由于本地计算机速度有限,所以使用Colab进行训练。训练用的是VOC类型的数据集
!git clone https://github.com/AlexeyAB/darknet
Cloning into 'darknet'...
remote: Enumerating objects: 5, done.[K
remote: Counting objects: 100% (5/5), done.[K
remote: Compressing objects: 100% (5/5), done.[K
remote: Total 13503 (delta 0), reused 1 (delta 0), pack-reused 13498[K
Receiving objects: 100% (13503/13503), 12.07 MiB | 6.55 MiB/s, done.
Resolving deltas: 100% (9222/9222), done.
%cd /content/darknet
!sed -i 's/OPENCV=0/OPENCV=1/' Makefile
!sed -i 's/GPU=0/GPU=1/' Makefile
!sed -i 's/CUDNN=0/CUDNN=1/' Makefile
/content/darknet
!/usr/local/cuda/bin/nvcc --version
nvcc: NVIDIA (R) Cuda compiler driver
Copyright (c) 2005-2019 NVIDIA Corporation
Built on Sun_Jul_28_19:07:16_PDT_2019
Cuda compilation tools, release 10.1, V10.1.243
!make
mkdir -p ./obj/
mkdir -p backup
chmod +x *.sh
g++ -std=c++11 -std=c++11 -Iinclude/ -I3rdparty/stb/include -DOPENCV `pkg-config --cflags opencv4 2> /dev/null || pkg-config --cflags opencv` -DGPU -I/usr/local/cuda/include/ -DCUDNN -Wall -Wfatal-errors -Wno-unused-result -Wno-
(后面删了)
from google.colab import drive
drive.mount('/content/mydrive')
Go to this URL in a browser: https://accounts.google.com/o/oauth2/auth?client_id=947318989803-6bn6qk8qdgf4n4g3pfee6491hc0brc4i.apps.googleusercontent.com&redirect_uri=urn%3aietf%3awg%3aoauth%3a2.0%3aoob&response_type=code&scope=email%20https%3a%2f%2fwww.googleapis.com%2fauth%2fdocs.test%20https%3a%2f%2fwww.googleapis.com%2fauth%2fdrive%20https%3a%2f%2fwww.googleapis.com%2fauth%2fdrive.photos.readonly%20https%3a%2f%2fwww.googleapis.com%2fauth%2fpeopleapi.readonly
Enter your authorization code:
··········
Mounted at /content/mydrive
!ls /content/mydrive/My\ Drive/yolo
!ln -s /content/mydrive/My\ Drive/ /mydrive
1111.jpg obj.zip
backup train.txt
Gao_ship_hh_02016082545020305.jpg yolo_1.ipynb
generate_train.py yolo.ipynb
myData yolov4.conv.137
obj_1.zip yolov4-custom2.cfg
obj.data yolov4.weights
obj.names ZHCAMRAW_ERR_20190817173627_512453.jpg
!cp /mydrive/yolo/yolov4.weights ./
def imShow(path):
import cv2
import matplotlib.pyplot as plt
%matplotlib inline
image = cv2.imread(path)
height, width = image.shape[:2]
resized_image = cv2.resize(image,(3*width, 3*height), interpolation = cv2.INTER_CUBIC)
fig = plt.gcf()
fig.set_size_inches(18, 10)
plt.axis("off")
plt.imshow(cv2.cvtColor(resized_image, cv2.COLOR_BGR2RGB))
plt.show()
!./darknet detector test cfg/coco.data cfg/yolov4.cfg yolov4.weights data/dog.jpg
CUDA-version: 10010 (10010), cuDNN: 7.6.5, GPU count: 1
OpenCV version: 3.2.0
0 : compute_capability = 370, cudnn_half = 0, GPU: Tesla K80
net.optimized_memory = 0
mini_batch = 1, batch = 8, time_steps = 1, train = 0
layer filters size/strd(dil) input output
0 conv 32 3 x 3/ 1 608 x 608 x 3 -> 608 x 608 x 32 0.639 BF
1 conv 64 3 x 3/ 2 608 x 608 x 32 -> 304 x 304 x 64 3.407 BF
2 conv 64 1 x 1/ 1 304 x 304 x 64 -> 304 x 304 x 64 0.757 BF
3 route 1 -> 304 x 304 x 64
4 conv 64 1 x 1/ 1 304 x 304 x 64 -> 304 x 304 x 64 0.757 BF
5 conv 32 1 x 1/ 1 304 x 304 x 64 -> 304 x 304 x 32 0.379 BF
6 conv 64 3 x 3/ 1 304 x 304 x 32 -> 304 x 304 x 64 3.407 BF
7 Shortcut Layer: 4, wt = 0, wn = 0, outputs: 304 x 304 x 64 0.006 BF
8 conv 64 1 x 1/ 1 304 x 304 x 64 -> 304 x 304 x 64 0.757 BF
9 route 8 2 -> 304 x 304 x 128
10 conv 64 1 x 1/ 1 304 x 304 x 128 -> 304 x 304 x 64 1.514 BF
。。。(省略)
[yolo] params: iou loss: ciou (4), iou_norm: 0.07, cls_norm: 1.00, scale_x_y: 1.05
nms_kind: greedynms (1), beta = 0.600000
Total BFLOPS 128.459
avg_outputs = 1068395
Allocate additional workspace_size = 6.65 MB
Loading weights from yolov4.weights...
seen 64, trained: 32032 K-images (500 Kilo-batches_64)
Done! Loaded 162 layers from weights-file
data/dog.jpg: Predicted in 171.094000 milli-seconds.
bicycle: 92%
dog: 98%
truck: 92%
pottedplant: 33%
Unable to init server: Could not connect: Connection refused
(predictions:1474): Gtk-[1;33mWARNING[0m **: [34m02:53:27.076[0m: cannot open display:
imShow('predictions.jpg')
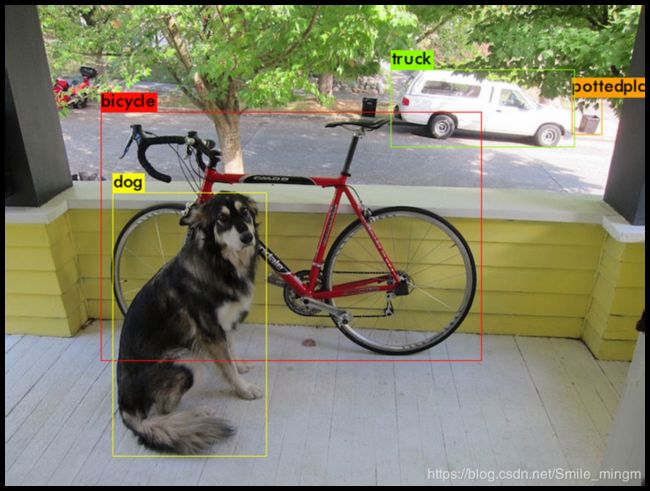
接下来需要如下六个文件
- obj.zip: 要包含如下两个文件夹,
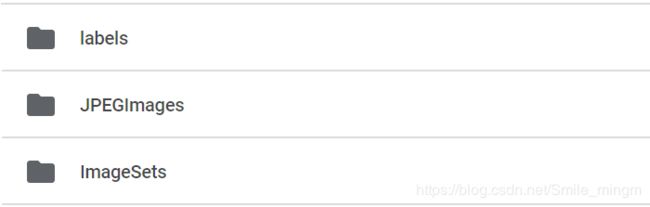
JPEGImages中包含所有的图片:
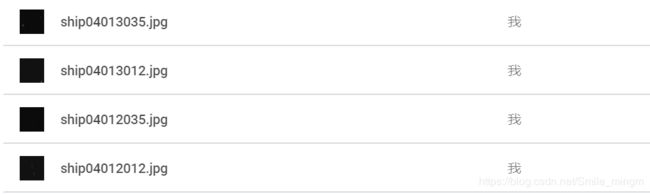
labels里包含所有的label.txt:
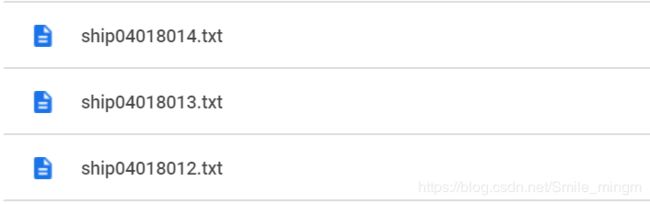
每一个label.txt中的具体内容如下,是每个图片bbox的坐标信息,可从xml文件中提取,这个处理过程可在网上搜素voc_label.py,有现成的代码:
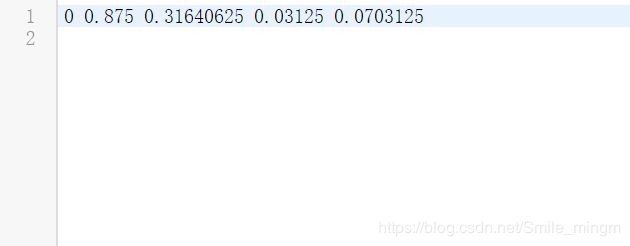
ImageSets/Main中存放了:
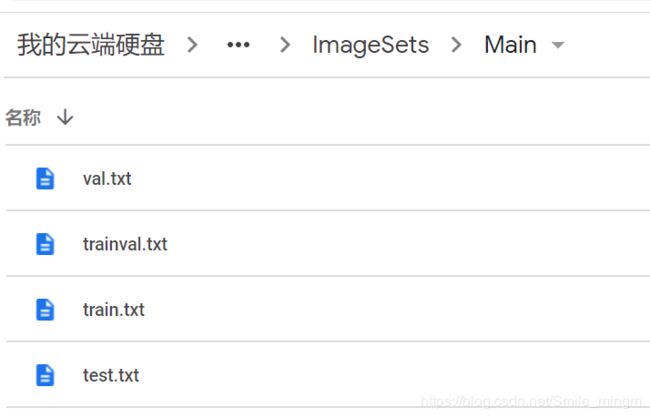
其中这些txt文件分别保存了图片的名字:
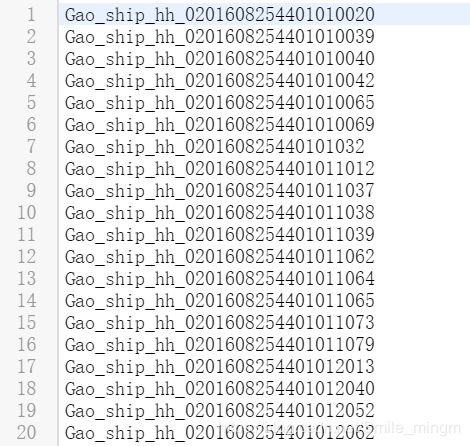
- obj.data:路径与种类数以及训练好的权重所存放的位置等信息
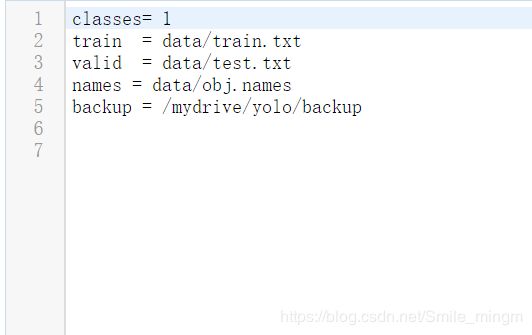
- obj.names:存放种类的信息
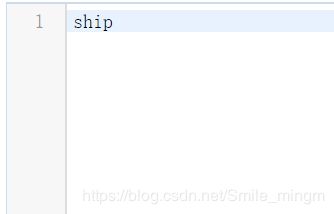
- yolov4-custom2.cfg:
- batch = 64 subdivisions = 16,如果有错,可将subdivisions改为32或64
- max_batches= ?:为自己数据集种类个数*2000
- steps=?,? :分别为max_batches的80%和90%
- 将每一个【yolo】(有三个)下的classes修改为自己的种类数
- 将每一个【yolo】(有三个)上面的【convolutional】中fiters改为(5+classes)x3
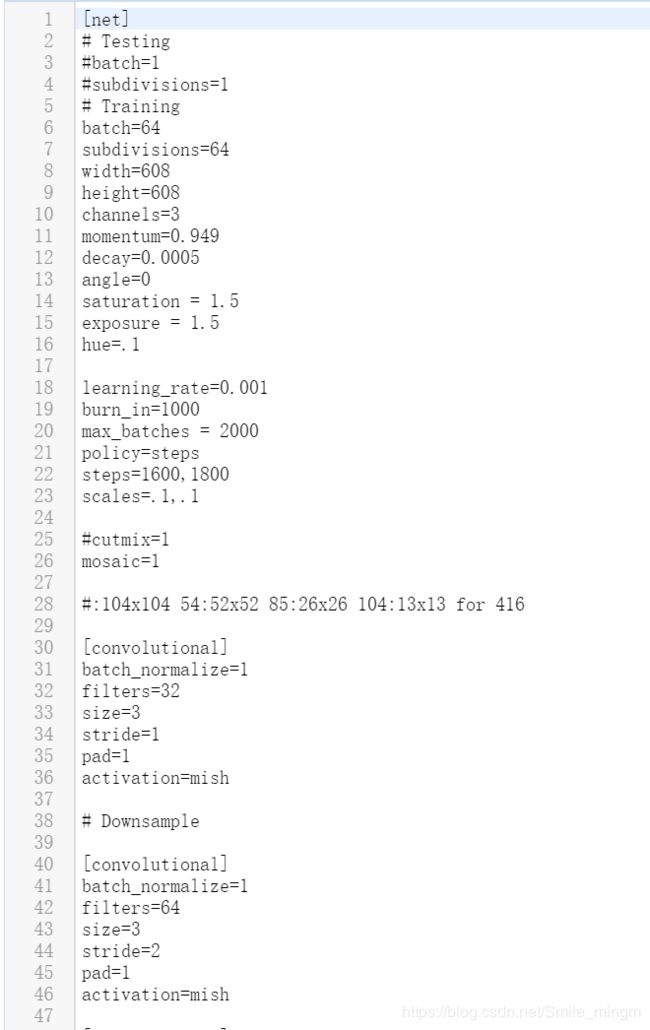
- train.txt:里面存放了训练图片的绝对路径,可用generate_train.py生成:
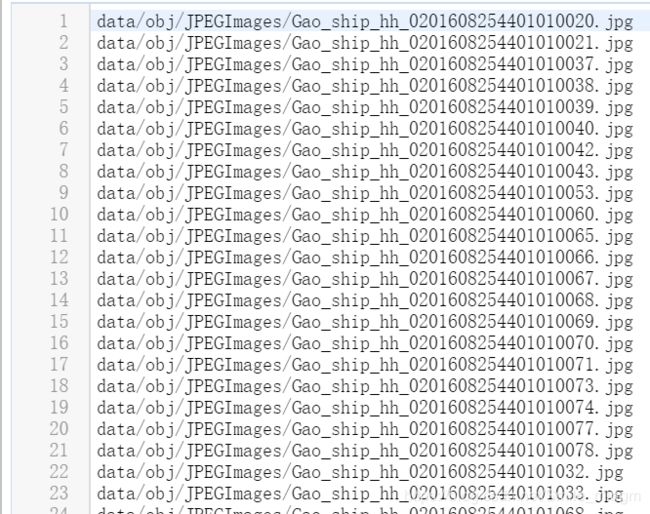
generate_train.py:
import os
image_files = []
for filename in os.listdir(r'E:\file\bupt\satellite\xudata\JPEGImages'):
if filename.endswith(".jpg"):
image_files.append("data/obj/JPEGImages/" + filename)
with open("train.txt", "w") as outfile:
for image in image_files:
outfile.write(image)
outfile.write("\n")
outfile.close()
接下来继续将准备好的这些文件放到对应位置并训练
!cp /mydrive/yolo/obj.zip ../
!unzip ../obj.zip -d data/
!cp /mydrive/yolo/yolov4-custom2.cfg ./cfg
!cp /mydrive/yolo/obj.data ./data
!cp /mydrive/yolo/obj.names ./data
!cp /mydrive/yolo/train.txt ./data
!cp /mydrive/yolo/yolov4.conv.137 ./
!./darknet detector train data/obj.data cfg/yolov4-custom2.cfg yolov4.conv.137 -dont_show
%cd cfg
!sed -i 's/batch=1/batch=64/' yolov4-custom2.cfg
!sed -i 's/subdivisions=1/subdivisions=64/' yolov4-custom2.cfg
%cd ..
!./darknet detector test data/obj.data cfg/yolov4-custom2.cfg /mydrive/yolo/backup/yolov4-ship_final.weights -thresh 0.02
imShow('predictions.jpg')