data:2016-02-19
author:laidefa
########################朴素贝叶斯##################################
###目标:利用朴素贝叶斯预测苹果是好的坏的
rm(list=ls())
gc()
library(plyr)
library(reshape2)
#训练集
train.apple<-data.frame(size=c("大","小","大","大","小","小"),weight=c("轻","重","轻","轻","重","轻"),
color=c("红","红","红","绿","红","绿"),taste=c("good","good","bad","bad","bad","good"))
View(train.apple)
#计算类别的概率
(length.train<-nrow(train.apple))
(dTemp<-ddply(train.apple,.(taste),summarize,num=length(taste)))
(dTemp$prob<-dTemp$num/length.train)
head(dTemp)
(class_prob<-dTemp[,-2])
colnames(class_prob)<-c("class.name","prob")
#计算每个类别下,特征取不同值的概率
(data.melt<-melt(train.apple,id=c("taste")))
(aa<-ddply(data.melt,.(taste,variable,value),"nrow"))
(bb<-ddply(aa,c("taste","variable"),mutate,sum=sum(nrow),prob=nrow/sum))
colnames(bb)<-c("class.name","feature.name","feature.value","feature.nrow","feature.sum","prob")
(feature_class_prob<-bb[,c(1,2,3,6)])
#测试集
(oneObs<-data.frame(feature.name=c("size","weight","color"),feature.value=c("大","重","红")))
#开始预测
pc<-class_prob
pfc<-feature_class_prob
#取出特征的取值的条件概率
(feature.all<-join(oneObs,pfc,by=c("feature.name","feature.value"),type="inner"))
#取出特征取值的条件概率连乘
(feature.prob<-ddply(feature.all,.(class.name),summarize,prob_fea=prod(prob)))
#取出类别的概率
(class.all<-join(feature.prob,pc,by="class.name",type="inner"))
#输出预测结果
(pre_class<-ddply(class.all,.(class.name),mutate,pre_prob=prob_fea*prob)[,c(1,4)])
#######################结果##################
结论:这个苹果巴准是坏的!
>
> #输出预测结果
> (pre_class<-ddply(class.all,.(class.name),mutate,pre_prob=prob_fea*prob)[,c(1,4)])
class.name pre_prob
1 bad 0.07407407
2 good 0.03703704
#####################决策树##############################################
目标:鸢尾花的种类预测
rm(list=ls())
##使用包party建立决策树
library(party)
str(iris)
######分为训练和测试数据两部分
set.seed(1234)
ind<-sample(2,nrow(iris),replace=TRUE,prob=c(0.7,0.3))
TrainData<-iris[ind==1,]
dim(TrainData)
TestData<-iris[ind==2,]
dim(TestData)
####训练模型
iris_ctree<-ctree(Species~Sepal.Length+Sepal.Width+ Petal.Length+Petal.Width,data=TrainData)
print(iris_ctree)
plot(iris_ctree)
plot(iris_ctree,type="simple")
table(predict(iris_ctree),TrainData$Species)
####测试模型
testPred<-predict(iris_ctree,newdata=TestData)
table(testPred,TestData$Species)
结果:
Model formula:
Species ~ Sepal.Length + Sepal.Width + Petal.Length + Petal.Width
Fitted party:
[1] root
| [2] Petal.Length <= 1.9: setosa (n = 40, err = 0.0%)
| [3] Petal.Length > 1.9
| | [4] Petal.Width <= 1.7
| | | [5] Petal.Length <= 4.4: versicolor (n = 21, err = 0.0%)
| | | [6] Petal.Length > 4.4: versicolor (n = 19, err = 15.8%)
| | [7] Petal.Width > 1.7: virginica (n = 32, err = 3.1%)
Number of inner nodes: 3
Number of terminal nodes: 4
> plot(iris_ctree)
> table(predict(iris_ctree),TrainData$Species)
setosa versicolor virginica
setosa 40 0 0
versicolor 0 37 3
virginica 0 1 31
> testPred<-predict(iris_ctree,newdata=TestData)
> table(testPred,TestData$Species)
testPred setosa versicolor virginica
setosa 10 0 0
versicolor 0 12 2
virginica 0 0 14
>
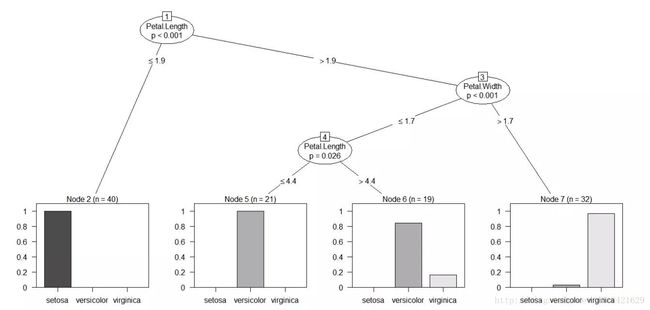