文章目录
- 概述
- Activation Functions激活函数
- sigoid的优缺点
- tanh(x)特点
- ReLU优缺点
- Leaky ReLU优缺点
- Exponential Linear Units (ELU)
- Maxout
- TLDR(Too long Don't Read)
- Data Preprocessing数据处理
- Weight Initialization权重初始化
- Batch Normalization批量归一化
- Babysitting the Learning Process调参过程实践
- Hyperparameter Optimization超参数优化
概述
在线Latex公式
本节包含两大块内容,第一块是前面三个,第二块是后面三个:
- Activation Functions激活函数
- Data Preprocessing数据处理
- Weight Initialization权重初始化
- Batch Normalization批量归一化
- Babysitting the Learning Process调参过程实践
- Hyperparameter Optimization超参数优化
这一节的内容在ng的深度学习中基本已经cover过了,所以不打算写得太详细,上点图大概总结一下。
Activation Functions激活函数
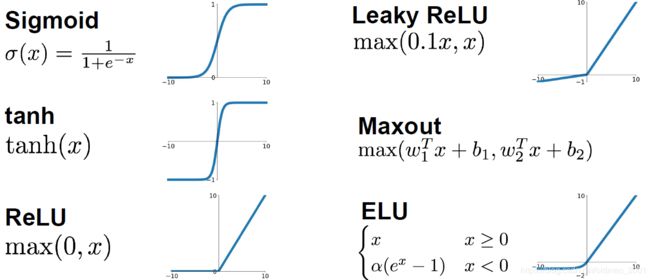
这里注意 t a n h ( x ) tanh(x) tanh(x)是取值区间是(-1,1),这里ELU是之前没有讲过的,其他都在ng和李宏毅的课里面有讲。
sigoid的优缺点
优点:
- Squashes numbers to range [0,1]
- Historically popular since they have nice interpretation as a saturating “firing rate” of a neuron
缺点:
- Saturated neurons “kill” the gradients(饱和:激活函数导数接近0)
- Sigmoid outputs are not zero-centered
- exp() is a bit compute expensive(对于后面矩阵什么计算虽然不算什么,但是对于其他激活函数而言sigmoid的求导计算量比较大)
tanh(x)特点
- Squashes numbers to range [-1,1]
- zero centered (nice)
- still kills gradients when saturated
ReLU优缺点
优点:
- Does not saturate (in +region)
- Very computationally efficient
- Converges much faster than sigmoid/tanh in practice (e.g. 6x)
缺点:
- Not zero-centered output
- An annoyance:小于0的区域都会出现梯度消失的问题
Leaky ReLU优缺点
- Does not saturate
- Computationally efficient
- Converges much faster than sigmoid/tanh in practice! (e.g. 6x)
- will not “die”.
Exponential Linear Units (ELU)
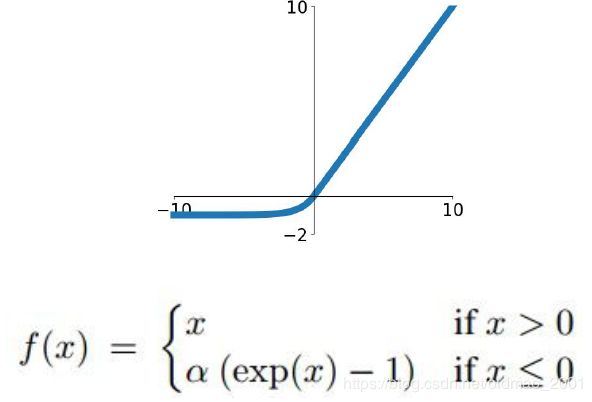
优点:
- All benefits of ReLU
- Closer to zero mean outputs
- Negative saturation regime compared with Leaky ReLU adds some robustness to noise
缺点:
- Computation requires exp()
Maxout
这个在李宏毅的课里面讲过,是抓爆的一般形式。
优点:
- Does not have the basic form of dot product ->nonlinearity
- Generalizes ReLU and Leaky ReLU
- Linear Regime! Does not saturate! Does not die!
缺点:
Problem: doubles the number of parameters/neuron
TLDR(Too long Don’t Read)
- Use ReLU. Be careful with your learning rates
- Try out Leaky ReLU / Maxout / ELU
- Try out tanh but don’t expect much
- Don’t use sigmoid
Data Preprocessing数据处理
Assume X [NxD] is data matrix, each example in a row,常用操作有:
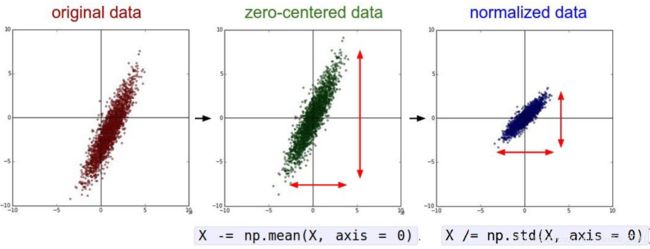
还有PCA和Whitening,白化是将不同特征的相关性去掉相,并且将新特征的数据的的方差化为相同。
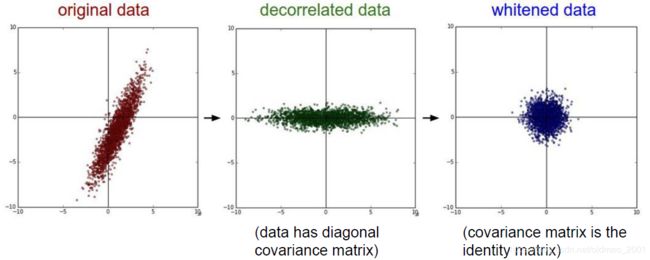
TLDR
对于图像处理,只做中心化,一般不做归一化也不做PCA和白化,原因是每个像素点的取值范围基本都是0-255,强行归一化会丢失图片特征。
e.g. consider CIFAR-10 example with [32,32,3] images
- Subtract the mean image (e.g. AlexNet)
(mean image = [32,32,3] array)
- Subtract per-channel mean (e.g. VGGNet)
(mean along each channel = 3 numbers)
- Subtract per-channel mean and Divide by per-channel std (e.g. ResNet)
(mean along each channel = 3 numbers)
学生问,归一化对于sigmoid激活函数是否有帮助
答:有,但仅仅对输入层进行归一化则仅仅对第一层有帮助,对后面没有帮助。
助教补充:剪切图片、图片灰度、二值化、缩放、数据增强都属于图像预处理,这个看个人需要。而减均值除方差是属于标准化输入。
Weight Initialization权重初始化
用 W = 0 W=0 W=0初始化是否可行?
所有权重为0,那么所有神经元都会做同样的操作,相同的输出,得到相同的梯度,相同的更新,这样不同的神经元就不能学习到不同的特征了。因此做的第一个事情就是:
- First idea: Small random numbers
(gaussian with zero mean and 1e-2 standard deviation)
常见设置,可以解决Symmetry breaking problem,ng的课里面解释了为什么这里是0.01或者说是一个比较小的数字,因为数字大意味w变大,使用tanh或者sigmoid函数计算输出的时候就会停在tanh/sigmoid函数平坦的地方,这些地方的梯度很小,做GD很慢。
w = 0.01 * np.random.randn(Din, Dout)
Works ~okay for small networks, but problems with deeper networks.
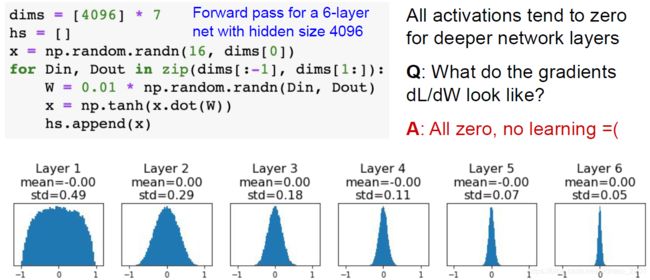
由于采用的tanh函数作为激活函数,tanh是零点对称,所以上面的每个层的输出均值都为0,但是标准差会变小,因为每层都乘以一个很小的w,导致std缩水。就是往前传递的过程中,输出变小,导致local gradient趋向于0,在计算梯度的时候也变成0.
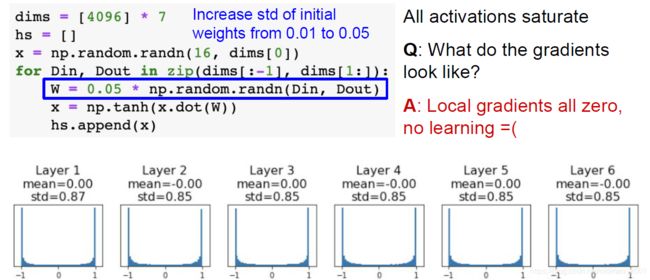
这里增大了weight,导致经过tanh后输出为-1或者1(回想tanh函数的图形),Local gradients也都是0(平滑区域斜率是0)
Xavier初始化
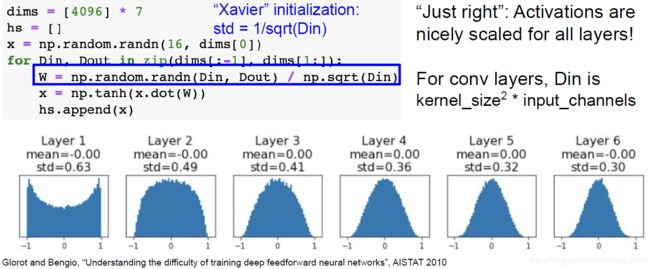
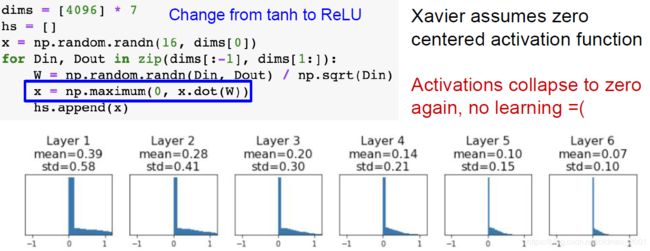
使用ReLU的话小于0的部分就会被kill,所以看到只有一半。
Batch Normalization批量归一化
我们希望每层的输入数据都是zero-mean的,原因上半节课有讲:https://blog.csdn.net/mooneve/article/details/81943904
因此就是要做归一化,其原始形式为:
x ^ ( k ) = x ( k ) − E [ x ( k ) ] V a r [ x ( k ) ] \widehat x^{(k)}=\frac{x^{(k)}-E[x^{(k)}]}{\sqrt{Var[x^{(k)}]}} x (k)=Var[x(k)] x(k)−E[x(k)]
对于输入 x x x是 N × D N×D N×D维的矩阵的时候计算就变成下面这样:
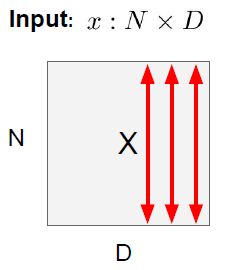
μ j = 1 N ∑ i = 1 N x i , j μ_j=\frac{1}{N}\sum_{i=1}^Nx_{i,j} μj=N1i=1∑Nxi,j Per-channel mean, shape is D
σ j 2 = 1 N ∑ i = 1 N ( x i , j − μ j ) 2 {\sigma }_j^2=\frac{1}{N}\sum_{i=1}^N(x_{i,j}-μ_j)^2 σj2=N1i=1∑N(xi,j−μj)2Per-channel var, shape is D
x ^ i , j = x i , j − μ j σ j 2 + ε \widehat x_{i,j}=\frac{x_{i,j}-μ_j}{\sqrt{{\sigma }_j^2+\varepsilon}} x i,j=σj2+ε xi,j−μj Normalized x, Shape is N x D
BN操作常常在FC或convolution操作后,在nonlinearity之前,原因是我们这这些地方要乘W,在这里做归一化可以减少不好的尺度效应:
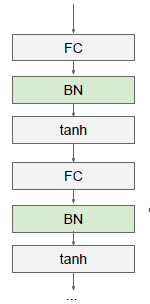
做完归一化后,通常我们会想要控制神经元饱和程度,会对归一化结果进行拉伸scale和平移shift,对应的参数是 γ \gamma γ和 β \beta β,这两个参数也是可以学习出来的(这里有些没理解,看ng讲的时候就不太明白,这里也是)
y i , j = γ j x ^ i , j + β j y_{i,j}={\gamma }_j\widehat x_{i,j}+\beta_j yi,j=γjx i,j+βj Output, Shape is N x D
BN小结
- Makes deep networks much easier to train!
- Improves gradient flow
- Allows higher learning rates, faster convergence(鲁棒性加强)
- Networks become more robust to initialization
- Acts as regularization during training
- Zero overhead at test-time: can be fused with conv!
- Behaves differently during training and testing: this is a very common source of bugs!
- BN讲解好文推荐
Babysitting the Learning Process调参过程实践
在ng的课有提过,两种模式Panda vs. Caviar相当于哺乳动物和卵生动物的比较。
这里也给出了一些实战建议:
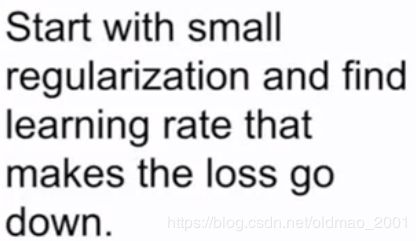
注意:数据集很小的时候,模型应该能够overfitting数据,如果不是则有问题。
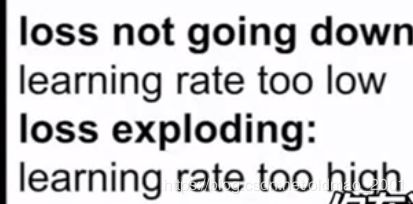
常见的学习率范围:
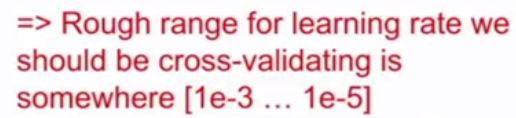
Hyperparameter Optimization超参数优化
交叉验证策略
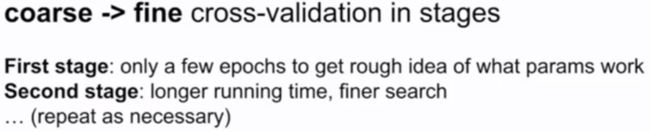
这里还有个tip

参数调整范围
这里就没有ng讲得细,贴个潦草的笔记。
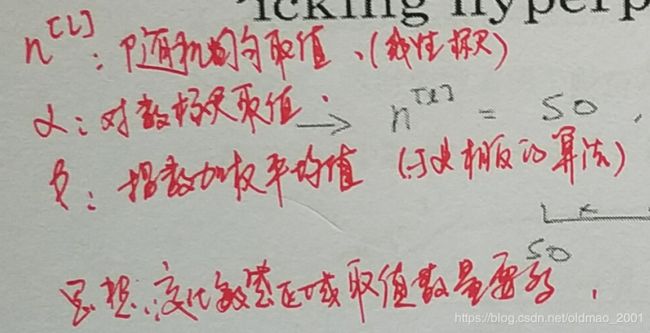