##coding utf-8
# 导入基本数据计算库
import numpy as np
import pandas as pd
# 导入基本绘图库
import matplotlib.pyplot as plt
import seaborn as sns
## 我们利用 sklearn 中自带的breast_cancer数据作为数据载入,并利用Pandas转化为DataFrame格式
from sklearn.datasets import load_breast_cancer
data = load_breast_cancer() #得到数据特征
breast_target = data.target #得到数据对应的标签
breast_features = pd.DataFrame(data=data.data, columns=data.feature_names) #利用Pandas转化为DataFrame格式
print(breast_features)
## 进行简单的数据查看,我们可以利用 .head() 头部.tail()尾部
breast_features.head()
mean radius | mean texture | mean perimeter | mean area | mean smoothness | mean compactness | mean concavity | mean concave points | mean symmetry | mean fractal dimension | … | worst radius | worst texture | worst perimeter | worst area | worst smoothness | worst compactness | worst concavity | worst concave points | worst symmetry | worst fractal dimension | |
---|---|---|---|---|---|---|---|---|---|---|---|---|---|---|---|---|---|---|---|---|---|
0 | 17.99 | 10.38 | 122.80 | 1001.0 | 0.11840 | 0.27760 | 0.3001 | 0.14710 | 0.2419 | 0.07871 | … | 25.38 | 17.33 | 184.60 | 2019.0 | 0.1622 | 0.6656 | 0.7119 | 0.2654 | 0.4601 | 0.11890 |
1 | 20.57 | 17.77 | 132.90 | 1326.0 | 0.08474 | 0.07864 | 0.0869 | 0.07017 | 0.1812 | 0.05667 | … | 24.99 | 23.41 | 158.80 | 1956.0 | 0.1238 | 0.1866 | 0.2416 | 0.1860 | 0.2750 | 0.08902 |
2 | 19.69 | 21.25 | 130.00 | 1203.0 | 0.10960 | 0.15990 | 0.1974 | 0.12790 | 0.2069 | 0.05999 | … | 23.57 | 25.53 | 152.50 | 1709.0 | 0.1444 | 0.4245 | 0.4504 | 0.2430 | 0.3613 | 0.08758 |
3 | 11.42 | 20.38 | 77.58 | 386.1 | 0.14250 | 0.28390 | 0.2414 | 0.10520 | 0.2597 | 0.09744 | … | 14.91 | 26.50 | 98.87 | 567.7 | 0.2098 | 0.8663 | 0.6869 | 0.2575 | 0.6638 | 0.17300 |
4 | 20.29 | 14.34 | 135.10 | 1297.0 | 0.10030 | 0.13280 | 0.1980 | 0.10430 | 0.1809 | 0.05883 | … | 22.54 | 16.67 | 152.20 | 1575.0 | 0.1374 | 0.2050 | 0.4000 | 0.1625 | 0.2364 | 0.07678 |
5 rows × 30 columns
breast_features.tail()
mean radius | mean texture | mean perimeter | mean area | mean smoothness | mean compactness | mean concavity | mean concave points | mean symmetry | mean fractal dimension | … | worst radius | worst texture | worst perimeter | worst area | worst smoothness | worst compactness | worst concavity | worst concave points | worst symmetry | worst fractal dimension | |
---|---|---|---|---|---|---|---|---|---|---|---|---|---|---|---|---|---|---|---|---|---|
564 | 21.56 | 22.39 | 142.00 | 1479.0 | 0.11100 | 0.11590 | 0.24390 | 0.13890 | 0.1726 | 0.05623 | … | 25.450 | 26.40 | 166.10 | 2027.0 | 0.14100 | 0.21130 | 0.4107 | 0.2216 | 0.2060 | 0.07115 |
565 | 20.13 | 28.25 | 131.20 | 1261.0 | 0.09780 | 0.10340 | 0.14400 | 0.09791 | 0.1752 | 0.05533 | … | 23.690 | 38.25 | 155.00 | 1731.0 | 0.11660 | 0.19220 | 0.3215 | 0.1628 | 0.2572 | 0.06637 |
566 | 16.60 | 28.08 | 108.30 | 858.1 | 0.08455 | 0.10230 | 0.09251 | 0.05302 | 0.1590 | 0.05648 | … | 18.980 | 34.12 | 126.70 | 1124.0 | 0.11390 | 0.30940 | 0.3403 | 0.1418 | 0.2218 | 0.07820 |
567 | 20.60 | 29.33 | 140.10 | 1265.0 | 0.11780 | 0.27700 | 0.35140 | 0.15200 | 0.2397 | 0.07016 | … | 25.740 | 39.42 | 184.60 | 1821.0 | 0.16500 | 0.86810 | 0.9387 | 0.2650 | 0.4087 | 0.12400 |
568 | 7.76 | 24.54 | 47.92 | 181.0 | 0.05263 | 0.04362 | 0.00000 | 0.00000 | 0.1587 | 0.05884 | … | 9.456 | 30.37 | 59.16 | 268.6 | 0.08996 | 0.06444 | 0.0000 | 0.0000 | 0.2871 | 0.07039 |
5 rows × 30 columns
breast_target
array([0, 0, 0, 0, 0, 0, 0, 0, 0, 0, 0, 0, 0, 0, 0, 0, 0, 0, 0, 1, 1, 1,
0, 0, 0, 0, 0, 0, 0, 0, 0, 0, 0, 0, 0, 0, 0, 1, 0, 0, 0, 0, 0, 0,
0, 0, 1, 0, 1, 1, 1, 1, 1, 0, 0, 1, 0, 0, 1, 1, 1, 1, 0, 1, 0, 0,
1, 1, 1, 1, 0, 1, 0, 0, 1, 0, 1, 0, 0, 1, 1, 1, 0, 0, 1, 0, 0, 0,
1, 1, 1, 0, 1, 1, 0, 0, 1, 1, 1, 0, 0, 1, 1, 1, 1, 0, 1, 1, 0, 1,
1, 1, 1, 1, 1, 1, 1, 0, 0, 0, 1, 0, 0, 1, 1, 1, 0, 0, 1, 0, 1, 0,
0, 1, 0, 0, 1, 1, 0, 1, 1, 0, 1, 1, 1, 1, 0, 1, 1, 1, 1, 1, 1, 1,
1, 1, 0, 1, 1, 1, 1, 0, 0, 1, 0, 1, 1, 0, 0, 1, 1, 0, 0, 1, 1, 1,
1, 0, 1, 1, 0, 0, 0, 1, 0, 1, 0, 1, 1, 1, 0, 1, 1, 0, 0, 1, 0, 0,
0, 0, 1, 0, 0, 0, 1, 0, 1, 0, 1, 1, 0, 1, 0, 0, 0, 0, 1, 1, 0, 0,
1, 1, 1, 0, 1, 1, 1, 1, 1, 0, 0, 1, 1, 0, 1, 1, 0, 0, 1, 0, 1, 1,
1, 1, 0, 1, 1, 1, 1, 1, 0, 1, 0, 0, 0, 0, 0, 0, 0, 0, 0, 0, 0, 0,
0, 0, 1, 1, 1, 1, 1, 1, 0, 1, 0, 1, 1, 0, 1, 1, 0, 1, 0, 0, 1, 1,
1, 1, 1, 1, 1, 1, 1, 1, 1, 1, 1, 0, 1, 1, 0, 1, 0, 1, 1, 1, 1, 1,
1, 1, 1, 1, 1, 1, 1, 1, 1, 0, 1, 1, 1, 0, 1, 0, 1, 1, 1, 1, 0, 0,
0, 1, 1, 1, 1, 0, 1, 0, 1, 0, 1, 1, 1, 0, 1, 1, 1, 1, 1, 1, 1, 0,
0, 0, 1, 1, 1, 1, 1, 1, 1, 1, 1, 1, 1, 0, 0, 1, 0, 0, 0, 1, 0, 0,
1, 1, 1, 1, 1, 0, 1, 1, 1, 1, 1, 0, 1, 1, 1, 0, 1, 1, 0, 0, 1, 1,
1, 1, 1, 1, 0, 1, 1, 1, 1, 1, 1, 1, 0, 1, 1, 1, 1, 1, 0, 1, 1, 0,
1, 1, 1, 1, 1, 1, 1, 1, 1, 1, 1, 1, 0, 1, 0, 0, 1, 0, 1, 1, 1, 1,
1, 0, 1, 1, 0, 1, 0, 1, 1, 0, 1, 0, 1, 1, 1, 1, 1, 1, 1, 1, 0, 0,
1, 1, 1, 1, 1, 1, 0, 1, 1, 1, 1, 1, 1, 1, 1, 1, 1, 0, 1, 1, 1, 1,
1, 1, 1, 0, 1, 0, 1, 1, 0, 1, 1, 1, 1, 1, 0, 0, 1, 0, 1, 0, 1, 1,
1, 1, 1, 0, 1, 1, 0, 1, 0, 1, 0, 0, 1, 1, 1, 0, 1, 1, 1, 1, 1, 1,
1, 1, 1, 1, 1, 0, 1, 0, 0, 1, 1, 1, 1, 1, 1, 1, 1, 1, 1, 1, 1, 1,
1, 1, 1, 1, 1, 1, 1, 1, 1, 1, 1, 1, 0, 0, 0, 0, 0, 0, 1])
## 合并标签和特征信息
breast_all = breast_features.copy() ##进行浅拷贝,防止对于原始数据的修改
breast_all['target'] = breast_target
for col in breast_features.columns:
sns.boxplot(x='target', y=col, saturation=0.5,palette='pastel', data=breast_all)
plt.title(col)
plt.show()
# 选取其前三个特征绘制三维散点图
from mpl_toolkits.mplot3d import Axes3D
fig = plt.figure(figsize=(10,8))
ax = fig.add_subplot(111, projection='3d')
breast_all_class0 = breast_all[breast_all['target']==0].values
breast_all_class1 = breast_all[breast_all['target']==1].values
#
ax.scatter(breast_all_class0[:,0], breast_all_class0[:,1], breast_all_class0[:,2],label='malignancy')
ax.scatter(breast_all_class1[:,0], breast_all_class1[:,1], breast_all_class1[:,2],label='benign')
plt.legend()# 设置图例
plt.show()
## 为了正确评估模型性能,将数据划分为训练集和测试集,并在训练集上训练模型,在测试集上验证模型性能。
from sklearn.model_selection import train_test_split
## 选择其类别为0和1的样本
breast_features_part = breast_features.iloc[:400]
breast_target_part = breast_target[:400]
## 测试集大小为20%, 80%/20%分
x_train, x_test, y_train, y_test = train_test_split(breast_features_part, breast_target_part, test_size = 0.2, random_state = 2020)
## 从sklearn中导入逻辑回归模型
from sklearn.linear_model import LogisticRegression
## 查看其对应的w
print('the weight of Logistic Regression:',clf.coef_)
## 查看其对应的w0
print('the intercept(w0) of Logistic Regression:',clf.intercept_)
the weight of Logistic Regression: [[ 1.58968483 0.00505748 0.38837107 -0.02644605 -0.06394019 -0.28711873
-0.37853229 -0.16487063 -0.08104816 -0.02073884 0.03589982 0.74089555
0.319864 -0.11417749 -0.00675931 -0.05530251 -0.07017069 -0.01922167
-0.03401847 -0.00483318 1.43728027 -0.293294 -0.44437706 -0.00940775
-0.1151745 -0.90699784 -1.02800043 -0.31155816 -0.31756381 -0.0902991 ]]
the intercept(w0) of Logistic Regression: [0.27969267]
## 在训练集和测试集上分布利用训练好的模型进行预测
train_predict = clf.predict(x_train)
test_predict = clf.predict(x_test)
from sklearn import metrics
## 利用accuracy(准确度)【预测正确的样本数目占总预测样本数目的比例】评估模型效果
print('The accuracy of the Logistic Regression is:',metrics.accuracy_score(y_train,train_predict))
print('The accuracy of the Logistic Regression is:',metrics.accuracy_score(y_test,test_predict))
## 查看混淆矩阵 (预测值和真实值的各类情况统计矩阵)
confusion_matrix_result = metrics.confusion_matrix(test_predict,y_test)
print('The confusion matrix result:\n',confusion_matrix_result)
# 利用热力图对于结果进行可视化
plt.figure(figsize=(8, 6))
sns.heatmap(confusion_matrix_result, annot=True, cmap='Blues')
plt.xlabel('Predicted labels')
plt.ylabel('True labels')
plt.show()
## 在训练集和测试集上分布利用训练好的模型进行预测
train_predict = clf.predict(x_train)
test_predict = clf.predict(x_test)
## 由于逻辑回归模型是概率预测模型(p = p(y=1|x,\theta)),所有我们可以利用 predict_proba 函数预测其概率
train_predict_proba = clf.predict_proba(x_train)
test_predict_proba = clf.predict_proba(x_test)
print('The test predict Probability of each class:\n',test_predict_proba)
## 其中第一列代表预测为0类的概率,第二列代表预测为1类的概率
## 利用accuracy(准确度)【预测正确的样本数目占总预测样本数目的比例】评估模型效果
print('The accuracy of the Logistic Regression is:',metrics.accuracy_score(y_train,train_predict))
The test predict Probability of each class:
[[1.00000000e+00 1.74550212e-20]
[1.39048275e-01 8.60951725e-01]
[5.93137883e-03 9.94068621e-01]
[9.99928649e-01 7.13512878e-05]
[1.10598806e-02 9.88940119e-01]
[1.51763229e-02 9.84823677e-01]
[9.99999976e-01 2.40399407e-08]
[6.62445901e-01 3.37554099e-01]
[9.99998635e-01 1.36531478e-06]
[9.99999937e-01 6.26178303e-08]
[6.90866554e-03 9.93091334e-01]
[5.05999535e-01 4.94000465e-01]
[9.99286913e-01 7.13086781e-04]
[1.76610793e-03 9.98233892e-01]
[2.80400461e-02 9.71959954e-01]
[1.24867157e-02 9.87513284e-01]
[2.83215334e-02 9.71678467e-01]
[1.15554505e-01 8.84445495e-01]
[1.00000000e+00 2.80618965e-19]
[9.53877184e-01 4.61228156e-02]
[9.99981034e-01 1.89663222e-05]
[9.99999998e-01 1.77364899e-09]
[9.99784834e-01 2.15165875e-04]
[9.49142700e-04 9.99050857e-01]
[1.64447085e-02 9.83555291e-01]
[2.33740738e-01 7.66259262e-01]
[6.99567302e-01 3.00432698e-01]
[3.04170042e-01 6.95829958e-01]
[1.44626331e-01 8.55373669e-01]
[1.00000000e+00 5.02507240e-26]
[9.97623824e-01 2.37617614e-03]
[2.58153192e-01 7.41846808e-01]
[3.06528727e-02 9.69347127e-01]
[2.36651118e-03 9.97633489e-01]
[9.99999630e-01 3.70006531e-07]
[1.38031809e-02 9.86196819e-01]
[8.14914321e-01 1.85085679e-01]
[9.99964597e-01 3.54026953e-05]
[2.63450870e-03 9.97365491e-01]
[1.00392014e-03 9.98996080e-01]
[8.71662147e-01 1.28337853e-01]
[4.38761196e-03 9.95612388e-01]
[8.36873412e-03 9.91631266e-01]
[1.06161420e-02 9.89383858e-01]
[9.99999997e-01 3.31516428e-09]
[9.94734026e-01 5.26597408e-03]
[5.13494043e-03 9.94865060e-01]
[9.99999939e-01 6.09370477e-08]
[9.99999998e-01 2.05445256e-09]
[2.46967502e-02 9.75303250e-01]
[1.00000000e+00 9.38730719e-11]
[1.77617121e-02 9.82238288e-01]
[1.60523832e-02 9.83947617e-01]
[9.99987835e-01 1.21654996e-05]
[9.82051340e-01 1.79486596e-02]
[4.18451580e-03 9.95815484e-01]
[1.00000000e+00 1.09319254e-11]
[6.97450777e-05 9.99930255e-01]
[1.37259706e-01 8.62740294e-01]
[1.00000000e+00 2.49456197e-12]
[7.97894321e-02 9.20210568e-01]
[5.37893016e-03 9.94621070e-01]
[1.96820584e-02 9.80317942e-01]
[1.17207761e-01 8.82792239e-01]
[7.35320492e-02 9.26467951e-01]
[9.99673213e-01 3.26787395e-04]
[2.57482071e-02 9.74251793e-01]
[1.71958276e-03 9.98280417e-01]
[9.97528713e-01 2.47128671e-03]
[9.80976661e-01 1.90233388e-02]
[6.21099876e-03 9.93789001e-01]
[8.15132942e-03 9.91848671e-01]
[9.23436304e-01 7.65636959e-02]
[4.19986782e-03 9.95800132e-01]
[1.27804652e-03 9.98721953e-01]
[1.87243107e-03 9.98127569e-01]
[1.85282178e-01 8.14717822e-01]
[6.44246823e-02 9.35575318e-01]
[9.99998455e-01 1.54502928e-06]
[6.62652442e-03 9.93373476e-01]
[8.57651190e-01 1.42348810e-01]
[7.16548957e-01 2.83451043e-01]
[3.06459764e-03 9.96935402e-01]
[9.96314395e-01 3.68560463e-03]
[1.47485316e-01 8.52514684e-01]
[9.99999787e-01 2.12826060e-07]
[1.18626317e-02 9.88137368e-01]
[1.57873994e-01 8.42126006e-01]
[3.78928093e-03 9.96210719e-01]
[1.29844105e-02 9.87015589e-01]
[9.99793895e-01 2.06105131e-04]
[9.99066816e-01 9.33183505e-04]
[9.29672666e-01 7.03273335e-02]
[3.54993590e-02 9.64500641e-01]
[9.99998018e-01 1.98162222e-06]
[1.40153070e-02 9.85984693e-01]
[1.13682784e-02 9.88631722e-01]
[9.99999974e-01 2.62916142e-08]
[1.00000000e+00 9.16315813e-13]
[5.19135133e-03 9.94808649e-01]
[1.85001642e-02 9.81499836e-01]
[2.35600902e-02 9.76439910e-01]
[5.37148641e-02 9.46285136e-01]
[6.41665354e-02 9.35833465e-01]
[7.27446091e-04 9.99272554e-01]
[7.95455974e-04 9.99204544e-01]
[2.91957313e-03 9.97080427e-01]
[8.42552515e-04 9.99157447e-01]
[9.28348894e-02 9.07165111e-01]
[9.98976712e-01 1.02328806e-03]
[9.99710759e-01 2.89240705e-04]
[1.00000000e+00 4.26245383e-16]
[9.98616497e-01 1.38350344e-03]
[9.65916735e-04 9.99034083e-01]]
The accuracy of the Logistic Regression is: 0.9516483516483516
```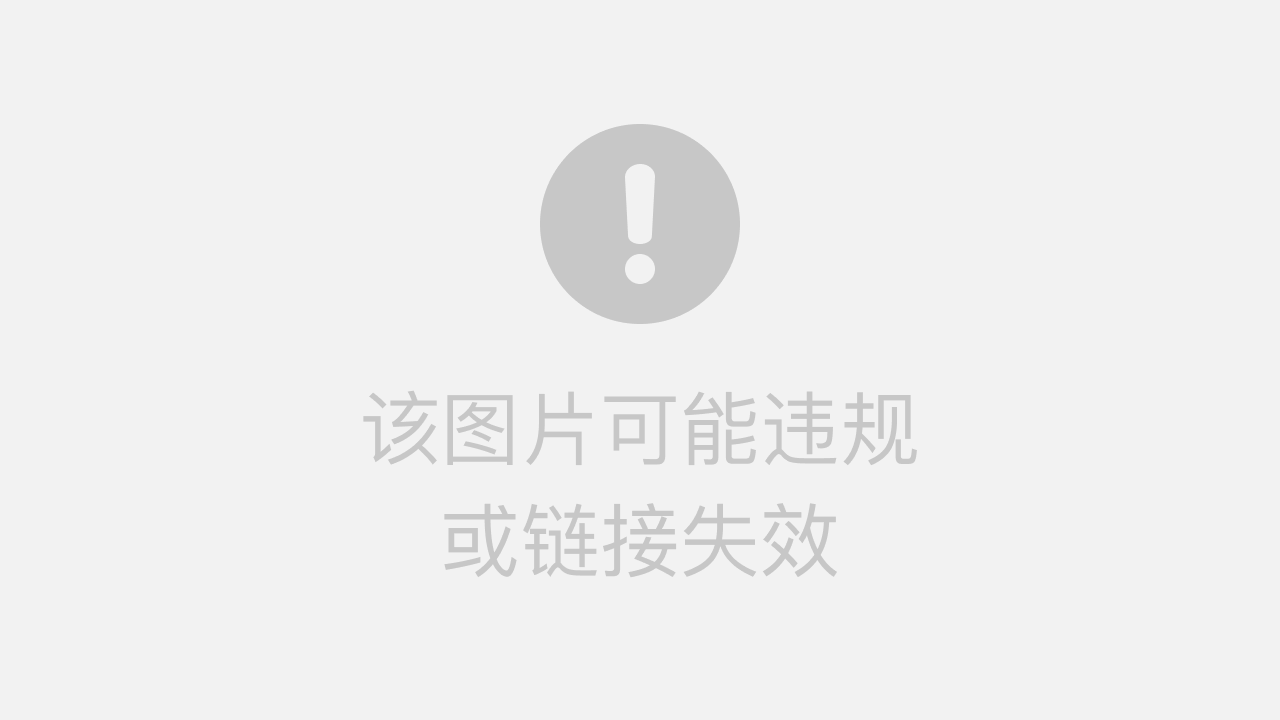
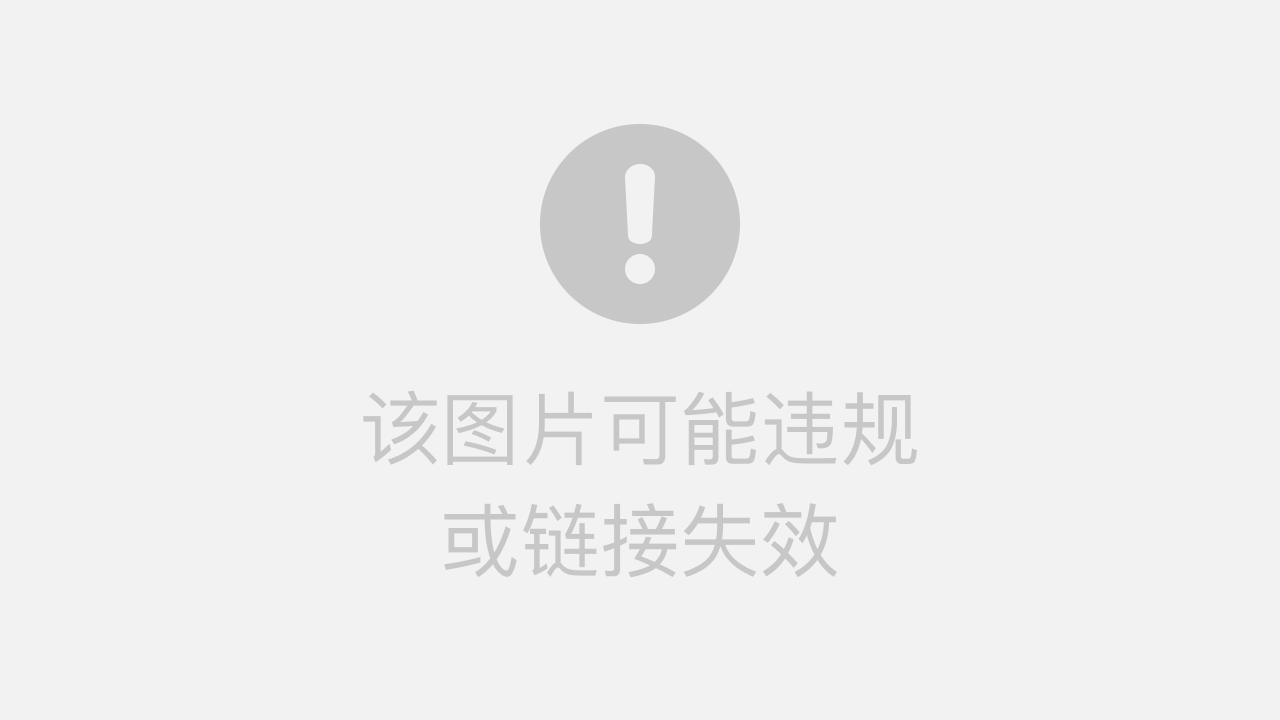