'''创建RBM基类,存放受限玻尔兹曼机'''
import tensorflow as tf
import numpy as np
import sys
def tf_xavier_init(fan_in,fan_out,*,const=1.0,dtype=np.float32):
k=const*np.sqrt(6.0/(fan_in+fan_out))
return tf.random_uniform((fan_in,fan_out),minval=-k,maxval=k,dtype=dtype)
class RBM:
def __init__(self,
n_visible,
n_hidden,
learning_rate=0.01,
momentum=0.95,
xavier_const=1.0,
err_function='mse',
use_tqdm=False,
tqdm=None):
if not 0.0<=momentum<=1.0:
raise ValueError('momentum should be in range [0, 1]')
if err_function not in {'mse','cosine'}:
raise ValueError('err_function should be either \'mse\' or \'cosine\'')
self._use_tqdm=use_tqdm
self._tqdm=None
if use_tqdm or tqdm is not None:
from tqdm import tqdm
self._tqdm=tqdm
self.n_visible = n_visible
self.n_hidden = n_hidden
self.learning_rate = learning_rate
self.momentum = momentum
self.x=tf.placeholder(tf.float32,[None,self.n_visible])
self.y=tf.placeholder(tf.float32,[None,self.n_hidden])
self.w=tf.Variable(tf_xavier_init(self.n_visible,self.n_hidden,const=xavier_const),dtype=tf.float32)
self.visible_bias=tf.Variable(tf.zeros([self.n_visible]),dtype=tf.float32)
self.hidden_bias=tf.Variable(tf.zeros([self.n_hidden]),dtype=tf.float32)
self.delta_w=tf.Variable(tf.zeros([self.n_visible,self.n_hidden]),dtype=tf.float32)
self.delta_visible_bias=tf.Variable(tf.zeros([self.n_visible]),dtype=tf.float32)
self.delta_hidden_bias=tf.Variable(tf.zeros([self.n_hidden]),dtype=tf.float32)
self.update_weights=None
self.update_deltas=None
self.compute_hidden=None
self.compute_visible=None
self.compute_visible_from_hidden=None
self._initialize_vars()
assert self.update_weights is not None
assert self.update_deltas is not None
assert self.compute_hidden is not None
assert self.compute_visible is not None
assert self.compute_visible_from_hidden is not None
if err_function=='cosine':
x1_norm=tf.nn.l2_normalize(self.x,1)
x2_norm=tf.nn.l2_normalize(self.compute_visible,1)
cos_val=tf.reduce_mean(tf.reduce_sum(tf.mul(x1_norm,x2_norm),1))
self.compute_err=tf.acos(cos_val)/tf.constant(np.pi)
else:
self.compute_err=tf.reduce_mean(tf.square(self.x-self.compute_visible))
init=tf.global_variables_initializer()
self.sess=tf.Session()
self.sess.run(init)
def _initialize_vars(self):
pass
def get_err(self,batch_x):
return self.sess.run(self.compute_err,feed_dict={self.x:batch_x})
def reconstruct(self,batch_x):
return self.sess.run(self.compute_visible,feed_dict={self.x:batch_x})
def partial_fit(self,batch_x):
self.sess.run(self.update_weights+self.update_deltas,feed_dict={self.x:batch_x})
def fit(self,
data_x,
n_epoches=10,
batch_size=10,
shuffle=True,
verbose=True
):
assert n_epoches>0
n_data=data_x.shape[0]
if batch_size>0:
n_batches=n_data//batch_size+(0 if n_data%batch_size==0 else 1)
else:
n_batches=1
if shuffle:
data_x_cpy=data_x.copy()
inds=np.arange(n_data)
else:
data_x_cpy=data_x
errs=[]
for e in range(n_epoches):
if verbose and not self._use_tqdm:
print('Epoch:{:d}'.format(e))
epoch_errs=np.zeros((n_batches,))
epoch_errs_ptr=0
if shuffle:
np.random.shuffle(inds)
data_x_cpy=data_x_cpy[inds]
r_batches=range(n_batches)
if verbose and self._use_tqdm:
r_batches=self._tqdm(r_batches,desc='Epoch:{:d}'.format(e),ascii=True,file=sys.stdout)
for b in r_batches:
batch_x=data_x_cpy[b*batch_size:(b+1)*batch_size]
self.partial_fit(batch_x)
batch_err=self.get_err(batch_x)
epoch_errs[epoch_errs_ptr]=batch_err
epoch_errs_ptr+=1
if verbose:
err_mean=epoch_errs.mean()
if self._use_tqdm:
self._tqdm.write('Train error:{:.4f}'.format(err_mean))
self._tqdm.write('')
else:
print('Train error:{:.4f}'.format(err_mean))
print('')
sys.stdout.flush()
errs=np.hstack([errs,epoch_errs])
return errs
'''在RBM基础上创建一个伯努利RBM深度网络结构,用类保存结构,该类继承于RBM类,根据不同的受限玻尔兹曼机的模型结构进行不一样的参数初始化'''
def sample_bernoulli(probs):
return tf.nn.relu(tf.sign(probs-tf.random_uniform(tf.shape(probs))))
class BBRBM(RBM):
def __init__(self,*args,**kwargs):
RBM.__init__(self,*args,**kwargs)
def _initialize_vars(self):
hidden_p=tf.nn.sigmoid(tf.matmul(self.x,self.w)+self.hidden_bias)
visible_recon_p=tf.nn.sigmoid(tf.matmul(sample_bernoulli(hidden_p),tf.transpose(self.w))+self.visible_bias)
hidden_recon_p=tf.nn.sigmoid(tf.matmul(visible_recon_p,self.w)+self.hidden_bias)
positive_grad=tf.matmul(tf.transpose(self.x),hidden_p)
negative_grad=tf.matmul(tf.transpose(visible_recon_p),hidden_recon_p)
def f(x_old,x_new):
return self.momentum*x_old+self.learning_rate * x_new * (1 - self.momentum) / tf.to_float(tf.shape(x_new)[0])
delta_w_new = f(self.delta_w, positive_grad - negative_grad)
delta_visible_bias_new = f(self.delta_visible_bias, tf.reduce_mean(self.x - visible_recon_p, 0))
delta_hidden_bias_new = f(self.delta_hidden_bias, tf.reduce_mean(hidden_p - hidden_recon_p, 0))
update_delta_w = self.delta_w.assign(delta_w_new)
update_delta_visible_bias = self.delta_visible_bias.assign(delta_visible_bias_new)
update_delta_hidden_bias = self.delta_hidden_bias.assign(delta_hidden_bias_new)
update_w = self.w.assign(self.w + delta_w_new)
update_visible_bias = self.visible_bias.assign(self.visible_bias + delta_visible_bias_new)
update_hidden_bias = self.hidden_bias.assign(self.hidden_bias + delta_hidden_bias_new)
self.update_deltas = [update_delta_w, update_delta_visible_bias, update_delta_hidden_bias]
self.update_weights = [update_w, update_visible_bias, update_hidden_bias]
self.compute_hidden = tf.nn.sigmoid(tf.matmul(self.x, self.w) + self.hidden_bias)
self.compute_visible = tf.nn.sigmoid(tf.matmul(self.compute_hidden, tf.transpose(self.w)) + self.visible_bias)
self.compute_visible_from_hidden = tf.nn.sigmoid(tf.matmul(self.y, tf.transpose(self.w)) + self.visible_bias)
import matplotlib.pyplot as plt
from tensorflow.examples.tutorials.mnist import input_data
'''创建主模块,对RBM网络结构进行训练和测试'''
mnist=input_data.read_data_sets('/mnist',one_hot=True)
mnist_images=mnist.train.images
model=BBRBM(n_visible=784,n_hidden=64,learning_rate=0.01,momentum=0.95,use_tqdm=True)
errs=model.fit(mnist_images,n_epoches=30,batch_size=10)
plt.plot(errs)
plt.show()
def show_digit(x):
plt.imshow(x.reshape((28,28)),cmap=plt.cm.gray)
plt.show()
IMAGE=1
image=mnist_images[IMAGE]
show_digit(image)
image_rec=model.reconstruct(image.reshape(1,-1))
show_digit(image_rec)
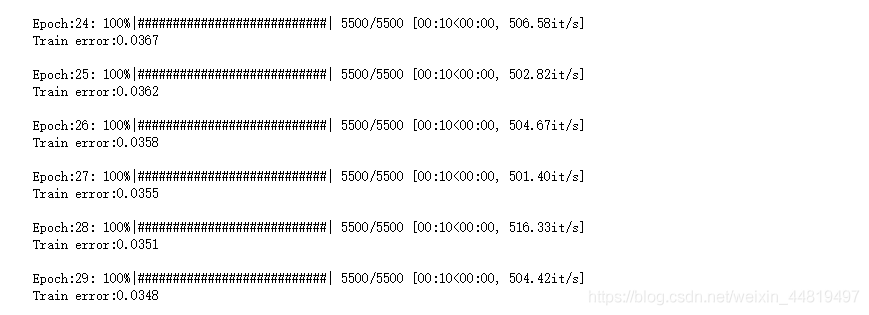
误差图:
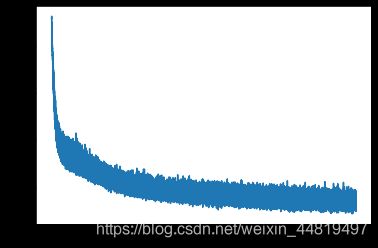
原图:
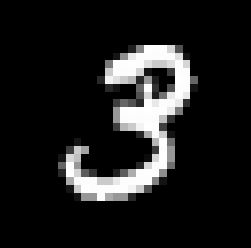
生成的训练结果图:
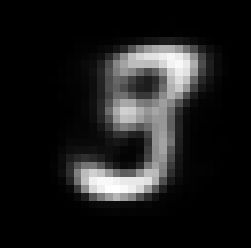
IMAGE=2
image=mnist_images[IMAGE]
show_digit(image)
image_rec=model.reconstruct(image.reshape(1,-1))
show_digit(image_rec)
原图:
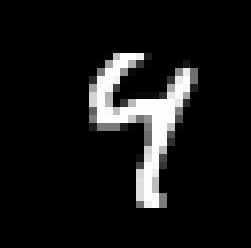
生成的训练结果图:
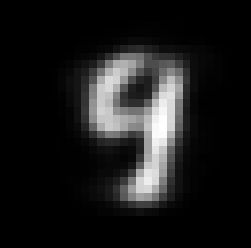