- 使用Airtest来爬取朋友圈的内容。参考链接,参考链接中的手机滑动的功能在我的实际操作过程不可使用,故对其进行改动。
该部分整体代码如下:
__author__ = " "
from airtest.core.api import *
from poco.drivers.android.uiautomation import AndroidUiautomationPoco
poco = AndroidUiautomationPoco(use_airtest_input=True, screenshot_each_action=False)
start_app('com.tencent.mm')
poco(text="通讯录").click()
while True:
if poco(text="要爬取的备注名"):
poco(text="要爬取的备注名").click()
break
else:
screenWidth,screenHeigth = poco.get_screen_size()
swipe((screenWidth*0.5,screenHeigth*0.9),vector=[0,-0.8],duration=2.5)
sleep(1)
poco(text="朋友圈").click()
a=list()
for k in range(100):
result_obj = poco("com.tencent.mm:id/ezu")
for i in range(15):
try:
content1 =result_obj.offspring("com.tencent.mm:id/mk")[i].get_text()
a.append(content1)
content2 =result_obj.offspring("com.tencent.mm:id/dbo")[i].get_text()
a.append(content2)
content3 =result_obj.offspring("com.tencent.mm:id/pi")[i].get_text()
a.append(content3)
except:
pass
screenWidth,screenHeigth = poco.get_screen_size()
swipe((screenWidth*0.5,screenHeigth*0.9),vector=[0,-0.8],duration=2.5)
sleep(3)
print(a)
auto_setup(__file__)
- 因为没有找到直接可以将 Airtest 获取的内容存到文本(有知道的可以交流一下),所以进行复制-粘贴。将获取的内容存到记事本中,并作为生成词云的原始数据。
- 爬取到的内容形式为
['第一条内容','第二条内容',' ' ,'', '。。。']
。首先去掉数据中的表情(/uxxxx和/Uxxxxxxx这种形式),去掉斜杠及回车符,提取不一样的内容,因为每条数据是存放在单引号内,所以进行单引号内内容的提取。python代码如下:
import re
import pandas as pd
def prepro(data):
data_str = str(data)
data_rem_u = re.sub(r"\\(u[0-9a-fA-F]*)","",data_str)
data_rem_U = re.sub(r"\\(U[0-9a-fA-F]*)","",data_rem_u)
data_rem_res = data_rem_U.replace(r"\n","").replace("\\","").replace("...","")
data_clean = re.findall(r"'([\S\s]+?)'",data_rem_res)
return data_clean
def getCategory(data,columns):
data_group = data.groupby(by = columns)
product_list = list(data_group.groups.keys())
return product_list
with open("moment-orginal-data.txt", "r", encoding = 'utf-8') as f:
data = f.readlines()
data_pro = prepro(data)
data_clean = pd.DataFrame(data_pro,columns=['one'])
data_clean_list = getCategory(data_clean,'one')
data_res = pd.DataFrame(data_clean_list,columns=['desc'])
file = open('moment-clean-data.txt','w');
file.write(str(data_clean_list));
file.close()
- 使用现行的分词工具对处理完的文本(moment-clean-data.txt)进行分词和去除停用词的简单操作,并生成词云图。分词可以使用jieba,nlp,pkuseg等。代码如下:
import jieba
import pkuseg
import pandas as pd
from pyhanlp import *
with open("moment-clean-data.txt", "r") as f:
data = f.readlines()
HanLP.Config.ShowTermNature = False
segment = DoubleArrayTrieSegment()
segment.enablePartOfSpeechTagging(True)
cut_pyhanlp = segment.seg(str(data))
def load_from_file(path):
""" 从词典文件加载DoubleArrayTrie :param path: 词典路径 :return: 双数组trie树 """
map = JClass('java.util.TreeMap')()
with open(path,'rb') as src:
for word in src:
word = word.strip()
map[word] = word
return JClass('com.hankcs.hanlp.collection.trie.DoubleArrayTrie')(map)
def remove_stopwords_termlist(termlist, trie):
return [term.word for term in termlist if not trie.containsKey(term.word)]
trie = load_from_file('denywords.txt')
termlist = segment.seg(str(data))
res = remove_stopwords_termlist(termlist,trie)
print(res)
- 利用wordcloud生成词云图,代码如下:
from wordcloud import WordCloud
import jieba
from scipy.misc import imread
from os import path
import matplotlib.pyplot as plt
def draw_wordcloud(cut_text):
color_mask = imread("h.jpg")
cloud = WordCloud(
font_path="STSONG.TTF",
background_color='white',
mask=color_mask,
max_words=5000,
max_font_size=60
)
word_cloud = cloud.generate(cut_text)
word_cloud.to_file("h-2.jpg")
plt.imshow(word_cloud)
plt.axis('off')
plt.show()
draw_wordcloud(str(res))
ps:背景图是读取白底中的黑色部分,如: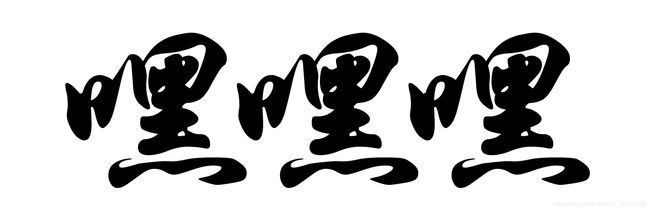
该背景图下的词云图为:
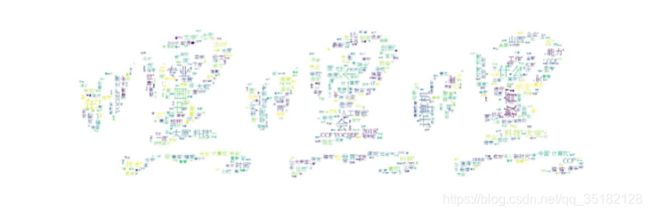