::Affine3f)Eigen::Translation3f(0.0f, 0.0f, 0.0f);
pcl::RangeImage::CoordinateFrame coordinate_frame = pcl::RangeImage::CAMERA_FRAME;
float noiseLevel = 0.00;
float minRange = 0.0f;
int borderSize = 1;
boost::shared_ptr<pcl::RangeImage> range_image_ptr(new pcl::RangeImage);
pcl::RangeImage& range_image = *range_image_ptr;
range_image.createFromPointCloud(pointCloud, angularResolution, maxAngleWidth, maxAngleHeight, sensorPose, coordinate_frame, noiseLevel, minRange, borderSize);
std::cout << range_image << "\n";
pcl::visualization::PCLVisualizer viewer("3D Viewer");
viewer.setBackgroundColor(0, 0, 0);
pcl::visualization::PointCloudColorHandlerCustom<pcl::PointWithRange> range_image_color_handler(range_image_ptr, 255, 255, 255);
viewer.addPointCloud(range_image_ptr, range_image_color_handler, "range image");
viewer.setPointCloudRenderingProperties(pcl::visualization::PCL_VISUALIZER_POINT_SIZE, 1, "range image");
viewer.resetCamera();
viewer.initCameraParameters();
setViewerPose(viewer, range_image.getTransformationToWorldSystem());
float* ranges = range_image.getRangesArray();
unsigned char* rgb_image = pcl::visualization::FloatImageUtils::getVisualImage(ranges, range_image.width, range_image.height);
pcl::io::saveRgbPNGFile("ha.png", rgb_image, range_image.width, range_image.height);
pcl::visualization::RangeImageVisualizer range_image_widget("Range image");
range_image_widget.showRangeImage(range_image);
Eigen::Affine3f scene_sensor_pose(Eigen::Affine3f::Identity());
while (!viewer.wasStopped())
{
range_image_widget.spinOnce();
viewer.spinOnce();
if (1)
{
scene_sensor_pose = viewer.getViewerPose();
range_image.createFromPointCloud(pointCloud,
angularResolution, pcl::deg2rad(360.0f),
pcl::deg2rad(180.0f),
scene_sensor_pose,
pcl::RangeImage::LASER_FRAME,
noiseLevel,
minRange,
borderSize);
range_image_widget.showRangeImage(range_image);
}
}
}
#endif
2.2 效果图
- 嗯生成的深度图非常的小

3. 无法打开输入文件“vtkViewsInfovisopencv_calib3d.lib”
- 之前同时配置PCL和opencv时就会报无法打开输入文件“vtkViewsInfovisopencv_calib3d.lib”这个错,每次都手动去链接器里删除这个lib,如下图。
- 今天我尝试着修改了下CMakeLists.txt才发现,之前是因为CMakeLists.txt中的TARGET_LINK_LIBRARIES里面的${PCL_LIBRARIES} ${OpenCV_LIBS}写的时候两个之间没有空格导致报错,加上空格就不会生成这个奇怪的lib和错误了。
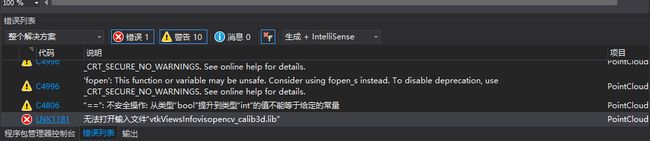