df
DataFrame[a: bigint, b: double, c: string, d: date, e: timestamp]
#### 通过由元组列表组成的RDD创建
rdd = spark.sparkContext.parallelize([
(1, 2., ‘string1’, date(2000, 1, 1), datetime(2000, 1, 1, 12, 0)),
(2, 3., ‘string2’, date(2000, 2, 1), datetime(2000, 1, 2, 12, 0)),
(3, 4., ‘string3’, date(2000, 3, 1), datetime(2000, 1, 3, 12, 0))
])
df = spark.createDataFrame(rdd, schema=[‘a’, ‘b’, ‘c’, ‘d’, ‘e’])
df
DataFrame[a: bigint, b: double, c: string, d: date, e: timestamp]
以上的DataFrame格式创建的都是一样的。
df.printSchema()
root
|-- a: long (nullable = true)
|-- b: double (nullable = true)
|-- c: string (nullable = true)
|-- d: date (nullable = true)
|-- e: timestamp (nullable = true)
### 2.查看
#### DataFrame.show()
使用格式:
df.show()
df.show(1)
±–±–±------±---------±------------------+
| a| b| c| d| e|
±–±–±------±---------±------------------+
| 1|2.0|string1|2000-01-01|2000-01-01 12:00:00|
±–±–±------±---------±------------------+
only showing top 1 row
#### spark.sql.repl.eagerEval.enabled
spark.sql.repl.eagerEval.enabled用于在notebooks(如Jupyter)中快速生成PySpark DataFrame的配置。控制行数可以使用spark.sql.repl.eagerEval.maxNumRows。
spark.conf.set(‘spark.sql.repl.eagerEval.enabled’, True)
df
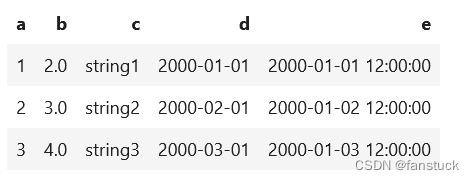
spark.conf.set(‘spark.sql.repl.eagerEval.maxNumRows’,1)
df
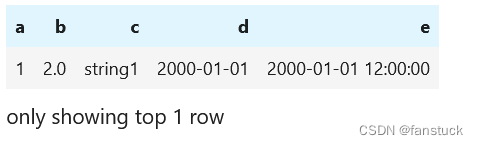
#### 纵向显示
行也可以垂直显示。当行太长而无法水平显示时,纵向显示就很明显。
df.show(1, vertical=True)
-RECORD 0------------------
a | 1
b | 2.0
c | string1
d | 2000-01-01
e | 2000-01-01 12:00:00
only showing top 1 row
#### 查看DataFrame格式和列名
df.columns
[‘a’, ‘b’, ‘c’, ‘d’, ‘e’]
df.printSchema()
root
|-- a: long (nullable = true)
|-- b: double (nullable = true)
|-- c: string (nullable = true)
|-- d: date (nullable = true)
|-- e: timestamp (nullable = true)
#### 查看统