文章目录
-
-
-
- 图像分类 Image Classification
- 图像分割 Image Segmentation
- 图像风格变换 Image Transformation with AnimeGAN
- 3D模型 3D models
图像分类 Image Classification
import gradio as gr
import torch
import requests
from torchvision import transforms
model = torch.hub.load('pytorch/vision:v0.6.0', 'resnet18', pretrained=True).eval()
response = requests.get("https://git.io/JJkYN")
labels = response.text.split("\n")
def predict(inp):
inp = transforms.ToTensor()(inp).unsqueeze(0)
with torch.no_grad():
prediction = torch.nn.functional.softmax(model(inp)[0], dim=0)
confidences = {labels[i]: float(prediction[i]) for i in range(1000)}
return confidences
demo = gr.Interface(fn=predict,
inputs=gr.inputs.Image(type="pil"),
outputs=gr.outputs.Label(num_top_classes=3),
examples=[["cheetah.jpg"]],
)
demo.launch()

图像分割 Image Segmentation
import gradio as gr
from transformers import pipeline
generator = pipeline('text-generation', model='gpt2')
def generate(text):
result = generator(text, max_length=30, num_return_sequences=1)
return result[0]["generated_text"]
examples = [
["The Moon's orbit around Earth has"],
["The smooth Borealis basin in the Northern Hemisphere covers 40%"],
]
demo = gr.Interface(
fn=generate,
inputs=gr.inputs.Textbox(lines=5, label="Input Text"),
outputs=gr.outputs.Textbox(label="Generated Text"),
examples=examples
)
demo.launch()
图像风格变换 Image Transformation with AnimeGAN
import gradio as gr
import torch
model2 = torch.hub.load(
"AK391/animegan2-pytorch:main",
"generator",
pretrained=True,
progress=False
)
model1 = torch.hub.load("AK391/animegan2-pytorch:main", "generator", pretrained="face_paint_512_v1")
face2paint = torch.hub.load(
'AK391/animegan2-pytorch:main', 'face2paint',
size=512,side_by_side=False
)
def inference(img, ver):
if ver == 'version 2 ( robustness, stylization)':
out = face2paint(model2, img)
else:
out = face2paint(model1, img)
return out
title = "AnimeGANv2"
description = "Gradio Demo for AnimeGanv2 Face Portrait. To use it, simply upload your image, or click one of the examples to load them. Read more at the links below. Please use a cropped portrait picture for best results similar to the examples below."
article = "Github Repo Pytorch
"
examples=[['groot.jpeg','version 2 ( robustness, stylization)'],['gongyoo.jpeg','version 1 ( stylization, robustness)']]
demo = gr.Interface(
fn=inference,
inputs=[gr.inputs.Image(type="pil"),gr.inputs.Radio(['version 1 ( stylization, robustness)','version 2 ( robustness, stylization)'], type="value", default='version 2 ( robustness, stylization)', label='version')],
outputs=gr.outputs.Image(type="pil"),
title=title,
description=description,
article=article,
examples=examples)
demo.launch()
3D模型 3D models
import gradio as gr
from transformers import DPTFeatureExtractor, DPTForDepthEstimation
import torch
import numpy as np
from PIL import Image
import open3d as o3d
from pathlib import Path
feature_extractor = DPTFeatureExtractor.from_pretrained("Intel/dpt-large")
model = DPTForDepthEstimation.from_pretrained("Intel/dpt-large")
def process_image(image_path):
image_path = Path(image_path)
image_raw = Image.open(image_path)
image = image_raw.resize(
(800, int(800 * image_raw.size[1] / image_raw.size[0])),
Image.Resampling.LANCZOS)
encoding = feature_extractor(image, return_tensors="pt")
with torch.no_grad():
outputs = model(**encoding)
predicted_depth = outputs.predicted_depth
prediction = torch.nn.functional.interpolate(
predicted_depth.unsqueeze(1),
size=image.size[::-1],
mode="bicubic",
align_corners=False,
).squeeze()
output = prediction.cpu().numpy()
depth_image = (output * 255 / np.max(output)).astype('uint8')
try:
gltf_path = create_3d_obj(np.array(image), depth_image, image_path)
img = Image.fromarray(depth_image)
return [img, gltf_path, gltf_path]
except Exception:
gltf_path = create_3d_obj(
np.array(image), depth_image, image_path, depth=8)
img = Image.fromarray(depth_image)
return [img, gltf_path, gltf_path]
except:
print("Error reconstructing 3D model")
raise Exception("Error reconstructing 3D model")
def create_3d_obj(rgb_image, depth_image, image_path, depth=10):
depth_o3d = o3d.geometry.Image(depth_image)
image_o3d = o3d.geometry.Image(rgb_image)
rgbd_image = o3d.geometry.RGBDImage.create_from_color_and_depth(
image_o3d, depth_o3d, convert_rgb_to_intensity=False)
w = int(depth_image.shape[1])
h = int(depth_image.shape[0])
camera_intrinsic = o3d.camera.PinholeCameraIntrinsic()
camera_intrinsic.set_intrinsics(w, h, 500, 500, w/2, h/2)
pcd = o3d.geometry.PointCloud.create_from_rgbd_image(
rgbd_image, camera_intrinsic)
print('normals')
pcd.normals = o3d.utility.Vector3dVector(
np.zeros((1, 3)))
pcd.estimate_normals(
search_param=o3d.geometry.KDTreeSearchParamHybrid(radius=0.01, max_nn=30))
pcd.orient_normals_towards_camera_location(
camera_location=np.array([0., 0., 1000.]))
pcd.transform([[1, 0, 0, 0],
[0, -1, 0, 0],
[0, 0, -1, 0],
[0, 0, 0, 1]])
pcd.transform([[-1, 0, 0, 0],
[0, 1, 0, 0],
[0, 0, 1, 0],
[0, 0, 0, 1]])
print('run Poisson surface reconstruction')
with o3d.utility.VerbosityContextManager(o3d.utility.VerbosityLevel.Debug):
mesh_raw, densities = o3d.geometry.TriangleMesh.create_from_point_cloud_poisson(
pcd, depth=depth, width=0, scale=1.1, linear_fit=True)
voxel_size = max(mesh_raw.get_max_bound() - mesh_raw.get_min_bound()) / 256
print(f'voxel_size = {voxel_size:e}')
mesh = mesh_raw.simplify_vertex_clustering(
voxel_size=voxel_size,
contraction=o3d.geometry.SimplificationContraction.Average)
bbox = pcd.get_axis_aligned_bounding_box()
mesh_crop = mesh.crop(bbox)
gltf_path = f'./{image_path.stem}.gltf'
o3d.io.write_triangle_mesh(
gltf_path, mesh_crop, write_triangle_uvs=True)
return gltf_path
title = "Demo: zero-shot depth estimation with DPT + 3D Point Cloud"
description = "This demo is a variation from the original DPT Demo. It uses the DPT model to predict the depth of an image and then uses 3D Point Cloud to create a 3D object."
examples = [["examples/1-jonathan-borba-CgWTqYxHEkg-unsplash.jpg"]]
iface = gr.Interface(fn=process_image,
inputs=[gr.Image(
type="filepath", label="Input Image")],
outputs=[gr.Image(label="predicted depth", type="pil"),
gr.Model3D(label="3d mesh reconstruction", clear_color=[
1.0, 1.0, 1.0, 1.0]),
gr.File(label="3d gLTF")],
title=title,
description=description,
examples=examples,
allow_flagging="never",
cache_examples=False)
iface.launch(debug=True, enable_queue=False)
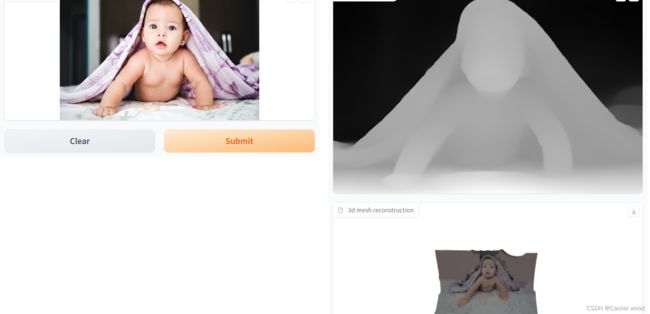