- Softmax回归模型是logistic回归模型在多分类问题上的推广,在多分类问题中,类标签 y 可以取两个以上的值。
- Softmax回归模型对于诸如MNIST手写数字分类等问题是很有用的,该问题的目的是辨识10个不同的单个数字。
- Softmax回归是有监督的
import numpy as np
import matplotlib.pylab as plt
import copy
from scipy.linalg import norm
from math import pow
from scipy.optimize import fminbound,minimize
import random
def _dot(a, b):
mat_dot = np.dot(a, b)
return np.exp(mat_dot)
def condProb(theta, thetai, xi):
numerator = _dot(thetai, xi.transpose())
denominator = _dot(theta, xi.transpose())
denominator = np.sum(denominator, axis=0)
p = numerator / denominator
return p
''' 下面就是求得代价函数 '''
def costFunc(alfa, *args):
i = args[2]
original_thetai = args[0]
delta_thetai = args[1]
x = args[3]
y = args[4]
lamta = args[5]
labels = set(y)
thetai = original_thetai
thetai[i, :] = thetai[i, :] - alfa * delta_thetai
k = 0
sum_log_p = 0.0
for label in labels:
index = y == label
xi = x[index]
p = condProb(original_thetai,thetai[k, :], xi)
log_p = np.log10(p)
sum_log_p = sum_log_p + log_p.sum()
k = k + 1
r = -sum_log_p / x.shape[0]+ (lamta / 2.0) * pow(norm(thetai),2)
return r
class Softmax:
def __init__(self, alfa, lamda, feature_num, label_mum, run_times = 500, col = 1e-6):
self.alfa = alfa
self.lamda = lamda
self.feature_num = feature_num
self.label_num = label_mum
self.run_times = run_times
self.col = col
self.theta = np.random.random((label_mum, feature_num + 1))+1.0
def oneDimSearch(self, original_thetai,delta_thetai,i,x,y ,lamta):
res = minimize(costFunc, 0.0, method = 'Powell', args =(original_thetai,delta_thetai,i,x,y ,lamta))
return res.x
def train(self, x, y):
tmp = np.ones((x.shape[0], x.shape[1] + 1))
tmp[:,1:tmp.shape[1]] = x
x = tmp
del tmp
labels = set(y)
self.errors = []
old_alfa = self.alfa
for kk in range(0, self.run_times):
i = 0
for label in labels:
tmp_theta = copy.deepcopy(self.theta)
one = np.zeros(x.shape[0])
index = y == label
one[index] = 1.0
thetai = np.array[self.theta[i,:]]
prob = self.condProb(thetai, x)
prob = np.array([one - prob])
prob = prob.transpose()
delta_thetai=-np.sum(x*prob, axis=0)/x.shape[0]+self.lamda * self.theta[i, :]
self.theta[i, :] = self.theta[i,:] - self.alfa*np.array([delta_thetai])
i = i + 1
self.errors.append(self.performance(tmp_theta))
def performance(self, tmp_theta):
return norm(self.theta - tmp_theta)
def dot(self, a, b):
mat_dot = np.dot(a, b)
return np.exp(mat_dot)
def condProb(self, thetai, xi):
numerator = self.dot(thetai, xi.transpose())
denominator = self.dot(self.theta, xi.transpose())
denominator = np.sum(denominator, axis = 0)
p = numerator[0] / denominator
return p
def predict(self, x):
tmp = np.ones((x.shape[0], x.shape[1]+1))
tmp[:,1:tmp.shape[1]] = x
x = tmp
row = x.shape[0]
col = self.theta.shape[0]
pre_res = np.zeros((row, col))
for i in range(0, row):
xi = x[i, :]
for j in range(0, col):
thetai = self.theta[j, :]
p = self.condProb(np.array([thetai]), np.array([xi]))
pre_res[i, j] = p
r = []
for i in range(0, row):
tmp = []
line = pre_res[i, :]
ind = line.argmax()
tmp.append(ind)
tmp.append(line[ind])
r.append(tmp)
return np.array(r)
def evaluate(self):
pass
def sample(sample_num, feature_num, label_num):
n = int(sample_num / label_num)
x = np.zeros((n*label_num, feature_num))
y = np.zeros(n*label_num, dtype = np.int)
for i in range(0, label_num):
x[i*n : i*n+n, :] = np.random.random((n,feature_num)) + i
y[i*n : i*n+n] = i
return [x,y]
def save(name, x, y):
writer = open(name, 'w')
for i in range(0, x.shape[0]):
for j in range(0, x.shape[1]):
writer.write(str(x[i,j]) + ' ')
writer.write(str(y[i])+'\n')
writer.close()
def load(name):
x = []
y = []
for line in open(name, 'r'):
ele = line.split(' ')
tmp = []
for i in range(0, len(ele) - 1):
tmp.append(float(ele[i]))
x.append(tmp)
y.append(int(ele[len(ele) - 1]))
return [x,y]
def plotRes(pre, real, test_x, l):
s = set(pre)
col = ['r', 'b', 'g', 'y', 'm']
fig = plt.figure()
ax = fig.add_subplot(111)
for i in range(0, len(s)):
index1 = pre == i
index2 = real == i
x1 = test_x[index1, :]
x2 = test_x[index2, :]
ax.scatter(x1[:,0], x1[:,1],color=col[i],marker='v',linewidths=0.5)
ax.scatter(x2[:,0], x2[:,1],color=col[i],marker='.',linewidths=12)
plt.title('learning rating='+str(1))
plt.legend('c1:predict','c1:true',
'c2:predict','c2:true',
'c3:predict','c3:true',
'c4:predict','c4:true',
'c5:predict','c5:true'),shadow = true,loc = (0.01, 0.4)
plt.show()
if __name__ == '__main__':
[x,y] = load('data.txt')
index = range(0, len(x))
random.shuffle(index)
x = np.array(x)
y = np.array(y)
x_train = x[index[0:700],:]
y_train = y[index[0:700]]
softmax = Softmax(0.4, 0.0, 2, 5)
softmax.train(x_train, y_train)
x_test = x[index[700:1000],:]
y_test = y[index[700:1000]]
r = softmax.predict(x_test)
plotRes(r[:,0], y_test, x_test, softmax.alfa)
t = r[:,0] != y_test
o = np.zeros(len(t))
o(t) = 1
err = sum(o)
如果帮到你了,请赞赏支持:
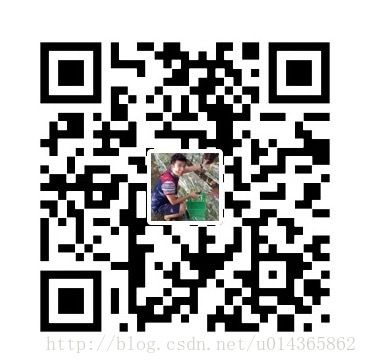