import torchvision
import torchvision.transforms as transforms
mnist=torchvision.datasets.FashionMNIST(
root="//UsersDocuments/MINST-FASHION"
,download=False
,train=True
,transform=transforms.ToTensor()
)
mnist
Dataset FashionMNIST
Number of datapoints: 60000
Root location: //Users/Documents/MINST-FASHION
Split: Train
StandardTransform
Transform: ToTensor()
len(mnist)
60000
mnist.data
tensor([[[0, 0, 0, ..., 0, 0, 0],
[0, 0, 0, ..., 0, 0, 0],
[0, 0, 0, ..., 0, 0, 0],
...,
[0, 0, 0, ..., 0, 0, 0],
[0, 0, 0, ..., 0, 0, 0],
[0, 0, 0, ..., 0, 0, 0]],
[[0, 0, 0, ..., 0, 0, 0],
[0, 0, 0, ..., 0, 0, 0],
[0, 0, 0, ..., 0, 0, 0],
...,
[0, 0, 0, ..., 0, 0, 0],
[0, 0, 0, ..., 0, 0, 0],
[0, 0, 0, ..., 0, 0, 0]],
[[0, 0, 0, ..., 0, 0, 0],
[0, 0, 0, ..., 0, 0, 0],
[0, 0, 0, ..., 0, 0, 0],
...,
[0, 0, 0, ..., 0, 0, 0],
[0, 0, 0, ..., 0, 0, 0],
[0, 0, 0, ..., 0, 0, 0]],
...,
[[0, 0, 0, ..., 0, 0, 0],
[0, 0, 0, ..., 0, 0, 0],
[0, 0, 0, ..., 0, 0, 0],
...,
[0, 0, 0, ..., 0, 0, 0],
[0, 0, 0, ..., 0, 0, 0],
[0, 0, 0, ..., 0, 0, 0]],
[[0, 0, 0, ..., 0, 0, 0],
[0, 0, 0, ..., 0, 0, 0],
[0, 0, 0, ..., 0, 0, 0],
...,
[0, 0, 0, ..., 0, 0, 0],
[0, 0, 0, ..., 0, 0, 0],
[0, 0, 0, ..., 0, 0, 0]],
[[0, 0, 0, ..., 0, 0, 0],
[0, 0, 0, ..., 0, 0, 0],
[0, 0, 0, ..., 0, 0, 0],
...,
[0, 0, 0, ..., 0, 0, 0],
[0, 0, 0, ..., 0, 0, 0],
[0, 0, 0, ..., 0, 0, 0]]], dtype=torch.uint8)
mnist.data.shape
torch.Size([60000, 28, 28])
mnist.targets
tensor([9, 0, 0, ..., 3, 0, 5])
mnist.targets.unique()
tensor([0, 1, 2, 3, 4, 5, 6, 7, 8, 9])
mnist.classes
['T-shirt/top',
'Trouser',
'Pullover',
'Dress',
'Coat',
'Sandal',
'Shirt',
'Sneaker',
'Bag',
'Ankle boot']
import matplotlib.pyplot as plt
import numpy as np
plt.imshow(mnist[0][0].view(28,28).numpy())
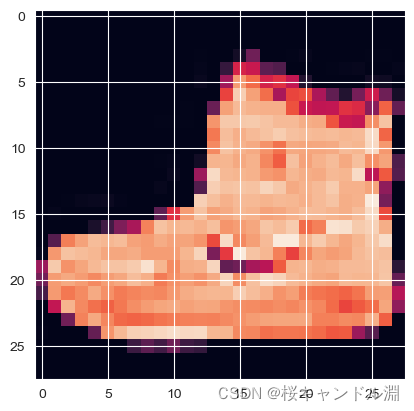
plt.imshow(mnist[1][0].view(28,28).numpy())
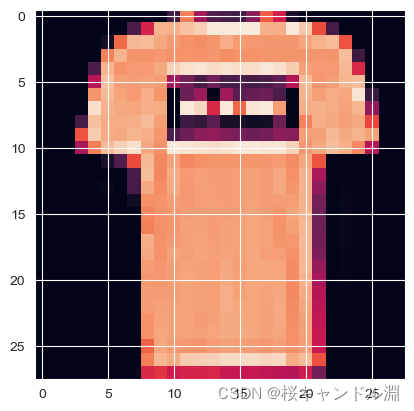
import torch
from torch import nn
from torch import optim
from torch.nn import functional as F
from torch.utils.data import DataLoader,TensorDataset
import torchvision
import torchvision.transforms as transforms
lr=0.15
gamma=0
epochs=10
bs=128
mnist=torchvision.datasets.FashionMNIST(
root="//Users/MINST-FASHION"
,download=False
,train=True
,transform=transforms.ToTensor()
)
batchdata=DataLoader(mnist,batch_size=bs,
shuffle=True)
for x,y in batchdata:
print(x.shape)
print(y.shape)
break
torch.Size([128, 1, 28, 28])
torch.Size([128])
input_=mnist.data[0].numel()
output_=len(mnist.targets.unique())
class Model(nn.Module):
def __init__(self,in_features=10,out_features=2):
super().__init__()
self.linear1=nn.Linear(in_features,128,bias=False)
self.output=nn.Linear(128,out_features,bias=False)
def forward(self,x):
x=x.view(-1,28*28)
sigma1=torch.relu(self.linear1(x))
sigma2=F.log_softmax(self.output(sigma1),dim=1)
return sigma2
def fit_(net,batchdata,lr=0.01,epochs=5,gamma=0):
criterion=nn.NLLLoss()
opt=optim.SGD(net.parameters(),lr=lr,momentum=gamma)
correct=0
samples=0
for epoch in range(epochs):
for batch_idx,(x,y) in enumerate(batchdata):
y=y.view(x.shape[0])
sigma=net.forward(x)
loss=criterion(sigma,y)
loss.backward()
opt.step()
opt.zero_grad()
yhat=torch.max(sigma,1)[1]
correct+=torch.sum(yhat==y)
samples+=x.shape[0]
if(batch_idx+1)%125==0 or batch_idx==len(batchdata)-1:
print("Epoch:{}:[{}/{}({:.0f})%],loss:{:.6f},accuracy:{:.3f}".format(
epoch+1,
samples,
epochs*len(batchdata.dataset),
100*samples/(epochs*len(batchdata.dataset)),
loss.data.item(),
float(100*correct/samples)))
torch.manual_seed(420)
net=Model(in_features=input_,out_features=output_)
fit_(net,batchdata,lr=lr,epochs=epochs,gamma=gamma)
Epoch:1:[16000/600000(3)%],loss:0.236640,accuracy:89.981
Epoch:1:[32000/600000(5)%],loss:0.356568,accuracy:89.750
Epoch:1:[48000/600000(8)%],loss:0.363261,accuracy:89.821
Epoch:1:[60000/600000(10)%],loss:0.276292,accuracy:89.833
Epoch:2:[76000/600000(13)%],loss:0.226447,accuracy:89.918
Epoch:2:[92000/600000(15)%],loss:0.264218,accuracy:89.930
Epoch:2:[108000/600000(18)%],loss:0.201081,accuracy:89.969
Epoch:2:[120000/600000(20)%],loss:0.293935,accuracy:89.957
Epoch:3:[136000/600000(23)%],loss:0.196868,accuracy:90.051
Epoch:3:[152000/600000(25)%],loss:0.285641,accuracy:90.057
Epoch:3:[168000/600000(28)%],loss:0.202996,accuracy:90.041
Epoch:3:[180000/600000(30)%],loss:0.315412,accuracy:90.051
Epoch:4:[196000/600000(33)%],loss:0.261344,accuracy:90.062
Epoch:4:[212000/600000(35)%],loss:0.415000,accuracy:90.080
Epoch:4:[228000/600000(38)%],loss:0.274316,accuracy:90.115
Epoch:4:[240000/600000(40)%],loss:0.326621,accuracy:90.126
Epoch:5:[256000/600000(43)%],loss:0.308148,accuracy:90.155
Epoch:5:[272000/600000(45)%],loss:0.243264,accuracy:90.185
Epoch:5:[288000/600000(48)%],loss:0.205354,accuracy:90.218
Epoch:5:[300000/600000(50)%],loss:0.241000,accuracy:90.222
Epoch:6:[316000/600000(53)%],loss:0.282183,accuracy:90.249
Epoch:6:[332000/600000(55)%],loss:0.231662,accuracy:90.274
Epoch:6:[348000/600000(58)%],loss:0.162190,accuracy:90.297
Epoch:6:[360000/600000(60)%],loss:0.283224,accuracy:90.301
Epoch:7:[376000/600000(63)%],loss:0.334327,accuracy:90.320
Epoch:7:[392000/600000(65)%],loss:0.270720,accuracy:90.357
Epoch:7:[408000/600000(68)%],loss:0.239996,accuracy:90.386
Epoch:7:[420000/600000(70)%],loss:0.379344,accuracy:90.386
Epoch:8:[436000/600000(73)%],loss:0.247614,accuracy:90.417
Epoch:8:[452000/600000(75)%],loss:0.234226,accuracy:90.429
Epoch:8:[468000/600000(78)%],loss:0.193927,accuracy:90.449
Epoch:8:[480000/600000(80)%],loss:0.216918,accuracy:90.461
Epoch:9:[496000/600000(83)%],loss:0.237355,accuracy:90.483
Epoch:9:[512000/600000(85)%],loss:0.254329,accuracy:90.498
Epoch:9:[528000/600000(88)%],loss:0.205053,accuracy:90.507
Epoch:9:[540000/600000(90)%],loss:0.151338,accuracy:90.527
Epoch:10:[556000/600000(93)%],loss:0.241480,accuracy:90.552
Epoch:10:[572000/600000(95)%],loss:0.267640,accuracy:90.581
Epoch:10:[588000/600000(98)%],loss:0.275014,accuracy:90.595
Epoch:10:[600000/600000(100)%],loss:0.249724,accuracy:90.601