在面对特定的下游任务时,如果进行Full FineTuning(即对预训练模型中的所有参数都进行微调),太过低效;而如果采用固定预训练模型的某些层,只微调接近下游任务的那几层参数,又难以达到较好的效果。
PEFT(Parameter-Efficient Fine-Tuning)是一个用于在不微调所有模型参数的情况下,有效地将预先训练的语言模型(PLM)适应各种下游应用的库。PEFT方法只微调少量(额外)模型参数,显著降低了计算和存储成本,因为微调大规模PLM的成本高得令人望而却步。最近最先进的PEFT技术实现了与完全微调相当的性能。
大模型训练的微调方法,包括prompt tuning、prefix tuning、LoRA、p-tuning和AdaLoRA等。
谷歌的研究人员首次在论文《Parameter-Efficient Transfer Learning for NLP》提出针对 BERT 的 PEFT 微调方式,拉开了 PEFT 研究的序幕。下图所示的 Adapter 结构,将其嵌入 Transformer 的结构里面,在训练时,固定住原来预训练模型的参数不变,只对新增的 Adapter 结构进行微调。
首先是一个 down-project 层将高维度特征映射到低维特征,然后过一个非线形层之后,再用一个 up-project 结构将低维特征映射回原来的高维特征;同时也设计了 skip-connection 结构,确保了在最差的情况下能够退化为 identity。
从实验结果来看,该方法能够在只额外对增加的 3.6% 参数规模(相比原来预训练模型的参数量)的情况下取得和 Full-finetuning 接近的效果(GLUE 指标在 0.4% 以内)。
在输入 token 之前构造一段任务相关的 virtual tokens 作为 Prefix,然后训练的时候只更新 Prefix 部分的参数,而 Transformer 中的其他部分参数固定。,学习到的前缀指令文本向量可以挖掘大模型的潜力去引导模型完成特定任务。
Prefix Tuning 和构造 Prompt 类似,只是 Prompt 是人为构造的“显式”的提示,并且无法更新参数,而 Prefix 则是可以学习的“隐式”的提示。
同时,为了防止直接更新 Prefix 的参数导致训练不稳定的情况,在 Prefix 层前面加了 MLP 结构(相当于将 Prefix 分解为更小维度的 Input 与 MLP 的组合后输出的结果),训练完成后,只保留 Prefix 的参数。
embedding = torch.nn.Embedding(num_virtual_tokens, token_dim)
transform = torch.nn.Sequential(
torch.nn.Linear(token_dim, encoder_hidden_size),
torch.nn.Tanh(),
torch.nn.Linear(encoder_hidden_size, num_layers * 2 * token_dim),
)
PREFIX_TUNING 需要设置一个参数,即 指令文本的长度 num_virtual_tokens,研究表明这个数量一般设置在10-20之间。不同的任务所需要的 Prefix 的长度有差异。
```python
model_name_or_path = "./unsup-simcse-roberta-base"
peft_type = PeftType.PREFIX_TUNING
peft_config = PrefixTuningConfig(task_type="SEQ_CLS", num_virtual_tokens=20)
lr = 1e-2
model = AutoModelForSequenceClassification.from_pretrained(model_name_or_path, return_dict=True)
model = get_peft_model(model, peft_config)
model.print_trainable_parameters()
论文《The Power of Scale for Parameter-Efficient Prompt Tuning》
只要模型规模够大,简单加入 Prompt tokens 进行微调,就能取得很好的效果。
固定预训练参数,为每一个任务额外添加一个或多个embedding,之后拼接query正常输入LLM,并只训练这些embedding。左图为单任务全参数微调,右图为prompt tuning。
文章表明随着模型越大,PROMPT_TUNING 的效果几乎能和模型整个微调的效果一样。形成了只是学习一个指令然后去模型中检索某种能力的范式。
from peft import PromptTuningConfig, get_peft_model
model_name_or_path = "./unsup-simcse-roberta-base"
peft_type = PeftType.PROMPT_TUNING
peft_config = PromptTuningConfig(task_type="SEQ_CLS", num_virtual_tokens=20)
lr = 1e-3
model = AutoModelForSequenceClassification.from_pretrained(model_name_or_path, return_dict=True)
model = get_peft_model(model, peft_config)
model.print_trainable_parameters()
https://huggingface.co/docs/peft/conceptual_guides/prompting
P-Tuning 方法的提出主要是为了解决这样一个问题:大模型的 Prompt 构造方式严重影响下游任务的效果。
P-Tuning 提出将 Prompt 转换为可以学习的 Embedding 层,只是考虑到直接对 Embedding 参数进行优化会存在这样两个挑战:
作者在这里提出用 MLP+LSTM 的方式来对 prompt embedding 进行一层处理
需要提醒的是这里的指令文本是伪文本,可能就是 unused1, unused2…等,目前源代码里面就是随机初始化的一个embeding.
self.lstm_head = torch.nn.LSTM(
input_size=self.input_size,
hidden_size=self.hidden_size,
num_layers=num_layers,
dropout=lstm_dropout,
bidirectional=True,
batch_first=True,
)
self.mlp_head = torch.nn.Sequential(
torch.nn.Linear(self.hidden_size * 2, self.hidden_size * 2),
torch.nn.ReLU(),
torch.nn.Linear(self.hidden_size * 2, self.output_size),
)
self.mlp_head(self.lstm_head(input_embeds)[0])
以上代码可清晰展示出prompt encoder的结构。
P-Tuning V1 与 Prefix-Tuning 的区别:
from peft import PromptTuningConfig, get_peft_model
model_name_or_path = "./unsup-simcse-roberta-base"
peft_type = PeftType.P_TUNING
lr = 1e-3
peft_config = PromptEncoderConfig(task_type="CAUSAL_LM", num_virtual_tokens=20, encoder_hidden_size=128)
model = AutoModelForCausalLM.from_pretrained(model_name_or_path, return_dict=True)
model = get_peft_model(model, peft_config)
model.print_trainable_parameters()
论文《P-Tuning v2: Prompt Tuning Can Be Comparable to Fine-tuning Universally Across Scales and Tasks》
P-Tuning v2 的目标就是要让 Prompt Tuning 能够在不同参数规模的预训练模型、针对不同下游任务的结果上都达到匹敌 Fine-tuning 的结果。
Prompt Tuning 方法可能在这两个方面都存在局限性:
相比 Prompt Tuning 和 P-tuning 的方法,P-tuning v2 方法在多层加入了 Prompts tokens 作为输入,带来两个方面的好处:
带来更多可学习的参数(从 P-tuning 和 Prompt Tuning 的 0.1% 增加到0.1%-3%),同时也足够 parameter-efficient。
加入到更深层结构中的 Prompt 能给模型预测带来更直接的影响。
v1到v2的可视化:蓝色部分为参数冻结,橙色部分为可训练部分
from peft import PromptTuningConfig, get_peft_model
model_name_or_path = "./unsup-simcse-roberta-base"
peft_type = PeftType.P_TUNING
lr = 1e-3
peft_config = PrefixTuningConfig(task_type="SEQ_CLS", num_virtual_tokens=20)
model = AutoModelForSequenceClassification.from_pretrained(model_name_or_path, return_dict=True)
model = get_peft_model(model, peft_config)
model.print_trainable_parameters()
现有的一些 PEFT 的方法还存在这样一些问题:
Low-Rank Adaption(LoRA),设计了如下所示的结构,在涉及到矩阵相乘的模块,引入 A、B 这样两个低秩矩阵模块去模拟 Full-finetune 的过程,相当于只对语言模型中起关键作用的低秩本质维度进行更新。
这么做就能完美解决以上存在的 3 个问题:
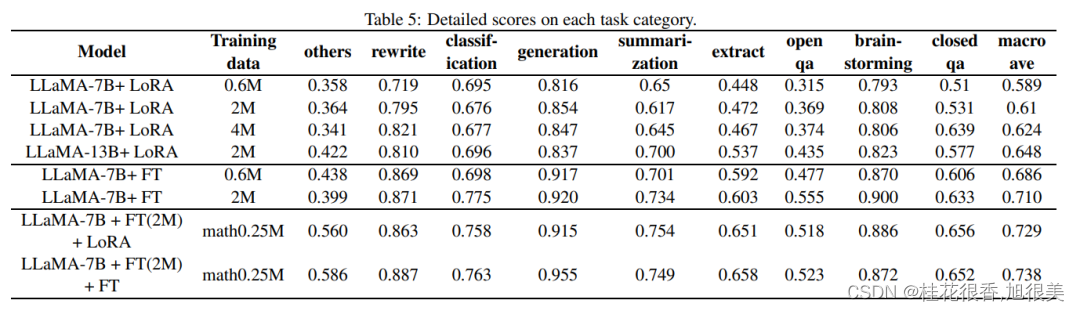
```python
from peft import PromptTuningConfig, get_peft_model
model_name_or_path = "./unsup-simcse-roberta-base"
peft_type = peft_type = PeftType.LORA
lr = 3e-4
peft_config = LoraConfig(task_type="SEQ_CLS", inference_mode=False, r=8, lora_alpha=16, lora_dropout=0.1)
model = AutoModelForCausalLM.from_pretrained(model_name_or_path, return_dict=True)
model = get_peft_model(model, peft_config)
model.print_trainable_parameters()
https://huggingface.co/docs/peft/conceptual_guides/lora
通过对 Prefix Tuning 的推导,得出了和 Adapter Tuning 以及 LoRA 形式一致的形式。
更近一步地,可以将这些 Tuning 的方法统一在同一套框架下,
包括这几大要素: