UNet最初在2015年的MICCAI会议上发表,4年多的时间,论文引用量已经达到了9700次。
UNet成为了主要做医疗影像语义分割任务的尺度,同时也启发了研究者针对U型网络结构的研究,发表了基于UNet网络结构的改进方法的论文。
U型网络结构,最主要的两个特点是:: U型网络结构和跳过连接跳层连接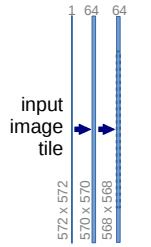从UNet网络中可以研磨,无论是下采样过程还是上采样过程,每一层都会连续进行两次卷积操作,这种操作在UNet网络中重复很多次,可以单独写一个DoubleConv模块:解释下,上述的火炬代码:torch.nn.Sequential是一个序列容器,模块会以其顺序的顺序被添加到容器中。上述上述的操作顺序是:卷积-> BN-> ReLU->卷积-> BN-> ReLU。
DoubleConv模块的in_channels和out_channels可以灵活设定,刹车扩展使用。
如上图所示的网络,in_channels添加1,out_channels为64。
输入图片大小为572 * 572,经过步长为1,padding为0的3 * 3卷积,得到570 * 570的功能图,再经过一次卷积得到568 * 568的功能图。
计算公式:O =(HF + 2×P)/ S + 1
H为输入特征图的大小,O为输出特征图的大小,F为卷积核的大小,P为padding的大小,S为步长。
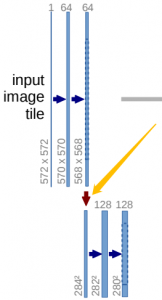这里的代码很简单,就是一个maxpool池化层,进行下采样,然后接一个DoubleConv模块。
至此,UNet网络的左半部分的下采样过程的代码都写好了,接下来是右半部分的上采样过程。
。[在这里插入图片描述(https://img-blog.csdnimg.cn/20200721180554562。上采样过程用到到的最大的当然就是上采样了,除了常规的上采样操作,还有进行特征的融合.PNG?X-OSS-过程=图像/水印,type_ZmFuZ3poZW5naGVpdGk,shadow_10,text_aHR0cHM6Ly9ibG9nLmNzZG4ubmV0L3dlaXhpbl80MzE0MzQxOQ ==,size_16,color_FFFFFF,t_70)代码复杂一些,我们可以分开来看,首先是__的初始化__初始化函数里定义的上采样方法以及卷积采用DoubleConv上采样,定义了两种方法:上采样和ConvTranspose2d,也就是双线性插值和反卷积。
=,size_16,color_FFFFFF,t_70)熟悉的双线性插值的朋友对于这幅图应该不陌生,简单地讲:已知Q11,Q12,Q21,Q22四个点坐标,通过Q11和Q21求R1,再通过Q12和Q22求R2,最后通过R1和R2求P,这个过程就是双线性插值。
对于一个feature map而言,其实就是在像素点中间补点,补的点的值是多少,是由相邻像素点的值决定的。
反卷积,顾名思义,就是反着卷积。卷积是让featuer map越来越小,反卷积就是让feature map越来越大,示意图:
下面蓝色为原始图片,周围白色的虚线方块为padding结果,通常为0,上面绿色为卷积后的图片。
这个示意图,就是一个从22的feature map->44的feature map过程。
在forward前向传播函数中,x1接收的是上采样的数据,x2接收的是特征融合的数据。特征融合方法就是,上文提到的,先对小的feature map进行padding,再进行concat。
用上述的DoubleConv模块、Down模块、Up模块就可以拼出UNet的主体网络结构了。UNet网络的输出需要根据分割数量,整合输出通道,结果如下图所示:
操作很简单,就是channel的变换,上图展示的是分类为2的情况(通道为2)。
import torch
import torch.nn as nn
import sys
import os.path as osp
from torch.nn import init
#https://github.com/ShawnBIT/UNet-family/blob/master/networks/UNet.py
"""跟MICCAI那篇文章一样的"""
def add_path(path):
if path not in sys.path:
sys.path.insert(0, path)
### initalize the module
def init_weights(net, init_type='normal'):
#print('initialization method [%s]' % init_type)
if init_type == 'kaiming':
net.apply(weights_init_kaiming)
else:
raise NotImplementedError('initialization method [%s] is not implemented' % init_type)
def weights_init_kaiming(m):
classname = m.__class__.__name__
#print(classname)
if classname.find('Conv') != -1:
init.kaiming_normal_(m.weight.data, a=0, mode='fan_in')
elif classname.find('Linear') != -1:
init.kaiming_normal_(m.weight.data, a=0, mode='fan_in')
elif classname.find('BatchNorm') != -1:
init.normal_(m.weight.data, 1.0, 0.02)
init.constant_(m.bias.data, 0.0)
### compute model params
def count_param(model):
param_count = 0
for param in model.parameters():
param_count += param.view(-1).size()[0]
return param_count
class unetConv2(nn.Module):
def __init__(self, in_size, out_size, is_batchnorm, n=2, ks=3, stride=1, padding=1):
super(unetConv2, self).__init__()
self.n = n
self.ks = ks
self.stride = stride
self.padding = padding
s = stride
p = padding
if is_batchnorm:
for i in range(1, n + 1):
conv = nn.Sequential(nn.Conv2d(in_size, out_size, ks, s, p),
nn.BatchNorm2d(out_size),
nn.ReLU(inplace=True), )
setattr(self, 'conv%d' % i, conv)
in_size = out_size
else:
for i in range(1, n + 1):
conv = nn.Sequential(nn.Conv2d(in_size, out_size, ks, s, p),
nn.ReLU(inplace=True), )
setattr(self, 'conv%d' % i, conv)
in_size = out_size
# initialise the blocks
for m in self.children():
init_weights(m, init_type='kaiming')
def forward(self, inputs):
x = inputs
for i in range(1, self.n + 1):
conv = getattr(self, 'conv%d' % i)
x = conv(x)
return x
class unetUp(nn.Module):
def __init__(self, in_size, out_size, is_deconv, n_concat=2):
super(unetUp, self).__init__()
self.conv = unetConv2(in_size + (n_concat - 2) * out_size, out_size, False)
if is_deconv:
self.up = nn.ConvTranspose2d(in_size, out_size, kernel_size=2, stride=2, padding=0)
else:
self.up = nn.Sequential(
nn.UpsamplingBilinear2d(scale_factor=2),
nn.Conv2d(in_size, out_size, 1))
# initialise the blocks
for m in self.children():
if m.__class__.__name__.find('unetConv2') != -1: continue
init_weights(m, init_type='kaiming')
def forward(self, high_feature, *low_feature):
outputs0 = self.up(high_feature)
for feature in low_feature:
outputs0 = torch.cat([outputs0, feature], 1)
return self.conv(outputs0)
class UNet(nn.Module):
def __init__(self, n_channels=1, n_classes=2, feature_scale=1, is_deconv=True, is_batchnorm=True):
super(UNet, self).__init__()
self.in_channels = n_channels
self.feature_scale = feature_scale
self.is_deconv = is_deconv
self.is_batchnorm = is_batchnorm
filters = [64, 128, 256, 512, 1024]
filters = [int(x / self.feature_scale) for x in filters]
# downsampling
self.maxpool = nn.MaxPool2d(kernel_size=2)
self.conv1 = unetConv2(self.in_channels, filters[0], self.is_batchnorm)
self.conv2 = unetConv2(filters[0], filters[1], self.is_batchnorm)
self.conv3 = unetConv2(filters[1], filters[2], self.is_batchnorm)
self.conv4 = unetConv2(filters[2], filters[3], self.is_batchnorm)
self.center = unetConv2(filters[3], filters[4], self.is_batchnorm)
#权重层冻结
# for p in self.parameters():
# p.requires_grad = False
# upsampling
self.up_concat4 = unetUp(filters[4], filters[3], self.is_deconv)
self.up_concat3 = unetUp(filters[3], filters[2], self.is_deconv)
self.up_concat2 = unetUp(filters[2], filters[1], self.is_deconv)
self.up_concat1 = unetUp(filters[1], filters[0], self.is_deconv)
# final conv (without any concat)
self.final_ = nn.Conv2d(filters[0], n_classes, 1)
self.dropout = nn.Dropout(p=0.5)
# initialise weights
for m in self.modules():
if isinstance(m, nn.Conv2d):
init_weights(m, init_type='kaiming')
elif isinstance(m, nn.BatchNorm2d):
init_weights(m, init_type='kaiming')
def forward(self, inputs):
conv1 = self.conv1(inputs) #10,64,224,224
maxpool1 = self.maxpool(conv1) #10,64,112,112
conv2 = self.conv2(maxpool1) # 10,128,112,112
maxpool2 = self.maxpool(conv2) # 10,128,56,56
conv3 = self.conv3(maxpool2) # 10,256,56,56
maxpool3 = self.maxpool(conv3) #10,256,28,28
conv4 = self.conv4(maxpool3) # 10,512,28,28
maxpool4 = self.maxpool(conv4) # 10,512,14,14
center = self.center(maxpool4) # 10,1024,14,14
# center = self.dropout(center)
up4 = self.up_concat4(center, conv4) # 128*64*64 #16,256,32,32
up3 = self.up_concat3(up4, conv3) # 64*128*128 #16,128,64,64
up2 = self.up_concat2(up3, conv2) # 32*256*256 #16,64,128,128
# up2 = self.dropout(up2)
up1 = self.up_concat1(up2, conv1) # 16*512*512 #16,32,256,256
最终= self.final_(UP1)#16,4,256,256 回报最终#如果__name__ == '__main__': #打印( '####测试案例###')从torch.autograd进口变量###X =变量(torch.rand(2,1,64,64))。cuda()#模型= UNet()。cuda()#参数= count_param(模型)#y =模型(x)#打印('输出形状:' ,y.shape)#print ('UNet总参数:%.2fM(%d)'%(参数/ 1e6,参数))`''