一些配置参数
hbase.lru.blockcache.min.factor
hbase.lru.blockcache.acceptable.factor
hbase.regionserver.global.memstore.upperLimit 默认为0.4
hbase.regionserver.global.memstore.lowerLimit 默认为0.35
cacheFlusher线程
主要用于定期清理 memstore中的数据,具体逻辑如下:
-
-
- MemStoreFlusher#run() {
- fqe = flushQueue.poll(threadWakeFrequency, TimeUnit.MILLISECONDS);
- if (fqe == null || fqe instanceof WakeupFlushThread) {
- if (isAboveLowWaterMark()) {
- flushOneForGlobalPressure();
- }
- continue;
- }
- FlushRegionEntry fre = (FlushRegionEntry)fqe;
- flushRegion(fre);
- }
-
-
- MemStoreFlusher#isAboveLowWaterMark() {
- return server.getRegionServerAccounting().
- getGlobalMemstoreSize() >= globalMemStoreLimitLowMark;
- }
-
- MemStoreFlusher#flushOneForGlobalPressure() {
- HRegion bestFlushableRegion = getBiggestMemstoreRegion(
- regionsBySize, excludedRegions, true);
- HRegion bestAnyRegion = getBiggestMemstoreRegion(
- regionsBySize, excludedRegions, false);
- if (bestFlushableRegion == null) {
- regionToFlush = bestAnyRegion;
- } else {
- regionToFlush = bestFlushableRegion;
- }
- flushRegion(regionToFlush, true);
- }
-
-
- MemStoreFlusher#flushRegion() {
- FlushRegionEntry fqe = this.regionsInQueue.remove(region);
- flushQueue.remove(fqe);
- boolean shouldCompact = HRegion.flushcache();
-
- boolean shouldSplit = HRegion.checkSplit() != null;
- if (shouldSplit) {
- HRegionServer.compactSplitThread.requestSplit(region);
- } else if (shouldCompact) {
- HRegionServer.compactSplitThread.requestCompaction(region, getName());
- }
- }
LruBlockCache
HBase上Regionserver的内存分为两个部分,一部分作为Memstore,主要用来写;另外一部分作为BlockCache,主要用于读。
写请求会先写入Memstore,Regionserver会给每个region提供一个Memstore,当Memstore满64MB以后,会启动 flush刷新到磁盘。当Memstore的总大小超过限制时(heapsize * hbase.regionserver.global.memstore.upperLimit * 0.9),会强行启动flush进程,从最大的Memstore开始flush直到低于限制。
读请求先到Memstore中查数据,查不到就到BlockCache中查,再查不到就会到磁盘上读,并把读的结果放入BlockCache。由于BlockCache采用的是LRU策略,因此BlockCache达到上限(heapsize * hfile.block.cache.size * 0.85)后,会启动淘汰机制,淘汰掉最老的一批数据。
一个Regionserver上有一个BlockCache和N个Memstore,它们的大小之和不能大于等于heapsize * 0.8,否则HBase不能正常启动。
默认配置下,BlockCache为0.2,而Memstore为0.4。在注重读响应时间的应用场景下,可以将 BlockCache设置大些,Memstore设置小些,以加大缓存的命中率。
HBase RegionServer包含三个级别的Block优先级队列:
Single:如果一个Block第一次被访问,则放在这一优先级队列中;
Multi:如果一个Block被多次访问,则从Single队列移到Multi队列中;
InMemory:如果一个Block是inMemory的,则放到这个队列中。
以上将Cache分级思想的好处在于:
首先,通过inMemory类型Cache,可以有选择地将in-memory的column families放到RegionServer内存中,例如Meta元数据信息;
通过区分Single和Multi类型Cache,可以防止由于Scan操作带来的Cache频繁颠簸,将最少使用的Block加入到淘汰算法中。
默认配置下,对于整个BlockCache的内存,又按照以下百分比分配给Single、Multi、InMemory使用:0.25、0.50和0.25。
注意,其中InMemory队列用于保存HBase Meta表元数据信息,因此如果将数据量很大的用户表设置为InMemory的话,可能会导致Meta表缓存失效,进而对整个集群的性能产生影响。
主要逻辑如下:
-
- LruBlockCache#构造函数() {
- map = new ConcurrentHashMap(mapInitialSize,
- mapLoadFactor, mapConcurrencyLevel);
- this.minFactor = minFactor;
- this.acceptableFactor = acceptableFactor;
- this.singleFactor = singleFactor;
- this.multiFactor = multiFactor;
- this.memoryFactor = memoryFactor;
- this.stats = new CacheStats();
- this.count = new AtomicLong(0);
- this.elements = new AtomicLong(0);
- this.overhead = calculateOverhead(maxSize, blockSize, mapConcurrencyLevel);
- this.size = new AtomicLong(this.overhead);
- if(evictionThread) {
- this.evictionThread = new EvictionThread(this);
- this.evictionThread.start();
- } else {
- this.evictionThread = null;
- }
- this.scheduleThreadPool.scheduleAtFixedRate(new StatisticsThread(this),
- statThreadPeriod, statThreadPeriod, TimeUnit.SECONDS);
- }
-
-
-
-
-
- LruBlockCache#cacheBlock() {
- CachedBlock cb = map.get(cacheKey);
- if(cb != null) {
- throw new RuntimeException("Cached an already cached block");
- }
- cb = new CachedBlock(cacheKey, buf, count.incrementAndGet(), inMemory);
- long newSize = updateSizeMetrics(cb, false);
- map.put(cacheKey, cb);
- elements.incrementAndGet();
- if(newSize > acceptableSize() && !evictionInProgress) {
- runEviction();
- }
- }
-
- class CachedBlock() {
- static enum BlockPriority {
-
- SINGLE,
-
- MULTI,
-
- MEMORY
- };
-
-
- public void access(long accessTime) {
- this.accessTime = accessTime;
- if(this.priority == BlockPriority.SINGLE) {
- this.priority = BlockPriority.MULTI;
- }
- }
- }
-
-
-
- LruBlockCache#run() {
- this.wait();
- LruBlockCache cache = this.cache.get();
- if(cache == null) {
- break;
- }
- cache.evict();
- }
-
-
- LruBlockCache#evict() {
-
-
- long currentSize = this.size.get();
- long bytesToFree = currentSize - minSize();
- if(bytesToFree <= 0) {
- return;
- }
-
-
-
-
- BlockBucket bucketSingle = new BlockBucket(bytesToFree, blockSize,
- singleSize());
- BlockBucket bucketMulti = new BlockBucket(bytesToFree, blockSize,
- multiSize());
- BlockBucket bucketMemory = new BlockBucket(bytesToFree, blockSize,
- memorySize());
-
-
- for(CachedBlock cachedBlock : map.values()) {
- switch(cachedBlock.getPriority()) {
- case SINGLE: {
- bucketSingle.add(cachedBlock);
- break;
- }
- case MULTI: {
- bucketMulti.add(cachedBlock);
- break;
- }
- case MEMORY: {
- bucketMemory.add(cachedBlock);
- break;
- }
- }
- }
-
-
-
- PriorityQueue bucketQueue =
- new PriorityQueue(3);
- bucketQueue.add(bucketSingle);
- bucketQueue.add(bucketMulti);
- bucketQueue.add(bucketMemory);
- int remainingBuckets = 3;
- long bytesFreed = 0;
- BlockBucket bucket;
-
-
-
- while((bucket = bucketQueue.poll()) != null) {
- long overflow = bucket.overflow();
- if(overflow > 0) {
- long bucketBytesToFree = Math.min(overflow,
- (bytesToFree - bytesFreed) / remainingBuckets);
-
- bytesFreed += bucket.free(bucketBytesToFree);
- }
- remainingBuckets--;
- }
- }
-
-
- LruBlockCache#free() {
- CachedBlock cb;
- long freedBytes = 0;
- while ((cb = queue.pollLast()) != null) {
- freedBytes += evictBlock(cb);
- if (freedBytes >= toFree) {
- return freedBytes;
- }
- }
- }
-
-
- LruBlockCache#evictBlock() {
- map.remove(block.getCacheKey());
- updateSizeMetrics(block, true);
- elements.decrementAndGet();
- stats.evicted();
- return block.heapSize();
- }
类图
block cache相关类图如下
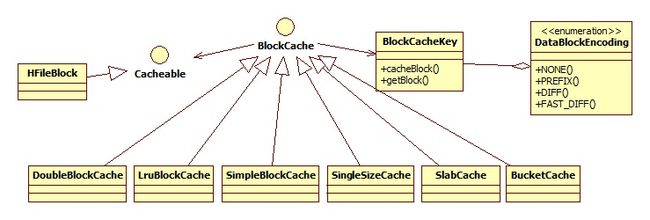
参考
HBase上关于CMS、GC碎片、大缓存的一种解决方案:Bucket Cache
HBase的Block Cache实现机制分析
memstore的flush流程分析
hbase定时memflush PeriodicMemstoreFlusher
MemStoreChunkPool&MSLAB提升HBASE GC性能