一、Rasa
Rasa是一个开源机器学习框架,用于构建上下文AI助手和聊天机器人。
Rasa有两个主要模块:
- Rasa NLU :用于理解用户消息,包括意图识别和实体识别,它会把用户的输入转换为结构化的数据。
- Rasa Core:是一个对话管理平台,用于举行对话和决定下一步做什么。
Rasa X 是一个工具,可帮助您构建、改进和部署由Rasa框架提供支持的AI Assistants。 Rasa X包括用户界面和REST API。
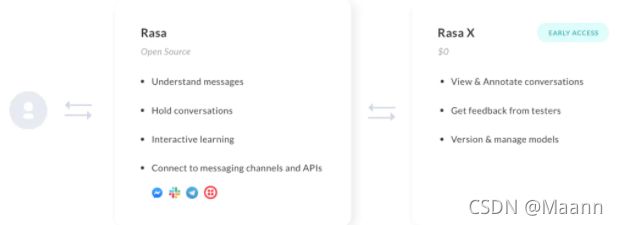
Rasa官方文档: Build contextual chatbots and AI assistants with Rasa
github地址:RasaHQ/rasa
pip安装:
$ pip install rasa_nlu
$ pip install rasa_core[tensorflow]
此图显示了使用Rasa构建的助手如何响应消息的基本步骤:
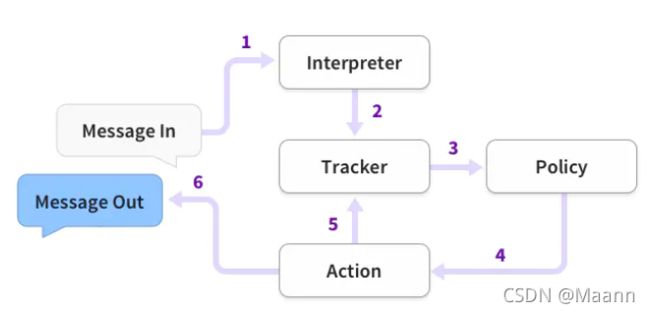
术语
- intents:意图
- pipeline:
- story:Core model 以训练“stories”的形式从真实的会话数据中学习。故事是用户和助手之间的真实对话.
- domain:定义了助手所处的universe:它应该获得的用户输入,应该能够预测的操作,如何响应以及要存储的信息
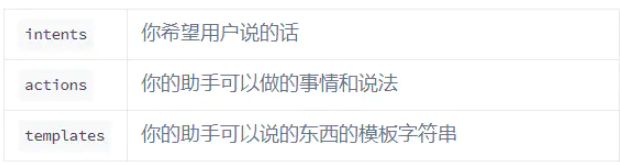
二、Rasa_NLU
Rasa NLU曾经是一个独立的库,但它现在是Rasa框架的一部分。
Rasa_NLU是一个开源的、可本地部署并配套有语料标注工具RASA NLU Trainer。其本身可支持任何语言,中文因其特殊性需要加入特定的tokenizer
作为整个流程的一部分。
Rasa NLU
用于聊天机器人中的意图识别和实体提取。例如,下面句子:
"I am looking for a Mexican restaurant in the center of town"
返回结构化数据:
{
"intent": "search_restaurant",
"entities": {
"cuisine" : "Mexican",
"location" : "center"
}
}
Rasa_NLU_Chi 作为 Rasa_NLU 的一个 fork 版本
,加入了jieba
作为中文的 tokenizer,实现了中文支持。
该部分简单介绍基于 Rasa_NLU_Chi
构建一个本地部署的特定领域的中文 NLU 系统的过程。
目标
- 输入: 中文测试文本
- 输出: 结构化的数据,识别出文本中对应的意图和实体
1. Pipeline
rasa nlu 支持不同的 Pipeline,其后端实现可支持spaCy、MITIE、MITIE + sklearn 以及 tensorflow
,其中 spaCy 是官方推荐的,另外值得注意的是从 0.12 版本后,MITIE 就被列入 Deprecated 了。
本例使用的 pipeline 为 MITIE+Jieba+sklearn
, rasa nlu 的配置文件为 config_jieba_mitie_sklearn.yml
如下:
language: "zh"
pipeline:
- name: "nlp_mitie"
model: "data/total_word_feature_extractor_zh.dat" // 加载 mitie 模型
- name: "tokenizer_jieba" // 使用 jieba 进行分词
- name: "ner_mitie" // mitie 的命名实体识别
- name: "ner_synonyms"
- name: "intent_entity_featurizer_regex"
- name: "intent_featurizer_mitie" // 特征提取
- name: "intent_classifier_sklearn" // sklearn 的意图分类模型
2. 准备工作:
2.1 MITIE安装:
我们把所有分好词的语料文件放在同一个文件路径下。接下来我们要训练MITIE模型。
首先将MITIE clone下来:
$ git clone https://github.com/mit-nlp/MITIE.git
我们要使用的只是MITIE其中wordrep这一个工具。我们先build它。
$ cd MITIE/tools/wordrep
$ mkdir build
$ cd build
$ cmake ..
$ cmake --build . --config Release
$ cd MITIE
$ python setup.py build
$ python setup.py install
2.2 训练MITIE模型文件:
由于在pipeline中使用了MITIE
,所以需要一个训练好的MITIE模型(先进行中文分词)。MITIE模型是非监督训练得到的,类似于word2vec中的word embedding
,需要大量中文语料,训练该模型对内存要求较高,并且非常耗时,直接使用网友分享的中文的维基百科和百度百科语料生成的模型文件。
链接:https://pan.baidu.com/s/1kNENvlHLYWZIddmtWJ7Pdg 密码:p4vx
将该模型放在
data/total_word_feature_extractor_zh.dat
3. rasa_nlu 语料
得到MITIE词向量模型之后,就可以使用标注好语料训练Rasa NLU模型。
Rasa提供了数据标注平台: rasa-nlu-trainer
那标注好的数据是什么样的呢?
标注好的语料存储在json
文件中,具体格式如下所示,包含text
, intent
,entities
,实体中start
和end
是实体对应在text
中的起止index
。
以data/examples/rasa/demo-rasa_zh.json
为例:
{
"rasa_nlu_data": {
"common_examples": [
{
"text": "你好",
"intent": "greet",
"entities": []
},
{
"text": "我想找地方吃饭",
"intent": "restaurant_search",
"entities": []
},
{
"text": "我想吃火锅啊",
"intent": "restaurant_search",
"entities": [
{
"start": 2,
"end": 5,
"value": "火锅",
"entity": "food"
}
]
}
]
}
}
4. 训练模型
到目前,已经获取了训练所需的标注好的语料,以及词向量模型MITIE文件。接下来就可以训练Rasa_NLU模型了。
插一句 安装:
$ git clone https://github.com/crownpku/Rasa_NLU_Chi.git // clone 源码
$ cd Rasa_NLU_Chi
$ python setup.py install // 安装依赖
模型训练命令
python -m rasa_nlu.train -c sample_configs/config_jieba_mitie_sklearn.yml --data data/examples/rasa/demo-rasa_zh.json --path models --project nlu
所需参数:
- 训练配置文件:
-c
- 训练语料:
--data
- 模型保存路径:
--path
- 项目名称:
--project
模型训练完成后,会在--path
指定的路径下保存训练好的模型文件,如果训练时指定了模型名称(即--project),模型就会存储在[path_name]/[project_name]/model_**
目录中,如models/nlu/model_20190821-160150
结构如下:
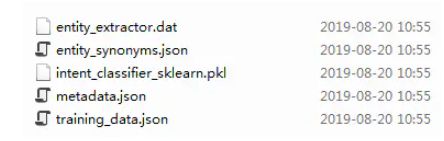
5. 测试验证
python -m rasa_nlu.server -c sample_configs/config_jieba_mitie_sklearn.yml --path models
- 测试服务(打开一个新的终端,使用curl命令获取结果)
curl -XPOST localhost:5000/parse -d '{"q":"明天天气预报", "project":"nlu", "model":"model_20190821-160150"}'
结果如下:
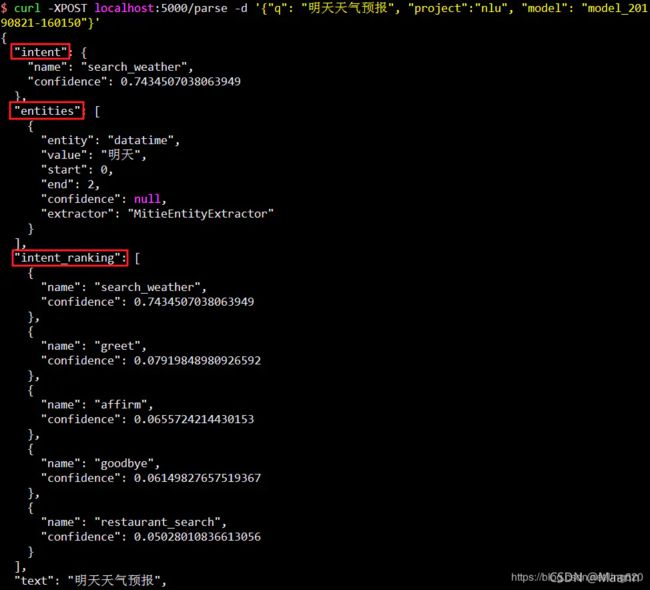
参考博文:
https://www.jianshu.com/p/ad11f5815447
https://blog.csdn.net/n66040927/article/details/108151192
三、在docker容器中构建、bug及解决方案
docker pull python:3.6-slim
本文以 python:3.6-slim 为例
1. 安装依赖
apt-get update
apt-get install -y git g++ cmake
error 1: The CXX compiler identification is unknown
(执行 cmake --build . --config Release 时)
解决方案:apt-get install g++
https://blog.csdn.net/qq_48169012/article/details/119509205
2. 安装MITIE
git clone https://github.com/mit-nlp/MITIE.git
cd MITIE/tools/wordrep
mkdir build
cd build
cmake ..
cmake --build . --config Release
cd MITIE
python setup.py build
python setup.py install
3. rasa_nlu_chi
git clone https://github.com/crownpku/rasa_nlu_chi.git
cd rasa_nlu_chi
python setup.py install
error 2: 安装过程中多次报错,如:RuntimeError: Python version >= 3.7 required.
可能因为版本不合适,需降低版本,参考:
pip install numpy==1.13
pip install matplotlib==3.0
pip install jieba
pip install rasa_nlu
pip install rasa_core[tensorflow]
4. 训练&测试验证
训练:
python -m rasa_nlu.train -c sample_configs/config_jieba_mitie_sklearn.yml --data data/examples/rasa/demo-rasa_zh.json --path models --project nlu
启动服务:
python -m rasa_nlu.server -c sample_configs/config_jieba_mitie_sklearn.yml --path models
测试:
curl -XPOST localhost:5000/parse -d '{"q":"明天天气预报", "project":"nlu", "model":"model_20190821-160150"}'
error 3: load() missing 1 required positional argument: ‘Loader‘
解决方案:降低 pyyaml 版本
pip install pyyaml==5.4.1
https://blog.csdn.net/qq_33580192/article/details/121080535
error 4: “error”: “bad value(s) in fds_to_keep”
解决方案:降低 scikit-learn 版本
pip install scikit-learn==0.19.2
https://blog.csdn.net/xuanzhuanguo/article/details/105033105
error 5: AttributeError: ‘SVC‘ object has no attribute ‘_probA‘"
解决方案:重新训练模型,在训练模型的过程中使用的是哪个版本的sklearn,那么在推理的时候需要使用同样版本的sklearn进行预测推理
https://blog.csdn.net/zhongkeyuanchongqing/article/details/119323909
附:python:3.6.15
absl-py==1.0.0
APScheduler==3.8.1
astor==0.8.1
attrs==21.2.0
Automat==20.2.0
backports.zoneinfo==0.2.1
boto3==1.20.3
botocore==1.23.3
cached-property==1.5.2
certifi==2021.10.8
cffi==1.15.0
characteristic==14.3.0
charset-normalizer==2.0.7
click==7.1.2
cloudpickle==0.6.1
colorclass==2.2.0
coloredlogs==10.0
colorhash==1.0.3
ConfigArgParse==0.13.0
constantly==15.1.0
cryptography==35.0.0
cycler==0.11.0
dataclasses==0.8
decorator==4.4.2
docopt==0.6.2
fakeredis==0.10.3
fbmessenger==5.6.0
Flask==1.1.4
Flask-Cors==3.0.10
Flask-JWT-Simple==0.0.3
future==0.17.1
gast==0.5.2
gevent==1.5.0
greenlet==1.1.2
grpcio==1.41.1
h5py==3.1.0
humanfriendly==10.0
hyperlink==21.0.0
idna==3.3
importlib-metadata==4.8.2
importlib-resources==5.4.0
incremental==21.3.0
itsdangerous==1.1.0
jieba==0.42.1
Jinja2==2.11.3
jmespath==0.10.0
jsonpickle==1.5.2
jsonschema==2.6.0
Keras-Applications==1.0.8
Keras-Preprocessing==1.1.2
kiwisolver==1.3.1
klein==17.10.0
Markdown==3.3.4
MarkupSafe==2.0.1
matplotlib==2.2.5
mattermostwrapper==2.2
mitie==0.7.0
mock==4.0.3
networkx==2.5.1
numpy==1.19.5
packaging==18.0
pathlib==1.0.1
pika==0.12.0
prompt-toolkit==3.0.22
protobuf==3.19.1
pycparser==2.21
pydot==1.4.2
PyJWT==1.7.1
pykwalify==1.7.0
pymongo==3.12.1
pyparsing==3.0.5
pyrsistent==0.18.0
python-dateutil==2.8.2
python-engineio==4.3.0
python-socketio==3.1.2
python-telegram-bot==11.1.0
pytz==2018.9
pytz-deprecation-shim==0.1.0.post0
PyYAML==5.4.1
questionary==1.10.0
rasa-core==0.14.5
rasa-core-sdk==0.14.0
rasa-nlu==0.15.1
redis==2.10.6
requests==2.26.0
requests-toolbelt==0.9.1
rocketchat-API==0.6.36
ruamel.yaml==0.15.100
s3transfer==0.5.0
scikit-learn==0.19.2
scipy==1.5.4
simplejson==3.17.5
six==1.16.0
slackclient==1.3.2
tensorboard==1.13.1
tensorflow==1.13.2
tensorflow-estimator==1.13.0
termcolor==1.1.0
terminaltables==3.1.0
tqdm==4.62.3
Tubes==0.2.0
twilio==6.63.2
Twisted==21.7.0
typing==3.10.0.0
typing-extensions==3.10.0.2
tzdata==2021.5
tzlocal==4.1
urllib3==1.26.7
wcwidth==0.2.5
webexteamssdk==1.6
websocket-client==0.54.0
Werkzeug==1.0.1
zipp==3.6.0
zope.event==4.5.0
zope.interface==5.4.0