本文对应代码和数据已上传至我的
Github
仓库https://github.com/CNFeffery/DataScienceStudyNotes
1 简介
在上一篇文章中我们对geopandas
中的坐标参考系有了较为深入的学习,而在日常空间数据分析工作中矢量文件的读入和写出,是至关重要的环节。
作为基于geopandas的空间数据分析系列文章的第三篇,通过本文你将会学习到geopandas
中的文件IO。
2 文件IO
2.1 矢量文件的读入
geopandas
将fiona
作为操纵矢量数据读写功能的后端,使用geopandas.read_file()
读取对应类型文件,而在后端实际上是使用fiona.open
来读入数据,即两者参数是保持一致的,读入的数据自动转换为GeoDataFrame
,下面是geopandas.read_file()
主要参数:
filename:str类型,传入文件对应的路径或url
layer:str类型,当要读入的数据格式为地理数据库.gdb
或QGIS
中的.gpkg
时,传入对应图层的名称
下面结合上述参数,来介绍一下使用geopandas.read_file()
在不同情况下读取常见格式矢量数据的方法,使用到的示例数据为中国地图,CRS
为EPSG:4326
,本文使用到的所有数据都可以在文章开头提及的Github
仓库对应本文路径下找到:
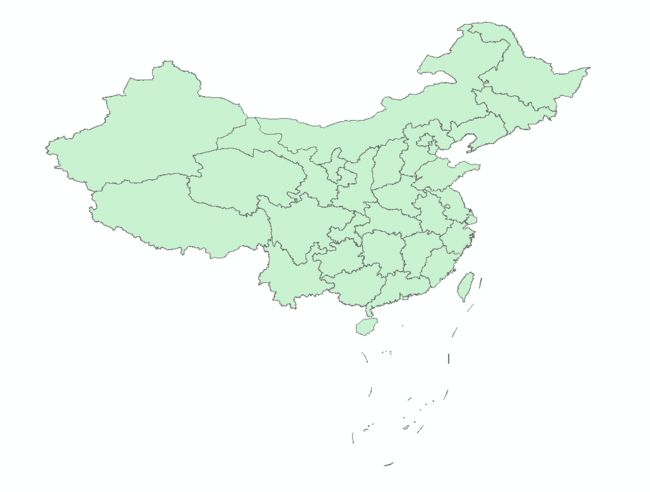
2.1.1 shapefile
作为非常常见的一种矢量文件格式,geopandas
对shapefile
提供了很好的读取和写出支持,下面分为不同情况来介绍:
- 完整的shapefile
如图2,这是一个完整的shapefile
:
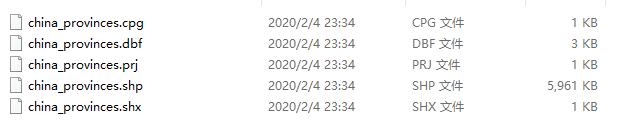
使用geopandas
来读取这种形式的shapefile
很简单:
import geopandas as gpd
data = gpd.read_file('geometry/china_provinces/china_provinces.shp')
print(data.crs) # 查看数据对应的crs
data.head() # 查看前5行
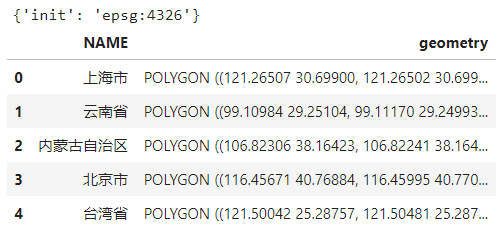
- 缺少投影的shapefile
当shapefile
中缺失.prj
文件时,使用geopandas
读入后形成的GeoDataFrame
会缺失crs
属性:

如果已经知道数据对应的CRS
,可以在读入数据后补充上crs
信息以进行其他操作:
import pyproj
data.crs = pyproj.CRS.from_user_input('EPSG:4326')
data.crs
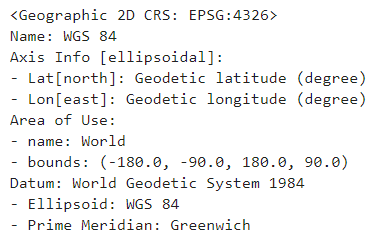
- 直接读取文件夹
当文件夹下只有单个shapefile时,可以直接读取该文件夹:
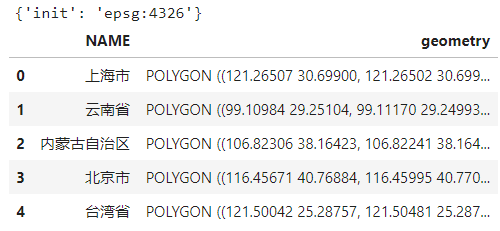
- 读取zip压缩包中的文件
geopandas
通过传入特定语法格式的文件路径信息,以支持直接读取.zip
格式压缩包中的shapefile
文件,主要分为两种情况。
当文件在压缩包内的根目录时,使用下面的语法规则来读取数据:
zip://路径/xxx.zip
譬如我们要读取图7所示的压缩包内文件:
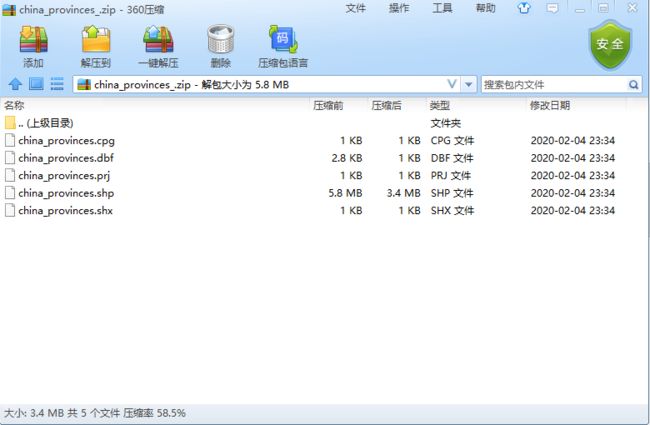
按照对应的语法规则,读取该类型数据方式如下:
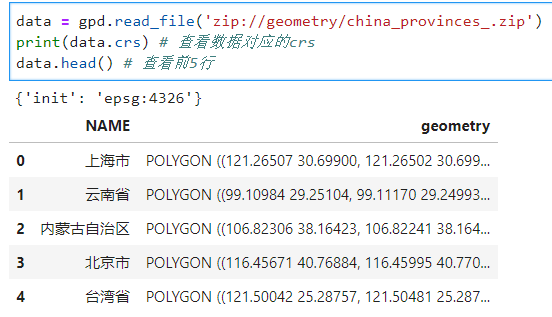
而当文件在压缩包内的文件夹中时,如图9:
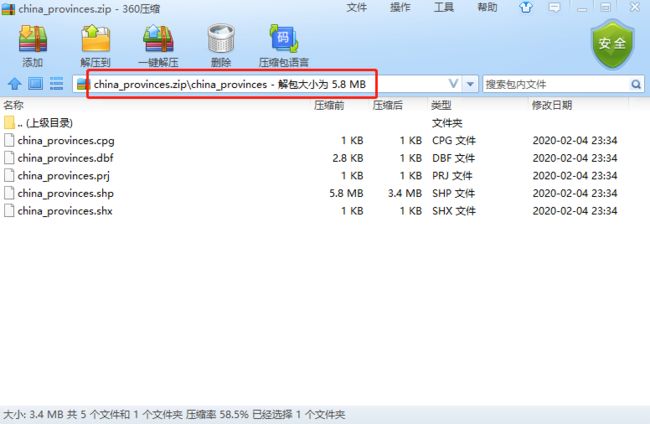
使用下面的语法规则来读取数据:
zip://路径/xxx.zip!压缩包内指定文件路径
将上述语法运用到上述文件:
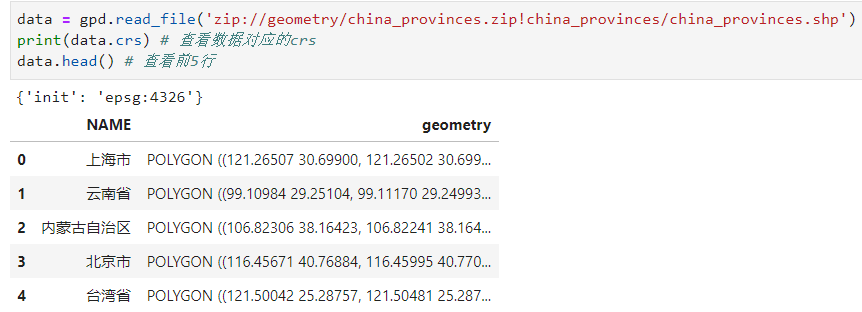
2.1.2 gdb与gpkg
对于Arcgis
中的地理数据库gdb
,以及QGIS
中的GeoPackage
,要读取其包含的矢量数据,就要涉及到图层的概念,对应geopandas.read_file()
的layer
参数,只需要将gdb
或gpkg
文件路径作为filename
参数,再将对应的图层名称作为layer
参数传入:
- gdb
data = gpd.read_file('geometry/china_provinces.gdb',
layer='china_provinces')
print(data.crs) # 查看数据对应的crs
data.head() # 查看前5行
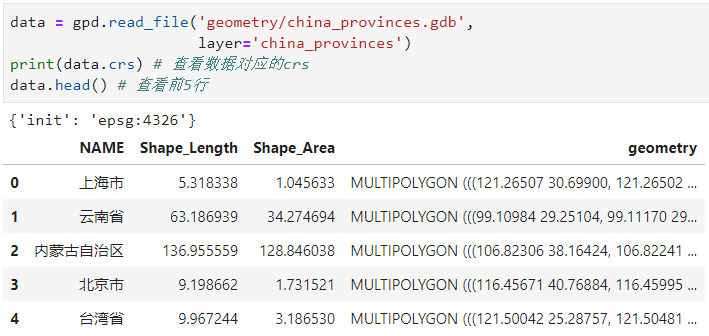
类似读入gdb
文件:
data = gpd.read_file('geometry/china_provinces.gpkg',
layer='china_provinces',
encoding='utf-8')
print(data.crs) # 查看数据对应的crs
data.head() # 查看前5行
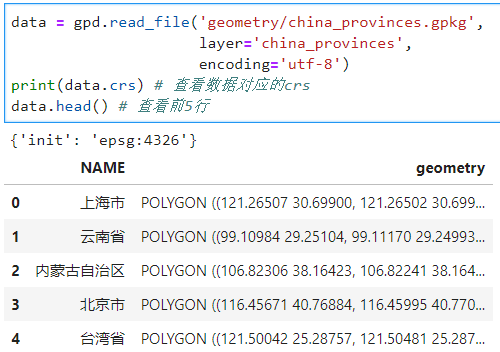
2.1.3 GeoJSON
作为web地图中最常使用的矢量数据格式,GeoJSON
几乎被所有在线地图框架作为数据源格式,在geopandas
中读取GeoJSON
非常简单,只需要传入文件路径名称即可,下面我们来读入图13所示的文件:
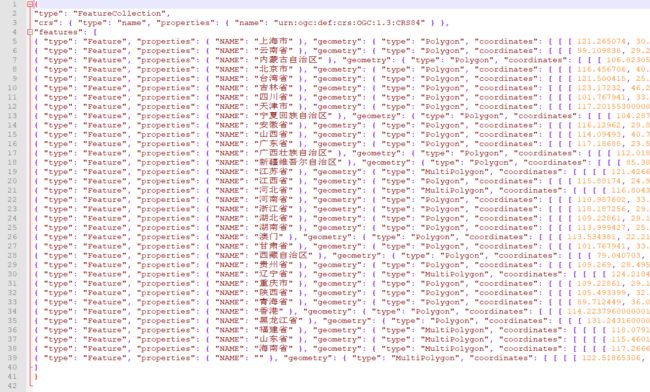
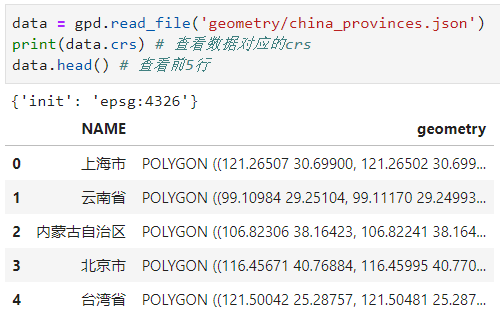
2.1.4 过滤
geopandas
在0.1.0版本中新增了bbox过滤,在0.7.0版本中新增了蒙版过滤和行过滤功能,可以辅助我们根据自己的需要读入原始数据中的子集,下面一一进行介绍:
- bbox过滤
bbox过滤允许我们在read_file()
中传入一个边界框作为参数bbox
,格式为(左下角x, 左下角y, 右上角x, 右上角y)
,这样在读入的过程中只会保留几何对象与bbox
有相交的数据记录,下面我们仍然以上文中使用过的中国地图数据为例,我们在读入的过程中,传入边界框:
from shapely import geometry
data = gpd.read_file('geometry/china_provinces.json',
bbox=(100, 20, 110, 30))
%matplotlib widget
ax = data.plot()
# 绘制bbox框示意
ax = gpd.GeoSeries([geometry.box(minx=100,
miny=20,
maxx=110,
maxy=30).boundary]).plot(ax=ax, color='red')
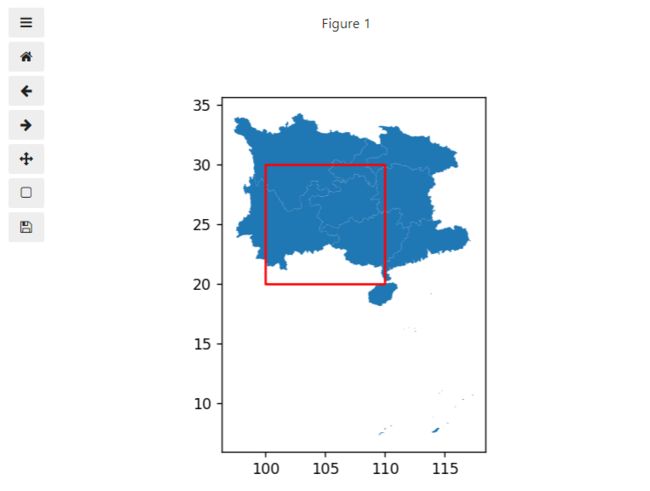
可以看到只有跟红色框有相交的几何对象被读入。
- 蒙版过滤
蒙版过滤和bbox过滤功能相似,都是筛选与指定区域相交的数据记录,不同的是蒙版过滤通过mask
参数可以传入任意形状的多边形,不再像bbox过滤那样只接受矩形:
data = gpd.read_file('geometry/china_provinces.json',
mask=geometry.Polygon([(100, 20), (110, 30), (120, 20)]))
ax = data.plot()
# 绘制bbox框示意
ax = gpd.GeoSeries([geometry.Polygon([(100, 20),
(110, 30),
(120, 20)]).boundary]).plot(ax=ax, color='red')
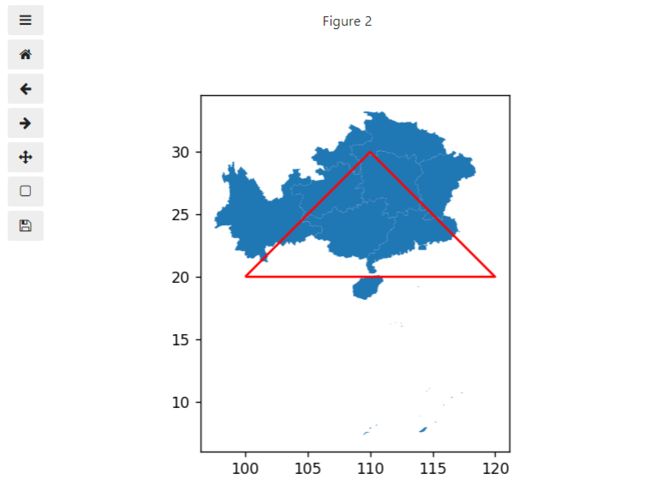
可以看到只有跟红色多边形相交的几何对象被读入。
- 行过滤
行过滤的功能就比较简单,通过参数rows
控制读入原数据的前若干行,可以用于在读取大型数据时先快速查看前几行以了解整个数据的格式:
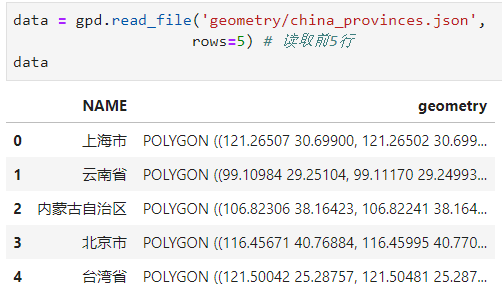
2.2 矢量文件的写出
在geopandas
中使用to_file()
来将GeoDataFrame
或GeoSeries
写出为矢量文件,主要支持shapefile
、GeoJSON
以及GeoPackage
,不像geopandas.read_file()
可以根据传入的文件名称信息自动推断类型,我们在写出矢量数据时就需要使用driver
参数来声明文件类型:
- ESRI Shapefile
我们将上文最后一次读入的GeoDataFrame
写出为ESRI Shapefile
,设置driver
参数为ESRI Shapefile
,如果你对文件编码有要求,这里可以使用encoding
参数来指定,譬如这里我们指定为utf-8
:
'''在工程根目录下创建output文件夹'''
import os
try:
os.mkdir('output')
except FileExistsError:
pass
data.to_file('output/output.shp',
driver='ESRI Shapefile',
encoding='utf-8')
可以看到在output文件夹下,成功导出了完整的shapefile
:
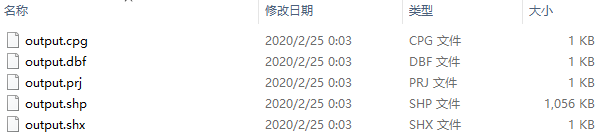
而如果导出的文件名不加后缀扩展名,则会生成包含在新目录下的shapefile
:
data.to_file('output/output_shapefile',
driver='ESRI Shapefile',
encoding='utf-8')
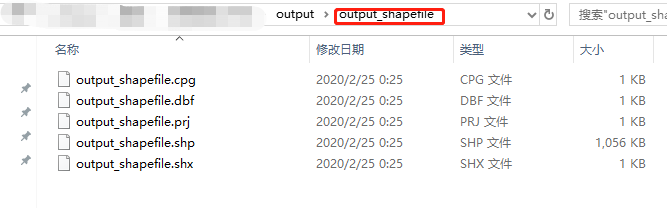
也可以向指定的文件夹下追加图层:
data.to_file('output/output_shapefile_multi_layer',
driver='ESRI Shapefile',
layer='layer1',
encoding='utf-8')
data.to_file('output/output_shapefile_multi_layer',
driver='ESRI Shapefile',
layer='layer2',
encoding='utf-8')
data.to_file('output/output_shapefile_multi_layer',
driver='ESRI Shapefile',
layer='layer3',
encoding='utf-8')
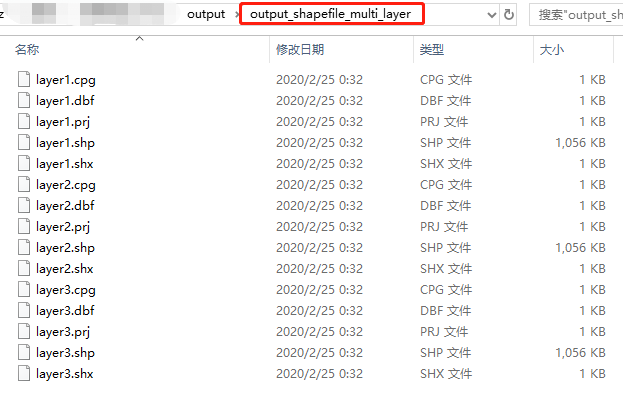
- GeoPackage
对于gdb
文件,由于ESRI
的限制,暂时无法在开源的geopandas
中导出,但我们可以用QGIS
中的GeoPackage
作为替代方案(开源世界万岁O(∩_∩)O~~),只需要将driver
参数设置为GPKG
即可,这里需要注意一个bug:在使用geopandas
导出GeoPackage
文件时,可能会出现图21所示错误:
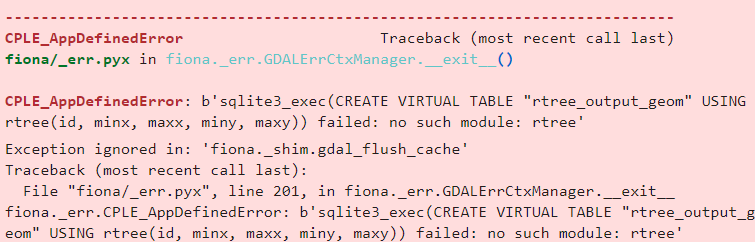
但我观察到即使出现了上述错误,GeoPackage
文件也是成功保存到路径下的且整个程序并未被打断,因此可以无视上述错误:
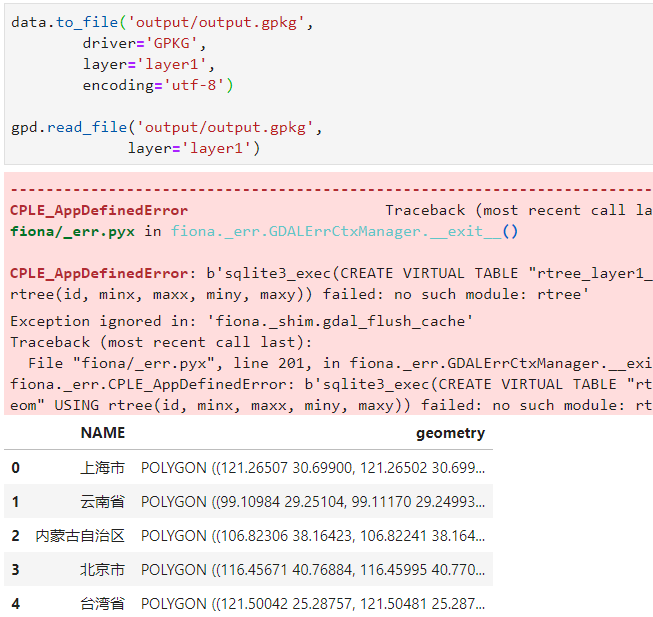
- GeoJSON
写出为GeoJSON
非常容易,只需要设置driver='GeoJSON'
即可:
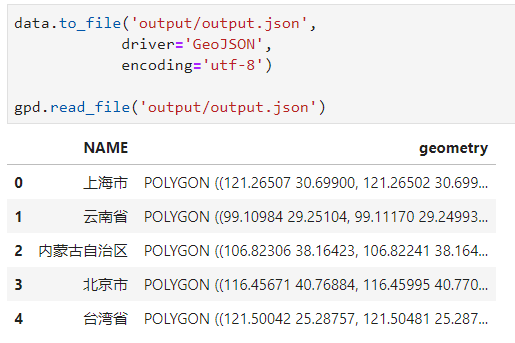
以上就是本文的全部内容,如有笔误望指出!