1.数据读取
import xgboost as xgb
data = xgb.DMatrix(libsvm文件)
from sklearn.datasets import load_svmlight_file
X_train,y_train = load_svmlight_file(libsvm文件)
2.模型训练过程
1.未调参基线模型
import xgboost as xgb
from sklearn.metrics import accuracy_score
dtrain = xgb.DMatrix(f_train, label = l_train)
dtest = xgb.DMatrix(f_test, label = l_test)
param = {'max_depth':2, 'eta':1, 'silent':0, 'objective':'binary:logistic' }
num_round = 2
bst = xgb.train(param, dtrain, num_round)
train_preds = bst.predict(dtrain)
train_predictions = [round(value) for value in train_preds]
train_accuracy = accuracy_score(l_train, train_predictions)
print ("Train Accuary: %.2f%%" % (train_accuracy * 100.0))
from xgboost import plot_importance
plot_importance(bst)
pyplot.show()
from xgboost import XGBClassifier
from sklearn.datasets import load_svmlight_file
from sklearn.metrics import accuracy_score
from matplotlib import pyplot
num_round = 100
bst1 =XGBClassifier(max_depth=2, learning_rate=1, n_estimators=num_round,
silent=True, objective='binary:logistic')
bst1.fit(f_train, l_train)
train_preds = bst1.predict(f_train)
train_accuracy = accuracy_score(l_train, train_preds)
print ("Train Accuary: %.2f%%" % (train_accuracy * 100.0))
preds = bst1.predict(f_test)
test_accuracy = accuracy_score(l_test, preds)
print("Test Accuracy: %.2f%%" % (test_accuracy * 100.0))
from xgboost import plot_importance
plot_importance(bst1)
pyplot.show()
2.两种交叉验证方式
from xgboost import XGBClassifier
from sklearn.model_selection import StratifiedKFold
from sklearn.model_selection import cross_val_score
from sklearn.metrics import accuracy_score
from matplotlib import pyplot
param = {'max_depth':2, 'eta':1, 'silent':0, 'objective':'binary:logistic' }
num_round = 100
bst2 =XGBClassifier(max_depth=2, learning_rate=0.1,n_estimators=num_round, silent=True, objective='binary:logistic')
bst2.fit(f_train, l_train)
kfold = StratifiedKFold(n_splits=10, random_state=7)
results = cross_val_score(bst2, f_train, l_train, cv=kfold)
print(results)
print("CV Accuracy: %.2f%% (%.2f%%)" % (results.mean()*100, results.std()*100))
from xgboost import plot_importance
plot_importance(bst2)
pyplot.show()
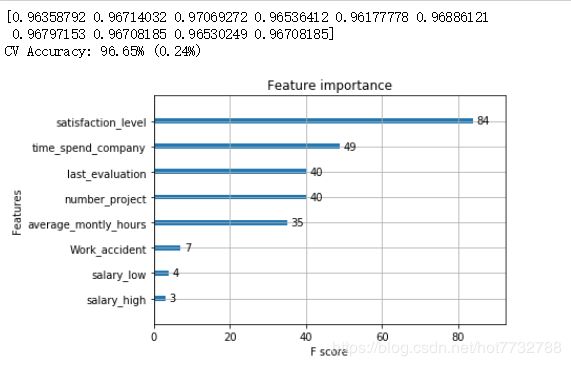
from xgboost import XGBClassifier
from sklearn.model_selection import GridSearchCV
from sklearn.metrics import accuracy_score
from matplotlib import pyplot
params = {'max_depth':2, 'eta':0.1, 'silent':0, 'objective':'binary:logistic' }
bst =XGBClassifier(max_depth=2, learning_rate=0.1, silent=True, objective='binary:logistic')
param_test = {
'n_estimators': range(1, 51, 1)
}
clf = GridSearchCV(estimator = bst, param_grid = param_test, scoring='accuracy', cv=5)
clf.fit(f_train, l_train)
preds = clf.predict(f_test)
test_accuracy = accuracy_score(l_test, preds)
print("Test Accuracy of gridsearchcv: %.2f%%" % (test_accuracy * 100.0))
clf.cv_results_, clf.best_params_, clf.best_score_
3.早停止调参–early_stopping_rounds(查看的是损失是否变化)
import xgboost as xgb
from xgboost import XGBClassifier
from sklearn.metrics import accuracy_score
from matplotlib import pyplot
param = {'max_depth':2, 'eta':1, 'silent':0, 'objective':'binary:logistic' }
num_round = 100
bst =XGBClassifier(max_depth=2, learning_rate=0.1, n_estimators=num_round, silent=True, objective='binary:logistic')
eval_set =[(f_test, l_test)]
bst.fit(f_train, l_train, early_stopping_rounds=10, eval_metric="error",eval_set=eval_set, verbose=True)
preds = bst.predict(f_test)
test_accuracy = accuracy_score(l_test, preds)
print("Test Accuracy: %.2f%%" % (test_accuracy * 100.0))
4.多数据观察训练损失
import xgboost as xgb
from xgboost import XGBClassifier
from sklearn.metrics import accuracy_score
from matplotlib import pyplot
num_round = 100
bst =XGBClassifier(max_depth=2, learning_rate=0.1, n_estimators=num_round, silent=True, objective='binary:logistic')
eval_set = [(f_train, l_train), (f_test, l_test)]
bst.fit(f_train, l_train, eval_metric=["error", "logloss"], eval_set=eval_set, verbose=True)
preds = bst.predict(f_test)
test_accuracy = accuracy_score(l_test, preds)
print("Test Accuracy: %.2f%%" % (test_accuracy * 100.0))
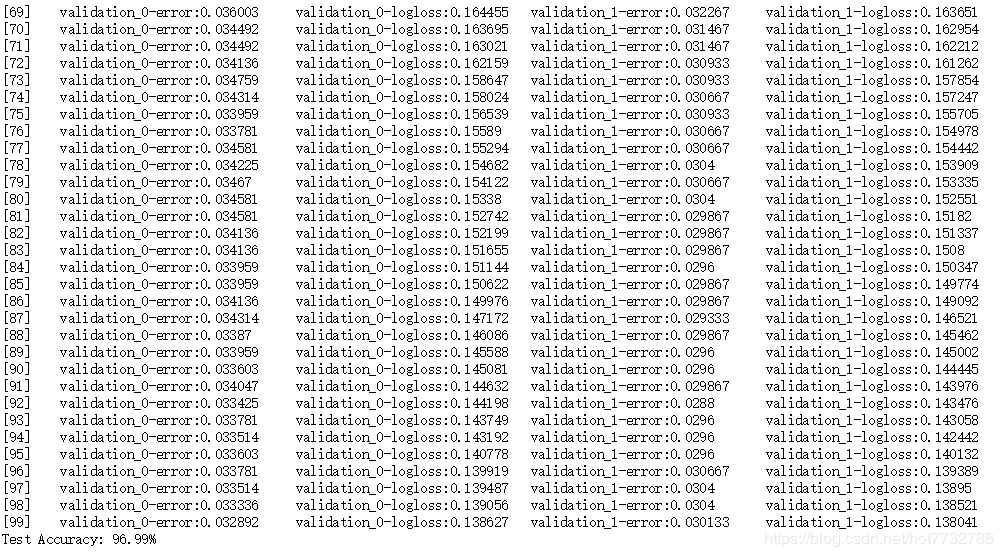
5.模型保存与读取
bst.save_model('demo.model')
modelfile = 'demo.model'
bst = xgb.Booster({'nthread':8}, model_file = modelfile)
f_test1 = xgb.DMatrix(f_test)
ypred1 = bst.predict(f_test1)
train_predictions = [round(value) for value in ypred1]
test_accuracy1 = accuracy_score(l_test, train_predictions)
print("Test Accuracy: %.2f%%" % (test_accuracy1 * 100.0))