import numpy as np
import pandas as pd
import matplotlib.pyplot as plt
%matplotlib inline
使用pandas的内建函数DataReader从雅虎财经网站读取股价数据
import tushare as ts
pingan = ts.get_k_data('601318',start='2011-01-01')
pingan.head()
|
date |
open |
close |
high |
low |
volume |
code |
0 |
2011-01-04 |
24.984 |
25.011 |
25.314 |
24.830 |
245626.0 |
601318 |
1 |
2011-01-05 |
24.870 |
24.110 |
24.962 |
24.083 |
427554.0 |
601318 |
2 |
2011-01-06 |
24.145 |
23.112 |
24.145 |
22.611 |
947078.0 |
601318 |
3 |
2011-01-07 |
23.279 |
23.297 |
23.842 |
22.765 |
659013.0 |
601318 |
4 |
2011-01-10 |
23.248 |
22.866 |
23.688 |
22.809 |
282919.0 |
601318 |
pingan.tail()
|
date |
open |
close |
high |
low |
volume |
code |
1999 |
2019-04-03 |
78.48 |
79.86 |
80.15 |
78.37 |
760219.0 |
601318 |
2000 |
2019-04-04 |
80.08 |
80.20 |
80.96 |
79.25 |
795626.0 |
601318 |
2001 |
2019-04-08 |
80.70 |
80.59 |
82.25 |
80.02 |
916122.0 |
601318 |
2002 |
2019-04-09 |
80.40 |
81.10 |
82.00 |
80.37 |
601737.0 |
601318 |
2003 |
2019-04-10 |
80.80 |
82.03 |
82.11 |
79.66 |
783620.0 |
601318 |
pingan['date'] = pd.to_datetime(pingan.date)
pingan.set_index('date',inplace = True)
pingan.index
DatetimeIndex(['2011-01-04', '2011-01-05', '2011-01-06', '2011-01-07',
'2011-01-10', '2011-01-11', '2011-01-12', '2011-01-13',
'2011-01-14', '2011-01-17',
...
'2019-03-27', '2019-03-28', '2019-03-29', '2019-04-01',
'2019-04-02', '2019-04-03', '2019-04-04', '2019-04-08',
'2019-04-09', '2019-04-10'],
dtype='datetime64[ns]', name='date', length=2004, freq=None)
pingan['close'].plot(figsize=(12,8))
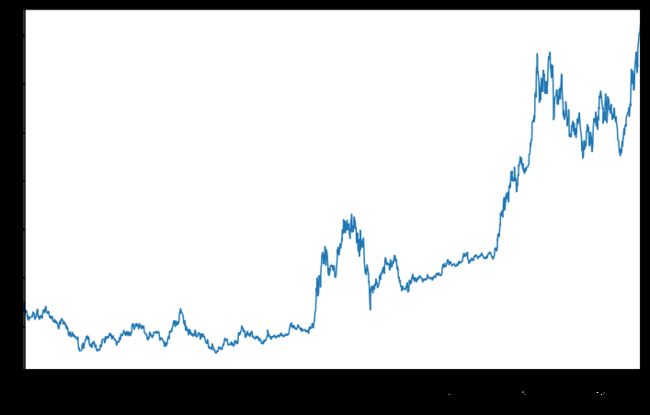
g_yue = pingan['close'].resample('M').ohlc()
g_yue
|
open |
high |
low |
close |
date |
|
|
|
|
2011-01-31 |
25.011 |
25.011 |
21.249 |
21.842 |
2011-02-28 |
21.952 |
22.980 |
21.477 |
21.868 |
2011-03-31 |
22.084 |
23.512 |
21.433 |
21.736 |
2011-04-30 |
22.514 |
24.123 |
22.514 |
22.954 |
2011-05-31 |
23.103 |
23.103 |
20.972 |
21.407 |
2011-06-30 |
21.310 |
21.310 |
19.495 |
21.213 |
2011-07-31 |
21.156 |
21.609 |
19.534 |
19.724 |
2011-08-31 |
19.906 |
19.906 |
17.867 |
18.053 |
2011-09-30 |
18.231 |
18.294 |
14.935 |
14.935 |
2011-10-31 |
15.082 |
17.226 |
15.082 |
17.208 |
2011-11-30 |
17.449 |
18.036 |
15.687 |
15.687 |
2011-12-31 |
16.541 |
16.901 |
14.935 |
15.322 |
2012-01-31 |
15.082 |
17.395 |
15.060 |
17.057 |
2012-02-29 |
16.643 |
18.556 |
16.643 |
18.160 |
2012-03-31 |
18.040 |
18.352 |
16.123 |
16.274 |
2012-04-30 |
16.799 |
18.405 |
16.750 |
18.111 |
2012-05-31 |
18.761 |
19.166 |
18.085 |
18.636 |
2012-06-30 |
18.761 |
20.518 |
18.094 |
20.349 |
2012-07-31 |
20.509 |
20.572 |
19.493 |
19.793 |
2012-08-31 |
20.227 |
20.227 |
17.193 |
17.381 |
2012-09-30 |
17.829 |
18.833 |
17.560 |
18.833 |
2012-10-31 |
18.613 |
19.018 |
17.091 |
17.423 |
2012-11-30 |
17.661 |
17.661 |
15.865 |
16.382 |
2012-12-31 |
16.449 |
20.338 |
16.449 |
20.338 |
2013-01-31 |
21.155 |
21.653 |
19.952 |
21.653 |
2013-02-28 |
22.799 |
23.701 |
20.194 |
21.137 |
2013-03-31 |
20.634 |
20.634 |
18.245 |
18.757 |
2013-04-30 |
18.407 |
19.265 |
17.877 |
17.877 |
2013-05-31 |
17.976 |
18.717 |
17.374 |
17.920 |
2013-06-30 |
17.929 |
17.929 |
15.558 |
15.726 |
... |
... |
... |
... |
... |
2016-11-30 |
33.393 |
35.031 |
32.964 |
34.602 |
2016-12-31 |
34.688 |
35.269 |
33.012 |
33.745 |
2017-01-31 |
33.983 |
34.774 |
33.536 |
34.774 |
2017-02-28 |
33.926 |
35.069 |
33.926 |
34.507 |
2017-03-31 |
34.440 |
35.250 |
33.907 |
35.250 |
2017-04-30 |
35.336 |
36.155 |
33.821 |
36.155 |
2017-05-31 |
36.107 |
43.327 |
35.488 |
42.927 |
2017-06-30 |
43.784 |
47.584 |
42.546 |
47.251 |
2017-07-31 |
46.613 |
52.003 |
45.641 |
50.087 |
2017-08-31 |
52.812 |
54.940 |
47.738 |
53.948 |
2017-09-30 |
54.083 |
54.685 |
51.567 |
52.616 |
2017-10-31 |
53.199 |
62.525 |
53.199 |
62.525 |
2017-11-30 |
62.292 |
76.203 |
62.185 |
67.926 |
2017-12-31 |
66.158 |
72.774 |
66.158 |
67.985 |
2018-01-31 |
70.520 |
76.514 |
68.014 |
72.939 |
2018-02-28 |
73.328 |
73.843 |
62.593 |
65.828 |
2018-03-31 |
66.965 |
71.977 |
63.448 |
63.448 |
2018-04-30 |
62.816 |
66.304 |
59.154 |
59.154 |
2018-05-31 |
59.591 |
62.272 |
58.921 |
60.358 |
2018-06-30 |
59.882 |
63.990 |
56.267 |
58.000 |
2018-07-31 |
54.673 |
61.545 |
54.673 |
60.990 |
2018-08-31 |
59.109 |
62.842 |
56.020 |
62.357 |
2018-09-30 |
62.109 |
68.500 |
60.600 |
68.500 |
2018-10-31 |
64.780 |
67.930 |
61.850 |
63.630 |
2018-11-30 |
64.300 |
67.350 |
62.640 |
63.490 |
2018-12-31 |
64.990 |
64.990 |
56.100 |
56.100 |
2019-01-31 |
55.180 |
62.980 |
55.180 |
62.980 |
2019-02-28 |
63.210 |
73.000 |
63.210 |
70.010 |
2019-03-31 |
72.390 |
77.100 |
68.700 |
77.100 |
2019-04-30 |
78.600 |
82.030 |
78.600 |
82.030 |
100 rows × 4 columns
pingan['close'].loc['2017'].plot(figsize=(12,8))
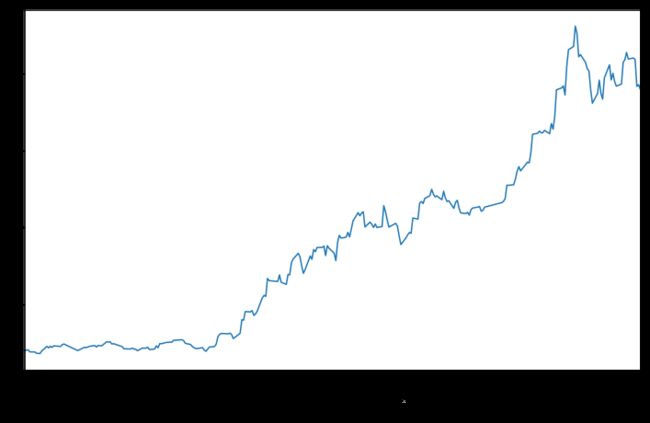
shouyi = (pingan['close'][-1] - pingan['close'][0])/pingan['close'][0]
shouyi
2.2797569069609374
y_num = pingan.index[-1].year - pingan.index[0].year
y_num
8
shouyi**(1/y_num)
1.1085009445718548
pingan['close'].to_period('A')
date
2011 25.011
2011 24.110
2011 23.112
2011 23.297
2011 22.866
2011 22.905
2011 23.288
2011 23.283
2011 22.844
2011 21.908
2011 21.974
2011 22.273
2011 21.438
2011 21.714
2011 21.249
2011 21.315
2011 21.504
2011 21.943
2011 21.710
2011 21.842
2011 21.952
2011 21.930
2011 22.224
2011 21.961
2011 22.980
2011 22.695
2011 22.629
2011 22.528
2011 22.492
2011 22.659
...
2019 69.980
2019 70.010
2019 72.390
2019 72.580
2019 72.070
2019 72.160
2019 70.290
2019 68.700
2019 68.790
2019 69.250
2019 71.540
2019 72.800
2019 73.850
2019 75.000
2019 75.350
2019 76.550
2019 75.800
2019 74.720
2019 72.290
2019 72.690
2019 74.220
2019 73.400
2019 77.100
2019 78.600
2019 78.960
2019 79.860
2019 80.200
2019 80.590
2019 81.100
2019 82.030
Freq: A-DEC, Name: close, Length: 2004, dtype: float64
pingan['close'].to_period('A').groupby(level = 0).first().plot()
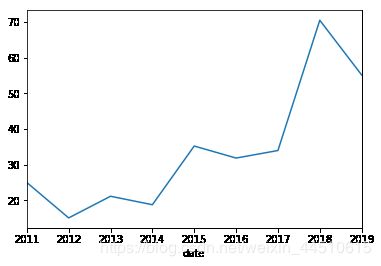
pingan['42d'] = pingan['close'].rolling(window=42).mean()
pingan['250d'] = pingan['close'].rolling(window=250).mean()
pingan[['close','42d','250d']].plot(figsize=(12,8),color = ['y','b','r'])
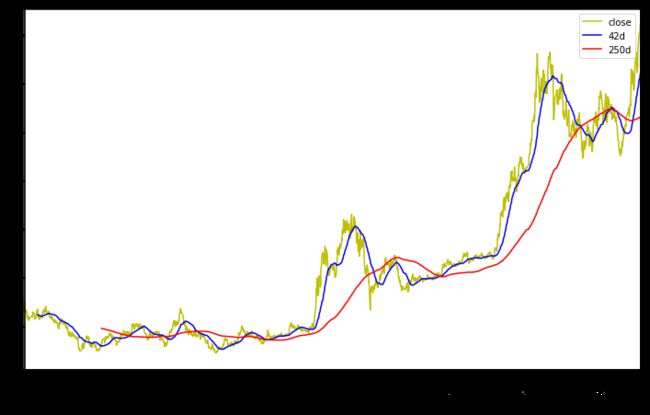
pingan['duishu'] = np.log(pingan['close']/pingan['close'].shift(1))
pingan[['close','duishu']].plot(subplots = True,figsize=(12,12))
array([,
],
dtype=object)
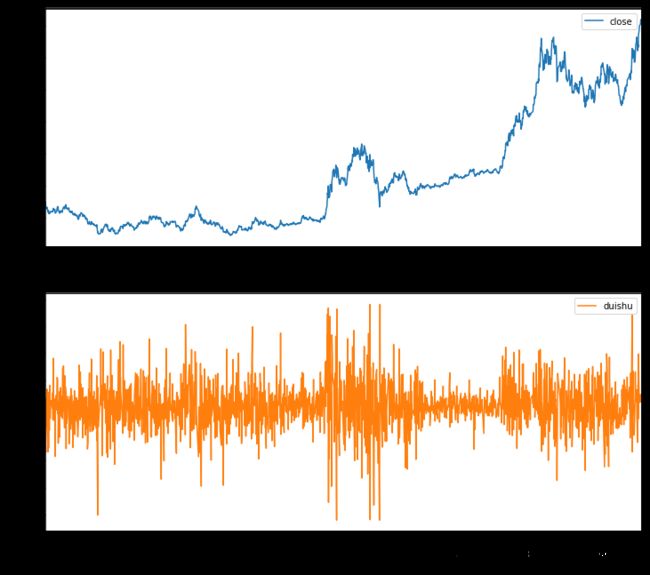