import tensorflow as tf
import tensorflow as tf
from tensorflow.keras import datasets,layers,models
import matplotlib.pyplot as plt
(train_images,train_labels),\
(test_images,test_labels)=datasets.mnist.load_data()
train_images,test_images = train_images/255.0,test_images/255.0
train_images.shape,test_images.shape,train_labels.shape,test_labels.shape
((60000, 28, 28), (10000, 28, 28), (60000,), (10000,))
plt.figure(figsize=(10,10))
for i in range(20):
plt.subplot(5,10,i+1)
plt.xticks([])
plt.yticks([])
plt.grid(False)
plt.imshow(train_images[i],cmap=plt.cm.binary)
plt.xlabel(train_labels[i])
plt.show()
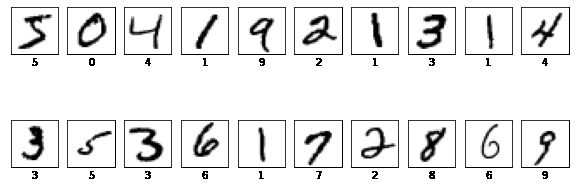
train_images = train_images.reshape((60000,28,28,1))
test_images = test_images.reshape((10000,28,28,1))
train_images.shape,test_images.shape,train_labels.shape,test_labels.shape
((60000, 28, 28, 1), (10000, 28, 28, 1), (60000,), (10000,))
model = models.Sequential([
layers.Conv2D(32,(3,3),activation='relu',input_shape=(28,28,1)),
layers.MaxPooling2D((2,2)),
layers.Conv2D(64,(3,3),activation='relu'),
layers.MaxPool2D((2,2)),
layers.Flatten(),
layers.Dense(64,activation='relu'),
layers.Dense(10)
])
model.summary()
Model: "sequential"
_________________________________________________________________
Layer (type) Output Shape Param #
=================================================================
conv2d (Conv2D) (None, 26, 26, 32) 320
_________________________________________________________________
max_pooling2d (MaxPooling2D) (None, 13, 13, 32) 0
_________________________________________________________________
conv2d_1 (Conv2D) (None, 11, 11, 64) 18496
_________________________________________________________________
max_pooling2d_1 (MaxPooling2 (None, 5, 5, 64) 0
_________________________________________________________________
flatten (Flatten) (None, 1600) 0
_________________________________________________________________
dense (Dense) (None, 64) 102464
_________________________________________________________________
dense_1 (Dense) (None, 10) 650
=================================================================
Total params: 121,930
Trainable params: 121,930
Non-trainable params: 0
_________________________________________________________________
2022-01-07 23:45:52.268745: I tensorflow/core/platform/cpu_feature_guard.cc:145] This TensorFlow binary is optimized with Intel(R) MKL-DNN to use the following CPU instructions in performance critical operations: SSE4.1 SSE4.2
To enable them in non-MKL-DNN operations, rebuild TensorFlow with the appropriate compiler flags.
2022-01-07 23:45:52.275905: I tensorflow/core/common_runtime/process_util.cc:115] Creating new thread pool with default inter op setting: 8. Tune using inter_op_parallelism_threads for best performance.
model.compile(optimizer='adam',
loss=tf.keras.losses.SparseCategoricalCrossentropy(from_logits=True),
metrics=['accuracy']
)
history = model.fit(train_images,
train_labels,
epochs=10,
validation_data=(test_images,test_labels)
)
Train on 60000 samples, validate on 10000 samples
Epoch 1/10
60000/60000 [==============================] - 138s 2ms/sample - loss: 0.1428 - accuracy: 0.9568 - val_loss: 0.0579 - val_accuracy: 0.9809
Epoch 2/10
60000/60000 [==============================] - 134s 2ms/sample - loss: 0.0456 - accuracy: 0.9861 - val_loss: 0.0388 - val_accuracy: 0.9877
Epoch 3/10
60000/60000 [==============================] - 132s 2ms/sample - loss: 0.0328 - accuracy: 0.9897 - val_loss: 0.0304 - val_accuracy: 0.9902
Epoch 4/10
60000/60000 [==============================] - 131s 2ms/sample - loss: 0.0232 - accuracy: 0.9922 - val_loss: 0.0301 - val_accuracy: 0.9897
Epoch 5/10
60000/60000 [==============================] - 134s 2ms/sample - loss: 0.0176 - accuracy: 0.9943 - val_loss: 0.0325 - val_accuracy: 0.9894
Epoch 6/10
60000/60000 [==============================] - 134s 2ms/sample - loss: 0.0135 - accuracy: 0.9956 - val_loss: 0.0307 - val_accuracy: 0.9907
Epoch 7/10
60000/60000 [==============================] - 134s 2ms/sample - loss: 0.0119 - accuracy: 0.9961 - val_loss: 0.0319 - val_accuracy: 0.9905
Epoch 8/10
60000/60000 [==============================] - 134s 2ms/sample - loss: 0.0086 - accuracy: 0.9973 - val_loss: 0.0327 - val_accuracy: 0.9905
Epoch 9/10
60000/60000 [==============================] - 132s 2ms/sample - loss: 0.0080 - accuracy: 0.9974 - val_loss: 0.0296 - val_accuracy: 0.9918
Epoch 10/10
60000/60000 [==============================] - 130s 2ms/sample - loss: 0.0065 - accuracy: 0.9979 - val_loss: 0.0314 - val_accuracy: 0.9911
plt.imshow(test_images[1])
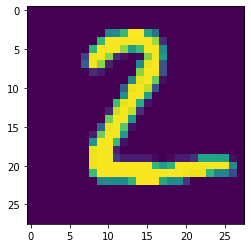
pre = model.predict(test_images)
pre[1]
array([-7.576601 , 0.24191436, 22.48119 , -7.913903 , -6.1873503 ,
-9.024768 , -0.274709 , -1.0671589 , 3.3178928 , -9.065157 ],
dtype=float32)
网络结构
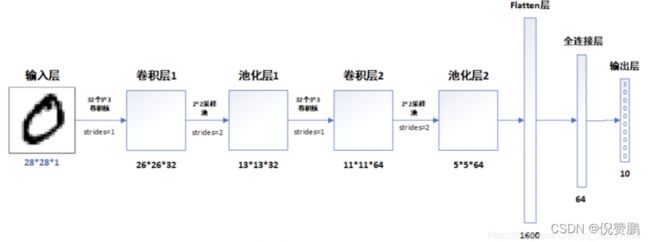
各层的作用
- 输入层:用于将数据输入到训练网络
- 卷积层:使用卷积核提取图片特征
- 池化层:进行下采样,用更高层的抽象表示图像特征
- Flatten层:将多维的输入一维化,常用在卷积层到全连接层的过渡
- 全连接层:起到“特征提取器”的作用
- 输出层:输出结果