近期学习了一下天池中o2o优惠券使用预测的学习赛,主要任务是通过分析建模,精准预测用户是否会在规定时间内使用相应优惠券。这次的参与主要是学习为主,牛刀小试。
1、导入相关的库
#导入常规用的库
import numpy as np
import pandas as pd
from datetime import date #日期
#数据集划分相关库
from sklearn.model_selection import KFold, train_test_split,StratifiedKFold, cross_val_score,GridSearchCV
#管道输入函数,自定义模型
from sklearn.pipeline import Pipeline
#SGD随机梯度下降分类器以及逻辑回归
from sklearn.linear_model import SGDClassifier, LogisticRegression
#数据预处理库,归一化处理
from sklearn.preprocessing import StandardScaler
#评价指标
from sklearn.metrics import log_loss, roc_auc_score, auc, roc_curve
from sklearn.preprocessing import MinMaxScaler
%matplotlib inline
%config InlineBackend.figure_format = 'retina' #高清图嵌入
2、导入数据集并观察分析
dfoff = pd.read_csv(r'E:\天池020优惠券使用预测\Code\data\ccf_offline_stage1_train.csv',keep_default_na=False).iloc[:,0:7]
dfon = pd.read_csv(r'E:\天池020优惠券使用预测\Code\data\ccf_online_stage1_train.csv',keep_default_na=False)
dftest = pd.read_csv(r'E:\天池020优惠券使用预测\Code\data\ccf_offline_stage1_test_revised.csv',keep_default_na=False
print('有优惠券,购买商品: %d'% dfoff.loc[(dfoff['Date_received']!='null')&(dfoff['Date']!='null')].shape[0])
print('有优惠券,未购买商品: %d'% dfoff.loc[(dfoff['Date_received']!='null')&(dfoff['Date']=='null')].shape[0])
print('无优惠券,购买商品: %d'% dfoff.loc[(dfoff['Date_received']=='null')&(dfoff['Date']!='null')].shape[0])
print('无优惠券,未购买商品: %d'% dfoff.loc[(dfoff['Date_received']=='null')&(dfoff['Date']=='null')].shape[0])
可以看出无优惠券但是也购买的商品的客户还是很多的,为了更加精准的把优惠券发放到会购买商品的客户手里,我们需要建立预测模型进行预测。
3、打折率(Discount_rate)特征处理
从上述显示的数据集来看,除了ID外先看第一个有用的特征,打折率,我们都知道如果商品打折得多购买的欲望就更强,因此我们应该好好分析一下打折率。
首先对打折率这个特征的值进行统计观察:
dfoff['Discount_rate'].unique()
发现打折率的值主要分为以下4中情况:
(1)没有打折,null值
(2)0~1范围,表示直接打折
(3)满多少减多少
(4)干扰值
我们可以先把干扰值所在处的样本去除,干扰值为’2418’和’7751’
#除去干扰值所在样本
dfoff = dfoff[(dfoff['Discount_rate']!='2418') & (dfoff['Discount_rate']!='7751') ]
dfoff['Discount_rate'].value_counts()
数据预处理完都,对打折率特征进行分析处理:
#区分打折函数,没打折返回null,满减返回1,直接打折返回0
def getDiscountType(row):
if row == 'null':
return 'null'
elif ':' in row:
return 1
else:
return 0
#折扣率转化,没打折的原价返回1,满减的计算出打折率返回,直接打折的返回原值
def convertRate(row):
if row == 'null':
return 1.0
elif ':' in row:
rows = row.split(':')
return 1.0 - float(rows[1])/float(rows[0])
else:
return row
#满多少特征提取
def getDiscountMan(row):
if ':' in row:
rows = row.split(':')
return rows[0]
else:
return 0
#减多少特征提取
def getDiscountJian(row):
if ':' in row:
rows = row.split(':')
return rows[1]
else:
return 0
#数据预处理,调用以上函数,进行封装
def processData(df):
df['discount_type'] = df['Discount_rate'].apply(getDiscountType)
df['discount_rate'] = df['Discount_rate'].apply(convertRate)
df['discount_man'] = df['Discount_rate'].apply(getDiscountMan)
df['discount_jian'] = df['Discount_rate'].apply(getDiscountJian)
return df
以上打折函数编写完毕后,输入要分析处理的数据集进行打折率特征处理:
dfoff = processData(dfoff)
dftest = processData(dftest)
dfoff.head()
#观察Distance的类型
dfoff['Distance'].unique()
dfoff['Distance'] = dfoff['Distance'].replace('null', -1).astype(int)
dftest['Distance'] = dftest['Distance'].replace('null', -1).astype(int)
5、领券日期(Date_received)
dfoff['Date_received'].unique()
weekday : {null, 1, 2, 3, 4, 5, 6, 7}
weekday_type : {1, 0}(周六和周日为1,其他为0)
Weekday_1 : {1, 0, 0, 0, 0, 0, 0}
Weekday_2 : {0, 1, 0, 0, 0, 0, 0}
Weekday_3 : {0, 0, 1, 0, 0, 0, 0}
Weekday_4 : {0, 0, 0, 1, 0, 0, 0}
Weekday_5 : {0, 0, 0, 0, 1, 0, 0}
Weekday_6 : {0, 0, 0, 0, 0, 1, 0}
Weekday_7 : {0, 0, 0, 0, 0, 0, 1}
(1)提取星期特征
def getWeekday(row):
if row == 'null':
return row
else:
return date(int(row[0:4]),int(row[4:6]),int(row[6:8])).weekday() + 1
dfoff['weekday'] = dfoff['Date_received'].astype(str).apply( getWeekday)
dftest['weekday'] = dftest['Date_received'].astype(str).apply( getWeekday)
dfoff.head()
#日期类型,周末返回1,工作日返回0
dfoff['weekday_type'] = dfoff['weekday'].apply(lambda x: 1 if x in [6,7] else 0)
dftest['weekday_type'] = dftest['weekday'].apply(lambda x: 1 if x in [6,7] else 0)
#回复索引
dfoff.index = range(dfoff.shape[0])
dftest.index = range(dftest.shape[0])
(3)把星期特征进行独热编码
weekdaycols = ['weekday_' + str(i) for i in range(1,8)]
tmpdf = pd.get_dummies(dfoff['weekday'].replace('null', np.nan))
tmpdf.columns = weekdaycols
dfoff[weekdaycols] = tmpdf
tmpdf = pd.get_dummies(dftest['weekday'].replace('null', np.nan))
tmpdf.columns = weekdaycols
dftest[weekdaycols] = tmpdf
6、标签提取
标签主要分为三种情况:
Date_received == ‘null’:表示没有领到优惠券,无需考虑,y = -1
(Date_received != ‘null’) & (Date != ‘null’) & (Date - Date_received <= 15):表示领取优惠券且在15天内使用,即正样本,y = 1
(Date_received != ‘null’) & ((Date == ‘null’) | (Date - Date_received > 15)):表示领取优惠券未在在15天内使用,即负样本,y = 0
pd.options.display.max_columns = None #解除列限制
#定义标签备注函数
def label(row):
if row['Date_received'] == 'null':
return -1
elif row['Date'] != 'null':
td = pd.to_datetime(row['Date'],format='%Y%m%d') - pd.to_datetime(row['Date_received'],format='%Y%m%d')
if td <= pd.Timedelta(15,'D'):
return 1
return 0
dfoff['label'] = dfoff.apply(label, axis=1)
7、建立线性模型 SGDClassifier
使用上面提取的14个特征,进行模型建立。
训练集:20160101-20160515;验证集:20160516-20160615。
用线性模型 SGDClassifier
使用上面提取的14个特征。
训练集:20160101-20160515;验证集:20160516-20160615。
用线性模型 SGDClassifier
(1)数据集划分
df = dfoff[dfoff['label']!=-1].copy()
train = df[df['Date_received'] < '20160516'].copy()
valid = df[df['Date_received'] >= '20160516'].copy()
(2)观察是否具有样本不平衡
#观察是否具有样本不平衡问题
train['label'].value_counts()
original_feature = ['discount_rate','discount_type','discount_man', 'discount_jian','Distance', 'weekday_type'] + weekdaycols
original_feature
def check_model(data,predictors):
classifier =lambda: SGDClassifier(
loss='log'#逻辑损失
,penalty='elasticnet'
,fit_intercept=True
,max_iter=100
,shuffle=True
,n_jobs=1
,class_weight=None)
# 管道机制使得参数集在新数据集(比如测试集)上的重复使用,管道机制实现了对全部步骤的流式化封装和管理。
model = Pipeline(steps=[
('ss', StandardScaler()), # transformer
('en', classifier()) # estimator
])
parameters = {
'en__alpha': [ 0.001, 0.01, 0.1],
'en__l1_ratio': [ 0.001, 0.01, 0.1]
}
# StratifiedKFold用法类似Kfold,但是他是分层采样,确保训练集,测试集中各类别样本的比例与原始数据集中相同。
folder = StratifiedKFold(n_splits=3, shuffle=True)
grid_search = GridSearchCV(
model,
parameters,
cv=folder,
n_jobs=-1, # -1 means using all processors
verbose=1)
grid_search = grid_search.fit(data[predictors],
data['label'])
return grid_search
模型训练:
predictors = original_feature
model = check_model(train, predictors)
8、验证
对验证集中每种优惠券预测的结果计算 AUC,再对所有优惠券的 AUC 求平均。计算 AUC 的时候,如果 label 只有一类,就直接跳过,因为 AUC 无法计算。
#第一列分类为0的概率,第二类分类为1的概率
y_valid_pred = model.predict_proba(valid[predictors])
y_valid_pred
```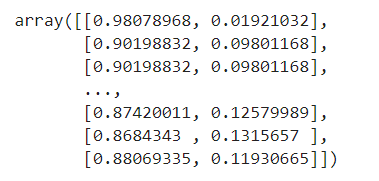
提取正类样本
```csharp
valid1 = valid.copy()
valid1['pred_prob'] = y_valid_pred[:,1]
valid1.head()
AUC面积计算
# 以优惠券种类数进行分局,计算出每种优惠券的AUC,并最后计算所有AUC的平均值
vg = valid1.groupby(['Coupon_id'])
aucs = []
for i in vg:
tmpdf = i[1]
if len(tmpdf['label'].unique()) != 2:
continue
fpr, tpr, thresholds = roc_curve(tmpdf['label'], tmpdf['pred_prob'], pos_label=1)
aucs.append(auc(fpr, tpr))
print(np.average(aucs))
9、测试
使用测试进行测试,并把结果保存为天池比赛中的结果形式
y_test_pred = model.predict_proba(dftest[predictors])
dftest1 = dftest[['User_id','Coupon_id','Date_received']].copy()
dftest1['Probability'] = y_test_pred[:,1]
dftest1.to_csv(r'E:\天池020优惠券使用预测\Code\data\submit1.csv',index=False, header=False)
dftest1.head(5)
import os
import pickle
if not os.path.isfile('1_model.pkl'):
with open(r'E:\天池020优惠券使用预测\Code\1_model.pkl', 'wb') as f:
pickle.dump(model, f)
else:
with open(r'E:\天池020优惠券使用预测\Code\1_model.pkl', 'rb') as f:
model = pickle.load(f)
从成绩来看效果欠佳,但笔者是本着学习的心态进行参与,后续会在这个基础上尝试使用其他一些更加出色的模型比如:XGboost、随机森林等模型进行尝试。