样本不均衡的影响
from sklearn.datasets import make_blobs
import numpy as np, matplotlib.pyplot as mp
from sklearn.linear_model import LogisticRegression
X, Y = make_blobs(centers=2, cluster_std=2, random_state=0)
X0 = np.concatenate([X if i < 1 else X[Y == 0] for i in range(5)])
Y0 = np.concatenate((Y, np.zeros(200, np.int32)))
X1 = np.concatenate([X if i < 1 else X[Y == 1] for i in range(5)])
Y1 = np.concatenate((Y, np.ones(200, np.int32)))
for i, (Xi, Yi) in enumerate([(X, Y), (X0, Y0), (X1, Y1)]):
model = LogisticRegression()
model.fit(Xi, Yi)
k = model.coef_[0]
b = model.intercept_[0]
mp.subplot(1, 3, i + 1)
x1, x2 = Xi[:, 0], Xi[:, 1]
mp.scatter(x1, x2, c=Yi, alpha=.2)
x = np.array([x1.min(), x1.max()])
y = (-b - k[0] * x) / k[1]
mp.plot(x, y)
mp.show()
如图示:样本不均衡时,决策边界不在中间
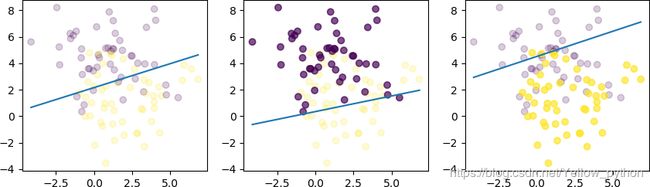
imblearn实施采样
from sklearn.datasets import make_blobs
import numpy as np, matplotlib.pyplot as mp
from sklearn.linear_model import LogisticRegression
from imblearn import over_sampling, under_sampling
X, Y = make_blobs(centers=2, cluster_std=2, random_state=0)
X0 = np.concatenate([X if i < 1 else X[Y == 0] for i in range(5)])
Y0 = np.concatenate((Y, np.zeros(200, np.int32)))
X1 = np.concatenate([X if i < 1 else X[Y == 1] for i in range(5)])
Y1 = np.concatenate((Y, np.ones(200, np.int32)))
X0u, Y0u = under_sampling.RandomUnderSampler().fit_sample(X0, Y0)
X1o, Y1o = over_sampling.RandomOverSampler().fit_sample(X1, Y1)
samples = [('zero:one = 1:1', X, Y), ('zero:one = 5:1', X0, Y0), ('zero:one = 1:5', X1, Y1),
('zero:one = 1:1', X, Y), ('Under Sampling', X0u, Y0u), ('Over Sampling', X1o, Y1o)]
for i, (title, Xi, Yi) in enumerate(samples):
model = LogisticRegression()
model.fit(Xi, Yi)
k = model.coef_[0]
b = model.intercept_[0]
mp.subplot(2, 3, i + 1)
mp.title(title)
mp.xticks(())
mp.yticks(())
x1, x2 = Xi[:, 0], Xi[:, 1]
mp.scatter(x1, x2, c=Yi, alpha=.2)
x = np.array([x1.min(), x1.max()])
y = (-b - k[0] * x) / k[1]
mp.plot(x, y)
mp.show()
实施采样策略后,决策边界偏离度减少
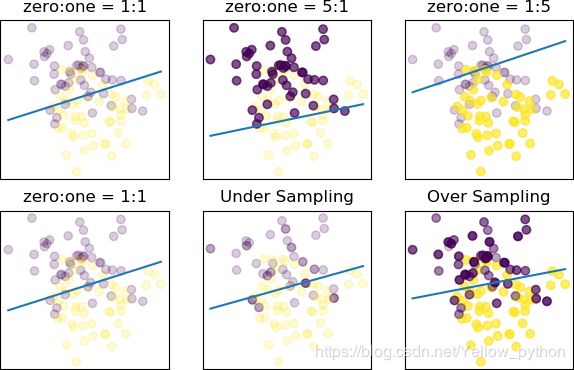