制作自己的coco关键点数据集
1、将我们需要标注的图片进行重命名,由于coco数据集图片的名称是12位数字,形式如 00000000001。代码如下:
import os
from tqdm import tqdm
import codecs
import json
for root, _, path in os.walk(<保存图片的文件夹路径>):
for s in tqdm(path):
os.renames('<保存图片的文件夹路径>/{}'.format(s), 'dataset_new/%012d.jpg' %int(s.split('.')[0]))
2、使用labelme标注软件进行数据集的标注。(labelme是专门用来标注的软件)直接pip install 即可。
使用labelme进行关键点标注前,我们需要明确我们需要的关节点有哪些,例如coco数据集中关键点如下:
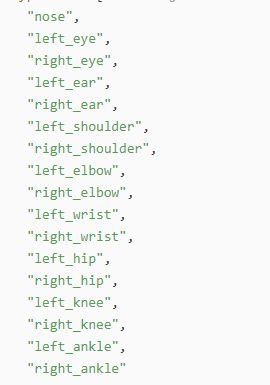
一共是17 个关节点,注意使用labelme标注的时候,序号是从1开始的,最后就是标注啦,数据集的标注自认为是最浪费时间的,但是我们一定得仔细,否则后面的训练就会很麻烦!标注后会生成对应的json文件,如下: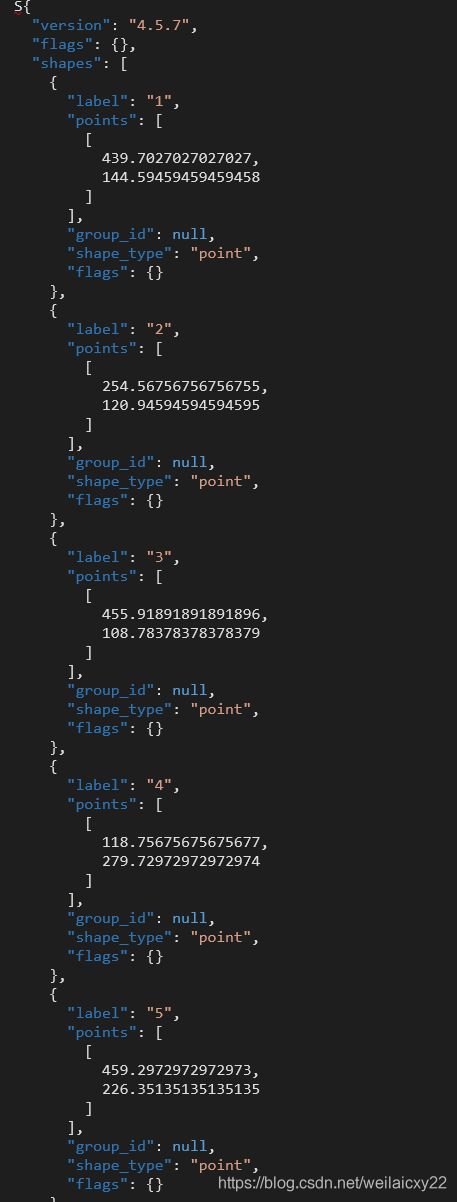
3、将label标注数据集进行格式转换,转换成coco格式
最后因为目前的大多数网络都是用的coco数据集,因此我们需要将数据集转换成coco数据集格式,在标注之前我们同样需要将json文件的名称改为 000000000011 这种格式,代码参见以上。转换代码如下:
import numpy as np
import json
import glob
import codecs
import os
class MyEncoder(json.JSONEncoder):
def default(self, obj):
if isinstance(obj, np.integer):
return int(obj)
elif isinstance(obj, np.floating):
return float(obj)
elif isinstance(obj, np.ndarray):
return obj.tolist()
else:
return super(MyEncoder, self).default(obj)
class tococo(object):
def __init__(self, jsonfile, save_path, a):
self.images = []
self.categories = [
{
"supercategory": "person",
"id": 1,
"name": "person",
"keypoints":
[
"nose",
"left_ear",
"right_ear",
"left_shoulder",
"right_shoulder",
"left_elbow",
"right_elbow",
"left_wrist",
"right_wrist"
],
"skeleton": [
[6, 8],
[4, 6],
[2, 4],
[1, 2],
[3, 1],
[5, 3],
[7, 5],
[9, 7],
]
}
]
self.annotations = []
self.jsonfile = os.listdir(jsonfile)
self.save_path = save_path
self.class_id = a
self.coco = {}
self.path = jsonfile
def labelme_to_coco(self):
for num, json_file in enumerate(self.jsonfile):
json_file = os.path.join(self.path, json_file)
data = codecs.open(json_file, 'r')
data = json.load(data)
self.images.append(self.get_images(json_file[-17:-4] + 'jpg', data["imageHeight"], data["imageWidth"]))
shapes = data["shapes"]
annotation = {}
num_keypoints = 0
keypoints = [0] * 3 * 9
flag = 0
for shape in shapes:
if shape['shape_type'] == 'rectangle' or shape["label"] == '90' or shape["label"] == '99':
bbox = []
temp = shape["points"]
try:
x_min = min(temp[0][0], temp[1][0])
except IndexError as e:
print('class: {}, image: {}'.format(self.class_id, int(json_file[-17:-5])))
x_max = max(temp[0][0], temp[1][0])
y_min = min(temp[0][1], temp[1][1])
y_max = max(temp[0][1], temp[1][1])
bbox.append(x_min)
bbox.append(y_min)
w = x_max - x_min + 1
h = y_max - y_min + 1
bbox.append(w)
bbox.append(h)
annotation['bbox'] = bbox
flag = flag + 1
else:
idx = int(shape['label'])
try:
keypoints[(idx - 1) * 3 + 0] = shape['points'][0][0]
keypoints[(idx - 1) * 3 + 1] = shape['points'][0][1]
keypoints[(idx - 1) * 3 + 2] = 2
num_keypoints = num_keypoints + 1
except IndexError as e:
print('class: {}, image: {}'.format(self.class_id, int(json_file[-17:-5])))
if flag == 0:
print('{}\\{} does not contain bbox\n'.format(self.class_id, json_file))
annotation['segmentation'] = [[]]
annotation['num_keypoints'] = num_keypoints
try:
annotation['area'] = 0
except ValueError as e:
print(json_file[-17:-5])
annotation['iscrowd'] = 0
annotation['keypoints'] = keypoints
annotation['image_id'] = int(json_file[-17:-5])
annotation['bbox'] = [0, 0, data['imageWidth'], data['imageHeight']]
annotation['category_id'] = 1
annotation['id'] = int(json_file[-17:-5])
self.annotations.append(annotation)
self.image_id = int(json_file[-17:-5])
self.coco["images"] = self.images
self.coco["categories"] = self.categories
self.coco["annotations"] = self.annotations
def get_images(self, filename, height, width):
image = {}
image["height"] = height
image['width'] = width
image["id"] = int(filename[-16:-4])
image["file_name"] = filename
return image
def get_categories(self, name, class_id):
category = {}
category["supercategory"] = "person"
category['id'] = class_id
category['name'] = name
return category
def save_json(self):
self.labelme_to_coco()
coco_data = self.coco
json.dump(coco_data, open(self.save_path, 'w'), indent=4, cls=MyEncoder)
return self.image_id
json_path = r'J:\keypoint_own/label_val'
c = tococo(json_path, save_path='val.json', a=1)
image_id = c.save_json()
4、使用coco api验证我们转换后的格式是否正确,代码如下:
import skimage.io as io
import pylab
import time as time
import json
import numpy as np
from collections import defaultdict
import itertools
import matplotlib.pyplot as plt
from matplotlib.collections import PatchCollection
def _isArrayLike(obj):
return hasattr(obj, '__iter__') and hasattr(obj, '__len__')
class COCO:
def __init__(self, annotation_file=None):
"""
Constructor of Microsoft COCO helper class for reading and visualizing annotations.
:param annotation_file (str): location of annotation file
:param image_folder (str): location to the folder that hosts images.
:return:
"""
self.dataset, self.anns, self.cats, self.imgs = dict(), dict(), dict(), dict()
self.imgToAnns, self.catToImgs = defaultdict(list), defaultdict(list)
if not annotation_file == None:
print('loading annotations into memory...')
tic = time.time()
dataset = json.load(open(annotation_file, 'r'))
assert type(dataset) == dict, 'annotation file format {} not supported'.format(type(dataset))
print('Done (t={:0.2f}s)'.format(time.time() - tic))
self.dataset = dataset
self.createIndex()
def createIndex(self):
print('creating index...')
anns, cats, imgs = {}, {}, {}
imgToAnns, catToImgs = defaultdict(list), defaultdict(list)
if 'annotations' in self.dataset:
for ann in self.dataset['annotations']:
imgToAnns[ann['image_id']].append(ann)
anns[ann['id']] = ann
if 'images' in self.dataset:
for img in self.dataset['images']:
imgs[img['id']] = img
if 'categories' in self.dataset:
for cat in self.dataset['categories']:
cats[cat['id']] = cat
if 'annotations' in self.dataset and 'categories' in self.dataset:
for ann in self.dataset['annotations']:
catToImgs[ann['category_id']].append(ann['image_id'])
print('index created!')
self.anns = anns
self.imgToAnns = imgToAnns
self.catToImgs = catToImgs
self.imgs = imgs
self.cats = cats
def getCatIds(self, catNms=[], supNms=[], catIds=[]):
"""
filtering parameters. default skips that filter.
:param catNms (str array) : get cats for given cat names
:param supNms (str array) : get cats for given supercategory names
:param catIds (int array) : get cats for given cat ids
:return: ids (int array) : integer array of cat ids
"""
catNms = catNms if _isArrayLike(catNms) else [catNms]
supNms = supNms if _isArrayLike(supNms) else [supNms]
catIds = catIds if _isArrayLike(catIds) else [catIds]
if len(catNms) == len(supNms) == len(catIds) == 0:
cats = self.dataset['categories']
else:
cats = self.dataset['categories']
cats = cats if len(catNms) == 0 else [cat for cat in cats if cat['name'] in catNms]
cats = cats if len(supNms) == 0 else [cat for cat in cats if cat['supercategory'] in supNms]
cats = cats if len(catIds) == 0 else [cat for cat in cats if cat['id'] in catIds]
ids = [cat['id'] for cat in cats]
return ids
def loadCats(self, ids=[]):
"""
Load cats with the specified ids.
:param ids (int array) : integer ids specifying cats
:return: cats (object array) : loaded cat objects
"""
if _isArrayLike(ids):
return [self.cats[id] for id in ids]
elif type(ids) == int:
return [self.cats[ids]]
def getImgIds(self, imgIds=[], catIds=[]):
'''
Get img ids that satisfy given filter conditions.
:param imgIds (int array) : get imgs for given ids
:param catIds (int array) : get imgs with all given cats
:return: ids (int array) : integer array of img ids
'''
imgIds = imgIds if _isArrayLike(imgIds) else [imgIds]
catIds = catIds if _isArrayLike(catIds) else [catIds]
if len(imgIds) == len(catIds) == 0:
ids = self.imgs.keys()
else:
ids = set(imgIds)
for i, catId in enumerate(catIds):
if i == 0 and len(ids) == 0:
ids = set(self.catToImgs[catId])
else:
ids &= set(self.catToImgs[catId])
return list(ids)
def loadImgs(self, ids=[]):
"""
Load anns with the specified ids.
:param ids (int array) : integer ids specifying img
:return: imgs (object array) : loaded img objects
"""
if _isArrayLike(ids):
return [self.imgs[id] for id in ids]
elif type(ids) == int:
return [self.imgs[ids]]
def getAnnIds(self, imgIds=[], catIds=[], areaRng=[], iscrowd=None):
"""
Get ann ids that satisfy given filter conditions. default skips that filter
:param imgIds (int array) : get anns for given imgs
catIds (int array) : get anns for given cats
areaRng (float array) : get anns for given area range (e.g. [0 inf])
iscrowd (boolean) : get anns for given crowd label (False or True)
:return: ids (int array) : integer array of ann ids
"""
imgIds = imgIds if _isArrayLike(imgIds) else [imgIds]
catIds = catIds if _isArrayLike(catIds) else [catIds]
if len(imgIds) == len(catIds) == len(areaRng) == 0:
anns = self.dataset['annotations']
else:
if not len(imgIds) == 0:
lists = [self.imgToAnns[imgId] for imgId in imgIds if imgId in self.imgToAnns]
anns = list(itertools.chain.from_iterable(lists))
else:
anns = self.dataset['annotations']
anns = anns if len(catIds) == 0 else [ann for ann in anns if ann['category_id'] in catIds]
anns = anns if len(areaRng) == 0 else [ann for ann in anns if
ann['area'] > areaRng[0] and ann['area'] < areaRng[1]]
if not iscrowd == None:
ids = [ann['id'] for ann in anns if ann['iscrowd'] == iscrowd]
else:
ids = [ann['id'] for ann in anns]
return ids
def loadAnns(self, ids=[]):
"""
Load anns with the specified ids.
:param ids (int array) : integer ids specifying anns
:return: anns (object array) : loaded ann objects
"""
if _isArrayLike(ids):
return [self.anns[id] for id in ids]
elif type(ids) == int:
return [self.anns[ids]]
def showAnns(self, anns):
"""
Display the specified annotations.
:param anns (array of object): annotations to display
:return: None
"""
if len(anns) == 0:
return 0
if 'segmentation' in anns[0] or 'keypoints' in anns[0]:
datasetType = 'instances'
elif 'caption' in anns[0]:
datasetType = 'captions'
else:
raise Exception('datasetType not supported')
if datasetType == 'instances':
ax = plt.gca()
ax.set_autoscale_on(False)
polygons = []
color = []
for ann in anns:
c = (np.random.random((1, 3)) * 0.6 + 0.4).tolist()[0]
if 'keypoints' in ann and type(ann['keypoints']) == list:
sks = np.array(self.loadCats(ann['category_id'])[0]['skeleton']) - 1
kp = np.array(ann['keypoints'])
x = kp[0::3]
y = kp[1::3]
v = kp[2::3]
for sk in sks:
if np.all(v[sk] > 0):
plt.plot(x[sk], y[sk], linewidth=1, color=c)
plt.plot(x[v > 0], y[v > 0], 'o', markersize=4, markerfacecolor=c, markeredgecolor='k',
markeredgewidth=1)
plt.plot(x[v > 1], y[v > 1], 'o', markersize=4, markerfacecolor=c, markeredgecolor=c,
markeredgewidth=1)
p = PatchCollection(polygons, facecolor=color, linewidths=0, alpha=0.4)
ax.add_collection(p)
p = PatchCollection(polygons, facecolor='none', edgecolors=color, linewidths=2)
ax.add_collection(p)
elif datasetType == 'captions':
for ann in anns:
print(ann['caption'])
pylab.rcParams['figure.figsize'] = (8.0, 10.0)
annFile = r'J:\keypoint_own\val.json'
img_prefix = 'J:\keypoint_own\pic_val'
coco = COCO(annFile)
catIds = coco.getCatIds(catNms=['person'])
imgIds = coco.getImgIds(catIds=catIds)
img = coco.loadImgs(imgIds[np.random.randint(0, len(imgIds))])[0]
I = io.imread('%s/%s' % (img_prefix, img['file_name']))
plt.imshow(I)
plt.axis('off')
ax = plt.gca()
annIds = coco.getAnnIds(imgIds=img['id'], catIds=catIds, iscrowd=None)
anns = coco.loadAnns(annIds)
print('anns:', anns)
coco.showAnns(anns)
plt.imshow(I)
plt.axis('off')
plt.show()
如果我们能在最后的验证代码中能够显示出我们标注好的图片即为成功啦,当然,这只是数据集的制作,后面还有还长的路要走,各位加油!