爬取链家二手房相关信息并进行数据分析 {[https://sh.lianjia.com/ershoufang/pg](https://sh.lianjia.com/ershoufang/pg)}
需求来源于生活
大数据时代来临,数据就是核心,数据就是生产力,越来越多的企业开始注重收集用户数据,而爬虫技术是收集数据的一种重要手段
python版本:3.6.5 ,系统环境是windows,
工具包:request获取页面数据
实现页面数据分析的两个库:from parsel import Selector;from bs4 import BeautifulSoup
法一:通过Selector 调用xpath方法,{XPath (它是一种在 XML 文档中查找信息的语言。XPath 可用来在 XML 文档中对元素和属性进行遍历)};
import requests
from parsel import Selector
# 进行网络请求的浏览器头部
headers={
'User-Agent':'Mozilla/5.0 (Windows NT 10.0; WOW64) AppleWebKit/537.36 (KHTML, like Gecko) Chrome/47.0.2526.106 BIDUBrowser/8.7 Safari/537.36'
}
url = 'https://m.lianjia.com/sh/ershoufang/pg'
wr=requests.get(url,headers=headers,stream=True)
sel=Selector(wr.text)
tag = sel.xpath('//div[@class="price_total"]//text()').extract()
print(tag)
法二:lxml 实现页面数据的分析,提取我们想要的数据
import requests
from bs4 import BeautifulSoup
url = 'https://m.lianjia.com/sh/ershoufang/pg'
res = requests.get(url, timeout=60).content
soup = BeautifulSoup(res, "lxml")
tag = soup.find_all("span", attrs={"class": "price_total"})
print(tag)
上海链家二手房链接:https://m.lianjia.com/sh/ershoufang/pg 在抓取的过程中,在翻页的过程中出现一点点意外(在翻页到60页左右数据就出现重复抓取的),后上网尝试发现还有一个链接:https://sh.lianjia.com/ershoufang/pg ,而且该链接有房子id的信息,故完整的代码用该链接进行获取数据。
链家二手房网址 https://sh.lianjia.com/ershoufang/pg
import requests
from parsel import Selector
import pandas as pd
import time
headers={
'User-Agent':'Mozilla/5.0 (Windows NT 10.0; WOW64) AppleWebKit/537.36 (KHTML, like Gecko) Chrome/47.0.2526.106 BIDUBrowser/8.7 Safari/537.36'
}
# pages是不同页码的网址列表
pages=['https://sh.lianjia.com/ershoufang/pg{}/'.format(x) for x in range(1,1000)]
lj_shanghai= pd.DataFrame(columns=['hou_code','title','infotitle','alt',
'positionIcon_region','positionIcon_new',
'positionInfo_new','position_xiaoqu','starIcon','price_total_new','unitPrice'])
count=0
def l_par_html(url):
wr=requests.get(url,headers=headers,stream=True)
sel=Selector(wr.text)
# hou_code用来获取房源的编号
hou_code=sel.xpath('//div[@class="title"]/a/@data-housecode').extract()
#获取标题
title=sel.xpath('//div[@class="title"]//text()').extract()
infotitle=sel.xpath('//div[@class="title"]/a/text()').extract()
# =============================================================================
# #图片
# # src=sel.xpath('//img[@class="lj-lazy"]//@src').extract()
# # print('src:%s'%src)
# =============================================================================
#图片地址
alt=sel.xpath('//img[@class="lj-lazy"]//@alt').extract()
positionIcon_region=sel.xpath('//div[@class="houseInfo"]/a/text()').extract()
#获取房屋信息
positionIcon = sel.xpath('//div[@class="houseInfo"]//text()').extract()
positionIcon_new=([x for x in positionIcon if x not in positionIcon_region ])
positionInfo = sel.xpath('//div[@class="positionInfo"]//text()').extract()
position_xiaoqu = sel.xpath('//div[@class="positionInfo"]/a/text()').extract()
positionInfo_new = ([x for x in positionInfo if x not in position_xiaoqu])
starIcon =sel.xpath('//div[@class="followInfo"]//text()').extract()
price_total = sel.xpath('//div[@class="totalPrice"]//text()').extract()
price_total_new =([x for x in price_total if x != '万' ])
unitPrice =sel.xpath('//div[@class="unitPrice"]//text()').extract()
wr=requests.get(url,headers=headers,stream=True)
sel=Selector(wr.text)
tag = sel.xpath('//div[@class="tag"]//text()').extract()
# print("tag:%s"%tag)
pages_info=pd.DataFrame(list(zip(hou_code,title,infotitle,alt,
positionIcon_region,positionIcon_new,
positionInfo_new,position_xiaoqu,starIcon,price_total_new,unitPrice)),
columns=['hou_code','title','infotitle','alt',
'positionIcon_region','positionIcon_new',
'positionInfo_new','position_xiaoqu','starIcon','price_total_new','unitPrice'])
# print(pages_info)
#由于抓取下来的信息是存储在列表中的,出现了一对多的情况,故将tag,title,infotitle单独取出分析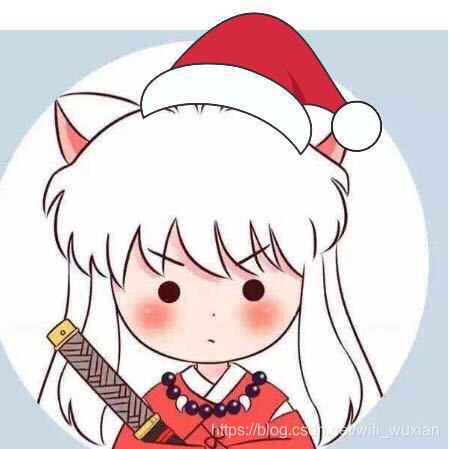
return pages_info,tag,title,infotitle
for page in pages:
a=l_par_html(page)[0]
b=l_par_html(page)[1:]
print('advantage:{}'.format(b))
count=count+1
print ('the '+str(count)+' page is sucessful')
#每隔20s翻页一次
time.sleep(20)
lj_shanghai=pd.concat([lj_shanghai,a],ignore_index=True)
print(lj_shanghai)
#将数据存储到excel表格中
lj_shanghai.to_excel(r'\\lianjia_ershou_shanghai.xlsx')
Q:使用xpath的text()函数定位界面元素,文本中含有空格如何解决呢?
抓取前1000页房价的相关数据,抓取3W+数据,但是对hou_code进行去重后,发现只有近3000条数据没有重复,上网搜了一下原因,没有找到原因,反而找到有相同问题的帖子(要么代码错了要么链家错了,反正wifi没错,hhhh~),此处有❓,等你来解 ~
发现原因:链家默认展示前100页房源,也就是说,当你从101页开始循环的时候,自动跳转到第一页重新开始循环,所以一次你只能抓取100页的房源信息
将数据导入mysql存储,并进行简单的数据清洗,得到:
插一句题外话:
关于python和mysql之间数据导入导出很方便,附上代码:
import pandas as pd
import pymysql
# 数据库对应地址及用户名密码,指定格式,解决输出中文乱码问题
conn = pymysql.connect(host=None, port=3306,
user='root', passwd='******', db='test',
charset='utf8')
# cursor获得python执行Mysql命令的方法,也就是操作游标
#使用cursor()方法创建一个游标对象
cur = conn.cursor()
v_sql = "select * from a"
#获取数据并以dataframe读取
table = pd.read_sql(v_sql,conn)
# =============================================================================
# 另一种方式获取数据库表
#使用execute()方法执行SQL语句
cur.execute(v_sql)
#使用fetall()获取全部数据
data = cur.fetchall()
# =============================================================================
#关闭游标和数据库的连接
cur.close()
conn.close()
table
data
这里也可以直接用python的pandas模块进行做处理,a.str.split(r’/’,expand = True)
需要注意的是,这里的面积、单价、总价等字段都是** str** 格式,需转成 ** flaot** 类型,再进行a.describe()统计分析,这里不一一展示了,只展示部分结果,注意这里的数据有3054条,比distinct hou_code数据要多,因为这里是根据一整条记录进行a.duplicated()的。(不同的原因是由于关注人数不同,可能是重复循环翻页的过程中,用户的关注增加导致的),null
根据标题信息进行结巴分词
import pandas as pd
file = open(r'\title.xlsx','rb')
data = pd.read_excel(file)
data.columns
title = data['标题']
import numpy as np
import os
lis = np.array(title)
lis = lis.tolist()
lis[:5]
str1 = "".join(lis)
item_main = str1.strip().replace('span','').replace('class','').replace('emoji','').replace(' ','')
def save_fig(fig_id, tight_layout=True):
path = os.path.join(r"D:\\python\爬虫", fig_id + ".png")
print("Saving figure", fig_id)
if tight_layout:
plt.tight_layout()
plt.savefig(path, format='png', dpi=300)
/*
这里没有使用结巴分词,因为这里的部分词语分词效果不是很好,由于时间不是很充裕并没有在本地分词库中添加房子相关的词语
import jieba
wordlist = jieba.cut(item_main,cut_all=True)
word_space_split =" ".join(wordlist)
type(word_space_split)
*/
import matplotlib.pyplot as plt
from wordcloud import WordCloud,ImageColorGenerator
import PIL.Image as Image
coloring = np.array(Image.open(r'\Desktop\图片.jpg'))
my_wordcloud = WordCloud(background_color='white',max_words=200,mask=coloring,max_font_size=60,random_state=42,
scale=2,font_path=r'C:\Windows\Fonts\simkai.ttf').generate(item_main)
image_colors = ImageColorGenerator(coloring)
plt.imshow(my_wordcloud.recolor(color_func=image_colors))
plt.imshow(my_wordcloud)
plt.axis('off')
save_fig('my_wordcloud_fangjia')
plt.show()
tips:本来打算用python调用百度接口的,申请了百度地图开发密匙,但是运行出来的结果显示接口禁用(APP 服务被禁用(240)),尝试没成功就暂时放弃了,https://jingyan.baidu.com/article/e73e26c0b5b75124adb6a786.html ,附上代码,有兴趣的小伙伴可以尝试一下,回头请教@-@~
echarts没尝试
from urllib.request import urlopen, quote
from json import loads as loadjson
#将申请的AK复制粘贴到字符串里
ak = '*********'
def _url(service,**kv):
qstr = ''
url = 'http://api.map.baidu.com/' \
+ service + '/v2/' \
+ '?output=json' \
+ '&ak=' + ak
for k, v in kv.items():
url += '&' + k + '=' + quote(v)
return url
def baidumapapi(service,**kv):
data = urlopen(_url(service,**kv)).read().decode('utf-8')
return loadjson(data)
geo = baidumapapi('geocoder',address='gd')
if geo['status'] == 0:
try:
print(geo['result']['location']['lng'])
except (Exception,):
print(geo)
else:
print('{}({})'.format(geo['msg'] if 'msg' in geo.keys() else geo['message'],
geo['status']))
下图是用excel的三维地图做的(在 Excel 2016 for Windows 中开始使用三维地图),该地图对地理位置大部分街道可以识别(本次地图展示的可信度99%),只有一个地址没能识别,不知道为什么这个地址一直识别不出来,按照标准格式修改还是没法识别,——,求解~
但是具体地址的识别率很低,要是想精确到小区地址,这个工具不是一个好的idea
附上部分截图,其中柱状图表示该地区总价的平均值,热力图表示该地区单价的平均值
对于地图可视化,最好通过经纬度进行展示,这是最好的方式,不知道,网上有没有通过输入地址之后可以输出经纬度的?回头研究一下~
Ps:
不同小区在地图上展示的链接地址
希望上述内容可以帮助到大家~
1、本文只是进行一些简单地爬虫,过程中遇到一些问题,比如获取tag,title,infotitle 存在列表中的,由于hou_code与这些字符串是一对多的关系,所以在进行dataframe(类似关系型数据库)之后,这些信息就会发生错位,这个问题没有解决;使用循环解决???尝试了it doesn’t work
2、每个房屋的详细信息可深一步研究
3、使用xpath的text()函数定位界面元素,文本中含有空格如何解决呢?
4、地址如何批量获取经纬度,在地图上完美展示?(已解决,详情 https://editor.csdn.net/md/?articleId=94437168)
5、可以进行模型分析,对于类别变量可进行独热编码,计算WOE进行分箱,回归预测房价走势