1.Inception
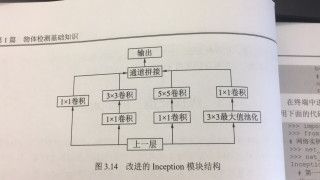
import torch
from torch import nn
import torch.nn.functional as F
class BasicConv2d(nn.Module):
def __init__(self,in_dim,out_dim,kernel_size,padding=0):
super(BasicConv2d,self).__init__()
self.conv=nn.Conv2d(in_dim,out_dim,kernel_size,padding=padding)
self.bn=nn.BatchNorm2d(out_dim,eps=0.001)
def forward(self, x) :
x=self.conv(x)
x=self.bn(x)
return F.relu(x,inplace=True)
class Inceptionv2(nn.Module):
def __init__(self):
super(Inceptionv2,self).__init__()
self.branch1=BasicConv2d(192,96,1,0)
self.branch2=nn.Sequential(
BasicConv2d(192,48,1,0),
BasicConv2d(48,64,3,1)
)
self.branch3=nn.Sequential(
BasicConv2d(192,64,1,0),
BasicConv2d(64,96,3,1),
BasicConv2d(96,96,3,1)
)
self.branch4=nn.Sequential(
nn.AvgPool2d(3,stride=1,padding=1,count_include_pad=False),
BasicConv2d(192,64,1,0)
)
def forward(self,x):
x0=self.branch1(x)
x1=self.branch2(x)
x2=self.branch3(x)
x3=self.branch4(x)
out=torch.cat((x0,x1,x2,x3),1)
return out
net_inceptionv2=Inceptionv2()
input=torch.randn(1,192,32,32)
print(net_inceptionv2(input).shape)
2.Resnet
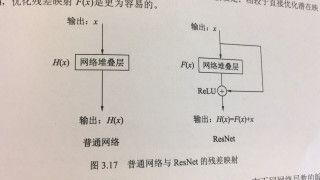
import torch.nn as nn
import torch
class Bottleneck(nn.Module):
def __init__(self,in_dim,out_dim,stride=1):
super(Bottleneck,self).__init__()
self.bottleneck=nn.Sequential(
nn.Conv2d(in_dim,in_dim,1,bias=False),
nn.BatchNorm2d(in_dim),
nn.ReLU(inplace=True),
nn.Conv2d(in_dim,in_dim,3,stride,1,bias=False),
nn.BatchNorm2d(in_dim),
nn.ReLU(inplace=True),
nn.Conv2d(in_dim,out_dim,1,bias=False),
nn.BatchNorm2d(out_dim)
)
self.relu=nn.ReLU(inplace=True)
self.downsample=nn.Sequential(
nn.Conv2d(in_dim,out_dim,1,1),
nn.BatchNorm2d(out_dim)
)
def forward(self,x):
identity=x
out=self.bottleneck(x)
identity=self.downsample(x)
out+=identity
out=self.relu(out)
return out
bottleneck_1_1=Bottleneck(64,256)
input=torch.randn(1,64,56,56)
print(bottleneck_1_1(input).shape)
3.DenseNet
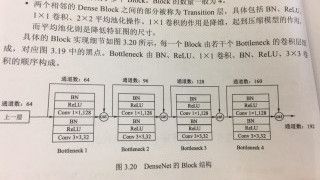
import torch
from torch import nn
import torch.nn.functional as F
class Bottleneck(nn.Module):
def __init__(self,nChannels,growthRate):
super(Bottleneck,self).__init__()
interChannels=4*growthRate
self.bn1=nn.BatchNorm2d(nChannels)
self.conv1=nn.Conv2d(nChannels,interChannels,kernel_size=1,bias=False)
self.bn2=nn.BatchNorm2d(interChannels)
self.conv2=nn.Conv2d(interChannels,growthRate,kernel_size=3,padding=1,bias=False)
def forward(self,x):
out=self.conv1(F.relu(self.bn1(x)))
out=self.conv2(F.relu(self.bn2(out)))
out=torch.cat((x,out),1)
return out
class Denseblock(nn.Module):
def __init__(self,nChannels,growthRate,nDenseBlocks):
super(Denseblock,self).__init__()
layers=[]
for i in range(int(nDenseBlocks)):
layers.append(Bottleneck(nChannels,growthRate))
nChannels+=growthRate
self.denseblock=nn.Sequential(*layers)
def forward(self,x):
return self.denseblock(x)
bottleneck=Bottleneck(64,32)
input=torch.randn(1,64,256,256)
print(bottleneck(input).shape)
4.FPN
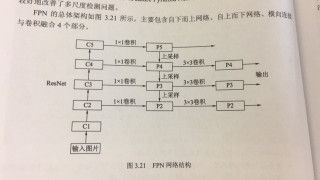
import torch.nn as nn
import torch.nn.functional as F
import math
import torch
class Bottleneck(nn.Module):
expansion = 4
def __init__(self,in_planes,planes,stride=1,downsample=None):
super(Bottleneck,self).__init__()
self.bottleneck=nn.Sequential(
nn.Conv2d(in_planes,planes,1,bias=False),
nn.BatchNorm2d(planes),
nn.ReLU(inplace=True),
nn.Conv2d(planes,planes,3,stride,1,bias=False),
nn.BatchNorm2d(planes),
nn.ReLU(inplace=True),
nn.Conv2d(planes,self.expansion*planes,1,bias=False),
nn.BatchNorm2d(self.expansion*planes),
)
self.relu=nn.ReLU(inplace=True)
self.downsample=downsample
def forward(self,x):
identity=x
out=self.bottleneck(x)
if self.downsample is not None:
identity=self.downsample(x)
out+=identity
out=self.relu(out)
return out
class FPN(nn.Module):
def __init__(self,layers):
super(FPN,self).__init__()
self.inplanes=64
self.conv1=nn.Conv2d(3,64,7,2,3,bias=False)
self.bn1=nn.BatchNorm2d(64)
self.relu=nn.ReLU(inplace=True)
self.maxpool=nn.MaxPool2d(3,2,1)
self.layer1=self.__make_layer(64,layers[0])
self.layer2=self.__make_layer(128,layers[1],2)
self.layer3=self.__make_layer(256,layers[2],2)
self.layer4=self.__make_layer(512,layers[3],2)
self.toplayer=nn.Conv2d(2048,256,1,1,0)
self.smooth1=nn.Conv2d(256,256,3,1,1)
self.latlayer1=nn.Conv2d(1024,256,1,1,0)
self.latlayer2=nn.Conv2d(512,256,1,1,0)
self.latlayer3=nn.Conv2d(256,256,1,1,0)
def __make_layer(self,planes,blocks,stride=1):
downsample=nn.Sequential(
nn.Conv2d(self.inplanes,Bottleneck.expansion*planes,1,stride,bias=False),
nn.BatchNorm2d(Bottleneck.expansion*planes)
)
layers=[]
layers.append(Bottleneck(self.inplanes,planes,stride,downsample))
self.inplanes=planes*Bottleneck.expansion
for i in range(1,blocks):
layers.append(Bottleneck(self.inplanes,planes))
return nn.Sequential(*layers)
def _upsample_add(self,x,y):
_,_,H,W=y.shape
return F.interpolate(x, size=(H, W), mode='bilinear', align_corners=True)+y
def forward(self,x):
c1=self.maxpool(self.relu(self.bn1(self.conv1(x))))
c2=self.layer1(c1)
c3=self.layer2(c2)
c4=self.layer3(c3)
c5=self.layer4(c4)
p5=self.toplayer(c5)
p4=self._upsample_add(p5,self.latlayer1(c4))
p3=self._upsample_add(p4,self.latlayer2(c3))
p2=self._upsample_add(p3,self.latlayer3(c2))
p4=self.smooth1(p4)
p3=self.smooth1(p3)
p2=self.smooth1(p2)
return p2,p3,p4,p5
input=torch.randn(1,3,224,224)
net_fpn=FPN([3,4,6,3])
print(net_fpn(input)[3].shape)
5.DetNet
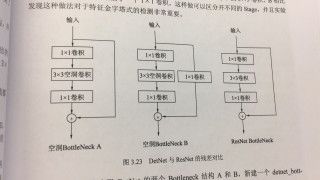
from torch import nn
import torch
class DetBottleneck(nn.Module):
def __init__(self, inplanes, planes, stride=1, extra=False):
super(DetBottleneck, self).__init__()
self.bottleneck = nn.Sequential(
nn.Conv2d(inplanes, planes, 1, bias=False),
nn.BatchNorm2d(planes),
nn.ReLU(inplace=True),
nn.Conv2d(planes, planes, kernel_size=3, stride=1, padding=2, dilation=2, bias=False),
nn.BatchNorm2d(planes),
nn.ReLU(inplace=True),
nn.Conv2d(planes, planes, 1, bias=False),
nn.BatchNorm2d(planes)
)
self.relu = nn.ReLU(inplace=True)
self.extra = extra
if self.extra:
self.extra_conv = nn.Sequential(
nn.Conv2d(inplanes, planes, 1, bias=False),
nn.BatchNorm2d(planes)
)
def forward(self, x):
if self.extra:
identity = self.extra_conv(x)
else:
identity = x
out = self.bottleneck(x)
out += identity
out = self.relu(out)
return out
bottleneck_b = DetBottleneck(1024, 256, 1, True)
print(bottleneck_b)
bottleneck_a1 = DetBottleneck(256, 256)
bottleneck_a2 = DetBottleneck(256, 256)
input = torch.randn(1, 1024, 14, 14)
output1 = bottleneck_b(input)
output2 = bottleneck_a1(output1)
output3 = bottleneck_a2(output2)
print(output1.shape)