风能matlab仿真
DL DATATHON- AI4Impact
DL DATATHON- AI4影响
Published by Team AI Traders — Suyash Lohia, Nguyen Khoi Phan, Nikunj Taneja, Naman Agarwal and Mihir Gupta
AI交易员团队发布 -Suyash Lohia,Nguyen Khoi Phan,Nikonj Taneja,Naman Agarwal和Mihir Gupta
介绍 (Introduction)
Most sources of energy that are of renewable nature are reliant on the environment. The production of wind energy is largely dependent on the wind speed and its direction. A simple, yet integral concept that is necessary for the production of wind energy is the blowing of the wind. Hence, producing energy is only possible, when wind blows.
大部分具有可再生性质的能源都依赖于环境。 风能的产生在很大程度上取决于风速及其方向。 产生风能所必需的一个简单但不可或缺的概念就是吹风。 因此,只有在风吹动时才可能产生能量。
Wind energy as a renewable source of energy is an extremely clean fuel source since it causes very minimal air pollution contrary to the power plants that are reliant on the combustion of fossil fuels such as coal and natural gas. Additionally, due its clean property, wind energy production is a rather safe process since it does not involve the emission of any hazardous gasses. However, despite the various benefits of using wind energy, it is an unfavourable source. Wind energy production’s heavy dependence on the environment causes wind energy to be of an unpredictable nature as compared to non renewable sources of energy such as fossil fuels and nuclear energy.
风能作为一种可再生能源是一种非常清洁的燃料,因为它与依赖煤和天然气等化石燃料燃烧的发电厂相反,它造成的空气污染极少。 此外,由于其清洁特性,风能生产是一个相当安全的过程,因为它不涉及任何有害气体的排放。 但是,尽管使用风能有许多好处,但它是不利的来源。 与不可再生的能源(如化石燃料和核能)相比,风能生产对环境的严重依赖导致风能具有不可预测的性质。
利益相关者分析 (Stakeholder Analysis)
Wind energy production primarily consists of three core stakeholders. They are collectively accountable for establishing the production line for wind energy. The three key stakeholders are:
风能生产主要由三个核心利益相关者组成。 他们共同负责建立风能生产线。 三个主要利益相关者是:
Energy traders: these are companies which help predict and trade many aspects of energy, including the expected production of wind energy. In this project, the role of the energy trader is to help solve the shortfall through financial instruments.
能源贸易商 :这些公司可以帮助预测和交易能源的许多方面,包括风能的预期产量。 在该项目中,能源交易员的作用是通过金融工具帮助解决短缺问题。
Wind energy producers: These refer to the companies that manage and operate wind farms. They act as the intermediary between the grid operators and the energy traders, thereby selling the energy they produce to the Grid Operators.
风能生产商 :这些是指管理和运营风电场的公司。 它们充当电网运营商和能源贸易商之间的中介,从而将他们生产的能源出售给电网运营商。
Grid Operators: These refer to the various companies bestowed with the responsibility to manage the power grids over a nation or region for a given country or region. They buy pre-determined blocks of energy (measured in kWh) from the wind energy producers.
电网运营商:这些是指赋予特定国家或地区管理一个国家或地区电网的各种公司。 他们从风能生产商那里购买了预定的能量块(以千瓦时为单位)。
In principle, the role of the grid operators is to create a steady supply of electrical energy for the society. In fact, in cases of power outages or shortages, the government is liable to fine grid operators. In turn, this risk further percolates down to the energy traders and wind energy producers who are held responsible for the issues arising and are further made accountable to compensate for the shortfalls and the fines charged.
原则上,电网运营商的作用是为社会创造稳定的电能供应。 实际上,在停电或短缺的情况下,政府有责任对电网经营者处以罚款。 反过来,这种风险会进一步渗透到能源贸易商和风能生产商,他们对所出现的问题负责,并进一步承担责任,以弥补不足和罚款。
Problem Statement: Having understood the relationship between the various stakeholders in the process of wind energy production and its lead up to the marketplace, it is essential to understand the problem at hand. In the aforementioned setup, the wind energy producers forecast the energy they will produce in the future to the grid. This forecast is understood as a promissory minimum that the grid would expect from the energy producers. Differences in the actual energy produced in an interval in comparison with the forecast could be of two types:
问题陈述:在了解风能生产过程中各个利益相关者之间的关系以及将风能推向市场之前,了解当前的问题至关重要。 在上述设置中,风能生产商预测未来将向电网生产的能源。 该预测被理解为电网对能源生产商的期望下限。 间隔内产生的实际能量与预测值之间的差异可能有两种:
Shortfall — The scenario where the actual energy produced is lesser than the forecast. When this happens, the grid receives a fine from the wind energy producer.
短缺—实际产生的能量小于预测的情况。 发生这种情况时,电网将从风能生产商处罚款。
Excess — The scenario where the actual energy produced is greater than the forecast. When this happens, there is no direct fine, however, the wind energy producers do not receive any compensation for the excess energy produced.
多余 -实际产生的能量大于预测的情况。 发生这种情况时,不会直接处以罚款,但是,风能生产者不会就产生的多余能量获得任何补偿。
Our role as the energy trader is to provide service to our client, the wind producer in 2 ways:
我们作为能源贸易商的角色是通过两种方式向我们的客户(风能生产商)提供服务:
- Create the forecast for the wind producers to be handed to the grid. 创建将要移交给电网的风力发电商的预测。
- Purchase overpriced energy from the spot market and provide it to the grid in the event of a shortfall. 从现货市场购买价格过高的能源,并在出现短缺时将其提供给电网。
Objective
目的
In the capacity of energy traders, we were required to get hourly T+18 hour energy forecasts for energy production which would then be passed on the energy producers. In light of this, the main objective of the task was to maximise profits While doing so,
以能源贸易商的身份,我们被要求获得每小时T + 18小时的能源生产能源预测,然后将其传递给能源生产商。 有鉴于此,任务的主要目标是实现利润最大化。
Our objective in this project is to play the role of an energy trader. To simplify the problem, the trading algorithm (described below) is fixed and cannot be altered. Your goal is to get a T+18 hour energy forecast, every hour. The objective is to maximise profits for your client using your energy production forecast and the given trading algorithm.
我们在该项目中的目标是扮演能源贸易商的角色。 为了简化问题,交易算法(如下所述)是固定的,不能更改。 您的目标是每小时获得T + 18小时的能源预测。 目的是使用您的能源产量预测和给定的交易算法为您的客户最大化利润。
交易设置的更深层次的复杂性: (Deeper Intricacies of the Trading Setup:)
In line with the aforementioned objective, we were required to make a T+18 hour hour forecast of the energy for our client’s wind farms. As per the simulated set-up, our client was to be paid 10 euro cents/kWh sold to the grid. However, the sale of the energy units were subject to certain conditions. We would only sell to the grid operators, what we in the capacity of energy traders forecasted for that particular day and if our forecast was below the actual energy production, then we were liable to buy the deficit from the spot market at the rate of 20 euro cents/kWh. On the other hand, if the actual energy production exceeded the forecast then the excess would be observed by the grid, without the grid operator (i.e. our client) being compensated for the excess.
根据上述目标,我们被要求对客户风电场的能源进行T + 18小时的预测。 根据模拟设置,向客户出售给电网的价格为10欧分/ kWh。 但是,出售能源单位要遵守某些条件。 我们只会向电网运营商出售该天在能源交易员的预测中所能达到的水平,如果我们的预测低于实际的能源产量,则我们有责任以20的比率从现货市场购买赤字。欧分/ kWh。 另一方面,如果实际的能源产量超过了预测,那么电网将观察到过量,而电网运营商(即我们的客户)将无法获得补偿。
As for the time scales involved, the first 18 hours (also termed as the warmup period) were deemed void — such that no trades were to be performed during this period. After the warm-up period, we were expected to produce a T+18 hour forecast for the energy production, every hour. Furthermore, this trading period was to continue over weekends and public holidays which would end at the end of the evaluation period.
至于所涉及的时间尺度,最初的18个小时(也称为预热期)被认为是无效的,因此在此期间不得进行任何交易。 在预热期之后,我们预计每小时会产生T + 18小时的能源生产预测。 此外,该交易期将持续到周末和公共假日,直到评估期结束。
As a part of the project, we will compare the actual energy produced with the forecast we prepare on an hourly basis. In the event of the forecast being equal or lesser than the actual energy produced (excess), we are paid 10 euro cents per kWh. This amount is added to our cash at hand, increasing its positive balance. If, in case our cash at hand balance turned negative, we would have to settle our debt(accumulated negative balance) before receiving the amount from the grid. As mentioned previously, excess production over the forecast is not compensated.
作为项目的一部分,我们将每小时产生的实际能量与我们准备的预测进行比较。 如果预测值等于或小于实际产生的能量(过量),我们将为每度电支付10欧分。 这笔款项将添加到我们的手头现金中,从而增加其正余额。 如果在手头现金余额变为负数的情况下,我们必须先偿还债务(累计负余额),然后才能从电网接收金额。 如前所述,超出预测的过剩产量将不予补偿。
Contrarily, if there happens to be a shortfall we will have to ensure supplying the promised forecast to the grid by purchasing the shortfall at an overpriced rate from the spot market. Naturally, our cash at hand balance has to be positive for us to make purchases from the spot market. However, if this is not the case, we will be fined 100 euro cents per kWh for the amount of shortfall, which further gets added to our cumulative debt.
相反,如果碰巧出现短缺,我们将必须通过从现货市场以过高的价格购买短缺来确保将承诺的预测提供给电网。 当然,我们的手头现金余额对我们来说必须是正数,以便我们从现货市场购买商品。 但是,如果不是这种情况,我们将对不足额度每千瓦时罚款100欧分,这进一步加重了我们的累积债务。
We were given a sum of 10,000,000 Euro-cents as part of our ‘cash at hand’ at the start and were required to return this amount at the end of the evaluation period, alongside the remainder which were to be our client’s profits.
我们一开始就获得了10,000,000欧分作为“手头现金”的一部分,并被要求在评估期结束时返还这笔款项,其余部分将作为客户的利润。
数据集: (Datasets:)
In this project we have used two main datasets:
在这个项目中,我们使用了两个主要的数据集:
Wind Energy Production: The source that we have used to obtain data on wind energy production is the French energy transmission authority, Réseau de transport d’électricité (RTE). We have used near real time data, standardised and averaged to 1 hour. The data used is for the period of Jan 2017 — present, with the data represented in kWh. As the dataset comprises wind production data for the Ile-de-France near Paris, it has been named energy-ile-de-france.
风能生产:我们用于获取风能生产数据的来源是法国能源传输机构Réseaude transport d'électricité(RTE)。 我们使用了近实时数据,经过标准化,平均时间为1小时。 所使用的数据为2017年1月至今的数据,以kWh表示。 由于数据集包含巴黎附近法兰西岛的风力生产数据,因此已被命名为ile-france。
Wind Forecasts: In addition to the Wind energy production data, we were provided with wind forecasts in 8 locations in the Ile-de-France region, with each location representing a key wind farm. Similar to the production data, the forecast data is from January 2017 to the present.The wind forecast data has been taken from two different wind models, each of which studies two variables, wind speed and direction. The wind speed has been represented in m/s and direction as a bearing in degrees. The data is interpolated to the base of 1 hour and is updated 4 times on a daily basis. The data has been obtained from Terra Weather.
风力预报:除了风力发电量数据,我们还为法兰西岛地区的8个地点提供了风力预报,每个地点代表一个关键的风力发电场。 与生产数据类似,天气预报数据为2017年1月至今的数据。天气预报数据来自两个不同的风模型,每个模型研究两个变量:风速和风向。 风速以m / s表示,方向以度表示。 数据以1小时为基准进行插值,每天更新4次。 数据已从Terra Weather获得。
The 8 locations in the Ile-de-France region used for the project are- Guitrancourt, Lieusaint, Les Vingt Sétiers, Parc du Gatinais, Arville, Boissy-la-Rivière, Angerville 1, and Angerville 2.
法兰西岛大区中用于该项目的8个地点分别是Guitrancourt,Lieusaint,Les VingtSétiers,Parc du Gatinais,Arville,Boissy-la-Rivière,Angerville 1和Angerville 2。
探索性数据分析 (Exploratory Data Analysis)
For the purpose of understanding the underlying trends in the raw data sets better, we decided to conduct an in-depth exploratory data analysis. As part of this analysis; we plotted the daily, monthly, quarterly and annual results for the total wind production in 2017, 2018 and 2019 respectively. Furthermore, in order to elaborate, we have also provided a brief set of observations which highlight some of the crucial trends emerging from the respective plots in order to enhance the scope of this preliminary research.
为了更好地理解原始数据集中的潜在趋势,我们决定进行深入的探索性数据分析。 作为此分析的一部分; 我们分别绘制了2017年,2018年和2019年风电总产量的每日,每月,季度和年度结果。 此外,为了详细说明,我们还提供了一组简短的意见,以突出各个地块中出现的一些关键趋势,以扩大此初步研究的范围。
Annual Trend:
年度趋势:
As can be seen from the graph, the wind energy produced has increased in each successive year from 2017 to 2019. The rate of growth has increased over time, with the jump in 2018 to 2019 being greater than the increase in 2017 to 2018.
从图表中可以看出,从2017年到2019年,风能发电量逐年增加。增长率随着时间的推移而增加,2018年至2019年的跃升大于2017年至2018年的增长。
Monthly Trend: Energy Production at the monthly level for 2018, followed a classic V-shaped recovery — tipping the least in the month of July. The aggregate levels of energy production were higher in the second half of the year as compared to the first half.
月度趋势: 2018年的月度能源生产量呈典型的V形回升-在7月份最低。 与下半年相比,下半年能源生产的总水平更高。
Contrarily, Energy Production at the monthly level for both 2017 and 2019 followed a ‘Nike’ swoosh recovery commencing March. For the first two months, the production levels were relatively low. For both of these years, the minimum amount of energy was produced in the summer months, commencing May/ June. Thereafter, the production levels surged in the Autumn months and made their respective highs in the month of December.
相反,2017年和2019年的月度能源生产跟随着从3月份开始的“耐克”狂风复苏。 前两个月的生产水平相对较低。 从这两个年份开始,从5月/ 6月开始,夏季都产生了最小的能量。 此后,秋季的产量猛增,并在12月达到了各自的最高水平。
Daily Trend: While the day-to-day changes in production remained heavily volatile across all three years, one definite trend that was observed were the increasing volumes of energy production daily on an aggregate level between 2017–2019.
每日趋势:尽管在过去三年中,每日的生产变化仍然剧烈波动,但可以观察到的一个明确趋势是,2017-2019年期间每日的能源生产总量不断增加。
Average Hourly Trend: On plotting the average hourly energy production value in a month wise manner for all three years, we observe that there is a clear seasonal trend as the curves have nearly identical peaks and troughs. There is a clear increase in the magnitude of production every subsequent year, which can be attributed to the increase in wind energy farms in the region.
平均每小时趋势:在绘制所有三年的每月平均每小时能源生产值时,我们观察到明显的季节性趋势,因为曲线具有几乎相同的高峰和低谷。 随后的每一年产量都明显增加,这可以归因于该地区风力发电场的增加。
数据准备 (DATA Preparation)
Speed/Direction Correlation with Energy Production
速度/方向与能量产生的关系
We used linear interpolation for the wind speed and direction data to get standardised hourly data. We calculated the Pearson correlation of each feature with the label and found that the wind speed energy was highly correlated to the power output with the correlation coefficient being 0.8. On the other hand, the correlation between wind direction and the label was significantly low with an average value of 0.1 . However, as Pearson correlation method could be misleading when two features are not linearly correlated, we decided to calculate the distance correlation between wind direction and wind energy production since it measured both linear and nonlinear correlation. It was shown that wind direction did not correlate well with power output, The coefficient of approximately 0.2 out of 1 being very low still couldn’t be completely neglected.
我们对风速和风向数据使用了线性插值,以获得标准化的小时数据。 我们用标签计算了每个特征的皮尔逊相关性,发现风速能量与功率输出高度相关,相关系数为0.8 。 另一方面,风向与标签之间的相关性非常低,平均值为0.1 。 但是,由于当两个特征不线性相关时,Pearson相关方法可能会产生误导,因此我们决定计算风向与风能产量之间的距离相关性,因为它同时测量了线性和非线性相关性。 结果表明,风向与功率输出没有很好的相关性,仍然无法完全忽略约0.2 / 1的系数非常低。
Normalisation (Min-Max v/s Gaussian)
归一化(Min-Max v / s Gaussian)
It is imperative for any prediction model to have normalised data as inputs. On plotting our data using histograms, we observed that both energy production values and the wind speed values were following a bell shaped curve. Hence, we decided to normalise the data by subtracting the mean from the values and further dividing it by standard deviation.
任何预测模型都必须将规范化数据作为输入。 在使用直方图绘制数据时,我们观察到能量产生值和风速值都遵循钟形曲线。 因此,我们决定通过从值中减去平均值并进一步除以标准差来对数据进行归一化。
We further divided our data by a factor of 2. This allowed us to reduce the range and attain more condensed values, thereby enabling a better learning curve. Furthermore, since the data provided for direction did not follow a bell shaped curve, we simply decided to convert it into radians for ease of calculations in terms of sine and cosine, which also assisted us in reducing the range.
我们将数据进一步除以2 。 这使我们能够缩小范围并获得更多的精简值,从而获得更好的学习曲线。 此外,由于提供的方向数据未遵循钟形曲线,因此我们仅决定将其转换为弧度,以便于根据正弦和余弦进行计算,这也有助于我们缩小范围。
The above figure comparing Min-Max Scaling with Gaussian Scaling.
上图比较了最小-最大缩放比例和高斯缩放比例。
Issues with Data:
数据问题:
Missing historical data in wind/direction:
缺少风向的历史数据:
We were using two different models for measuring the wind speed and direction data which were using two different methods to calculate the values. Since, the correlation of the wind speeds/directions with the energy produced as well as the raw values were nearly identical in both models for each of the eight locations (see figure below), we decided to fuse the two and merge the dataset by taking an average of the wind speeds. All values which were either missing or 0 were ignored in the calculations.
我们使用两种不同的模型来测量风速和风向数据,分别使用两种不同的方法来计算值。 由于两个模型中八个位置中的每个位置的风速/风向与产生的能量以及原始值的相关性几乎相同(请参见下图),因此我们决定将两者融合并合并平均风速。 在计算中将忽略所有丢失或为0的值。
2. Interpolation of direction data:
2.方向数据的插值:
Interpolating direction data was a major challenge that we faced in our model. The primary reason behind this was the fact that direction, contrary to wind speed, is a circular statistic. Instead of converting the data to a standardised variable, we have used raw data for wind direction, by converting them to radians. Although there were methods to standardise the data, we chose not to. This is because of the low correlation of wind direction with energy production. Consequently, we did not use wind direction extensively in our model’s feature engineering.
插值方向数据是我们在模型中面临的主要挑战。 其背后的主要原因是,与风速相反的方向是一个循环统计量。 我们没有将数据转换为标准变量,而是通过将原始数据转换为弧度来使用风向。 尽管有使数据标准化的方法,但我们选择不这样做。 这是因为风向与能源生产之间的相关性较低。 因此,我们没有在模型的特征工程中广泛使用风向。
3. Handling delay of Real Time data of energy production:
3.能源生产实时数据的处理延迟:
In the deployment phase, we used 30 days of past data and 19 hours of forecast data (wind speed and direction) for our features and to handle the delay in the energy production data we used the default 72 hours “previous” interpolation (which gives the previous value during the gap, i.e., the last data point that comes before the desired timestamp in a 72 hour window).
在部署阶段,我们将30天的过去数据和19小时的预测数据(风速和风向)用于我们的功能,并且为了处理能源生产数据中的延迟,我们使用了默认的72小时“先前”插值法(间隔期间的前一个值,即72小时窗口中所需时间戳之前的最后一个数据点)。
特征工程 (Feature Engineering)
One should always remember to keep in mind the ‘curse of dimensionality’ while using neural networks in order to avoid faulty analysis. Consequently, it is essential to select the correct amount of features, before advancing to the modelling stage. It is therefore of paramount importance for us to select a certain number of features to use.
人们应该永远记住在使用神经网络时要牢记“维数的诅咒”,以避免错误的分析。 因此,在进入建模阶段之前,必须选择正确数量的特征。 因此,对于我们而言,选择要使用的某些功能至关重要。
Firstly, we decided that past data of wind energy production and wind speed, as indicated by the high correlation with our target, would be the two most important features to predict future wind energy production. An increase in speed led to a direct increase in the production values. Due to which we focused more on feature tweaking of Energy Production data and Wind speed data.
首先,我们认为,与目标高度相关的过去的风能发电和风速数据将是预测未来风能发电的两个最重要的特征。 速度的提高导致生产价值的直接增加。 因此,我们将重点更多地放在了能源生产数据和风速数据的功能调整上。
Secondly, we decided that we should not exclude wind direction data from the neural network since both wind speed and direction affect power output. However, due to the low correlation between wind direction and wind energy production, the inputs of this feature used to train the network were limited solely to future wind forecast as we do not want to put much emphasis on this feature.
其次,我们决定不应该从神经网络中排除风向数据,因为风速和风向都会影响功率输出。 但是,由于风向与风能产量之间的相关性较低,因此用于训练网络的此功能的输入仅限于未来的风能预测,因为我们不想过多地强调此功能。
Thirdly, we noticed a nonlinear relationship between wind speed and wind direction, thus using the product of wind speed and wind direction as a feature. Instead of higher orders of wind forecast data, cross-product of speed and direction data offered an easier approach for the neural network to learn the nonlinearity of weather data.
第三,我们注意到风速与风向之间存在非线性关系,因此将风速与风向的乘积作为特征。 代替高阶的天气预报数据,速度和方向数据的叉积为神经网络提供了一种更简单的方法来学习天气数据的非线性。
Finally, on conducting exploratory data analysis we came to the realisation that the Energy Production values and the wind speed had a seasonal trend and our primary aim was to capture this trend for more accurate predictions. Our data window was from the 30 days of past data to 19 days of forecast data giving our model only the necessary features and data requires to capture seasonal trends.
最后,在进行探索性数据分析时,我们意识到能源生产值和风速具有季节性趋势,而我们的主要目标是捕捉这种趋势以进行更准确的预测。 我们的数据窗口是从过去30天到19天的预测数据,这为我们的模型提供了捕获季节性趋势所需的必要特征和数据。
Difference Network
差异网络
Our neural network was a differencing model, of which the output is added to the average of wind energy data of T-0, T-1 to T-12 to make a prediction of 18 hours in advance. We decided to take the mean from T-0 to T-12 in order to reduce the “noise” of the dataset. Furthermore, we used Smojo programming language for modelling. The list of features is shown as follows:
我们的神经网络是一个差分模型,该模型的输出被添加到T-0,T-1至T-12的风能数据的平均值中,从而可以提前18小时进行预测。 我们决定采用T-0到T-12的平均值,以减少数据集的“噪音”。 此外,我们使用Smojo编程语言进行建模。 功能列表如下所示:
我们提供给网络的详细输入如下: (Detailed inputs that we feed to our network are listed as follows:)
: transform
: 转变
A:19:17 MEAN \ training labels, average for noise removal
A:19:17 MEAN \培训标签,用于去除噪音的平均值
A:18 \ testing labels
A:18 \测试标签
A:0:-12 MEAN \ y0, average for noise removal
A:0:-12 MEAN \ y0,去除噪音的平均值
\ — — — — FEATURES — — — -
\ - - - - 特征 - - - -
A:0:-30 24 momentum \ wind energy, T-0 — T-24, T-1 — T-25,…, T-6 — T-30
A:0:-30 24动量\风能,T-0-T-24,T-1-T-25,…,T-6-T-30
A:0:-30 24 force \ wind energy, 2nd order of 24 DIFFERENCE
A:0:-30 24力\风能,24阶二阶
A:0:-24 SKEWNESS \ wind energy — skewness
A:0:-24偏斜\风能–偏斜
A:0:-24 KURTOSIS \ wind energy — kurtosis
A:0:-24 KURTOSIS \风能-峰度
A:0:-24 MEAN \ wind energy — mean
A:0:-24平均值\风能-均值
A:0:-24 SD \ wind energy — standard deviation
A:0:-24 SD \风能-标准差
A:0:-24 RANGE \ wind energy — range
A:0:-24范围\风能-范围
A:0:-719 MEAN \ wind energy — mean, past 30 days
A:0:-719平均值\风能-过去30天的平均值
A:0:-719 SD \ wind energy — standard deviation, past 30 days
A:0:-719 SD \风能-标准偏差,过去30天
A:0:-719 RANGE \ wind energy — range, past 30 days
A:0:-719 RANGE \风能-范围,过去30天
A:0:-24 \ wind energy
A:0:-24 \风能
B:19:17 MEAN \ forecasted wind speed — mean
B:19:17 MEAN \预测风速-均值
B:18 \ forecasted wind speed
B:18 \预测风速
B:0:-24 MAX \ past wind speed — max
B:0:-24 MAX \过去的风速-最大
B:0:-24 MIN \ past wind speed — min
B:0:-24 MIN \过去的风速—分钟
B:-5:-7 MEAN \ past wind speed — mean
B:-5:-7平均值\过去的风速-均值
C:19:17 MEAN \ forecasted wind direction — mean
C:19:17 MEAN \预测风向-均值
C:18 \ forecasted wind direction
C:18 \预测风向
D:19:17 MEAN \ product of forecasted speed and direction
D:19:17 MEAN \预测速度和方向的乘积
D:18 \ product of forecasted speed and direction
D:18 \预测速度和方向的乘积
训练与预测 (Training and Prediction)
For the training and prediction we tried myriad different configurations, network architectures, dimensionality reduction techniques, scaling methods etc. After over 100 such test cases we obtained our best configuration with the following specifications.
为了进行培训和预测,我们尝试了无数种不同的配置,网络体系结构,降维技术,缩放方法等。经过100多个此类测试案例,我们获得了具有以下规格的最佳配置。
Deep Learning Neural Network Flowchart
深度学习神经网络流程图
The above figure represents the architecture of our neural network prediction model including the datasets and the important statistics fed.
上图代表了我们的神经网络预测模型的体系结构,其中包括数据集和重要的统计数据。
Neural Network Specifications
神经网络规范
After hyperparameter tuning we observed that a neural network of 4 layers followed by a Linear Combinator (LC) gives the most accurate predictions. A deep network with more layers led to overfitting and memorisation of the training samples whereas a really shallow network didn’t fit the actual data so well. On researching and consulting with our trainers we found that the optimum layer-by-layer shrinking factor was around ⅔ and the neural network size / number of perceptrons in the first layer was 128.
经过超参数调整后,我们观察到4层神经网络以及线性组合器(LC)给出了最准确的预测。 多层的深层网络导致训练样本的过度拟合和记忆化,而真正浅层的网络则无法很好地拟合实际数据。 通过与我们的培训师进行研究和咨询,我们发现最佳的逐层收缩因子约为1/3,并且第一层的神经网络大小/感知器数量为128。
Test Loss
测试损失
The persistence loss to be beaten was 0.11117 and the best tost loss obtained after repeated training and configuration tweaking is 0.0443546 .
被击败的持久性损失为0.11117 ,经过反复训练和构形调整后的最佳面包损失为0.0443546 。
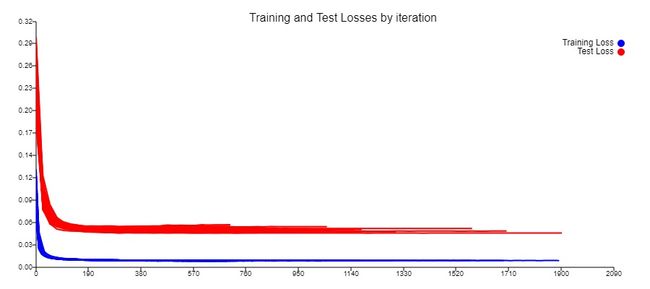
As seen from the figure and the configurations result tables, we beat the persistence result by a relative margin of 60.1 % . Furthermore, we were also able to marginally reduce the test loss to approximately 0.040. However, it induced comparatively more outliers and an additional lag.
从该图和配置结果表可以看出,我们以60.1%的相对余量击败了持久性结果。 此外,我们还能够将测试损失略微降低至约0.040。 但是,它引起了更多的异常值和额外的滞后。
Predictions:
预测:
We plotted graphs for Actual vs. Training Prediction, Action vs Testing Prediction and a Lag Correlation graph between Training and Testing phase to visually validate our results.
我们绘制了实际与训练预测,动作与测试预测的图表以及训练与测试阶段之间的滞后相关图,以直观地验证我们的结果。
As seen from Fig X. the training predictions mostly fit the actual data leading to finer training. However, the test predictions on the other hand rigidly followed the peaks and valleys of the actual data, thereby leading in slightly under-predicting.
从图X可以看出,训练预测大多适合实际数据,从而可以进行更精细的训练。 但是,另一方面,测试预测严格遵循实际数据的峰值和谷值,从而导致预测不足。
We further plotted scatter plots to check the distribution of our best configuration and observe the nature of all prevalent outliers
我们进一步绘制了散点图,以检查最佳配置的分布并观察所有流行异常值的性质
As seen from the above figures, while on the one hand, the Actual vs Training Predictions plot is roughly a straight line with very few outliers, on the other the Actual vs Testing Predictions Plot had a majority of points — either really near to the red line or below it. This was an indication of the fact that our model was under-predicting more than it was over predicting.
从以上数据可以看出,一方面,实际与训练预测图之间的直线大致是很少的异常值;另一方面,实际与测试预测图之间的关系主要是点–要么实际上接近红色线或下方。 这表明我们的模型对预测的误解多于对预测的误解。
The Lagged Correlation Graph depicted that both the Training and the Testing curve had a zero lag peak with almost similar heights. This was an indication of the fact that there was no expedition or delay in our predictions ultimately leading to more accurate results.
滞后相关图描述了训练曲线和测试曲线都具有零延迟峰,高度几乎相似。 这表明以下事实:我们的预测没有任何探索或延迟,最终会导致更准确的结果。
Judgement Calls made based on Problem Specification
根据问题说明进行判断调用
The trading algorithm defined for wind energy trading penalises over-prediction more than under-prediction with a ratio of 2:1. Due to this reason, we tweaked our model in such a manner that over-prediction is more harshly penalised. This can also be seen from the Actual vs Test Prediction Line Graph and Scatter Plot. Our total profit improved by approximately 23 % on adopting this strategy.
为风能交易定义的交易算法会以2:1的比率对高估多于低估进行惩罚。 由于这个原因,我们对模型进行了调整,以至于过高的预测更为严厉。 这也可以从实际与测试预测线图和散点图看出。 采用这一策略,我们的总利润提高了约23% 。
Since our goal was to maximise profit, we took the decision to pursue a fine balance between the test loss and the lagged correlation curve. A lower loss, was in most cases leading to a worse correlation curve. We prioritised the lagged correlation curve over reducing test loss as the trading algorithm is very volatile and due to its high sensitivity, even a minor lag would significantly change the profit for worse.
由于我们的目标是使利润最大化,因此我们决定在测试损失和滞后的相关曲线之间寻求良好的平衡。 较低的损耗在大多数情况下会导致较差的相关曲线。 由于交易算法非常不稳定,并且由于其高灵敏度,我们优先考虑滞后相关曲线,以降低测试损失,即使是很小的滞后也会严重改变利润。
局限性 (Limitations)
Our model tends to under-predict by a large margin during high energy production phases (especially above 35 kWh). Additionally, it sometimes fails to efficiently capture a downward trend, possibly due to lag in the predictions. These might be because of the low production capacity pattern in 2017 that the model learns that affects the predictions during high production phase.
在高能发电阶段(尤其是35 kWh以上),我们的模型往往会出现较大幅度的预测不足。 另外,有时由于预测的滞后,有时无法有效捕获下降趋势。 这可能是由于该模型了解到的2017年低产能模式会影响高产能阶段的预测。
To tackle these limitations, we think of implementing a loss function using weighted mean squared error that puts more weight on the squared error of energy data of higher values. Another approach is to exclude data in 2017 and use a loss function that penalises over-prediction more.
为了解决这些局限性,我们考虑使用加权均方误差来实现损失函数,该函数将更大的权重放在较高值的能量数据的平方误差上。 另一种方法是在2017年排除数据,并使用损失函数来惩罚过度预测。
结论与教训 (Conclusion and Lessons)
To conclude with, we would like to highlight all of the crucial steps that we took as well as the major lessons that we learnt while analysing, processing, training and testing the data. The first step we undertook was to conduct the exploratory data analysis for the purpose of understanding the underlying trends in the data and subsequently plot the frequency distributions of the raw data in order to decide which normalisation to use. Among some of the most important observations made, were the facts that as per the annual trend plotted, energy produced in every successive year was increasing over time and as per the quarterly and daily trends produced, the levels of volatility and volume produced of energy were far greater in 2018 and 2019 as compared to 2017.
最后,我们要重点介绍我们在分析,处理,训练和测试数据时所采取的所有关键步骤以及所学的主要课程。 我们进行的第一步是进行探索性数据分析,以了解数据的潜在趋势,然后绘制原始数据的频率分布,以决定使用哪种归一化方法。 在一些最重要的观察结果中,有一个事实是,按照年度趋势绘制,每隔一年的能源产量随着时间的推移而增加,并且按照产生的季度和每日趋势,能源的波动水平和发电量与2017年相比,2018年和2019年的数字要大得多。
Next, we used linear interpolation for the wind speed and the wind direction data to get the standardised hourly data. As per our findings, we found a high correlation between wind speed and the power output but a relatively lower correlation between wind direction and power output. However, provided that we were using the Pearson correlation method which could be misleading in case of non-linear correlation between two features — we decided to calculate the distance correlation between wind direction and wind energy production. Having done so, we eventually concluded that although there wasn’t a strong correlation between wind direction and power output, we still couldn’t totally neglect the feature due the presence of a minimalistic correlation.
接下来,我们对风速和风向数据使用线性插值,以获得标准化的小时数据。 根据我们的发现,我们发现风速与功率输出之间的相关性较高,而风向与功率输出之间的相关性相对较低。 但是,假设我们使用的Pearson相关方法在两个要素之间存在非线性相关的情况下可能会产生误导,因此我们决定计算风向与风能产量之间的距离相关性。 这样做之后,我们最终得出结论,尽管风向与功率输出之间没有很强的相关性,但是由于存在极简相关性,我们仍然不能完全忽略该特征。
After this we decided on the best technique to normalise our data. Having plotted the data using histograms, we observed that both energy production values and the wind speed values were following a bell shaped curve because of which we decided to subtract the values by the mean and divide it further by the SD. We further divided these values by 2 to condense the range. As for the data on direction, we simply decided to convert into radians for the ease of calculation. However, it must be highlighted that we did face some issues with the data such as the presence of missing historical data, dealing with interpolating the direction data and handling the delay of real time data for energy production.
之后,我们决定采用最佳技术对数据进行标准化。 使用直方图绘制数据后,我们观察到能量产生值和风速值均遵循钟形曲线,因此我们决定将这些值除以平均值,再除以SD。 我们进一步将这些值除以2以压缩范围。 至于方向数据,为了简化计算,我们只是决定将其转换为弧度。 但是,必须强调的是,我们确实在数据方面遇到了一些问题,例如缺少历史数据,处理方向数据的插值以及处理用于发电的实时数据的延迟。
Our extensive data analysis helped us with the feature engineering and we were able to select useful features rather early in the process. Apart from the wind energy production, wind speed and wind direction data, to handle the non-linear relationship between the wind speed and direction, we used the product of the two as a feature as well. Furthermore, our neural network was based in a differencing model with the mean from T-0 to T-12 taken to reduce the noise of the dataset. During the training and prediction phase we tried a variety of configurations and dimensionality reduction techniques to conclude that a neural network of 4 layers followed by a Linear combinator was giving us the most accurate predictions. Also we concluded that the optimum layer-by-layer shrinking factor was around ⅔ and the neural network size / number of perceptrons in the first layer was 128. Having used all of the aforementioned specifications we finally arrived at the point where our best configuration beat persistence result by a relative margin of 60.1%.
我们广泛的数据分析帮助我们进行了功能设计,并且能够在此过程的早期选择有用的功能。 除了产生风能,风速和风向数据以外,为了处理风速和风向之间的非线性关系,我们还使用两者的乘积作为特征。 此外,我们的神经网络基于差分模型,取平均值从T-0到T-12以减少数据集的噪声。 在训练和预测阶段,我们尝试了多种配置和降维技术,得出的结论是,四层神经网络后接线性组合器,为我们提供了最准确的预测。 我们还得出结论,最佳的逐层收缩因子约为1/3,第一层的神经网络大小/感知器数为128。使用所有上述规范,我们终于到达了最佳配置的地步持久性的相对幅度为60.1%。
Lastly, having plotted the graphs for Actual vs. Training Prediction, Action vs Testing Prediction and the Lag Correlation, we observed that in case of the test predictions, our model was rigidly following the peaks and valleys of the actual data, thereby slightly under-predicting. This observation was also consistent with the results observed from the Actual vs Training Prediction scatter plot graph.
最后,在绘制了实际预测与训练预测,动作预测与测试预测以及滞后相关性的图表后,我们观察到在进行测试预测的情况下,我们的模型严格遵循实际数据的波峰和波谷,因此略低于-预测。 该观察结果也与从“实际与训练预测”散布图图中观察到的结果一致。
— — X— — — — — —X — — — — — — — — —X — — — — — — — — X — —
— — X — — — — — — — X — — — — — — — — — X — — — — — — — — — — — — X –
翻译自: https://medium.com/@lololnomu/wind-energy-forecasting-1306c3ccfc12
风能matlab仿真