Pandas 11-综合练习
import pandas as pd
import numpy as np
np.seterr(all = 'ignore')
{'divide': 'ignore', 'over': 'ignore', 'under': 'ignore', 'invalid': 'ignore'}
【任务一】企业收入的多样性
【题目描述】一个企业的产业收入多样性可以仿照信息熵的概念来定义收入熵指标 :
I = − ∑ i p ( x i ) l o g ( p ( x i ) ) I=-\sum_i{p(x_i)log(p(x_i))} I=−i∑p(xi)log(p(xi))
其中 p ( x i ) p(x_i) p(xi)是企业该年某产业收入额占该年所有产业总收入的比重。
在company.csv
中存有需要计算的企业和年份 , 在company_data.csv
中存有企业、各类收入额和收入年份的信息。现请利用后一张表中的数据 , 在前一张表中增加一列表示该公司该年份的收入熵指标I
。
【数据下载】链接:https://pan.baidu.com/s/1leZZctxMUSW55kZY5WwgIw 53 密码:u6fd
My solution :
df1 = pd.read_csv('company.csv')
df2 = pd.read_csv('company_data.csv')
df1.head()
|
证券代码 |
日期 |
0 |
#000007 |
2014 |
1 |
#000403 |
2015 |
2 |
#000408 |
2016 |
3 |
#000408 |
2017 |
4 |
#000426 |
2015 |
df2.head()
|
证券代码 |
日期 |
收入类型 |
收入额 |
0 |
1 |
2008/12/31 |
1 |
1.084218e+10 |
1 |
1 |
2008/12/31 |
2 |
1.259789e+10 |
2 |
1 |
2008/12/31 |
3 |
1.451312e+10 |
3 |
1 |
2008/12/31 |
4 |
1.063843e+09 |
4 |
1 |
2008/12/31 |
5 |
8.513880e+08 |
- 经观察两表的证券代码列和日期格式都不一致 , 因当首先变一致
- 将
df1
表中证券代码
列里的#
去掉转为int
- 将
df2
表日期
列取前四位year
转为int
df1_ = df1.copy()
df1_['证券代码'] = df1_['证券代码'].str[1:].astype('int64')
df2['日期'] = df2['日期'].str[:4].astype('int64')
- 定义
entropy
函数计算信息熵 , 并跳过NaN
值
- 用
df1
表左连接df2
表 , 连接列为证券代码和日期 , 再继续对这两列分组 , 取出收入额列用apply
调用信息熵函数 , 重置索引
def entropy(x):
if x.any():
p = x/x.sum()
return -(p*np.log2(p)).sum()
return np.nan
res = df1_.merge(df2, on=['证券代码','日期'], how='left').groupby(['证券代码','日期'])['收入额'].apply(entropy).reset_index()
res.head()
|
证券代码 |
日期 |
收入额 |
0 |
7 |
2014 |
4.429740 |
1 |
403 |
2015 |
4.025963 |
2 |
408 |
2016 |
4.066295 |
3 |
408 |
2017 |
NaN |
4 |
426 |
2015 |
4.449655 |
- 将
df1
表新增一列收入熵指标 , 值为结果表中的收入额
df1['收入熵指标'] = res['收入额']
df1
|
证券代码 |
日期 |
收入熵指标 |
0 |
#000007 |
2014 |
4.429740 |
1 |
#000403 |
2015 |
4.025963 |
2 |
#000408 |
2016 |
4.066295 |
3 |
#000408 |
2017 |
NaN |
4 |
#000426 |
2015 |
4.449655 |
... |
... |
... |
... |
1043 |
#600978 |
2011 |
4.788391 |
1044 |
#600978 |
2014 |
4.022378 |
1045 |
#600978 |
2015 |
4.346303 |
1046 |
#600978 |
2016 |
4.358608 |
1047 |
#600978 |
2017 |
NaN |
1048 rows × 3 columns
def information_entropy():
df1 = pd.read_csv('company.csv')
df2 = pd.read_csv('company_data.csv')
df1_ = df1.copy()
df1_['证券代码'] = df1_['证券代码'].str[1:].astype('int64')
df2['日期'] = df2['日期'].str[:4].astype('int64')
res = df1_.merge(df2, on=['证券代码','日期'], how='left').groupby(['证券代码','日期'])['收入额'].apply(entropy).reset_index()
df1['收入熵指标'] = res['收入额']
return df1
%timeit -n 5 information_entropy()
1.62 s ± 44.5 ms per loop (mean ± std. dev. of 7 runs, 5 loops each)
【任务二】组队学习信息表的变换
【题目描述】请把组队学习的队伍信息表变换为如下形态,其中'是否队长'
一列取1
表示队长,否则为0
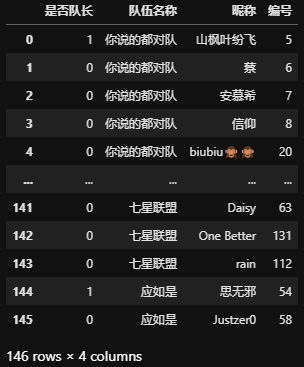
【数据下载】链接:https://pan.baidu.com/s/1ses24cTwUCbMx3rvYXaz-Q 34 密码:iz57
My solution :
df = pd.read_excel('组队信息汇总表_Pandas.xlsx')
df.drop(columns='所在群', inplace=True)
df.head(2)
|
队伍名称 |
队长编号 |
队长_群昵称 |
队员1 编号 |
队员_群昵称 |
队员2 编号 |
队员_群昵称.1 |
队员3 编号 |
队员_群昵称.2 |
队员4 编号 |
... |
队员6 编号 |
队员_群昵称.5 |
队员7 编号 |
队员_群昵称.6 |
队员8 编号 |
队员_群昵称.7 |
队员9 编号 |
队员_群昵称.8 |
队员10编号 |
队员_群昵称.9 |
0 |
你说的都对队 |
5 |
山枫叶纷飞 |
6 |
蔡 |
7.0 |
安慕希 |
8.0 |
信仰 |
20.0 |
... |
NaN |
NaN |
NaN |
NaN |
NaN |
NaN |
NaN |
NaN |
NaN |
NaN |
1 |
熊猫人 |
175 |
鱼呲呲 |
44 |
Heaven |
37.0 |
吕青 |
50.0 |
余柳成荫 |
82.0 |
... |
25.0 |
Never say never |
55.0 |
K |
120.0 |
Y. |
28.0 |
X.Y.Q |
151.0 |
swrong |
2 rows × 23 columns
- 为了使用
wide_to_long
将宽表转长表 ,需要先对表columns
进行重命名
- 对照结果表中的名字 , 分别将队长和队员用
leader
和member
区分 , 结果表中队长和队员分别用1
和0
分类 , 不妨在重命名时就先分好类 , 在重命名的末尾追加1
和0
,最后直接取出字符串最后一位即可
col_1 = np.array(['队伍名称','编号_leader01','昵称_leader01'])
col_2 = np.array([[f'编号_member{i}0', f'昵称_member{i}0']for i in range(1,11)]).flatten()
df.columns = np.r_[col_1,col_2]
df.head(2)
|
队伍名称 |
编号_leader01 |
昵称_leader01 |
编号_member10 |
昵称_member10 |
编号_member20 |
昵称_member20 |
编号_member30 |
昵称_member30 |
编号_member40 |
... |
编号_member60 |
昵称_member60 |
编号_member70 |
昵称_member70 |
编号_member80 |
昵称_member80 |
编号_member90 |
昵称_member90 |
编号_member100 |
昵称_member100 |
0 |
你说的都对队 |
5 |
山枫叶纷飞 |
6 |
蔡 |
7.0 |
安慕希 |
8.0 |
信仰 |
20.0 |
... |
NaN |
NaN |
NaN |
NaN |
NaN |
NaN |
NaN |
NaN |
NaN |
NaN |
1 |
熊猫人 |
175 |
鱼呲呲 |
44 |
Heaven |
37.0 |
吕青 |
50.0 |
余柳成荫 |
82.0 |
... |
25.0 |
Never say never |
55.0 |
K |
120.0 |
Y. |
28.0 |
X.Y.Q |
151.0 |
swrong |
2 rows × 23 columns
- 将重命名好的表用
wide_to_long
转换为长表 , 命名对照结果表 , 省的还要再重命名
- 转换后
dropna
删除NaN
值 , 恢复索引
res = pd.wide_to_long( df.reset_index(),
stubnames = ['昵称','编号'],
i = ['index','队伍名称'],
j = '是否队长',
sep = '_',
suffix = '.+').dropna().reset_index().drop(columns='index')
res
|
队伍名称 |
是否队长 |
昵称 |
编号 |
0 |
你说的都对队 |
leader01 |
山枫叶纷飞 |
5.0 |
1 |
你说的都对队 |
member10 |
蔡 |
6.0 |
2 |
你说的都对队 |
member20 |
安慕希 |
7.0 |
3 |
你说的都对队 |
member30 |
信仰 |
8.0 |
4 |
你说的都对队 |
member40 |
biubiu |
20.0 |
... |
... |
... |
... |
... |
141 |
七星联盟 |
member40 |
Daisy |
63.0 |
142 |
七星联盟 |
member50 |
One Better |
131.0 |
143 |
七星联盟 |
member60 |
rain |
112.0 |
144 |
应如是 |
leader01 |
思无邪 |
54.0 |
145 |
应如是 |
member10 |
Justzer0 |
58.0 |
146 rows × 4 columns
- 到这里已经接近结果了 , 把是否队长一列的值最后一个取出最为该列的分类
- 编号列的类型为
float
转为int
- 是否队长和队伍名称两列顺序倒了 , 恢复一下即可
res['是否队长'],res['编号'] = res['是否队长'].str[-1],res['编号'].astype('int64')
res.reindex(columns=['是否队长','队伍名称','昵称','编号'])
|
是否队长 |
队伍名称 |
昵称 |
编号 |
0 |
1 |
你说的都对队 |
山枫叶纷飞 |
5 |
1 |
0 |
你说的都对队 |
蔡 |
6 |
2 |
0 |
你说的都对队 |
安慕希 |
7 |
3 |
0 |
你说的都对队 |
信仰 |
8 |
4 |
0 |
你说的都对队 |
biubiu |
20 |
... |
... |
... |
... |
... |
141 |
0 |
七星联盟 |
Daisy |
63 |
142 |
0 |
七星联盟 |
One Better |
131 |
143 |
0 |
七星联盟 |
rain |
112 |
144 |
1 |
应如是 |
思无邪 |
54 |
145 |
0 |
应如是 |
Justzer0 |
58 |
146 rows × 4 columns
def transform_table():
df = pd.read_excel('组队信息汇总表_Pandas.xlsx')
df.drop(columns='所在群', inplace=True)
col_1 = np.array(['队伍名称','编号_leader01','昵称_leader01'])
col_2 = np.array([[f'编号_member{i}0', f'昵称_member{i}0']for i in range(1,11)]).flatten()
df.columns = np.r_[col_1,col_2]
res = pd.wide_to_long( df.reset_index(),
stubnames = ['昵称','编号'],
i = ['index','队伍名称'],
j = '是否队长',
sep = '_',
suffix = '.+').dropna().reset_index().drop(columns='index')
res['是否队长'], res['编号'] = res['是否队长'].str[-1], res['编号'].astype('int64')
res.reindex(columns=['是否队长','队伍名称','昵称','编号'])
%timeit -n 50 transform_table()
45.7 ms ± 3 ms per loop (mean ± std. dev. of 7 runs, 50 loops each)
【任务三】美国大选投票情况
【题目描述】两张数据表中分别给出了美国各县(county
)的人口数以及大选的投票情况 , 请解决以下问题 :
- 有多少县满足总投票数超过县人口数的一半
- 把州(
state
)作为行索引 , 把投票候选人作为列名 , 列名的顺序按照候选人在全美的总票数由高到低排序 , 行列对应的元素为该候选人在该州获得的总票数
此处是一个样例,实际的州或人名用原表的英语代替
拜登 川普
威斯康星州 2 1
德克萨斯州 3 4
- 每一个州下设若干县 , 定义拜登在该县的得票率减去川普在该县的得票率为该县的
BT
指标 , 若某个州所有县BT
指标的中位数大于0
, 则称该州为Biden State
, 请找出所有的Biden State
【数据下载】链接:https://pan.baidu.com/s/182rr3CpstVux2CFdFd_Pcg 32 提取码:q674
My solution :
df1 = pd.read_csv('president_county_candidate.csv')
df2 = pd.read_csv('county_population.csv')
df1.head()
|
state |
county |
candidate |
party |
total_votes |
won |
0 |
Delaware |
Kent County |
Joe Biden |
DEM |
44552 |
True |
1 |
Delaware |
Kent County |
Donald Trump |
REP |
41009 |
False |
2 |
Delaware |
Kent County |
Jo Jorgensen |
LIB |
1044 |
False |
3 |
Delaware |
Kent County |
Howie Hawkins |
GRN |
420 |
False |
4 |
Delaware |
New Castle County |
Joe Biden |
DEM |
195034 |
True |
df2.head()
|
US County |
Population |
0 |
.Autauga County, Alabama |
55869 |
1 |
.Baldwin County, Alabama |
223234 |
2 |
.Barbour County, Alabama |
24686 |
3 |
.Bibb County, Alabama |
22394 |
4 |
.Blount County, Alabama |
57826 |
- 为了后续分组或合并操作 , 先统一
state
和county
列名和值
- 将
df2
中US County
按,
拆分 , 注意逗号后还有个空格 , 否则拆分后值并不相同
df2[['county','state']] = pd.DataFrame([*df2['US County'].str.split(', ')])
df2.county = df2.county.str[1:]
df2.drop(columns='US County', inplace=True)
df2.head()
|
Population |
county |
state |
0 |
55869 |
Autauga County |
Alabama |
1 |
223234 |
Baldwin County |
Alabama |
2 |
24686 |
Barbour County |
Alabama |
3 |
22394 |
Bibb County |
Alabama |
4 |
57826 |
Blount County |
Alabama |
1. 有多少县满足总投票数超过县人口数的一半 ?
- 对
df1
按state
和county
分组 , 求和计算每个county
总票数
- 再与
df2
按state
和county
两列merge
, 将Population
转移过来
df_merge = df1.groupby(['state','county'])['total_votes'].sum().reset_index().merge(df2, on=['state','county'], how='left')
df_merge.head()
|
state |
county |
total_votes |
Population |
0 |
Alabama |
Autauga County |
27770 |
55869.0 |
1 |
Alabama |
Baldwin County |
109679 |
223234.0 |
2 |
Alabama |
Barbour County |
10518 |
24686.0 |
3 |
Alabama |
Bibb County |
9595 |
22394.0 |
4 |
Alabama |
Blount County |
27588 |
57826.0 |
- 对上述结果取出
total_votes
与Population
作比较筛选出即可
df_merge[df_merge['total_votes'] > 0.5*df_merge['Population']]
|
state |
county |
total_votes |
Population |
11 |
Alabama |
Choctaw County |
7464 |
12589.0 |
12 |
Alabama |
Clarke County |
13135 |
23622.0 |
13 |
Alabama |
Clay County |
6930 |
13235.0 |
16 |
Alabama |
Colbert County |
27886 |
55241.0 |
17 |
Alabama |
Conecuh County |
6441 |
12067.0 |
... |
... |
... |
... |
... |
4626 |
Wyoming |
Sheridan County |
16428 |
30485.0 |
4627 |
Wyoming |
Sublette County |
4970 |
9831.0 |
4629 |
Wyoming |
Teton County |
14677 |
23464.0 |
4631 |
Wyoming |
Washakie County |
4012 |
7805.0 |
4632 |
Wyoming |
Weston County |
3542 |
6927.0 |
1434 rows × 4 columns
2. 把州(state
)作为行索引 , 把投票候选人作为列名 , 列名的顺序按照候选人在全美的总票数由高到低排序 , 行列对应的元素为该候选人在该州获得的总票数
- 依题意可以用
pivot_table
透视 , 填入行和列 , 对同一位置用sum
聚合 , 打开margins
汇总 , 对最后一行All
降序排列
- 可以看到第一列是每行的汇总 , 也就是每个
state
的汇总 , 第二列是Biden
最高票 , Trump
紧随其后
df1.pivot_table(values = ['total_votes'],
index = ['state'],
columns = 'candidate',
aggfunc = 'sum',
margins = True).sort_values('All', 1, ascending=False).head()
|
total_votes |
candidate |
All |
Joe Biden |
Donald Trump |
Jo Jorgensen |
Howie Hawkins |
Write-ins |
Rocky De La Fuente |
Gloria La Riva |
Kanye West |
Don Blankenship |
... |
Tom Hoefling |
Ricki Sue King |
Princess Jacob-Fambro |
Blake Huber |
Richard Duncan |
Joseph Kishore |
Jordan Scott |
Gary Swing |
Keith McCormic |
Zachary Scalf |
state |
|
|
|
|
|
|
|
|
|
|
|
|
|
|
|
|
|
|
|
|
|
Alabama |
2323304 |
849648.0 |
1441168.0 |
25176.0 |
NaN |
7312.0 |
NaN |
NaN |
NaN |
NaN |
... |
NaN |
NaN |
NaN |
NaN |
NaN |
NaN |
NaN |
NaN |
NaN |
NaN |
Alaska |
391346 |
153405.0 |
189892.0 |
8896.0 |
NaN |
34210.0 |
318.0 |
NaN |
NaN |
1127.0 |
... |
NaN |
NaN |
NaN |
NaN |
NaN |
NaN |
NaN |
NaN |
NaN |
NaN |
Arizona |
3387326 |
1672143.0 |
1661686.0 |
51465.0 |
NaN |
2032.0 |
NaN |
NaN |
NaN |
NaN |
... |
NaN |
NaN |
NaN |
NaN |
NaN |
NaN |
NaN |
NaN |
NaN |
NaN |
Arkansas |
1219069 |
423932.0 |
760647.0 |
13133.0 |
2980.0 |
NaN |
1321.0 |
1336.0 |
4099.0 |
2108.0 |
... |
NaN |
NaN |
NaN |
NaN |
NaN |
NaN |
NaN |
NaN |
NaN |
NaN |
California |
17495906 |
11109764.0 |
6005961.0 |
187885.0 |
81025.0 |
80.0 |
60155.0 |
51036.0 |
NaN |
NaN |
... |
NaN |
NaN |
NaN |
NaN |
NaN |
NaN |
NaN |
NaN |
NaN |
NaN |
5 rows × 39 columns
3. 每一个州下设若干县 , 定义拜登在该县的得票率减去川普在该县的得票率为该县的BT
指标 , 若某个州所有县BT
指标的中位数大于0
, 则称该州为Biden State
, 请找出所有的Biden State
方法一 :
- 定义一个计算
BT
指标的函数 , 分别取出Biden
的票数 , Trump
的票数 , 计算每个county
总票数 , 做差相除得到BT
- 对
state
和county
分组 , 取出candidate
和total_votes
两列调用apply
计算BT
def BT(x):
biden = x[x['candidate']=='Joe Biden']['total_votes'].values
trump = x[x['candidate']=='Donald Trump']['total_votes'].values
return pd.Series((biden-trump)/x['total_votes'].sum(), index=['BT'])
bt = df1.groupby(['state','county'])[['candidate','total_votes']].apply(BT)
bt.head()
|
|
BT |
state |
county |
|
Alabama |
Autauga County |
-0.444184 |
Baldwin County |
-0.537623 |
Barbour County |
-0.076631 |
Bibb County |
-0.577280 |
Blount County |
-0.800022 |
- 将
bt
结果恢复索引重新对state
分组 , 用filter
过滤每个state
下county
的BT
指标中位数是否大于0
- 对
state
去重后即满足条件的所有state
, 只有9
个
bt.reset_index().groupby('state').filter(lambda x:x.BT.median()>0)[['state']].drop_duplicates()
|
state |
197 |
California |
319 |
Connecticut |
488 |
Delaware |
491 |
District of Columbia |
725 |
Hawaii |
1878 |
Massachusetts |
2999 |
New Jersey |
3536 |
Rhode Island |
4065 |
Vermont |
方法二 :
- 分别用
bool
条件取出biden
和trump
的所有行 , 再对state
和county
分组求出每个county
的总票数
- 这三个
df
巧了都是一样的大小 , 说明每个county
都有biden
和trump
的票
biden_df = df1[df1['candidate']=='Joe Biden'][['state','county','total_votes']]
trump_df = df1[df1['candidate']=='Donald Trump'][['state','county','total_votes']]
sum_df = df1.groupby(['state','county'])[['total_votes']].sum().reset_index()
res = biden_df.merge(trump_df, on=['state','county'] ,suffixes=('_biden','_trump')).merge(sum_df, on=['state','county'])
res.head()
|
state |
county |
total_votes_biden |
total_votes_trump |
total_votes |
0 |
Delaware |
Kent County |
44552 |
41009 |
87025 |
1 |
Delaware |
New Castle County |
195034 |
88364 |
287633 |
2 |
Delaware |
Sussex County |
56682 |
71230 |
129352 |
3 |
District of Columbia |
District of Columbia |
39041 |
1725 |
41681 |
4 |
District of Columbia |
Ward 2 |
29078 |
2918 |
32881 |
- 分别取出
biden
列和trump
列做差后除以sum
列得出BT
指标
res['BT'] = (res['total_votes_biden']-res['total_votes_trump'])/res['total_votes']
res.head()
|
state |
county |
total_votes_biden |
total_votes_trump |
total_votes |
BT |
0 |
Delaware |
Kent County |
44552 |
41009 |
87025 |
0.040712 |
1 |
Delaware |
New Castle County |
195034 |
88364 |
287633 |
0.370855 |
2 |
Delaware |
Sussex County |
56682 |
71230 |
129352 |
-0.112468 |
3 |
District of Columbia |
District of Columbia |
39041 |
1725 |
41681 |
0.895276 |
4 |
District of Columbia |
Ward 2 |
29078 |
2918 |
32881 |
0.795596 |
- 同样的 , 按要求过滤后取出所有满足条件的
state
, 也是9
个
res[['state','BT']].groupby('state').filter(lambda x:x.median()>0)[['state']].drop_duplicates()
|
state |
0 |
Delaware |
3 |
District of Columbia |
237 |
Hawaii |
1390 |
Massachusetts |
2511 |
New Jersey |
3048 |
Rhode Island |
3577 |
Vermont |
4327 |
California |
4449 |
Connecticut |
def method1():
bt = df1.groupby(['state','county'])[['candidate','total_votes']].apply(BT)
bt.reset_index().groupby('state').filter(lambda x:x.BT.median()>0)[['state']].drop_duplicates()
def method2():
biden_df = df1[df1['candidate']=='Joe Biden'][['state','county','total_votes']]
trump_df = df1[df1['candidate']=='Donald Trump'][['state','county','total_votes']]
sum_df = df1.groupby(['state','county'])[['total_votes']].sum().reset_index()
res = biden_df.merge(trump_df, on=['state','county'] ,suffixes=('_biden','_trump')).merge(sum_df, on=['state','county'])
res['BT'] = (res['total_votes_biden']-res['total_votes_trump'])/res['total_votes']
res[['state','BT']].groupby('state').filter(lambda x:x.median()>0)[['state']].drop_duplicates()
%timeit method1()
6.56 s ± 210 ms per loop (mean ± std. dev. of 7 runs, 1 loop each)
%timeit method2()
90.9 ms ± 4.83 ms per loop (mean ± std. dev. of 7 runs, 10 loops each)
- 可以看到方法二虽然拆分好多步骤 , 但是没有用
apply
调用自定义函数 , 性能强到飞起
【任务四】计算城市间的距离矩阵
【题目描述】数据中给出了若干城市的经纬度,请构造关于城市的距离DataFrame
,其横纵索引为城市名称 , 值为矩阵 M M M , M i j M_ij Mij表示城市i
与城市j
间的球面距离(可以利用geopy
包中distance
模块的geodesic
函数),并规定与自身的距离为0
。
My solution :
df = pd.read_table('map.txt', sep=',', names=['city1','longitude','latitude'], skiprows=1)
df.head()
|
city1 |
longitude |
latitude |
0 |
沈阳市 |
123.429092 |
41.796768 |
1 |
长春市 |
125.324501 |
43.886841 |
2 |
哈尔滨市 |
126.642464 |
45.756966 |
3 |
北京市 |
116.405289 |
39.904987 |
4 |
天津市 |
117.190186 |
39.125595 |
- 将纬度和经度打包成元组供后面计算距离用
- 删除原来的两列经纬度
df['coord1'] = pd.Series([*zip(df.latitude, df.longitude)])
df.drop(columns=['longitude','latitude'], inplace=True)
df.head(3)
|
city1 |
coord1 |
0 |
沈阳市 |
(41.796768, 123.429092) |
1 |
长春市 |
(43.886841, 125.324501) |
2 |
哈尔滨市 |
(45.756966, 126.64246399999999) |
- 复制一份
df
表 , 并重命名列名加以区分 , 并设置city2
为index
, 为后续透视表做准备
df2 = df.rename(columns={
'city1':'city2','coord1':'coord2'}).set_index('city2')
df2.head(3)
|
coord2 |
city2 |
|
沈阳市 |
(41.796768, 123.429092) |
长春市 |
(43.886841, 125.324501) |
哈尔滨市 |
(45.756966, 126.64246399999999) |
- 将
df
和df2
扩展 , 先借用groupby
对df
两列分组 , 看似分了个寂寞 , 实则用apply
将df2
一个一个拼上去了 , 将原表在索引里的坐标coord1
恢复到数据列 , 用stack
把列移下来做一个reshape
, 再重置索引 , 将空列名起个名字coords
, 这一列都是坐标了 , 为后续透视表做完了准备
df_expand = df.groupby(['city1','coord1']).apply(lambda x:df2).reset_index(1).stack().reset_index().rename(columns={
0:'coords'})
df_expand.head(3)
|
city1 |
city2 |
level_2 |
coords |
0 |
上海市 |
沈阳市 |
coord1 |
(31.231707, 121.472641) |
1 |
上海市 |
沈阳市 |
coord2 |
(41.796768, 123.429092) |
2 |
上海市 |
长春市 |
coord1 |
(31.231707, 121.472641) |
- 导入计算距离的函数
geodesic
- 将上述准备好的表进行透视 , 并对透视结果坐标列用
geodesic
计算距离 , 用km
做单位 , 再保留两位小数
from geopy.distance import geodesic
df_expand.pivot_table(values = 'coords',
index = 'city1',
columns = 'city2',
aggfunc = lambda x : geodesic(*x).km
).round(2).head(3)
city2 |
上海市 |
乌鲁木齐市 |
兰州市 |
北京市 |
南京市 |
南宁市 |
南昌市 |
台北市 |
合肥市 |
呼和浩特市 |
... |
福州市 |
西宁市 |
西安市 |
贵阳市 |
郑州市 |
重庆市 |
银川市 |
长春市 |
长沙市 |
香港 |
city1 |
|
|
|
|
|
|
|
|
|
|
|
|
|
|
|
|
|
|
|
|
|
上海市 |
0.00 |
3272.69 |
1718.73 |
1065.83 |
271.87 |
1601.34 |
608.49 |
687.22 |
403.87 |
1378.10 |
... |
609.42 |
1912.40 |
1220.03 |
1527.44 |
827.47 |
1449.73 |
1606.18 |
1444.77 |
887.53 |
1229.26 |
乌鲁木齐市 |
3272.69 |
0.00 |
1627.85 |
2416.78 |
3010.73 |
3005.60 |
3023.06 |
3708.72 |
2907.56 |
2009.15 |
... |
3466.84 |
1443.79 |
2120.86 |
2572.82 |
2448.76 |
2305.96 |
1666.74 |
3004.86 |
2850.22 |
3415.02 |
兰州市 |
1718.73 |
1627.85 |
0.00 |
1182.61 |
1447.27 |
1529.94 |
1397.55 |
2085.95 |
1326.02 |
870.72 |
... |
1841.48 |
194.64 |
506.74 |
1086.41 |
904.20 |
765.89 |
343.02 |
2023.00 |
1226.07 |
1826.19 |
3 rows × 34 columns
def calculate_M():
df = pd.read_table('map.txt', sep=',', names=['city1','longitude','latitude'], skiprows=1)
df['coord1'] = pd.Series([*zip(df.latitude, df.longitude)])
df.drop(columns=['longitude','latitude'], inplace=True)
df2 = df.rename(columns={
'city1':'city2', 'coord1':'coord2'}).set_index('city2')
df_expand = df.groupby(['city1','coord1']).apply(lambda x:df2).reset_index(1).stack().reset_index().rename(columns={
0:'coords'})
return df_expand.pivot_table(values = 'coords',
index = 'city1',
columns = 'city2',
aggfunc = lambda x : geodesic(*x).km
).round(2)
%timeit -n 10 calculate_M()
395 ms ± 23.5 ms per loop (mean ± std. dev. of 7 runs, 10 loops each)
附录 :
geopy
包
- 根据城市名查城市位置
from geopy.geocoders import Nominatim
geolocator = Nominatim(user_agent="Mozilla/5.0 (Windows NT 10.0; Win64; x64) AppleWebKit/537.36 (KHTML, like Gecko) Chrome/72.0.3626.121 Safari/537.36")
location = geolocator.geocode("南京市雨花台区")
location.address
'雨花台区, 南京市, 江苏省, 中国'
location.longitude
118.7724224
location.latitude
31.9932018
- 根据经纬度查询位置 :
location = geolocator.reverse("31.997858805465647, 118.78544536405718")
location.address
'雨花东路, 雨花台区, 建邺区, 南京市, 江苏省, 21006, 中国'
location.raw
{'place_id': 134810031,
'licence': 'Data © OpenStreetMap contributors, ODbL 1.0. https://osm.org/copyright',
'osm_type': 'way',
'osm_id': 189414212,
'lat': '31.99705152324867',
'lon': '118.78513775762214',
'display_name': '雨花东路, 雨花台区, 建邺区, 南京市, 江苏省, 21006, 中国',
'address': {'road': '雨花东路',
'suburb': '雨花台区',
'city': '建邺区',
'state': '江苏省',
'postcode': '21006',
'country': '中国',
'country_code': 'cn'},
'boundingbox': ['31.9964788', '31.9989487', '118.7819222', '118.7866616']}
- 计算距离 :
from geopy.distance import distance, geodesic
wellington, salamanca = (-41.32, 174.81), (40.96, -5.50)
distance(wellington, salamanca, ellipsoid='GRS-80').miles
12402.369702934551
shanghai, beijing = (31.235929042252014,121.48053886017651), (39.910924547299565,116.4133836971231)
distance(shanghai, beijing).km
1065.985103985533
geodesic(shanghai, beijing).km
1065.985103985533
因此 , 任务四的数据集就可以自己造了 :
cities = df['city1']
cities.head()
0 沈阳市
1 长春市
2 哈尔滨市
3 北京市
4 天津市
Name: city1, dtype: object
def get_lon_lat(city):
location = geolocator.geocode(city)
return location.longitude,location.latitude
longitude, latitude = [*zip(*[get_lon_lat(city) for city in cities])]
data = pd.DataFrame({
'city':cities, 'longitude':longitude, 'latitude':latitude})
data.head()
|
city |
longitude |
latitude |
0 |
沈阳市 |
123.458674 |
41.674989 |
1 |
长春市 |
125.317122 |
43.813074 |
2 |
哈尔滨市 |
126.530400 |
45.798827 |
3 |
北京市 |
116.718521 |
39.902080 |
4 |
天津市 |
117.195107 |
39.085673 |