1.对于要处理的文件集合会根据设定大小将文件分块,每个文件分成多块,不是把所有文件合并再根据大小分块,每个文件的最后一块都可能比设定的大小要小
块大小128m
a.txt 120m 1个块
b.txt 500m 4个块
reducetask的并行度
1.reducetask并行度就是将原来的一个大任务,分成多个小任务,每一个任务负责一部分计算数据。
2.reduce任务有几个,最直观的的显示就是结果文件的个数。一个结果文件对应于一个reducetask的执行结果。底层分reducetask任务的时候,是按照分区规则分的,每一个reducetask最终对应一个分区的数据。reducetsak的个数和用户设定的有关。
3.默认的分区partition算法:
public class HashPartitionerextends Partitioner { /** Use {@link Object#hashCode()} to partition. */ //K key map输出的lkey, V value map输出的value int numReduceTasks job.setNumReducetask(3) public int getPartition(K key, V value,int numReduceTasks(3)) { //key.hashCode() & Integer.MAX_VALUE 防止溢出 return (key.hashCode() & Integer.MAX_VALUE) % numReduceTasks; } } hash%reducetask的个数,假设分区三个,余数为0 1 2 分区3个 余数为0的分为一个区 相同的单词肯定会分到一个区中----启动reducetask1任务-------part-r-00000 余数为1的分为一个区-----启动reducetask2任务-----part-r-00001 余数为2的分为一个区-----启动reducetask3任务-----part-r-00002
自定义partition
import java.util.HashMap; import org.apache.hadoop.io.Text; import org.apache.hadoop.mapreduce.Partitioner; /** * 本类是提供给MapTask用的 * MapTask通过这个类的getPartition方法,来计算它所产生的每一对kv数据该分发给哪一个reduce task
* 例子应用场景是模拟按手机号前几位确定是哪里的手机号,模拟分为六个,job.setNumReducetask()建议设为6,
* 这样结果文件就分成六个,里面就是每个地区分别对应的手机号,不多不少 * @author ThinkPad * */ public class ProvincePartitioner extends Partitioner{ static HashMap codeMap = new HashMap<>(); static{ codeMap.put("135", 0); codeMap.put("136", 1); codeMap.put("137", 2); codeMap.put("138", 3); codeMap.put("139", 4); } @Override public int getPartition(Text key, FlowBean value, int numPartitions) { Integer code = codeMap.get(key.toString().substring(0, 3)); return code==null?5:code; } }
自定义partition步骤:
1)继承Partitioner
2)重写getPartition
3)Driver类中:
//添加自定义分区
job.setPartitionerClass(MyPartitioner.class);
// 这个参数如果不指定,默认reducetask 1
job.setNumReduceTasks(6);
5.自定义分区的时候:
最终启动几个reducetask任务?由job.setNumReducetask()设定
每个reducetask任务的数据分配是谁决定的?自定义的分区决定的。
自定义分区的个数是4个,reducetask可以是几个?1个或者大于等于4个。
注意:自定义分区的时候,分区编号从0开始,最好是连续的整数。如果不连续的话,job.setNumReducetask至少比最大的返回值+1,如果没有返回值的分区号 会返回空文件。
6.一个maptask或者reducetask只能在一个节点上运行,一个节点上可以运行多个maptask或者reducetask任务,这是yarn负责分配的
hadoop执行时加载参数
/** * 通过代码设置参数 */
Configuration conf = new Configuration(); conf.setInt("top.n", 3); /** * 通过eclipse的run configuration设置参数 或者 hadoop jar xx.jar xx.yy.JobSubmitter 3 */ conf.setInt("top.n", Integer.parseInt(args[0]));
/** * 通过属性配置文件获取参数 */
Configuration conf = new Configuration(); Properties props = new Properties(); props.load(JobSubmitter.class.getClassLoader().getResourceAsStream("topn.properties")); conf.setInt("top.n", Integer.parseInt(props.getProperty("top.n")));
topn.properties
top.n=5
/** * 通过加载classpath下的*.xml文件解析参数 */ Configuration conf = new Configuration(); conf.addResource("xx-oo.xml");
xx-oo.xml
<configuration> <property> <name>top.nname> <value>6value> property> configuration>
/** * 通过加载classpath下的*-site.xml文件解析参数 */ Configuration conf = new Configuration();
对象的引用
public class KengTest { public static void main(String[] args) throws FileNotFoundException, IOException { //ArrayListbeans = new ArrayList<>(); ObjectOutputStream oos = new ObjectOutputStream(new FileOutputStream("d:/keng.dat", true)); OrderBean bean = new OrderBean(); bean.set("1", "u", "a", 1.0f, 2); //beans.add(bean); oos.writeObject(bean); bean.set("2", "t", "b", 2.0f, 3); //beans.add(bean); oos.writeObject(bean); bean.set("3", "r", "c", 2.0f, 3); //beans.add(bean); oos.writeObject(bean); //System.out.println(beans); oos.close(); } }
输出三个"3", "r", "c"
原因:引用,覆盖了前面的
下面的代码不会出现上述问题
因为write方法有序列化过程,序列化后数据和这个对象就不关联了,数据被保存起来了
public static class OrderTopnMapper extends Mapper{ OrderBean orderBean = new OrderBean(); Text k = new Text(); @Override protected void map(LongWritable key, Text value, Mapper .Context context) throws IOException, InterruptedException { String[] fields = value.toString().split(","); orderBean.set(fields[0], fields[1], fields[2], Float.parseFloat(fields[3]), Integer.parseInt(fields[4])); k.set(fields[0]); // 从这里交给maptask的kv对象,会被maptask序列化后存储,所以不用担心覆盖的问题 context.write(k, orderBean); } }
reduce task提供的values迭代器,每次迭代返回给我们的都是同一个对象,只是set了不同的值
在使用迭代器时,构造一个新的对象,来存储本次迭代出来的值
public static class OrderTopnReducer extends Reducer{ @Override protected void reduce(Text key, Iterable values, Reducer .Context context) throws IOException, InterruptedException { // 获取topn的参数 int topn = context.getConfiguration().getInt("order.top.n",3); ArrayList beanList = new ArrayList<>(); // reduce task提供的values迭代器,每次迭代返回给我们的都是同一个对象,只是set了不同的值 for (OrderBean orderBean : values) { // 构造一个新的对象,来存储本次迭代出来的值 OrderBean newBean = new OrderBean(); newBean.set(orderBean.getOrderId(), orderBean.getUserId(), orderBean.getPdtName(),
orderBean.getPrice(), orderBean.getNumber()); beanList.add(newBean); } // 对beanList中的orderBean对象排序(按总金额大小倒序排序,如果总金额相同,则比商品名称) Collections.sort(beanList); for (int i=0;i) { context.write(beanList.get(i), NullWritable.get()); } } }
每次的对象都是新的,和之前不一样
mapreduce编程模型--及hadoop中的具体实现框架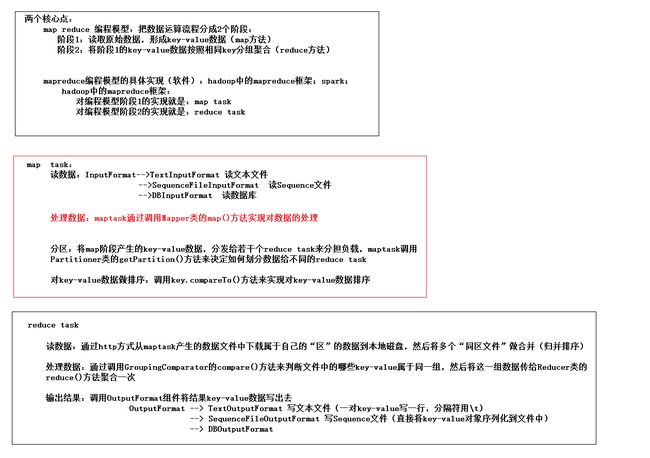
源码 Mapper.class
Mapper调用run()
启动时只执行一遍setup
后面循环遍历key value 执行map()
最后执行cleanup()