支持向量机是怎么画分类平面的?
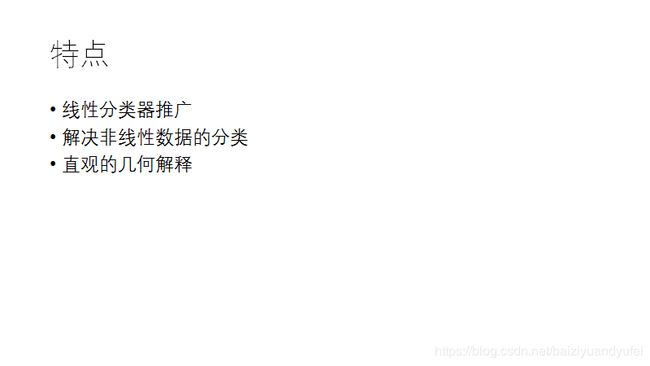
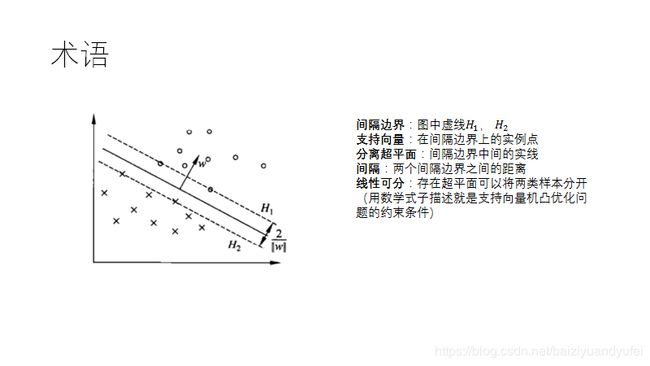
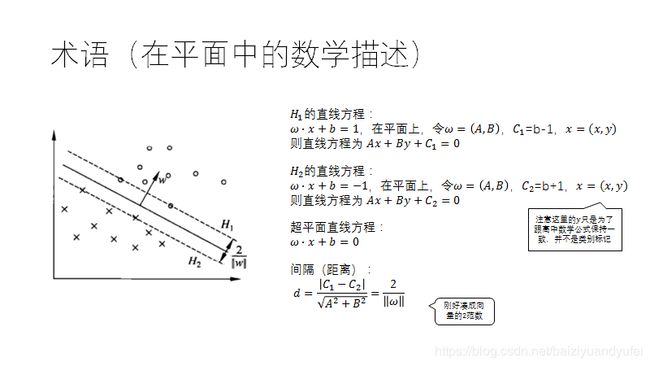
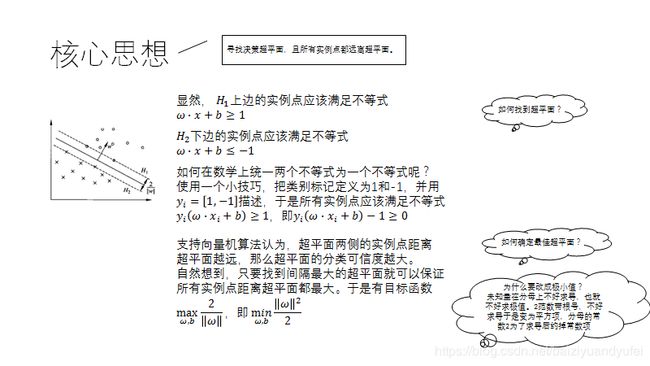
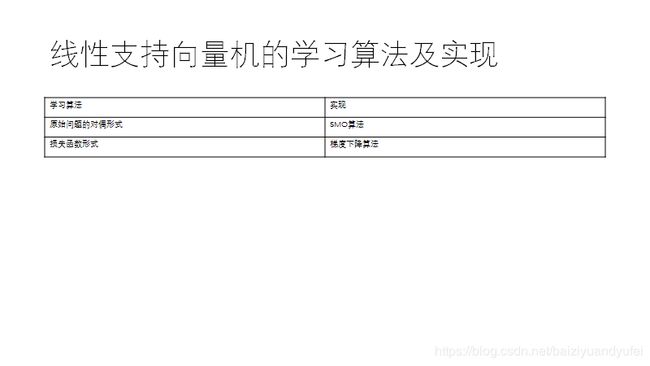
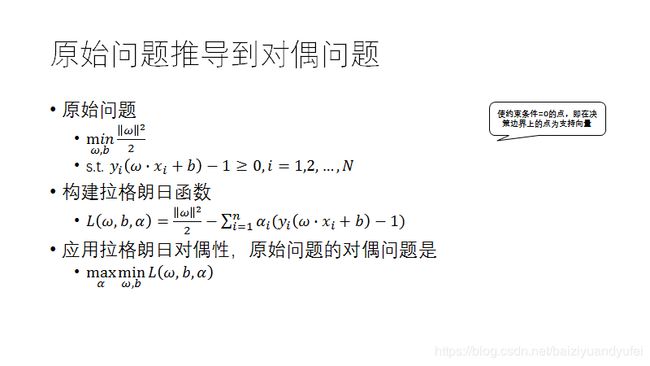
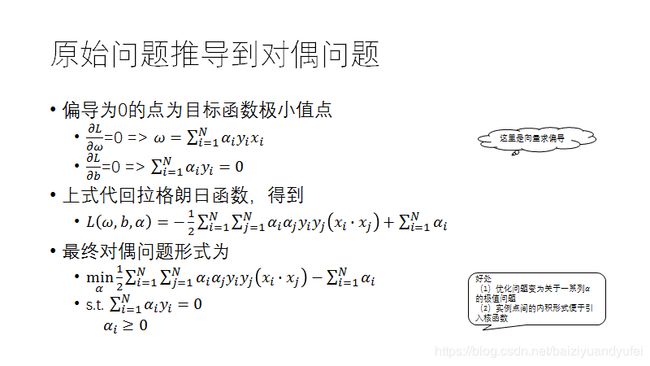
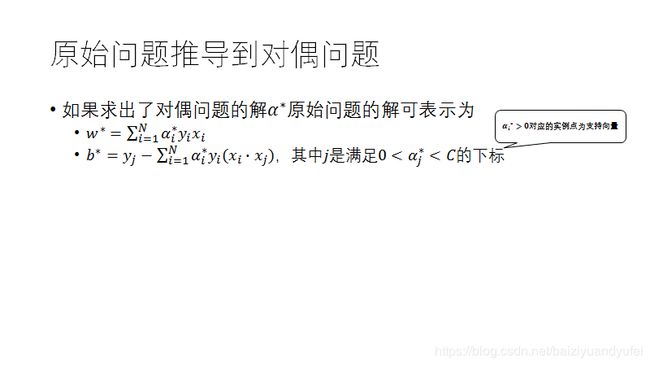
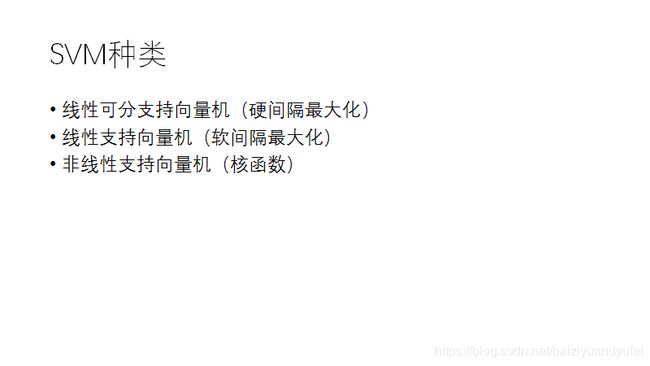
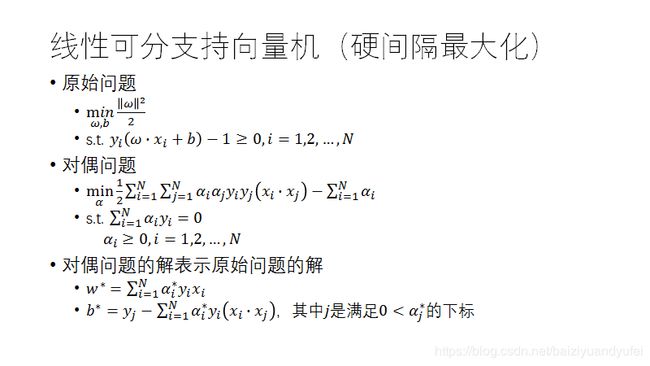
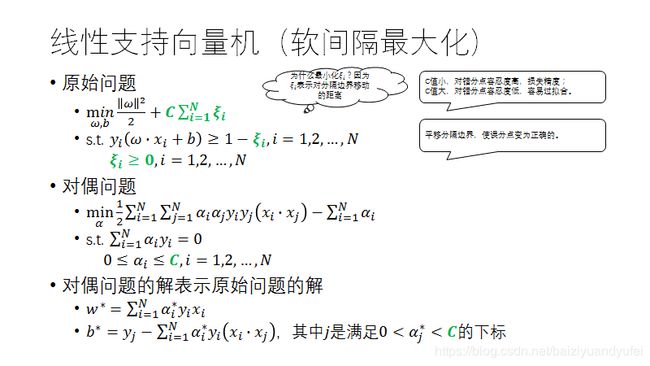
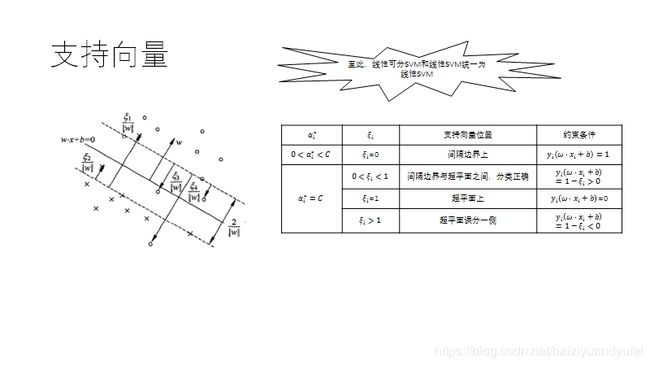
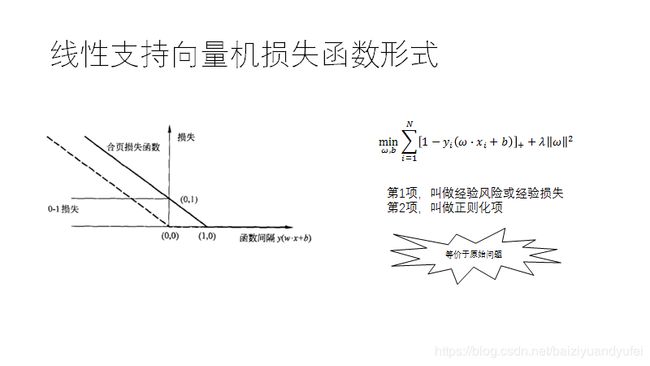
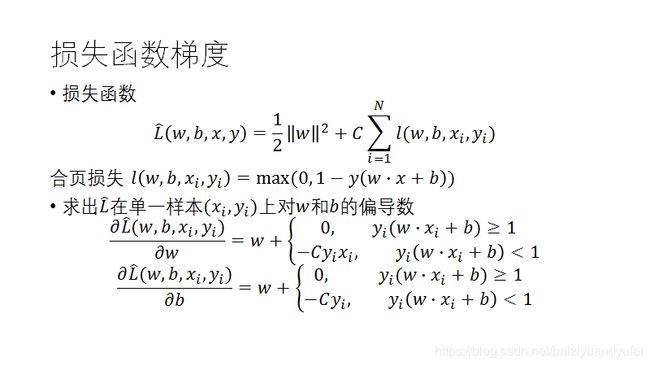
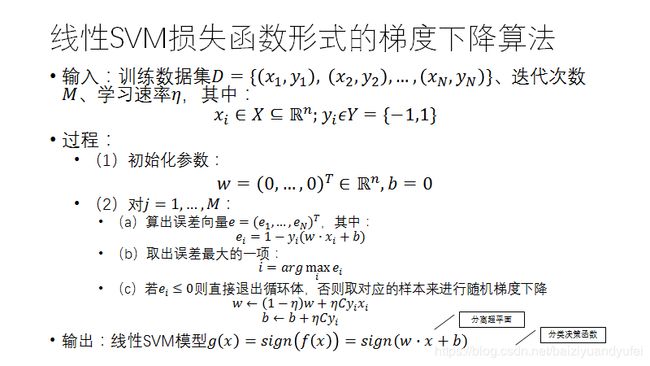
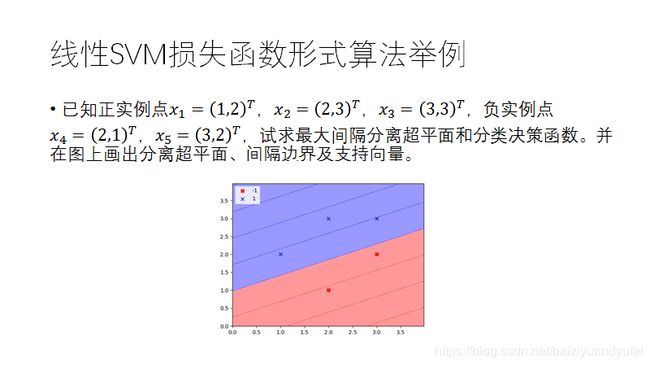
#coding:utf-8
"""
损失函数形式的支持向量机演示算法
"""
import numpy as np
from matplotlib import pyplot as plt
from matplotlib.colors import ListedColormap
# 线性支持向量机模型
class LinearSVM:
# 构建分类模型
def __init__(self, x, y, c=1, learning_rate=0.01, iterations=1000):
"""
:param x: 训练实例
:param y: 训练标记
:param c: 惩罚因子
:param learning_rate: 学习率
:param iterations: 最大迭代次数
"""
# 权值初始值
self.w = np.array([0, 0])
# 偏置初始值
self.b = 0
# 训练过程
for j in range(1, iterations+1):
print("iter ", j)
print("w=", self.w)
print("b=", self.b)
# 计算误差向量
ei_li = []
for i in range(x.shape[0]):
ei = 1 - y[i] * (np.dot(self.w, x[i, :]) + self.b)
ei_li.append(ei)
# 取出误差最大项
max_e = max(ei_li)
max_e_index = ei_li.index(max_e)
# 最大误差项<=0 退出
if max_e <= 0:
break
# 更新参数
self.w = (1-learning_rate) * self.w + learning_rate * c * y[max_e_index] * x[max_e_index, :]
self.b = self.b + learning_rate * c * y[max_e_index]
# 训练结束
print("w=", self.w)
print("b=", self.b)
# 画决策面
colors = ('red', 'blue')
cmap = ListedColormap(colors[:len(np.unique(y))])
x1_min, x1_max = x[:, 0].min() - 1, x[:, 0].max() + 1
x2_min, x2_max = x[:, 1].min() - 1, x[:, 1].max() + 1
xx1, xx2 = np.meshgrid(np.arange(x1_min, x1_max, 0.02),
np.arange(x2_min, x2_max, 0.02))
Z = self.predict(np.array([xx1.ravel(), xx2.ravel()]))
Z = Z.reshape(xx1.shape)
plt.contourf(xx1, xx2, Z, alpha=0.4, cmap=cmap)
plt.xlim(xx1.min(), xx1.max())
plt.ylim(xx2.min(), xx2.max())
# 画样本点
markers = ('s', 'x')
for idx, ci in enumerate(np.unique(y)):
plt.scatter(x=x[y == ci, 0], y=x[y == ci, 1], alpha=0.8, c=cmap(idx), marker=markers[idx], label=ci)
# 画图例
plt.legend(loc='upper left')
plt.show()
# 预测
def predict(self, x):
return np.dot(self.w, x) + self.b
if __name__ == "__main__":
x = np.array([[1, 2],
[2, 3],
[3, 3],
[2, 1],
[3, 2]])
y = np.array([1, 1, 1, -1, -1])
svm = LinearSVM(x, y)
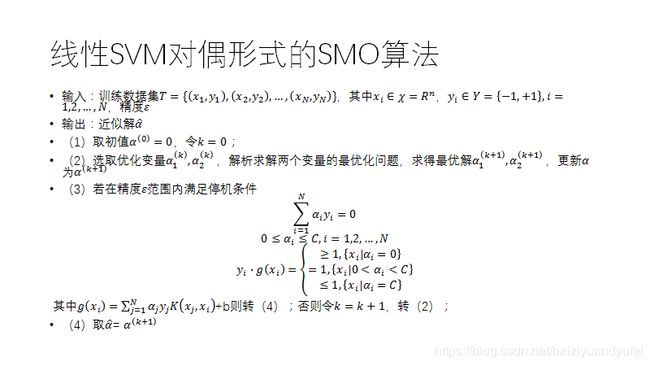
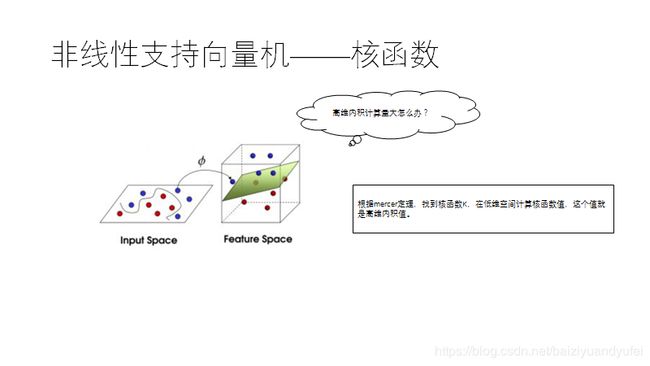
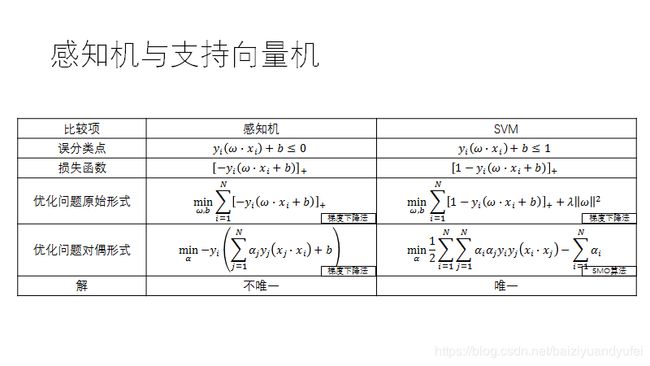