NMS和几种IOU的复现
- 输入为一张图片以及图片经过Yolo v3的推理结果,下载链接,提取码ha4o。
- NMS的实现参考的yolo v3,在细节上做了一些优化
- 实现了iou,giou,diou,ciou的计算
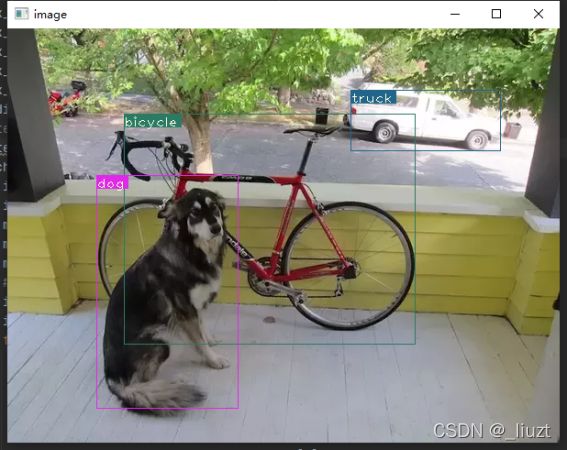
import cv2
import numpy as np
import random
import torch
import math
def bbox_giou(box1, box2):
"""
Returns the GIoU of two bounding boxes
"""
# Get the coordinates of bounding boxes
b1_x1, b1_y1, b1_x2, b1_y2 = box1[:, 0], box1[:, 1], box1[:, 2], box1[:, 3]
b2_x1, b2_y1, b2_x2, b2_y2 = box2[:, 0], box2[:, 1], box2[:, 2], box2[:, 3]
inter_rect_x1 = torch.max(b1_x1, b2_x1)
inter_rect_x2 = torch.min(b1_x2, b2_x2)
inter_rect_y1 = torch.max(b1_y1, b2_y1)
inter_rect_y2 = torch.min(b1_y2, b2_y2)
inter_area = torch.clamp(inter_rect_x2 - inter_rect_x1 + 1, min=0) * \
torch.clamp(inter_rect_y2 - inter_rect_y1 + 1, min=0)
outer_rect_x1 = torch.min(b1_x1, b2_x1)
outer_rect_x2 = torch.max(b1_x2, b2_x2)
outer_rect_y1 = torch.min(b1_y1, b2_y1)
outer_rect_y2 = torch.max(b1_y2, b2_y2)
outer_area = (outer_rect_x2 - outer_rect_x1) * (outer_rect_y2 - outer_rect_y1)
b1_area = (b1_x2 - b1_x1) * (b1_y2 - b1_y1)
b2_area = (b2_x2 - b2_x1) * (b2_y2 - b2_y1)
iou = inter_area / (b1_area + b2_area - inter_area)
giou = iou - (outer_area - (b1_area + b2_area - inter_area)) / outer_area
return giou
def bbox_diou(box1, box2):
"""
Returns the DIoU of two bounding boxes
"""
# Get the coordinates of bounding boxes
b1_x1, b1_y1, b1_x2, b1_y2 = box1[:, 0], box1[:, 1], box1[:, 2], box1[:, 3]
b2_x1, b2_y1, b2_x2, b2_y2 = box2[:, 0], box2[:, 1], box2[:, 2], box2[:, 3]
inter_rect_x1 = torch.max(b1_x1, b2_x1)
inter_rect_x2 = torch.min(b1_x2, b2_x2)
inter_rect_y1 = torch.max(b1_y1, b2_y1)
inter_rect_y2 = torch.min(b1_y2, b2_y2)
inter_area = torch.clamp(inter_rect_x2 - inter_rect_x1 + 1, min=0) * \
torch.clamp(inter_rect_y2 - inter_rect_y1 + 1, min=0)
b1_area = (b1_x2 - b1_x1) * (b1_y2 - b1_y1)
b2_area = (b2_x2 - b2_x1) * (b2_y2 - b2_y1)
iou = inter_area / (b1_area + b2_area - inter_area)
outer_rect_x1 = torch.min(b1_x1, b2_x1)
outer_rect_x2 = torch.max(b1_x2, b2_x2)
outer_rect_y1 = torch.min(b1_y1, b2_y1)
outer_rect_y2 = torch.max(b1_y2, b2_y2)
d2 = ((b2_x1 + (b2_x2 - b2_x1)/2) - (b1_x1 + (b1_x2 - b1_x1)/2)).pow(2) + \
((b2_y1 + (b2_y2 - b2_y1)/2) - (b1_y1 + (b1_y2 - b1_y1)/2)).pow(2)
c2 = (outer_rect_y2 - outer_rect_y1).pow(2) + (outer_rect_x2 - outer_rect_x1).pow(2)
diou = iou - d2 / c2
return diou
def bbox_ciou(box1, box2):
"""
Returns the CIoU of two bounding boxes
"""
# Get the coordinates of bounding boxes
b1_x1, b1_y1, b1_x2, b1_y2 = box1[:, 0], box1[:, 1], box1[:, 2], box1[:, 3]
b2_x1, b2_y1, b2_x2, b2_y2 = box2[:, 0], box2[:, 1], box2[:, 2], box2[:, 3]
inter_rect_x1 = torch.max(b1_x1, b2_x1)
inter_rect_x2 = torch.min(b1_x2, b2_x2)
inter_rect_y1 = torch.max(b1_y1, b2_y1)
inter_rect_y2 = torch.min(b1_y2, b2_y2)
inter_area = torch.clamp(inter_rect_x2 - inter_rect_x1 + 1, min=0) * \
torch.clamp(inter_rect_y2 - inter_rect_y1 + 1, min=0)
b1_area = (b1_x2 - b1_x1) * (b1_y2 - b1_y1)
b2_area = (b2_x2 - b2_x1) * (b2_y2 - b2_y1)
iou = inter_area / (b1_area + b2_area - inter_area)
outer_rect_x1 = torch.min(b1_x1, b2_x1)
outer_rect_x2 = torch.max(b1_x2, b2_x2)
outer_rect_y1 = torch.min(b1_y1, b2_y1)
outer_rect_y2 = torch.max(b1_y2, b2_y2)
d2 = ((b2_x1 + (b2_x2 - b2_x1)/2) - (b1_x1 + (b1_x2 - b1_x1)/2)).pow(2) + \
((b2_y1 + (b2_y2 - b2_y1)/2) - (b1_y1 + (b1_y2 - b1_y1)/2)).pow(2)
c2 = (outer_rect_y2 - outer_rect_y1).pow(2) + (outer_rect_x2 - outer_rect_x1).pow(2)
v = (4 / math.pi * math.pi) * \
(torch.atan((b1_x2 - b1_x1)/(b1_y2 - b1_y1)) - torch.atan((b2_x2 - b2_x1)/(b2_y2 - b2_y1))).pow(2)
alpha = v / (1 - iou + v)
ciou = iou - d2 / c2 - alpha * v
return ciou
def bbox_iou(box1, box2):
"""
Returns the GIoU of two bounding boxes
"""
# Get the coordinates of bounding boxes
b1_x1, b1_y1, b1_x2, b1_y2 = box1[:, 0], box1[:, 1], box1[:, 2], box1[:, 3]
b2_x1, b2_y1, b2_x2, b2_y2 = box2[:, 0], box2[:, 1], box2[:, 2], box2[:, 3]
inter_rect_x1 = torch.max(b1_x1, b2_x1)
inter_rect_x2 = torch.min(b1_x2, b2_x2)
inter_rect_y1 = torch.max(b1_y1, b2_y1)
inter_rect_y2 = torch.min(b1_y2, b2_y2)
inter_area = torch.clamp(inter_rect_x2 - inter_rect_x1 + 1, min=0) * \
torch.clamp(inter_rect_y2 - inter_rect_y1 + 1, min=0)
b1_area = (b1_x2 - b1_x1) * (b1_y2 - b1_y1)
b2_area = (b2_x2 - b2_x1) * (b2_y2 - b2_y1)
iou = inter_area / (b1_area + b2_area - inter_area)
return iou
CLASSES = ('person', 'bicycle', 'car', 'motorcycle', 'airplane', 'bus',
'train', 'truck', 'boat', 'traffic light', 'fire hydrant',
'stop sign', 'parking meter', 'bench', 'bird', 'cat', 'dog',
'horse', 'sheep', 'cow', 'elephant', 'bear', 'zebra', 'giraffe',
'backpack', 'umbrella', 'handbag', 'tie', 'suitcase', 'frisbee',
'skis', 'snowboard', 'sports ball', 'kite', 'baseball bat',
'baseball glove', 'skateboard', 'surfboard', 'tennis racket',
'bottle', 'wine glass', 'cup', 'fork', 'knife', 'spoon', 'bowl',
'banana', 'apple', 'sandwich', 'orange', 'broccoli', 'carrot',
'hot dog', 'pizza', 'donut', 'cake', 'chair', 'couch',
'potted plant', 'bed', 'dining table', 'toilet', 'tv', 'laptop',
'mouse', 'remote', 'keyboard', 'cell phone', 'microwave',
'oven', 'toaster', 'sink', 'refrigerator', 'book', 'clock',
'vase', 'scissors', 'teddy bear', 'hair drier', 'toothbrush')
def write(prediction, img):
for x in prediction:
cls = int(x[-1])
label = "{0}".format(CLASSES[cls])
c1 = tuple(list(map(int, x[1:3].tolist())))
c2 = tuple(list(map(int, x[3:5].tolist())))
r = random.randint(0, 255)
g = random.randint(0, 255)
b = random.randint(0, 255)
color = (b, g, r)
cv2.rectangle(img, c1, c2, color, 1)
t_size = cv2.getTextSize(label, cv2.FONT_HERSHEY_PLAIN, 1, 1)[0]
c2 = c1[0] + t_size[0] + 3, c1[1] + t_size[1] + 4
cv2.rectangle(img, c1, c2, color, -1)
cv2.putText(img, label, (c1[0], c1[1] + t_size[1] + 4), cv2.FONT_HERSHEY_PLAIN, 1, [225, 255, 255], 1);
return img
def unique(tensor):
tensor_np = tensor.cpu().numpy()
unique_np = np.unique(tensor_np)
unique_tensor = torch.from_numpy(unique_np)
tensor_res = tensor.new(unique_tensor.shape)
tensor_res.copy_(unique_tensor)
return tensor_res
def write_results(prediction, confidence, num_classes, nms_conf = 0.4):
# prediction (batch, point_num, 85) : center x y , w h, confidence, cls scores
# Step 1. 筛选出置信度大于confidence的
conf_mask = (prediction[:, :, 4] > confidence).float().unsqueeze(2)
prediction = prediction * conf_mask
non_zero = prediction[:, :, 4] > 0
prediction = prediction[non_zero].unsqueeze(0)
# Step 2. 根据中心点坐标和wh算出左上角右下角坐标,这里需要注意左上角为坐标原点
bbox_corner = prediction.new(prediction.shape)
bbox_corner[:, :, 0] = prediction[:, :, 0] - prediction[:, :, 2]/2
bbox_corner[:, :, 1] = prediction[:, :, 1] - prediction[:, :, 3]/2
bbox_corner[:, :, 2] = prediction[:, :, 0] + prediction[:, :, 2]/2
bbox_corner[:, :, 3] = prediction[:, :, 1] + prediction[:, :, 3]/2
prediction[:, :, :4] = bbox_corner[:, :, :4]
# Step3. 分Batch进行NMS
write = False
batch_size = prediction.shape[0]
for i in range(batch_size):
img_pred = prediction[i]
max_cls_score, max_cls = torch.max(img_pred[:, 5:5 + num_classes], 1)
max_cls_score = max_cls_score.float().unsqueeze(1)
max_cls = max_cls.float().unsqueeze(1)
# img_pred (point_num, 7) 角点坐标, 置信度, 分类分数, 类别
img_pred = torch.cat((img_pred[:, :5], max_cls_score, max_cls), 1)
img_classes = unique(img_pred[:, -1])
for cls in img_classes:
pos_index = img_pred[:, -1] == cls
img_pred_cls = img_pred[pos_index]
conf_sort_index = torch.sort(img_pred_cls[:, 4], descending=True)[1]
img_pred_cls = img_pred_cls[conf_sort_index]
index = 0
while index + 1 < img_pred_cls.shape[0]:
ious = bbox_ciou(img_pred_cls[index].unsqueeze(0), img_pred_cls[index + 1:])
iou_mask = (ious < nms_conf).float().unsqueeze(1)
img_pred_cls[index+1:] = img_pred_cls[index+1:] * iou_mask
# Remove the non-zero entries
non_zero_ind = torch.nonzero(img_pred_cls[:, 4]).squeeze()
img_pred_cls = img_pred_cls[non_zero_ind].view(-1, 7)
index += 1
batch_ind = img_pred_cls.new(img_pred_cls.size(0), 1).fill_(i) # Repeat the batch_id for as many detections of the class cls in the images
seq = batch_ind, img_pred_cls
if not write:
output = torch.cat(seq, 1)
write = True
else:
out = torch.cat(seq, 1)
output = torch.cat((output, out))
return output
def reshape_output(prediction_output):
radio = min(416/image.shape[0], 416/image.shape[1])
prediction_output[:, [1, 3]] -= (416 - radio * image.shape[1]) / 2
prediction_output[:, [1, 3]] /= radio
prediction_output[:, [2, 4]] -= (416 - radio * image.shape[0]) / 2
prediction_output[:, [2, 4]] /= radio
return prediction_output
if __name__ == '__main__':
image = cv2.imread('dog-cycle-car.png')
# prediction (b, point_num, 85) : center x y , w h, confidence, cls scores
prediction = np.load('prediction.npy')
prediction = torch.from_numpy(prediction)
# (第几张,左上x,左上y,右下x,右下y,bbox置信度,目标种类得分,什么物体)
output = write_results(prediction, 0.5, num_classes=80, nms_conf=0.4)
output = reshape_output(output)
image = write(output, image)
cv2.imshow('image', image)
while 1:
cv2.waitKey(1)