Logistic回归——手写数字识别
可视化数据集
该训练样本为5,000张20*20的书写数字的灰度图。
X:5000*400
y : 5000*1
在X中随机选取100张图像并显示
function [h, display_array] = displayData(X, example_width)
if ~exist('example_width', 'var') || isempty(example_width)
example_width = round(sqrt(size(X, 2)));
end
colormap(gray);
[m n] = size(X);
example_height = (n / example_width);
display_rows = floor(sqrt(m));
display_cols = ceil(m / display_rows);
pad = 1;
display_array = - ones(pad + display_rows * (example_height + pad), ...
pad + display_cols * (example_width + pad));
curr_ex = 1;
for j = 1:display_rows
for i = 1:display_cols
if curr_ex > m,
break;
end
max_val = max(abs(X(curr_ex, :)));
display_array(pad + (j - 1) * (example_height + pad) + (1:example_height), ...
pad + (i - 1) * (example_width + pad) + (1:example_width)) = ...
reshape(X(curr_ex, :), example_height, example_width) / max_val;
curr_ex = curr_ex + 1;
end
if curr_ex > m,
break;
end
end
h = imagesc(display_array, [-1 1]);
axis image off
drawnow;
end
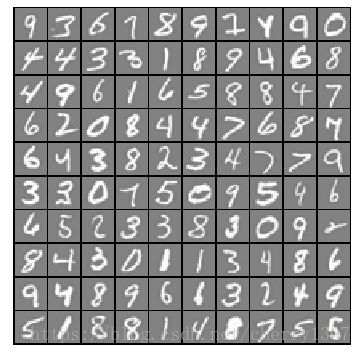
代价函数和梯度下降
function [J, grad] = lrCostFunction(theta, X, y, lambda)
m = length(y);
J = 0;
grad = zeros(size(theta));
J = (1/m)*(-y'*log(sigmoid(X*theta))-(1-y)'*log(1-sigmoid(X*theta)))+lambda/2/m*sum(theta(2:end).^2);
temp = theta;
temp(1) = 0;
grad = (1/m)*(X'*(sigmoid(X*theta)-y))+lambda/m*temp;
grad = grad(:);%21*1
end
一对多
function [all_theta] = oneVsAll(X, y, num_labels, lambda)
m = size(X, 1);%5000
n = size(X, 2);%20
all_theta = zeros(num_labels, n + 1);%10*21
X = [ones(m, 1) X]; %5000*21
options = optimset('GradObj', 'on', 'MaxIter', 50);
for i = 1: num_labels
all_theta(i,:) = fmincg (@(t)(lrCostFunction(t, X, (y == i), lambda)), all_theta(i,:)', options);
end
预测
function p = predictOneVsAll(all_theta, X)
m = size(X, 1);
num_labels = size(all_theta, 1);
p = zeros(size(X, 1), 1);
X = [ones(m, 1) X];
[r, p] = max(X * all_theta', [], 2);
end
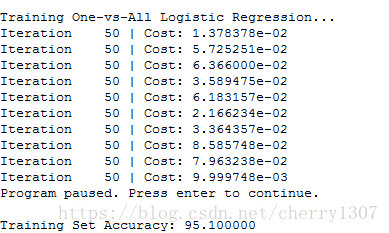