1. 引言
前序博客有:
- Lasso、Jolt 以及 Lookup Singularity——Part 1
- Lasso、Jolt 以及 Lookup Singularity——Part 2
相关论文见:
- Srinath Setty(微软研究中心)、Justin Thaler(a16z密码学研究中心以及Georgetown University)、Riad Wahby(Carnegie Mellon University)2023年论文《Unlocking the lookup singularity with Lasso》
- Arasu Arun(New York University)、Srinath Setty(微软研究中心)、Justin Thaler(a16z密码学研究中心以及Georgetown University)2023年论文《Jolt: SNARKs for Virtual Machines via Lookups》
相应的开源代码实现见:
- https://github.com/a16z/Lasso(Rust)
本文总体结构为:
- 何为lookup arguments?
- 何为Lasso/Jolt?
- Lasso细节
- Jolt细节
- 如何将Lasso看成是工具?
- 以及,lookup argument在zkVM之外的应用场景?
2. 何为lookup arguments?
lookup arguments可分为:
- 1)Unindexed lookup argument:
- 为subset relation proof,即,batch set-membership proof—— a a a为table t t t的某subset。
- 2)Indexed lookup argument:【Lasso和Jolt使用的是indexed lookup argument】
- 为对只读内存的读取。可将 t t t看成是内存, a i = t [ b i ] a_i=t[b_i] ai=t[bi]表示读取 b i b_i bi内存单元中的值。
以range check为例,lookup argument是指:
- 1)令 t = ( 0 , 1 , 2 , 3 , ⋯ , 2 128 − 1 ) t=(0,1,2,3,\cdots,2^{128}-1) t=(0,1,2,3,⋯,2128−1)为具有 N N N个table元素的向量(即 t t t为pre-determined table)。
- 2)令 a ∈ F m a\in\mathbf{F}^m a∈Fm为一组应包含在上述table的值列表。
- 3)Verifier V V V已知: a a a的承诺值 c m a cm_a cma。
- 4)Prover P P P声称: a a a中所有元素均在table t t t中。
即Prover P P P声称其知道 c m a cm_a cma的opening a a a,使得:
- 对于每个 i = 0 , ⋯ , m − 1 i=0,\cdots, m-1 i=0,⋯,m−1,存在某index b i ∈ { 0 , 1 , ⋯ , N − 1 } b_i\in\{0,1,\cdots, N-1\} bi∈{0,1,⋯,N−1},使得 a i = t [ b i ] a_i=t[b_i] ai=t[bi]。
- 5)通常将这种lookup argument称为unindexed lookup argument。
而indexed lookup argument是指待证明元素中还附加了索引值,indexed lookup argument定义为:【Verifier知道table t t t的succinct描述。】
- 1)令 t ∈ F n t\in\mathbf{F}^n t∈Fn为具有 N N N个table元素的向量(即 t t t为pre-determined table)。
- 2)Prover P P P对向量 ( ( a 1 , b 1 ) , ⋯ , ( a m , b m ) ) ((a_1,b_1),\cdots,(a_m,b_m)) ((a1,b1),⋯,(am,bm))进行承诺。
- 3)Prover P P P声称,对于 i = 1 , ⋯ , m i=1,\cdots,m i=1,⋯,m,有 a i = t [ b i ] a_i=t[b_i] ai=t[bi]。【即 a i a_i ai为value, b i b_i bi对应为table t t t的索引号,Prover P P P声称 a a a中所有元素均存在于 t t t中相应的index。】
3. 何为Lasso/Jolt?
Lasso:为一组(indexed)lookup argument:
- Lasso Prover P P P的速度要比之前的方案快一个量级。
- Prover P P P的关键瓶颈在于:承诺开销。
- Lasso Prover P P P对更少的field elements进行commit,且所有这些待承诺的field elements都是small的。
- No commitment to t t t needed for many tables。【对于(Jolt中需要的)大量的lookup tables,无需honest party来对table进行 commit和预处理。基于Lasso的Verifier可自行处理巨大table(仅需约50/100/200个field运算),而不是接收table的承诺值。】
- 支持巨大tables(可分解,或LDE-structured):
- Prover P P P的承诺开销为: O ( c ( m + N 1 / c ) ) O(c(m+N^{1/c})) O(c(m+N1/c))个field elements。
- 将table分解主要有2方面的Prover开销,通常取 c = 6 c=6 c=6来对这2方面开销进行平衡:
- 仅依赖于table size的开销
- 仅依赖于lookup次数的开销
Jolt:为新的zkVM技术:
- Jolt Prover P P P的承诺开销要比之前的方案低得多。
- 通过对单个指令的整个evaluation table的一次lookup,来实现Primitive instruction。
4. Lasso细节
4.1 Lasso开销细节
对size为 N N N、具有参数 c c c的table,做 m m m次indexed lookup:
- Lasso Prover P P P开销为:对 3 c m + c N 1 / c 3cm+cN^{1/c} 3cm+cN1/c个field elements进行commit。
- 所有field elements都是small的,即在set { 0 , 1 , ⋯ , m } \{0,1,\cdots,m\} {0,1,⋯,m}中:
- 基于MSM的多项式承诺方案,Lasso Prover P P P对每个(small)committed field element,仅需要约做1次group 运算。
目前基于MSM的多项式承诺方案有:
- KZG-based多项式承诺方案
- IPA/Bulletproofs多项式承诺方案
- Hyrax多项式承诺方案
- Dory多项式承诺方案
- Lasso Verifier V V V开销为:
- O ( log m ) O(\log m) O(logm)次field运算 和 hash evaluations(from Fiat-Shamir)。
- + 1个evaluation proof for a committed polynomial of size N 1 / c N^{1/c} N1/c。
- 通过composition/recursion,可将以上足够低的 Lasso Verifier V V V开销 进一步reduce。
当 c = 1 c=1 c=1时,对应的为Lasso的特例情况——Basic-Lasso:
- Basic-Lasso Prover P P P对 m + N m+N m+N个field elements进行commit。
- 这 m + N m+N m+N个field elements中,有很多为0值。在MSM-based多项式承诺方案中,对0值进行commit的开销也为0,即“free”。
- 这 m + N m+N m+N个field elements中,最多有 2 m 2m 2m个为非零值:
- 若每次读取的为不同的table cell,则有 m m m个field elements为1,其它的均为0。
4.2 将Lasso用于巨型table: c > 1 c>1 c>1
实际上,大多数大型lookup table都是可分解的:
- 可将对大型size为 N N N的table的一次lookup,分解为,对size为 N 1 / c N^{1/c} N1/c的table的约 c c c次lookup,然后"整理(collating)"这 c c c个lookup的结果 :
- Lasso使用sum-check protocol来"整理"这 c c c个lookup的结果。因此对Lasso Prover P P P来说,没有额外的承诺开销。
可将Lasso看成是 c > 1 c>1 c>1,将对单个大型、可分解table的lookup,reduce为,对多个small tables的 c c c个lookup:
- 对small tables,可使用任意lookup argument。
- 对于small tables,Lasso中使用Basic-Lasso lookup argument方案。
- 附加说明:small-table lookup argument必须是indexed。
- 目前已知的方案来将unindexed lookup argument 转换为 indexed lookup argument。
- 但是,这些已知方案要么无法保留table entries的“smallness”,要么无法保留big table的可分解性——原因在于,这些方案将indices和values "pack"为了单个field element。
【proof size不取决于不同table的数量,也不取决于small table的size。Verifier需在某random point上 evaluate每个small table的 multi-linear extension polynomial,small tables数量的增加会引起Verifier time的增加,但这些都是field运算,相比于其它密码学操作来说,这不会成为Verifier的瓶颈。
Jolt中有约45种不同的subtables。
】
4.3 背景知识:Grand Product Arguments
所有已知的lookup argument都采用了grand product argument:
- grand product argument是一种SNARK方案,用于证明 n n n个committed values的乘积。
- 当今流行的grand product argument,其Prover P P P需额外再对 n n n个值(partial products)进行commit。
- 这是没必要的。
- T13论文中:给出了GKR protocol的优化版本(所谓GKR protocol,为sum-check-based interactive proof for circuit evaluation):【对类似于binary tree of multiplication gates的电路进行了优化。】
- Prover P P P无承诺开销。
- Prover P P P做线性次数的field运算。
- Proof size/Verifier V V V time为 O ( log ( n ) 2 ) O(\log(n)^2) O(log(n)2)次field运算(以及hash evaluations from Fiat-Shamir):
- 远少于FRI的Proof size/Verifier V V V time。
- [Lee, Setty 2019]中将Verifier V V V开销进一步reduce为约 O ( log ( n ) ) O(\log(n)) O(log(n)),只需增加Prover P P P的一点点承诺开销。
4.4 Basic-Lasso关键点
- 对于很多现有的lookup arguments,若swap out the invoked grand product argument for T13,则Prover P P P仅需对small field elements进行commit。
- 详细可参看Ulrich Hab¨ock的 logUp-Multivariate lookups based on logarithmic derivatives:借助对数导数,将grand product argument 转换为了 grand sum argument。
- More involved than just a simple swap of a grand product argument。
- Lasso/Jolt需要使用indexed lookup argument,来将small-table lookup results “collating” into big-table results。
- 技术卖点:当前社区仍未充分利用sum-check的潜力来避免Prover P P P的开销。
- 关于Basic-Lasso的工作原理,可参看:
- Lasso、Jolt 以及 Lookup Singularity——Part 2
5. Jolt细节
当前VM execution的前端(front-end):
- Prover P P P声称运行某computer program for m m m steps:
- 该程序以某VM的汇编语言来编写。
- 流行的VM面向目标有:RISC-V、Ethereum Virtual Machine(EVM)。
- 当前,前端为每个computation step生成一个电路:
- 步骤1:指出在该step应执行哪个指令。
- 步骤2:执行该指令。
- 而Lasso将上面的 步骤2 替换为 a single lookup:
- 对于每个指令,该table中存储了该指令的整个evaluation table。
- 若指令 f f f有2个64-bit输入,则对于每个64-bit input pair ( x , y ) (x,y) (x,y),lookup table会存储所有的 f ( x , y ) f(x,y) f(x,y):
- 该lookup table size为 2 128 2^{128} 2128。
- Jolt中展示了,所有的RISC-V指令都是可分解的。
Jolt概览图为:
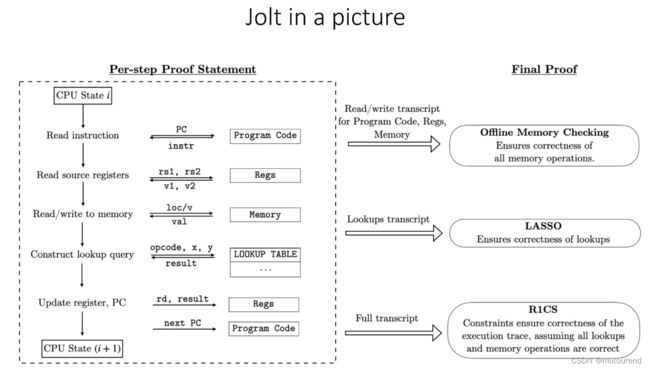
Jolt有望实现Barry Whitehat的Lookup Singularity愿景:
- 具有易审计、简单化、可扩展性等优势
- 性能优势
- 本质不同的构建zkVM的方式:
- 与人们现有在做的事情有很多相似之处。
- 人们愿景将类似bitwise-AND这样的计算函数转换为 对small tables的多次lookup,并将结果合并。
- Jolt的关键不同之处在于:
- a)新的small-table lookup argument具有快得多的Jolt Prover P P P。
- b)新的small-table lookup argument为天然indexed。
- c)Jolt Prover P P P具有快得多的collating技术:
- 可对small-table lookup results进行“Free” multiply和add。
- Jolt的这些不同之处,使得可借助lookup 来实现VM emulation中的almost everything。
5.1 Jolt分解示例1——Bitwise-AND
对于有2个64-bit输入 x , y x,y x,y的bitwise-AND原酸,其分解思路为:
- 将 x , y x,y x,y分解为 c = 8 c=8 c=8个chunks,每个chunk有8 bit。
- 计算每个chunk的bitwise-AND
- 将各chunk结果拼接起来,即输出为:
∑ i = 1 8 8 i − 1 ⋅ bitwiseAND ( x i , y i ) \sum_{i=1}^{8}8^{i-1}\cdot \text{bitwiseAND}(x_i,y_i) ∑i=188i−1⋅bitwiseAND(xi,yi)
为避免需要honest-party来对sub-table进行commit:
- bitwiseAND ( x i , y i ) = ∑ j = 1 8 2 j − 1 ⋅ x j ⋅ y j \text{bitwiseAND}(x_i,y_i)=\sum_{j=1}^{8}2^{j-1}\cdot x_j\cdot y_j bitwiseAND(xi,yi)=∑j=182j−1⋅xj⋅yj为multilinear多项式,可 以少于25个field运算来evaluate。
- Lasso Verifier V V V需要知道该sub-table,转为,只需要知道该多项式的一个evaluation。
5.2 Jolt分解示例2——RISC-V Addition
对2个64-bit number x , y x,y x,y 求和,RISC-V规定其相加正确,并忽略任意“overflow bit”。
Jolt(通过对ancillary R1CS添加一个约束来)在有限域内计算 z = x + y z=x+y z=x+y,然后使用lookup来标识相应的overflow bit,若有overflow bit,则据此调整相应的结果。
- Jolt Prover P P P,对field element z = x + y z=x+y z=x+y的“limb-decomposition” ( b 1 , ⋯ , b c ) (b_1,\cdots,b_c) (b1,⋯,bc)进行commit。
- 令 M = 2 64 / c M=2^{64/c} M=264/c为每个limb的最大值。
- 向R1CS添加一个约束,以确认:
- 步骤1: z = ∑ j = 1 c M j − 1 ⋅ b j z=\sum_{j=1}^{c}M^{j-1}\cdot b_j z=∑j=1cMj−1⋅bj
- 步骤2:并对每个 b j b_j bj通过lookup into 存储了 { 0 , ⋯ , M − 1 } \{0,\cdots,M-1\} {0,⋯,M−1}的subtable,来做range check。
- 通过以上约束的步骤1和步骤2,就可确保 b 1 , ⋯ , b j b_1,\cdots,b_j b1,⋯,bj确实为 z z z的规定的limb-decomposition。
- 为识别overflow bit,仅需要做a lookup at index b c b_c bc, into a table whose i i i’th entry spits out the relevant high-order bit of i i i。
5.3 Jolt分解示例2——LESS THAN UNSIGNED
对2个64-bit输入 x , y x,y x,y做less than运算:
- 将 x , y x,y x,y分解为 c = 8 c=8 c=8个chunks,每个chunk有8 bit。
- 计算每个chunk的LESS-THAN(LT) 和 EQUALITY(EQ)
- 输出为: ∑ i = 1 8 2 i − 1 ⋅ LT ( x i , y i ) ∏ j = i + 1 8 EQ ( x j , y j ) \sum_{i=1}^{8}2^{i-1}\cdot \text{LT}(x_i,y_i)\prod_{j=i+1}^{8}\text{EQ}(x_j,y_j) ∑i=182i−1⋅LT(xi,yi)∏j=i+18EQ(xj,yj)
为避免对2个sub-table进行commit:
- EQ ( x j , y j ) = ∏ k = 1 8 ( x j , k y j , k + ( 1 − x j , k ) ( 1 − y j , k ) ) \text{EQ}(x_j,y_j)=\prod_{k=1}^{8}(x_{j,k}y_{j,k}+(1-x_{j,k})(1-y_{j,k})) EQ(xj,yj)=∏k=18(xj,kyj,k+(1−xj,k)(1−yj,k))
- LT ( x i , y i ) = ∑ k = 1 8 ( 1 − x i ) y i ∏ z = k 8 ( x i , k y i , k + ( 1 − x i , k ) ( 1 − y i , k ) ) \text{LT}(x_i,y_i)=\sum_{k=1}^{8}(1-x_i)y_i\prod_{z=k}^{8}(x_{i,k}y_{i,k}+(1-x_{i,k})(1-y_{i,k})) LT(xi,yi)=∑k=18(1−xi)yi∏z=k8(xi,kyi,k+(1−xi,k)(1−yi,k))
- 这些均为multilinear多项式,可 以少于50个filed运算来evaluate。
6. 如何将Lasso看成是工具?以及,lookup argument在zkVM之外的应用场景?
6.1 Lasso as a tool的直觉
- Lasso支持对field element的bit-decompositions的简单运算,无需Lasso Prover P P P对各个bit进行commit。
- sub-tables具有quickly-evaluable multilinear extension,若每个sub-table对应a simple function on the (bits of) the table indices:
- 从而可确保无需honest party在预处理时对这些sub-tables进行commit。
- 对2个field elements取值为 { 0 , 1 , ⋯ , 2 64 − 1 } \{0,1,\cdots,2^{64}-1\} {0,1,⋯,264−1}的bitwiseAND运算,相比于Plonk中每个加法或乘法门的开销,Lasso Prover 开销更低。
- 注意:Lookup arguments为规模经济。适于do many lookups into one table(即对同一函数的多次调用)。
6.2 将indexed lookup arguments看成是repeated function evaluation的SNARKs方案
之前有很多关于“SNARKs for repeated function evaluation”的研究:
- 基于很多不同输入 x 1 , ⋯ , x m x_1,\cdots,x_m x1,⋯,xm,计算同一函数 f f f。
- 可将其看成是“polynomial” amount of data parallelism:
- 若函数 f f f的输入长度为 n n n,则不同输入的数量为 m = poly ( n ) m=\text{poly}(n) m=poly(n)。
- 仍要求Prover P P P evaluate f f f in a very specific way:
- Executing a specific circuit to compute f f f。
看待lookup arguments新视角:
- 将lookup table看成是存储了某函数 f f f的所有evaluations
- lookup argument则为a SNARK for highly repeated evaluation of f f f:
- 令Prover证明,committed vector ( ( a 1 , f ( a 1 ) ) , ⋯ , ( a m , f ( a m ) ) ) ((a_1,f(a_1)),\cdots,(a_m,f(a_m))) ((a1,f(a1)),⋯,(am,f(am))),中包含了对不同输入 a 1 , ⋯ , a m a_1,\cdots,a_m a1,⋯,am的正确 f f f evaluations。
- Lasso Prover P P P的开销为 O ( c ( m + N 1 / c ) ) O(c(m+N^{1/c})) O(c(m+N1/c)),当lookup次数 m m m 不比 table size N N N,小太多时,Lasso Prover P P P是高效的:
- 即运行 f f f的次数 应为 exponential in the input size of f f f。
7. 小结
- Lasso适于对同一函数evaluate很多次的场景。
- zkVM只是Lasso的应用场景之一:
- 根据定义,VM抽象表示的是对primitive指令的重复执行的计算。
- 实现VM抽象通常具有大量的性能开销。
- 未来感兴趣的方向:
- 隔离计算中重复结构的其它更好方式
- 示例工作有:
- Bit-slicing
- evaluate 哈希函数或block cipher(如SHA/AES),可构建具有64个不同输入的Boolean circuit C C C来计算:
- 将每个输入的第一个bit pack进a single field element,将每个输入的第二个bit pack进a single field element,以此类推。
- 将circuit C C C中的每个AND 门,替换为bitwiseAND;将circuit C C C中的每个OR 门,替换为bitwiseOR等等。
- circuit C C C中每个gate的output为SHA/AES所有64 evaluations的one bit。
- 对circuit C C C运用Lasso。
参考资料
[1] Justin Thaler 2023年8月在zkStudyClub视频分享 zkStudyClub - Lasso/Jolt (Justin Thaler, Georgetown University/a16z)
相应slide见:zkStudyClub - Lasso/Jolt (Justin Thaler, GWU/a16z)
Justin Thaler系列博客
- SNARK Design
- Rollup项目的SNARK景观
- SNARK原理示例
- SNARK性能及安全——Prover篇
- SNARK性能及安全——Verifier篇
- sum-check protocol in zkproof
- sum-check protocol深入研究
- Lasso、Jolt 以及 Lookup Singularity——Part 1
- Lasso、Jolt 以及 Lookup Singularity——Part 2
lookup系列博客
- PLOOKUP
- PLOOKUP代码解析
- Efficient polynomial commitment schemes for multiple points and polynomials学习笔记
- PLONK + PLOOKUP
- PlonKup: Reconciling PlonK with plookup
- PLONK: permutations over lagrange-bases for oecumenical noninteractive arguments of knowledge 学习笔记
- Plonk代码解析
- RapidUp: Multi-Domain Permutation Protocol for Lookup Tables学习笔记
- Lookup argument总览
- Halo2 学习笔记——设计之Proving system之Lookup argument(1)
- logUp-Multivariate lookups based on logarithmic derivatives
- cq:fast lookup argument
- Lookup Argument性能优化——Caulk
- 2023年 ZK Hack以及ZK Summit 亮点记
- Research Day 2023:Succinct ZKP最新进展
- Lasso、Jolt 以及 Lookup Singularity——Part 1
- Lasso、Jolt 以及 Lookup Singularity——Part 2