文章目录
- 1.条件筛选
- 1.1 单条件筛选
- 1.2 多条件筛选
- 1.3 排除特定行
- 2. 索引筛选
- 2.1 切片操作
- 2.2 loc函数
- 2.3 iloc
- 2.4 ix函数
- 2.5 at函数
- 2.6 iat函数
众所周知
pandas
的
DataFrame
数据结构提供了功能强大的数据操作功能,例如运算,筛选,统计等。
今天我们就来谈一谈其强大的数据筛选功能,主要包括两大类,
按照条件筛选和
按照索引筛选。可以
对行进行筛选,也可以
按照列进行筛选。
import numpy as np
import pandas as pd
df = pd.DataFrame({"a": list(range(1,10,1)),"b": list(range(2,11,1)),"c": list(range(3,12,1))})
df
|
a |
b |
c |
0 |
1 |
2 |
3 |
1 |
2 |
3 |
4 |
2 |
3 |
4 |
5 |
3 |
4 |
5 |
6 |
4 |
5 |
6 |
7 |
5 |
6 |
7 |
8 |
6 |
7 |
8 |
9 |
7 |
8 |
9 |
10 |
8 |
9 |
10 |
11 |
1.条件筛选
1.1 单条件筛选
df[df['a']>3]
|
a |
b |
c |
3 |
4 |
5 |
6 |
4 |
5 |
6 |
7 |
5 |
6 |
7 |
8 |
6 |
7 |
8 |
9 |
7 |
8 |
9 |
10 |
8 |
9 |
10 |
11 |
- 如果想筛选a列的取值大于3的记录,但是只显示满足条件的b,c列的值可以这么写
df[['b','c']][df['a']>3]
|
b |
c |
3 |
5 |
6 |
4 |
6 |
7 |
5 |
7 |
8 |
6 |
8 |
9 |
7 |
9 |
10 |
8 |
10 |
11 |
- 使用
isin
函数根据特定值筛选记录。筛选a值等于3或者5的记录
df[df.a.isin([3, 5])]
1.2 多条件筛选
可以使用&(并)
与|(或)
操作符或者特定的函数实现多条件筛选
- 使用
&
筛选a列的取值大于3,b列的取值大于6的记录
df[(df['a'] > 3) & (df['b'] > 6)]
|
a |
b |
c |
5 |
6 |
7 |
8 |
6 |
7 |
8 |
9 |
7 |
8 |
9 |
10 |
8 |
9 |
10 |
11 |
- 使用
numpy
的logical_and
函数完成同样的功能
df[np.logical_and(df['a']> 3,df['b']>6)]
|
a |
b |
c |
5 |
6 |
7 |
8 |
6 |
7 |
8 |
9 |
7 |
8 |
9 |
10 |
8 |
9 |
10 |
11 |
1.3 排除特定行
筛选特定行做起来很方便,可以使用特定的函数完成,但是排除含特定值的行就需要做一些变通了。
例如,我们选出a列的值不等于3或者5的记录。基本的做法是将a列选择出来,把值3和5剔除,再使用isin
函数。
ex_list = list(df['a'])
ex_list.remove(3)
ex_list.remove(5)
ex_list
[1, 2, 4, 6, 7, 8, 9]
df[df.a.isin(ex_list)]
|
a |
b |
c |
0 |
1 |
2 |
3 |
1 |
2 |
3 |
4 |
3 |
4 |
5 |
6 |
5 |
6 |
7 |
8 |
6 |
7 |
8 |
9 |
7 |
8 |
9 |
10 |
8 |
9 |
10 |
11 |
2. 索引筛选
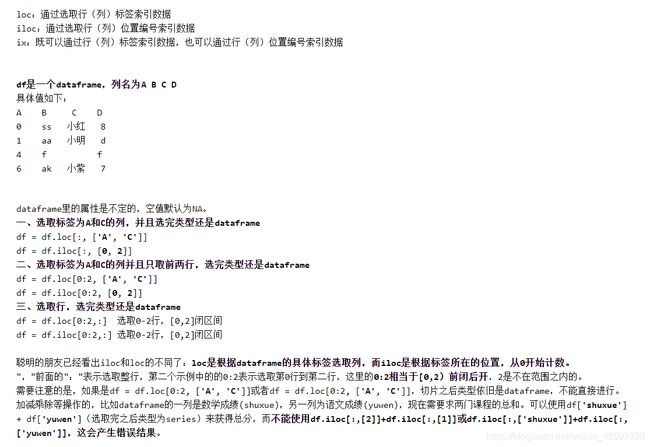
2.1 切片操作
df[1:4]
|
a |
b |
c |
1 |
2 |
3 |
4 |
2 |
3 |
4 |
5 |
3 |
4 |
5 |
6 |
df[['a','c']]
|
a |
c |
0 |
1 |
3 |
1 |
2 |
4 |
2 |
3 |
5 |
3 |
4 |
6 |
4 |
5 |
7 |
5 |
6 |
8 |
6 |
7 |
9 |
7 |
8 |
10 |
8 |
9 |
11 |
2.2 loc函数
当每列已有column name时,用 df ['a']
就能选取出一整列数据。如果你知道column names和index(这里df的index没有指定,是默认生成的下标),且两者都很好输入,可以选择.loc
同时进行行列选择。
df.loc[0,'c']
3
df.loc[1:4,['a','c']]
|
a |
c |
1 |
2 |
4 |
2 |
3 |
5 |
3 |
4 |
6 |
4 |
5 |
7 |
df.loc[[1,3,5],['a','c']]
2.3 iloc
如果column name
太长,输入不方便,或者index
是一列时间序列,更不好输入,那就可以选择 .iloc
了,该方法接受列名的index,iloc
使得我们可以对column
使用slice
(切片)的方法对数据进行选取。这边的 i 我觉得代表index,比较好记点。
df.iloc[0,2]
3
df.iloc[1:4,[0,2]]
df.iloc[[1,3,5],[0,2]]
df.iloc[[1,3,5],0:2]
2.4 ix函数
ix的功能更加强大,参数既可以是索引,也可以是名称,相当于,loc
和iloc
的合体。需要注意的是在使用的时候需要统一,在行选择时同时出现索引和名称, 同样在同行选择时同时出现索引和名称。
df.ix[1:3,['a','b']]
e:\anaconda3.5\lib\site-packages\ipykernel_launcher.py:1: DeprecationWarning:
.ix is deprecated. Please use
.loc for label based indexing or
.iloc for positional indexing
See the documentation here:
http://pandas.pydata.org/pandas-docs/stable/indexing.html#ix-indexer-is-deprecated
"""Entry point for launching an IPython kernel.
df.ix[[1,3,5],['a','b']]
df.ix[[1,3,5],[0,2]]
2.5 at函数
根据指定行index及列label,快速定位DataFrame的元素,选择列时仅支持列名
df.at[3,'a']
4
2.6 iat函数
与at的功能相同,只使用索引参数。
df.iat[3,0]
4
df.iat[3,'a']
---------------------------------------------------------------------------
ValueError Traceback (most recent call last)
in
----> 1 df.iat[3,'a']
e:\anaconda3.5\lib\site-packages\pandas\core\indexing.py in __getitem__(self, key)
1783 raise ValueError('Invalid call for scalar access (getting)!')
1784
-> 1785 key = self._convert_key(key)
1786 return self.obj.get_value(*key, takeable=self._takeable)
1787
e:\anaconda3.5\lib\site-packages\pandas\core\indexing.py in _convert_key(self, key, is_setter)
1852 for a, i in zip(self.obj.axes, key):
1853 if not is_integer(i):
-> 1854 raise ValueError("iAt based indexing can only have integer "
1855 "indexers")
1856 return key
ValueError: iAt based indexing can only have integer indexers