数据合并
pandas包中,进行数据合并有join()
、merge()
、concat()
, append()
四种方法。它们的区别是:
- df.join() 相同行索引的数据被合并在一起,因此拼接后的行数不会增加(可能会减少)、列数增加;
- df.merge()通过指定的列索引进行合并,行列都有可能增加;merge也可以指定行索引进行合并;
- pd.concat()通过axis参数指定在水平还是垂直方向拼接;
- df.append()在DataFrame的末尾添加一行或多行;大致等价于
pd.concat([df1,df2],axis=0,join='outer')
。
import numpy as np
import pandas as pd
df1 = pd.DataFrame(np.arange(1,9).reshape(2, 4),index=["A","B"],columns=list("abcd"))
df1
|
a |
b |
c |
d |
A |
1 |
2 |
3 |
4 |
B |
5 |
6 |
7 |
8 |
df2 = pd.DataFrame(np.zeros((3,3)),index=list("ABC"),columns=list("xyz"))
df2
|
x |
y |
z |
A |
0.0 |
0.0 |
0.0 |
B |
0.0 |
0.0 |
0.0 |
C |
0.0 |
0.0 |
0.0 |
1 df.join()按相同index拼接列
- 相同行索引的不同列被合并在一起;
- 左拼接(how=‘left’)时,other中与DataFrame中不同的行索引会被忽略(如C行只在df2中,合并后的df1_new中没有C行);
- 没同时在两个对象中的位置,会用NaN填充。如:左拼接(how=‘left’)时,DataFrame中有,但other中没有的行索引会保留,并用NaN填充;
- 要合并的两个DataFrame对象默认不能有相同的列索引。若有相同列索引,需要通过lsuffix或rsuffix参数为相同列指定后缀,否则会报错。
源码:
DataFrame.join(other, on=None, how='left', lsuffix='', rsuffix='', sort=False)
参数 |
说明 |
other |
右表, DataFrame, Series, or list of DataFrame |
on |
关联字段, 是关联index的 |
how |
拼接方式,默认left,{‘left’, ‘right’, ‘outer’, ‘inner’} |
lsuffix |
左表相同列索引的后缀 |
rsuffix |
右表相同列索引的后缀 |
sort |
根据连接键对合并后的数据进行排列,默认为False |
1.1 how参数取值含义示意图:
how=‘inner’ 内连接:A.join(B, how=‘inner’),取同时出现在AB中的index:
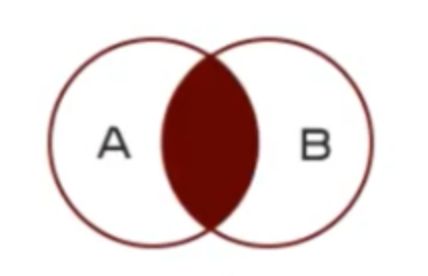
how=‘outer’ 外连接:A.join(B, how=‘outer’),取AB中的index的并集:
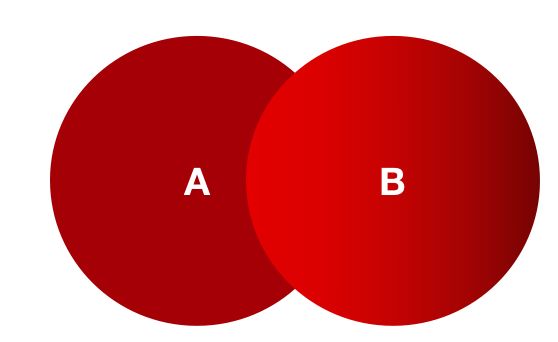
how=‘right’ 右连接:A.join(B, how=‘right’),B中的所有index,独自出现在A中的index被忽略:
how=‘left’ 右连接:A.join(B, how=‘left’),A中所有的index,独自出现在B中的index被忽略:
1.2 有相同行索引且没有重复列索引时:
how参数默认left,代表左连接,以左边的DataFrame的index为拼接依据;
print("df1.index:\n", df1.index)
print()
df1_new = df1.join(df2)
df1_new
df1.index:
Index(['A', 'B'], dtype='object')
|
a |
b |
c |
d |
x |
y |
z |
A |
1 |
2 |
3 |
4 |
0.0 |
0.0 |
0.0 |
B |
5 |
6 |
7 |
8 |
0.0 |
0.0 |
0.0 |
df2.join(df1)
|
x |
y |
z |
a |
b |
c |
d |
A |
0.0 |
0.0 |
0.0 |
1.0 |
2.0 |
3.0 |
4.0 |
B |
0.0 |
0.0 |
0.0 |
5.0 |
6.0 |
7.0 |
8.0 |
C |
0.0 |
0.0 |
0.0 |
NaN |
NaN |
NaN |
NaN |
1.3 有相同行索引和列索引
df = pd.DataFrame({'key': ['K0', 'K1', 'K2', 'K3', 'K4', 'K5'],
'A': ['A0', 'A1', 'A2', 'A3', 'A4', 'A5']})
df
|
key |
A |
0 |
K0 |
A0 |
1 |
K1 |
A1 |
2 |
K2 |
A2 |
3 |
K3 |
A3 |
4 |
K4 |
A4 |
5 |
K5 |
A5 |
other = pd.DataFrame({'key': ['K0', 'K1', 'K2'],
'B': ['B0', 'B1', 'B2']})
other
|
key |
B |
0 |
K0 |
B0 |
1 |
K1 |
B1 |
2 |
K2 |
B2 |
列索引’key’在左右表中都存在,必须指定左右表的后缀,可以2个参数一起使用,或只指定左表或右表的后缀
df.join(other, lsuffix='_left', rsuffix='_ritht')
|
key_left |
A |
key_ritht |
B |
0 |
K0 |
A0 |
K0 |
B0 |
1 |
K1 |
A1 |
K1 |
B1 |
2 |
K2 |
A2 |
K2 |
B2 |
3 |
K3 |
A3 |
NaN |
NaN |
4 |
K4 |
A4 |
NaN |
NaN |
5 |
K5 |
A5 |
NaN |
NaN |
df.join(other, lsuffix='_left')
|
key_left |
A |
key |
B |
0 |
K0 |
A0 |
K0 |
B0 |
1 |
K1 |
A1 |
K1 |
B1 |
2 |
K2 |
A2 |
K2 |
B2 |
3 |
K3 |
A3 |
NaN |
NaN |
4 |
K4 |
A4 |
NaN |
NaN |
5 |
K5 |
A5 |
NaN |
NaN |
df.join(other, rsuffix='_ritht')
|
key |
A |
key_ritht |
B |
0 |
K0 |
A0 |
K0 |
B0 |
1 |
K1 |
A1 |
K1 |
B1 |
2 |
K2 |
A2 |
K2 |
B2 |
3 |
K3 |
A3 |
NaN |
NaN |
4 |
K4 |
A4 |
NaN |
NaN |
5 |
K5 |
A5 |
NaN |
NaN |
1.4 on参数指定关联字段
默认情况下,左连接(how=‘left’)时,当想拼接的两个表中,虽然行索引不同,但右表的行索引与左表中某一列的值有相同值时,可以 左表.join(右表, on='右表列索引')
来进行拼接。
other.set_index(keys=['key'])
df
|
key |
A |
0 |
K0 |
A0 |
1 |
K1 |
A1 |
2 |
K2 |
A2 |
3 |
K3 |
A3 |
4 |
K4 |
A4 |
5 |
K5 |
A5 |
df.join(other.set_index(keys=['key']), on='key')
|
key |
A |
B |
0 |
K0 |
A0 |
B0 |
1 |
K1 |
A1 |
B1 |
2 |
K2 |
A2 |
B2 |
3 |
K3 |
A3 |
NaN |
4 |
K4 |
A4 |
NaN |
5 |
K5 |
A5 |
NaN |
2 df.merge()按列索引拼接列
2.1 两表中有共同列索引时:
nan代替没同时出现的元素
源码:
DataFrame.merge(right, how='inner', on=None, left_on=None, right_on=None, left_index=False, right_index=False, sort=False, suffixes='_x', '_y', copy=True, indicator=False, validate=None)
参数 |
说明 |
right |
右表, DataFrame, Series, or list of DataFrame |
how |
拼接方式,默认inner,{‘left’, ‘right’, ‘outer’, ‘inner’} |
on |
默认None,自动根据相同列拼接。关联字段, 是关联columns的,必须同时存在于2个表中 |
left_on |
默认None,左表中用作连接键的列索引 |
right_on |
默认None,右表中用作连接键的列索引 |
left_index |
默认Flase,是否将左表中的行索引用作连接键 |
right_index |
默认Flase,是否将右表中的行索引用作连接键 |
sort |
根据连接键对合并后的数据进行排列,默认为Flase |
df1
|
a |
b |
c |
d |
A |
1 |
2 |
3 |
4 |
B |
5 |
6 |
7 |
8 |
df3 = pd.DataFrame(np.zeros((3,3)),columns=list("fax"))
df3
|
f |
a |
x |
0 |
0.0 |
0.0 |
0.0 |
1 |
0.0 |
0.0 |
0.0 |
2 |
0.0 |
0.0 |
0.0 |
2.1.1 默认内连接 how=‘inner’
df1
|
a |
b |
c |
d |
A |
1 |
2 |
3 |
4 |
B |
5 |
6 |
7 |
8 |
df1.merge(df3, on="a")
df3 = pd.DataFrame(np.arange(12).reshape((3,4)),columns=list("faxy"))
df3
|
f |
a |
x |
y |
0 |
0 |
1 |
2 |
3 |
1 |
4 |
5 |
6 |
7 |
2 |
8 |
9 |
10 |
11 |
df1.merge(df3, on="a")
|
a |
b |
c |
d |
f |
x |
y |
0 |
1 |
2 |
3 |
4 |
0 |
2 |
3 |
1 |
5 |
6 |
7 |
8 |
4 |
6 |
7 |
2.1.2 外连接 how=‘outer’
df1.merge(df3, on="a", how="outer")
|
a |
b |
c |
d |
f |
x |
y |
0 |
1 |
2.0 |
3.0 |
4.0 |
0 |
2 |
3 |
1 |
5 |
6.0 |
7.0 |
8.0 |
4 |
6 |
7 |
2 |
9 |
NaN |
NaN |
NaN |
8 |
10 |
11 |
2.1.3 左连接 how=‘left’
df1.merge(df3, on="a", how="left")
|
a |
b |
c |
d |
f |
x |
y |
0 |
1 |
2 |
3 |
4 |
0 |
2 |
3 |
1 |
5 |
6 |
7 |
8 |
4 |
6 |
7 |
2.1.4 右连接 how=‘right’
df1.merge(df3, on="a", how="right")
|
a |
b |
c |
d |
f |
x |
y |
0 |
1 |
2.0 |
3.0 |
4.0 |
0 |
2 |
3 |
1 |
5 |
6.0 |
7.0 |
8.0 |
4 |
6 |
7 |
2 |
9 |
NaN |
NaN |
NaN |
8 |
10 |
11 |
2.2 当2表中没有共同列索引:
t1.merge(t2, left_on="O", right_on="X")
- 通过left_on指定t1中的按照O列进行合并
- 通过right_on指定t2中的按照X列进行合并
- O列和X列中必须有相同的元素,比如下例中的元素"c"
- NAN代替没同时出现的元素
t1 = pd.DataFrame(np.ones((3, 4)), index=list("ABC"), columns=list("MNOP"))
for i in t1.index:
t1.loc[i, "O"] = i.lower()
t1
|
M |
N |
O |
P |
A |
1.0 |
1.0 |
a |
1.0 |
B |
1.0 |
1.0 |
b |
1.0 |
C |
1.0 |
1.0 |
c |
1.0 |
t2 = pd.DataFrame(np.zeros((2, 5)), index=list("AB"), columns=list("VWXYZ"))
t2.loc["A", "X"] = "c"
t2.loc["B", "X"] = "d"
t2
|
V |
W |
X |
Y |
Z |
A |
0.0 |
0.0 |
c |
0.0 |
0.0 |
B |
0.0 |
0.0 |
d |
0.0 |
0.0 |
2.2.1 how='inner’交集
t1.merge(t2, left_on="O", right_on="X")
|
M |
N |
O |
P |
V |
W |
X |
Y |
Z |
0 |
1.0 |
1.0 |
c |
1.0 |
0.0 |
0.0 |
c |
0.0 |
0.0 |
2.2.2 how='outer’并集
t1.merge(t2, left_on="O", right_on="X", how="outer")
|
M |
N |
O |
P |
V |
W |
X |
Y |
Z |
0 |
1.0 |
1.0 |
a |
1.0 |
NaN |
NaN |
NaN |
NaN |
NaN |
1 |
1.0 |
1.0 |
b |
1.0 |
NaN |
NaN |
NaN |
NaN |
NaN |
2 |
1.0 |
1.0 |
c |
1.0 |
0.0 |
0.0 |
c |
0.0 |
0.0 |
3 |
NaN |
NaN |
NaN |
NaN |
0.0 |
0.0 |
d |
0.0 |
0.0 |
2.2.3 how='left’左连接
t1.shape, t2.shape
((3, 4), (2, 5))
t1.merge(t2, left_on="O", right_on="X", how="left")
|
M |
N |
O |
P |
V |
W |
X |
Y |
Z |
0 |
1.0 |
1.0 |
a |
1.0 |
NaN |
NaN |
NaN |
NaN |
NaN |
1 |
1.0 |
1.0 |
b |
1.0 |
NaN |
NaN |
NaN |
NaN |
NaN |
2 |
1.0 |
1.0 |
c |
1.0 |
0.0 |
0.0 |
c |
0.0 |
0.0 |
2.2.4 how='right’右连接
t1.merge(t2, left_on="O", right_on="X", how="right")
|
M |
N |
O |
P |
V |
W |
X |
Y |
Z |
0 |
1.0 |
1.0 |
c |
1.0 |
0.0 |
0.0 |
c |
0.0 |
0.0 |
1 |
NaN |
NaN |
NaN |
NaN |
0.0 |
0.0 |
d |
0.0 |
0.0 |
2.3 存在相同列,但指定以其他不同列拼接时,suffixes参数为相同列增加后缀
df1 = pd.DataFrame({'lkey': ['foo', 'bar', 'baz', 'foo'],
'value': [1, 2, 3, 5]})
df1
|
lkey |
value |
0 |
foo |
1 |
1 |
bar |
2 |
2 |
baz |
3 |
3 |
foo |
5 |
df2 = pd.DataFrame({'rkey': ['foo', 'bar', 'baz', 'foo'],
'value': [5, 6, 7, 8]})
df2
|
rkey |
value |
0 |
foo |
5 |
1 |
bar |
6 |
2 |
baz |
7 |
3 |
foo |
8 |
df1.merge(df2)
|
lkey |
value |
rkey |
0 |
foo |
5 |
foo |
df1.merge(df2, left_on='lkey', right_on='rkey')
|
lkey |
value_x |
rkey |
value_y |
0 |
foo |
1 |
foo |
5 |
1 |
foo |
1 |
foo |
8 |
2 |
foo |
5 |
foo |
5 |
3 |
foo |
5 |
foo |
8 |
4 |
bar |
2 |
bar |
6 |
5 |
baz |
3 |
baz |
7 |
2.4 指定以相同行索引进行拼接,等价于join()方法:
df1
|
lkey |
value |
0 |
foo |
1 |
1 |
bar |
2 |
2 |
baz |
3 |
3 |
foo |
5 |
df2
|
rkey |
value |
0 |
foo |
5 |
1 |
bar |
6 |
2 |
baz |
7 |
3 |
foo |
8 |
df1.merge(df2, left_index=True, right_index=True)
|
lkey |
value_x |
rkey |
value_y |
0 |
foo |
1 |
foo |
5 |
1 |
bar |
2 |
bar |
6 |
2 |
baz |
3 |
baz |
7 |
3 |
foo |
5 |
foo |
8 |
3 按方向拼接 pd.concat()
pandas.concat(objs: Union[Iterable[FrameOrSeries], Mapping[Label, FrameOrSeries]], axis='0', join: str = "'outer'", ignore_index: bool = 'False', keys='None', levels='None', names='None', verify_integrity: bool = 'False', sort: bool = 'False', copy: bool = 'True') → FrameOrSeriesUnion
-
按方向拼接(pd.concat()按行或列进行合并,axis参数决定拼接方向)
-
pd.concat([data1, data2], axis=0) 默认axis=0,垂直方向拼接,默认join='outer’并集拼接;当join='inner’进行交集拼接时,对列索引取交集;
-
pd.concat([data1, data2], axis=1) 水平方向拼接,默认并集拼接;当join='inner’进行交集拼接时,对行索引取交集;
-
并集拼接时,若列标签均不相同,则行列标签数量均会增加,未同时存在在2个表中的字段的values为NaN;
常用参数 |
说明 |
objs |
要合并的DataFrame或Series,以列表传入。如[df1, df2] |
axis |
拼接方向,{0/’index’, 1/’columns’},默认0,代表垂直方向拼接;1代表水平方向拼接 |
join |
拼接方式,默认outer并集,{‘outer’, ‘inner’} ,inner交集 |
ignore_index |
默认False,是否需要重置索引。 |
df1
|
lkey |
value |
0 |
foo |
1 |
1 |
bar |
2 |
2 |
baz |
3 |
3 |
foo |
5 |
df2
|
rkey |
value |
0 |
foo |
5 |
1 |
bar |
6 |
2 |
baz |
7 |
3 |
foo |
8 |
3.1 axis=0 垂直方向
3.1.1 join='outer’并集拼接
相同的字段的列被合并,不同的列也被保留,未同时出现在2表中的字段以空值NaN代替
pd.concat([df1, df2])
|
lkey |
value |
rkey |
0 |
foo |
1 |
NaN |
1 |
bar |
2 |
NaN |
2 |
baz |
3 |
NaN |
3 |
foo |
5 |
NaN |
0 |
NaN |
5 |
foo |
1 |
NaN |
6 |
bar |
2 |
NaN |
7 |
baz |
3 |
NaN |
8 |
foo |
3.1.2 join='inner’交集拼接,未重置索引
垂直交集拼接时,相同列索引的字段垂直拼接,不同列索引的字段被忽略。
pd.concat([df1, df2], join='inner')
|
value |
0 |
1 |
1 |
2 |
2 |
3 |
3 |
5 |
0 |
5 |
1 |
6 |
2 |
7 |
3 |
8 |
3.1.3 join='inner’交集+重置索引ignore_index=True
pd.concat([df1, df2], join='inner', ignore_index=True)
|
value |
0 |
1 |
1 |
2 |
2 |
3 |
3 |
5 |
4 |
5 |
5 |
6 |
6 |
7 |
7 |
8 |
3.2 axis=1水平方向
3.2.1 join='outer’并集拼接
水平方向并集拼接时,相同列索引被当做2个不同列保留
df1.index = [1, 2, 3, 4]
df1
|
lkey |
value |
1 |
foo |
1 |
2 |
bar |
2 |
3 |
baz |
3 |
4 |
foo |
5 |
df2
|
rkey |
value |
0 |
foo |
5 |
1 |
bar |
6 |
2 |
baz |
7 |
3 |
foo |
8 |
pd.concat([df1, df2], axis=1)
|
lkey |
value |
rkey |
value |
0 |
NaN |
NaN |
foo |
5.0 |
1 |
foo |
1.0 |
bar |
6.0 |
2 |
bar |
2.0 |
baz |
7.0 |
3 |
baz |
3.0 |
foo |
8.0 |
4 |
foo |
5.0 |
NaN |
NaN |
3.2.2 join='inner’交集拼接
pd.concat([df1, df2], axis=1, join='inner')
|
lkey |
value |
rkey |
value |
1 |
foo |
1 |
bar |
6 |
2 |
bar |
2 |
baz |
7 |
3 |
baz |
3 |
foo |
8 |
4 df.append()在DataFrame末尾添加行
- 和pd.concat方法的区别:
- append只能做行的拼接
- append方法是外连接
- 相同点:
- append可以支持多个DataFrame的拼接
- append大致等同于
pd.concat([df1,df2],axis=0,join='outer')
源码:
DataFrame.append(other, ignore_index=False, verify_integrity=False, sort=False)
常用参数 |
说明 |
other |
要被拼接进去的对象 |
ignore_index |
是否需要重置索引,默认False不重置,会保留other的原索引; |
verify_integrity |
默认False,是否在创建具有重复项的索引时引发ValueError |
sort |
默认False,否,是否在df和other的列不对齐时,对列进行排序 |
df = pd.DataFrame([[1, 2], [3, 4]], index=[1, 2], columns=list('AB'))
df
df2 = pd.DataFrame([[5, 6], [7, 8]], columns=list('AB'))
df2
df.append(df2)
|
A |
B |
1 |
1 |
2 |
2 |
3 |
4 |
0 |
5 |
6 |
1 |
7 |
8 |
df.append(df2, ignore_index=True)
|
A |
B |
0 |
1 |
2 |
1 |
3 |
4 |
2 |
5 |
6 |
3 |
7 |
8 |
上一行代码,与下面pd.concat()等价
pd.concat([df, df2], axis=0, join='outer', ignore_index=True)
|
A |
B |
0 |
1 |
2 |
1 |
3 |
4 |
2 |
5 |
6 |
3 |
7 |
8 |