想要快速的了解一个算法,最好的方式便是拿个例子手动进行实现算一遍。这里借鉴了网络上的一个例子,求解如下的一个函数:
f(x)=x∗sin(10∗π∗x)+2x∈[−1,2]
其函数图像为:
例子来源:
http://blog.csdn.net/emiyasstar__/article/details/6938608/
求解流程与概念
染色体(编码)
在遗传算法中,一个个体一般只包含一条染色体。染色体上包含这一组基因组。
- 基因 ( Gene ) :一个遗传因子。
- 染色体 ( Chromosome ) :一组的基因。
- 个体 ( individual ):单个生物。
- 群体:一群个体
在上述的例子中自变量只有x,所有只有一个gene,因此在本例子中:
一个个体=一条染色体=一个基因
将x表达为gene的过程,称之为编码,常见的编码格式有二进制编码和浮点编码。本文采用2进制编码:
- 设我们求解精度为 e=0.01 ,
- 那么我们需要将x的区间【-1,2】,切分成 (2−−1)/0.01=300 份。
- 又因为采用二进制编码所以实际需要的编码位数为: 28=256<300<29=512 故当我们需要精度为0.01时,采用二进制编码最少需要9位数。
- 那么实际的求解精度为:
e=3512≈0.00586
有编码就存在着解码,按照本文的例子,可以想到以下的映射:
000000000=−1111111111=2
因此可以得到以下的解码公式:
(111111111)into10∗e−1=512∗3512−1=2(000000000)into10∗e−1=0−1=−1
ps:忽略上述由二进制转换为十进制的写法细节,不知道怎么写其数学表达式。
其中编码和解码的R代码
GetCodeParameter <- function(e, limitX){
range <- limitX[2] - limitX[1]
splitNum <- range/e
bitsPower <- 1
while(2^bitsPower <= splitNum ){
bitsPower <- bitsPower + 1
}
xMax <- max(limitX)
e <- range/2^bitsPower
diff <- 2^bitsPower*e - xMax
c(bitsPower, e, diff)
}
DeCode <- function(x, limitX, codeParameter){
x <- strtoi(x, base = 2)
x <- x*codeParameter[2]-codeParameter[3]
x
}
- 1
- 2
- 3
- 4
- 5
- 6
- 7
- 8
- 9
- 10
- 11
- 12
- 13
- 14
- 15
- 16
- 17
- 18
- 19
- 20
- 21
- 22
- 23
- 24
- 25
- 26
- 27
- 28
- 29
- 30
- 31
- 1
- 2
- 3
- 4
- 5
- 6
- 7
- 8
- 9
- 10
- 11
- 12
- 13
- 14
- 15
- 16
- 17
- 18
- 19
- 20
- 21
- 22
- 23
- 24
- 25
- 26
- 27
- 28
- 29
- 30
- 31
适者生存
适者生存 ( The survival of the fittest ):对环境适应度高的个体参与繁殖的机会比较多,后代就会越来越多。适应度低的个体参与繁殖的机会比较少,后代就会越来越少。
适应度指的是求解的目标,该例子中适应度计算公式便是求解的目标:
f(x)=x∗sin(10∗π∗x)+2
其中 f(x) 便是适应度计算公式。
适者生存其实指的是对后代的一种选择策略,常见的选择策略有轮盘赌、锦标赛、精英保留策略。轮盘赌就是按照一定的概率抽取子代,重复n次,每个个体被抽中的概率为:
pi=f(xi)∑nj=1f(xj)
轮盘赌举例说明:
group <- CreateGroup(groupNum, codeParameter)
group
adaptive <- myFun(group)
existProb <- adaptive/sum(adaptive)
group <- sample(group, groupNum,prob = existProb, replace = T)
group
length(unique(group))
8
- 1
- 2
- 3
- 4
- 5
- 6
- 7
- 8
- 9
- 10
- 11
- 12
- 13
- 14
- 15
- 16
- 17
- 18
- 19
- 20
- 21
- 22
- 23
- 24
- 25
- 1
- 2
- 3
- 4
- 5
- 6
- 7
- 8
- 9
- 10
- 11
- 12
- 13
- 14
- 15
- 16
- 17
- 18
- 19
- 20
- 21
- 22
- 23
- 24
- 25
锦标赛进行优胜劣汰的方法是:每次从群体中随机抽取p个人,将p个人中适应度最好的保留下来,重复N次,得到N个保留下的个体形成下一代。
交叉
交叉指的是交换染色体片段产生后代两个新的后代,例如典型的单点交叉方式:随机选择两个个体进行交叉,按照以下的方式产生新的子代。
配上代码
candidate <- 1:groupNum
parentNum <- floor(groupNum/2)
parentInx1 <- sample(candidate, parentNum, replace = F)
parentInx2 <- sample(candidate[!candidate %in% parentInx1], parentNum, replace = F)
parentInx1
parentInx2
matingPoint <- sample(2:(codeParameter[1]-1), 1, replace = T)
matingPoint
previousGene_1 <- substr(parent1, 1, matingPoint[i2])
lastGene_1 <- substr(parent1, matingPoint[i2]+1, codeParameter[1])
previousGene_2 <- substr(parent2, 1, matingPoint[i2])
lastGene_2 <- substr(parent2, matingPoint[i2]+1, codeParameter[1])
child_1 <- paste(previousGene_1, lastGene_2, sep="")
child_2 <- paste(previousGene_2, lastGene_1, sep="")
child_1;child_2
- 1
- 2
- 3
- 4
- 5
- 6
- 7
- 8
- 9
- 10
- 11
- 12
- 13
- 14
- 15
- 16
- 17
- 18
- 19
- 20
- 21
- 22
- 23
- 24
- 25
- 26
- 1
- 2
- 3
- 4
- 5
- 6
- 7
- 8
- 9
- 10
- 11
- 12
- 13
- 14
- 15
- 16
- 17
- 18
- 19
- 20
- 21
- 22
- 23
- 24
- 25
- 26
交叉操作存在着多种方式,例如:多点杂交、均匀杂交,离散杂交、中间杂交、线性杂交和扩展线性杂交等算法。其中有些交叉操作是基于编码的方式的。
变异
变异的作用,指的是染色体的某个基因片段或者某个基因点发生突变。例如单点突变可以通过下图进行表示:
突变的作用,是希望能够摆脱局部最优点,往更好的地方去。但是效果具有很大的随机性。
mutationProb <- 0.01
mutationGene <- sample(c(0,1), groupNum, replace = T,
prob = c(1-mutationProb, mutationProb))
mutationGene
mutationIdx <- which(mutationGene==1)
lenMutation <- length(mutationIdx)
if (lenMutation> 0) {
matingPoint <- sample(1:codeParameter[1], lenMutation, replace = T)
for (i in 1:lenMutation){
group[mutationIdx[i]] <- Mutation(group[mutationIdx[i]], matingPoint[i])
}
}
- 1
- 2
- 3
- 4
- 5
- 6
- 7
- 8
- 9
- 10
- 11
- 12
- 13
- 14
- 15
- 16
- 17
- 18
- 19
- 1
- 2
- 3
- 4
- 5
- 6
- 7
- 8
- 9
- 10
- 11
- 12
- 13
- 14
- 15
- 16
- 17
- 18
- 19
总流程
遗传算法的求解过程,是一个不断重复的过程,其流程如图所示:
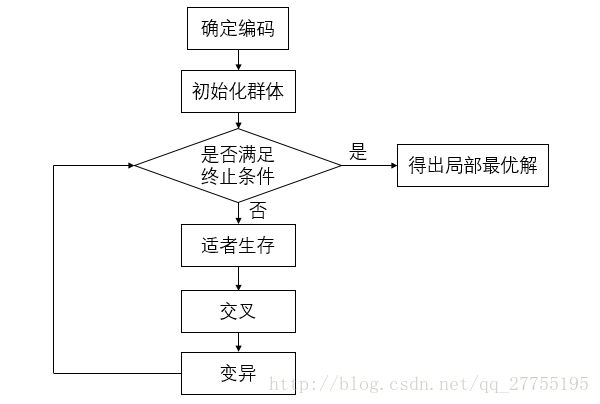
求解结果
贴图比较好展示:
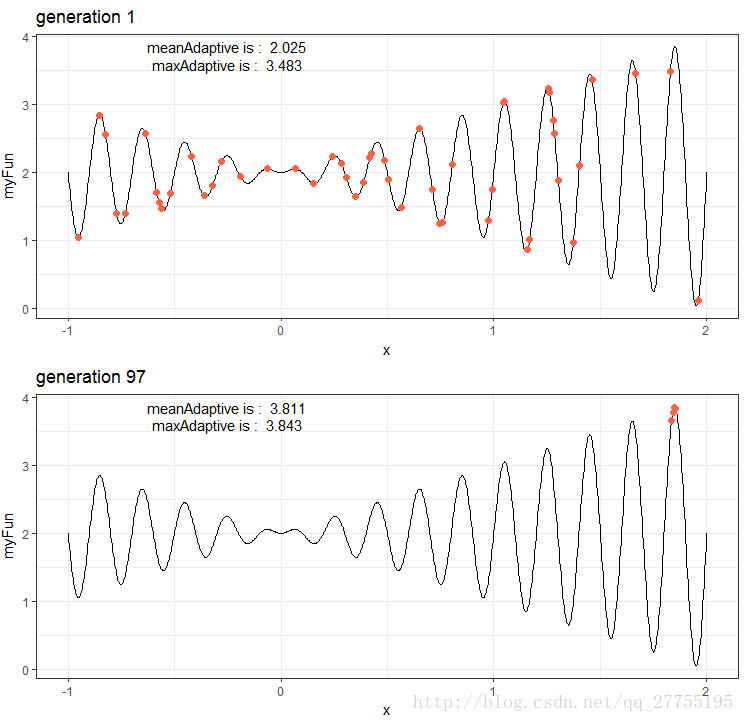
这里附上一个小知识:
假如在R中想要将其过程以一个动态过程展示出来,可以通过animation包进行实现。其中HTML格式输出的话,还能进行交互式的调整展示的速度。
其过程也很简单,每次迭代时,都将图画扔进一个list当中然后print出来。例如:
library(animation)
saveHTML({
for(i in 1:93){
print(plotGA[[i]])
}
})
效果如下:
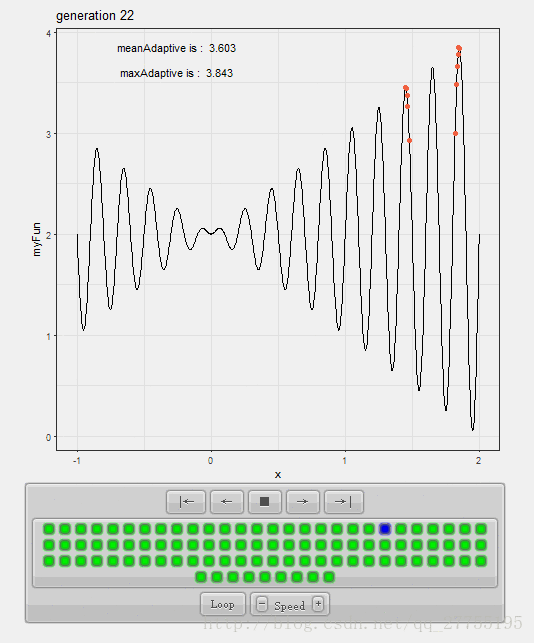
代码汇总
myFun <- function(x){
x*sin(10*pi * x) + 2
}
limitX <- c(-1, 2)
e <- 0.01
groupNum <- 50
mutationProb <- 0.01
generation <- 500
plotGA <- list()
codeParameter <- GetCodeParameter(e, limitX)
group <- CreateGroup(groupNum, codeParameter)
for(i in 1:generation){
deCodeGroup <- DeCode(group, limitX, codeParameter)
adaptive <- myFun(deCodeGroup)
existProb <- adaptive/sum(adaptive)
group <- sample(group, groupNum,prob = existProb, replace = T)
meanAdaptive <- mean(adaptive)
maxAdaptive <- max(adaptive)
main <- paste("generation", i)
plotGA[[i]] <- plotShow(x = deCodeGroup, y = adaptive, limitX, main, meanAdaptive, maxAdaptive)
candidate <- 1:groupNum
parentNum <- floor(groupNum/2)
parentInx1 <- sample(candidate, parentNum, replace = F)
parentInx2 <- sample(candidate[!candidate %in% parentInx1], parentNum, replace = F)
matingPoint <- sample(2:(codeParameter[1]-1), parentNum, replace = T)
newgroup <- NULL
for(i2 in 1:parentNum){
previousGene_1 <- substr(group[parentInx1[i2]], 1, matingPoint[i2])
lastGene_1 <- substr(group[parentInx1[i2]], matingPoint[i2]+1, codeParameter[1])
previousGene_2 <- substr(group[parentInx2[i2]], 1, matingPoint[i2])
lastGene_2 <- substr(group[parentInx2[i2]], matingPoint[i2]+1,codeParameter[1])
child_1 <- paste(previousGene_1, lastGene_2, sep="")
child_2 <- paste(previousGene_2, lastGene_1, sep="")
newgroup <- c(newgroup, child_1, child_2)
}
single <- which(!candidate %in% c(parentInx1, parentInx2))
group <- c(newgroup, group[single])
mutationGene <- sample(c(0,1), groupNum, prob = c(1-mutationProb, mutationProb), replace = T)
mutationIdx <- which(mutationGene==1)
lenMutation <- length(mutationIdx)
if( lenMutation> 0){
matingPoint <- sample(1:codeParameter[1], lenMutation, replace = F)
for (i in 1:lenMutation){
group[mutationIdx[i]] <- Mutation(group[mutationIdx[i]], matingPoint[i])
}
}
if((maxAdaptive - meanAdaptive) <= e) break()
}
- 1
- 2
- 3
- 4
- 5
- 6
- 7
- 8
- 9
- 10
- 11
- 12
- 13
- 14
- 15
- 16
- 17
- 18
- 19
- 20
- 21
- 22
- 23
- 24
- 25
- 26
- 27
- 28
- 29
- 30
- 31
- 32
- 33
- 34
- 35
- 36
- 37
- 38
- 39
- 40
- 41
- 42
- 43
- 44
- 45
- 46
- 47
- 48
- 49
- 50
- 51
- 52
- 53
- 54
- 55
- 56
- 57
- 58
- 59
- 60
- 61
- 62
- 63
- 64
- 65
- 66
- 67
- 68
- 69
- 70
- 71
- 72
- 73
- 74
- 1
- 2
- 3
- 4
- 5
- 6
- 7
- 8
- 9
- 10
- 11
- 12
- 13
- 14
- 15
- 16
- 17
- 18
- 19
- 20
- 21
- 22
- 23
- 24
- 25
- 26
- 27
- 28
- 29
- 30
- 31
- 32
- 33
- 34
- 35
- 36
- 37
- 38
- 39
- 40
- 41
- 42
- 43
- 44
- 45
- 46
- 47
- 48
- 49
- 50
- 51
- 52
- 53
- 54
- 55
- 56
- 57
- 58
- 59
- 60
- 61
- 62
- 63
- 64
- 65
- 66
- 67
- 68
- 69
- 70
- 71
- 72
- 73
- 74
其他求解方法
粒子群:
http://blog.csdn.net/qq_27755195/article/details/62216762
模拟退火:
http://blog.csdn.net/qq_27755195/article/details/62505046